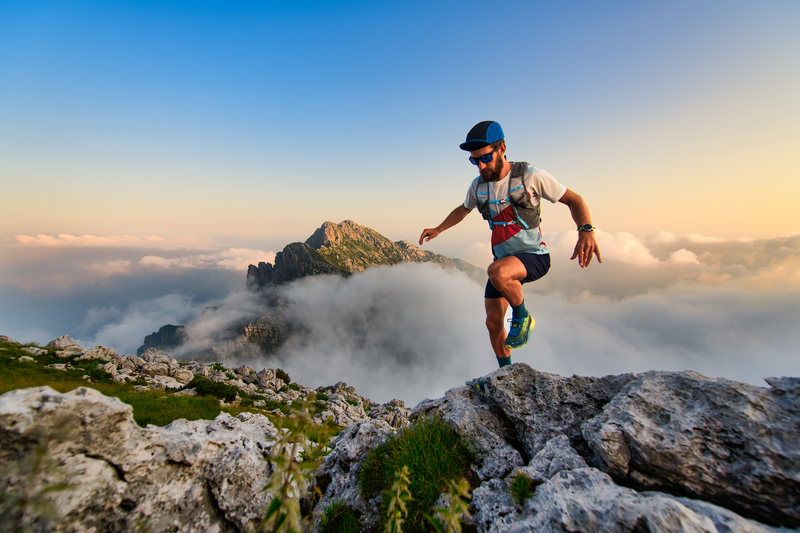
95% of researchers rate our articles as excellent or good
Learn more about the work of our research integrity team to safeguard the quality of each article we publish.
Find out more
ORIGINAL RESEARCH article
Front. Educ. , 22 November 2024
Sec. Higher Education
Volume 9 - 2024 | https://doi.org/10.3389/feduc.2024.1456085
This article is part of the Research Topic Institutional Impact Measurement in Higher Education View all 11 articles
Introduction: Gender stereotypes considerably influence human behavior, creating gaps between men and women in higher education. Society faces the challenge of preventing the loss of human talent in Science, Technology, Engineering, and Mathematics (STEM) disciplines and Social Sciences and Humanities (SSH). However, it remains unclear which factors influence the gender gap that may hinder attracting and retaining female talent in these fields. This study aimed to develop and validate an instrument, the ‘Assessing Gender Gap Factors in Higher Education’ (FACT-GÉN), to identify and measure the factors influencing the gender gap.
Method: This study employed an instrumental design to develop and validate the ‘Assessing Gender Gap Factors in Higher Education’ (FACT-GÉN) instrument. Using a cross-sectional quantitative approach, data were collected from 851 students across STEM and Social Sciences and Humanities (SSH) disciplines to ensure the instrument’s reliability and validity. Internal Consistency Analysis was conducted to assess the reliability of the identified factors, followed by Exploratory Factor Analysis (EFA) to identify underlying structures, and Confirmatory Factor Analysis (CFA) to validate the instrument’s structure.
Results: The model demonstrated a good fit to the data, with indices such as CFI (0.911), TLI (0.894), and RMSEA (0.049), supporting its validity. The scaled chi-square difference test confirmed the adjusted model’s superiority over the null model [χ2_diff(4) = 30.805, p < 0.001]. Internal consistency was acceptable, with Cronbach’s alpha ranging from 0.62 to 0.78, and McDonald’s omega from 0.69 to 0.80. The strong factor loadings and significant correlations between the latent factors validated the model’s structure, making it a reliable tool for addressing the gender gap in academic fields.
Discussion: The validated instrument offers valuable data for research and policy, aiding efforts to mitigate the gender gap and promote equity in academia. This study highlights the instrument’s effectiveness in identifying barriers and formulating solutions for gender equality, thus filling a critical gap with a reliable tool to address the gender gap in higher education.
The gender gap in STEM careers, as well as in the Social Sciences and Humanities, represents a significant issue that affects the social, cultural, and economic development of countries (Huber and Paule Paludkiewicz, 2024). It also hinders progress towards the Sustainable Development Goals (SDGs) of the 2030 Agenda, particularly SDG 4 (quality education), SDG 5 (gender equality), and SDG 10 (reduced inequalities) (ECLAC, 2019). This gap is reflected in the lower presence of women and the higher predominance of men in STEM fields, while in the Social Sciences and Humanities, women predominate and men are underrepresented (World Economic Forum, 2023). The situation is concerning due to the lack of an integrated model that explains the factors influencing the gender gap, considering sociocultural (Olsson et al., 2023; Presler-Marshall et al., 2024), psychological (Bertoletti et al., 2023), cognitive (Kaya, 2023), contextual (Echavarren, 2023), and collaborative aspects (Borgonovi et al., 2023).
As a socializing agent (Nguyen and Truong, 2024), the university environment facilitates interactions between men and women that reinforce gender stereotypes (Tanyildiz, 2023). Stereotypes not only emphasize negative aspects of ability or temperament but also dictate social norms about appropriate or inappropriate activities according to gender roles (Rothermund and de Paula Couto, 2024; del Carmen Triana et al., 2024). Humans internalize gender stereotypes associated with specific disciplinary areas, perpetuating the idea that women are less talented and brilliant than men (Napp and Breda, 2022). Women are perceived as less capable in scientific and technological subjects (Vos et al., 2023), and their abilities and competencies to lead are questioned (Eagly and Wood, 2016). Furthermore, women are associated with service professions, whereas men are linked to agency attributes (Rucker et al., 2018). These stereotypes influence both society’s beliefs (Risman and Davis, 2013) and career choices and professional associations (Eagly and Karau, 2002). They also contribute to the creation of biases related to competencies (Liu, 2018), anxiety (Berkowitz et al., 2015), choices, and interest (Bian et al., 2017). Stereotypes affect behavior as individuals adapt to the expectations that society attributes to them, reinforcing these stereotypes through educational institutions (Adu et al., 2023).
Stereotypes impact society by shaping behaviors, influencing productivity (Ali et al., 2023). This is reflected in the underrepresentation of women in professions considered more demanding by agents, such as high-level leadership positions in corporations or governments (Fellegi et al., 2023), and their overrepresentation in subareas considered more demanding by the community, such as human resource management (Levanon and Grusky, 2016). Additionally, men are underrepresented in communal roles traditionally occupied by women (Moskos, 2020). This neotraditional division of labor perpetuates stereotypical gender beliefs about agency and communion, as well as sex differences in gender identity (Sczesny et al., 2018).
Research on the gender gap has identified various factors that influence gender bias. One of the main factors is the higher self-efficacy observed in male secondary school students compared to their female counterparts (Chan, 2022; Romero and Blanco, 2019). In this regard, the school context plays a crucial role, as it can either reinforce or weaken stereotypes, significantly affecting students’ interest in STEM areas, which tends to decline during secondary school (Legewie and DiPrete, 2014; Salmela-Aro, 2020). There is also a positive relationship between attitudes towards STEM and student interest, influenced by gender and family background, such as having parents in scientific fields or their educational level (Alam et al., 2021; Anaya et al., 2022; Ciftci and Erdogan, 2020). Beliefs about achievement in STEM careers are generally higher in men (Moè et al., 2021), while women tend to experience higher levels of anxiety in STEM exams and underestimate their abilities (Cotner et al., 2020). Additionally, men perceive themselves as more capable in STEM areas from primary education (Ayuso et al., 2021; Master et al., 2017).
In the fields of Social Sciences and Humanities (SSH), the secondary education context also influences the interest in pursuing university careers (Barone and Assirelli, 2020), as well as perceptions of job stability and accessibility (Prakasam and Mukesh, 2019; Presler-Marshall et al., 2024). Other determining factors include academic achievement in secondary education (Alfarhan and Dauletova, 2019), self-image (Gylfason and Zoega, 2021), and attitudes towards disciplinary area choices (Chiu, 2011). In STEM and SSH, the educational context and family background are crucial in shaping attitudes and self-efficacy. However, while gender stereotypes and anxiety affect women more in STEM, SSH decisions are influenced more by perceptions of job stability and self-image.
Most studies have relied on questionnaires, scales, or surveys developed by other institutions. However, significantly fewer studies have designed and validated a psychometric instrument that includes a rigorous process of content and construct validation (Shin et al., 2016). Moreover, the majority of these studies focus on academic performance (Demirtaş et al., 2020; van Aalderen-Smeets et al., 2019), while few examine other factors involved in the gender gap. Additionally, there is no evidence of specific instruments to assess the gender gap in Social Sciences and Humanities fields. Therefore, an instrument is needed to comprehensively measure this gap in such contexts.
This study was guided by the following question: What are the measurable indicators that determine the gender gap in the university population? The primary objective was to design and validate a psychometric instrument to measure the factors contributing to the gender gap across five dimensions: cognitive, psychological, contextual, sociocultural, and collaborative. The cognitive dimension includes perceptions, knowledge, and academic skills; the psychological dimension involves expectations, motivation, and self-perceived ability; the contextual dimension relates to environmental factors within family and educational settings; the sociocultural dimension addresses social interactions and cultural influences on gender roles; and the collaborative dimension focuses on teamwork within university spaces. Together, these dimensions provide a comprehensive framework for understanding the factors influencing the gender gap in higher education (Beroíza-Valenzuela and Salas-Guzmán, 2024).
A quantitative methodology with a descriptive cross-sectional design was employed to achieve these objectives. Structured surveys were designed and applied to representative samples of university students selected through non-probabilistic sampling. The collected data were analyzed using advanced statistical techniques, such as exploratory and confirmatory factor analyses, to ensure the validity and reliability of the instrument.
This study fills an important gap in the current research by providing a comprehensive and multidimensional approach to understanding the gender gap in higher education, which has been underexplored with validated psychometric instruments. The contribution of this study lies in its ability to offer specific and reliable tools to identify and address the underlying factors of the gender gap, facilitating the development of more equitable and effective educational policies and programs. This article is structured as follows: First, the methodological design and sample characteristics are detailed. Next, the results of the data analysis are presented. Finally, a thorough discussion and recommendations for future research and educational policies are provided.
This study is quantitative and has a cross-sectional design. This quantitative approach allows for the systematic collection and statistical analysis of numerical data, thus facilitating an objective evaluation of the instrument’s properties. Cross-sectional studies implement data collection at a single point in time, yielding a precise snapshot of the study population at the time of research. This study focuses on the design and validation of an instrument using Exploratory Factor Analysis (EFA) and Confirmatory Factor Analysis (CFA).
The research will be conducted in three main stages: pre-piloting, piloting, and final implementation. The designed instrument aimed to assess multiple dimensions related to the gender gap in higher education, including cognitive, psychological, contextual, collaborative, and sociocultural aspects. This study followed research protocols approved by the Ethics Committee of the University of Santiago, Chile, N°024, and all stages of the study were conducted in accordance with the Declaration of Helsinki. All participants signed an informed consent form before participating in the study.
Through purposive sampling, three distinct samples were formed in separate phases of the research: an initial sample of 40 undergraduate university students for pre-piloting, a second sample of 307 undergraduate university students for piloting, and a final sample of 504 participants for implementation of the definitive instrument. In all phases, the same inclusion criteria were followed, ensuring that the participants were students over 18 years old and belonged to either public or private universities from Chile. No exclusion criteria were applied because the objective was to generate a representative sample of the general university population. In total, the research involved 851 university students, distributed across three main groups for the various stages of analysis: Pre-piloting, Piloting, and Final Implementation Phase.
The design process of the “Assessing Gender Gap Factors in Higher Education” (FACT-GÉN) instrument followed several systematic stages, divided into two studies. The first study focused on construct development and included the following activities:
• Literature Review: A comprehensive review of the existing literature was conducted to identify relevant constructs related to the gender gap in higher education.
• Expert Interviews: Qualitative interviews were conducted with experts in higher education and gender studies to gain insights and validate the relevance of the proposed items.
• Delphi Method: Psychometric experts reviewed and refined the items to ensure their clarity and validity.
The quantitative phase encompasses three distinct stages: pre-piloting, piloting, and final implementation. To that end, university students were sourced from a variety of channels, including social media platforms and connections with public and private universities, such as the gender and equity departments of these institutions. It should be emphasized that considering that the primary aim of this study is the development and validation of a psychometric tool, the qualitative aspect of the instrument design process will not be delved into.
A draft of the instrument was administered to 40 university students. Subsequently, a discussion was held to identify and improve the ambiguous aspects. Finally, three students were randomly selected for cognitive interviews to evaluate in-depth their understanding of the questions and the appropriateness of the responses provided. During these cognitive interviews, the students were asked to verbalize their thoughts while responding to each instrument item. This process allowed for the identification of potential misunderstandings, difficulties in interpreting the questions, and suggestions for improving the wording and clarity of the instrument. As a result, items were identified for revision and reformulation, simplified, and modified for vocabulary that was incomprehensible to the students.
Pilot tests were conducted with a preliminary sample to assess the clarity and comprehensibility of the items and to calculate the initial internal consistency of the instrument. Using a sample of 307 participants, an Exploratory Factor Analysis (EFA) was performed to identify the underlying structures of the instrument and group the items into coherent factors.
An independent sample of 504 participants was used to conduct a Confirmatory Factor Analysis (CFA) to validate and confirm the factor structure identified during the EFA, evaluating the model’s fit to the collected data.
The psychometric instrument “Assessing Gender Gap Factors in Higher Education” (FACT-GÉN) was designed and administered online, including specific items to measure cognitive, psychological, contextual, collaborative, and sociocultural dimensions. Additionally, both the pilot survey and the final survey included sociodemographic questions focused on sex, gender, age, university, and OECD disciplines, aiming to contextualize participants’ responses and allow for additional analyses.
The design and validation of the instrument included an initial content validation phase in which items were developed based on semi-structured interviews with experts in key areas of the gender gap in the university population. Subsequently, the Delphi method was used, involving nine experts in instrument validation and gender specialists. They were presented with a form containing the draft instrument and evaluation criteria (sufficiency, clarity, coherence, and relevance). The instrument’s subscales corresponded to five constructs: Psychological, Contextual, Sociocultural, Cognitive, and Collaborative, and a rating scale ranging from “strongly agree (5)” to “strongly disagree (1)” was used. After successive revisions guided by experts’ opinions, adjustments were made to the instrument. After three rounds of iterations, the concordance of the responses was evaluated using Cohen’s kappa coefficient, which showed a value of 0.85, indicating a high degree of concordance among the experts.
The complete statistical analysis was performed using RStudio 4.3.1 (Wickham et al., 2023).
The analyses included:
• Exploratory Factor Analysis (EFA) to identify underlying structures.
• Confirmatory Factor Analysis (CFA) was used to validate the instrument’s structure.
• Internal Consistency Analysis to assess the reliability of the identified factors.
The instrument’s structure was evaluated using a mixed approach of confirmatory factor analysis (CFA) and exploratory factor analysis (EFA). Due to the multidimensional nature of this instrument, Parallel Analysis and Optimal Coordinates methods were used to determine the number of factors to extract. Given the non-normality of the data, Principal Axis Factoring was chosen over Maximum Likelihood as the extraction method. The EFA was estimated using 100 iterations of the naïve bootstrap, reporting the mean loadings and 90% confidence intervals. This procedure was implemented iteratively by removing cross-loadings (loadings on more than one factor) and low loadings (loadings in the range of −0.3 to +0.3).
Simultaneously, the CFA structure was evaluated to identify weaker elements by assessing the residuals and local fit (R2). Both procedures were conducted with careful consideration of the theoretical constructs and the relevance of item loadings to each construct. Model fit was evaluated using the Comparative Fit Index (CFI), Tucker-Lewis Index (TLI), Root Mean Square Error of Approximation (RMSEA), and Standardized Root Mean Square Residual (SRMR). Once the final structure was established, reliability was assessed through internal consistency analysis using Cronbach’s alpha (Cronbach, 1951), with values above 0.7 considered adequate (Kline, 2016). Additionally, due to critiques of Cronbach’s alpha, McDonald’s Omega was employed (Hayes and Coutts, 2020).
A pilot survey was conducted, which included sociodemographic aspects such as age, gender, sex, and university, as well as the psychometric instrument on university students’ perceptions of the factors influencing the gender gap across five dimensions: cognitive, psychological, contextual, collaborative, and sociocultural, with the aim of conducting Exploratory Factor Analysis (EFA). The sample consisted of 307 participants, had a Mage = 22.5 years (SD = 3.34). In terms of sex, 73.6% identified as women, 26% as men, and 0.4% as other. Regarding gender, 65% identified as female, 24% as male, 7% as non-binary, and 4% as other. The age distribution showed that 32.57% of participants were 18–20 years old, 46.57% were 21–24, 12.71% were 25–29, and 8.15% were 30 or older. Based on OECD disciplinary classifications, 9% of participants were from the Natural Sciences, 14% from Engineering and Technology, 9% from Medical and Health Sciences, 1% from Agricultural Sciences, 46% from Social Sciences, and 22% from the Humanities. Additionally, 72% of participants attended public universities, while 28% were enrolled in private institutions.
Principal component factor analysis with varimax rotation revealed the existence of six factors. (See Figure 1).
The scree plot (Figure 1), showing the eigenvalues obtained from the exploratory factor analysis, revealed that the first six factors explained most of the variance in the data. Initially, the slope of the plot descends steeply to the third factor, suggesting that these principal factors capture a significant amount of variance. From the fourth factor onwards, the slope began to flatten, and a second notable inflection was observed at the sixth factor, where the curve became almost flat. This indicates that additional factors beyond the sixth factor explain only a marginal amount of the additional variance. Therefore, it was determined that retaining six factors is optimal for this analysis, providing a suitable balance between complexity and explanatory power.
The retention of the six factors was justified by both empirical and theoretical criteria. The scree plot showed a steep decline in eigenvalues up to the third factor, followed by gradual flattening up to the sixth factor, where a significant inflection point was observed, suggesting that retaining more factors would add minimal explanatory value. This finding aligns with Field (2017) recommendation to identify inflection points in the scree plots. Additionally, sample adequacy, indicated by a KMO index of 0.81, and a highly significant Bartlett’s test of sphericity (χ2 = 5527.075, p < 0.0001), supports the suitability of the data for factor analysis. Together, these six factors explain 35.4% of the total variance, which is a reasonable value in educational and psychological research (Hair et al., 2014). This number of factors strikes a balance between model complexity and explanatory power, ensuring a parsimonious and reliable factor structure with satisfactory internal consistency (α = 0.75). Furthermore, the factor structure obtained was consistent with prior studies that addressed similar conceptual dimensions, reinforcing the relevance of the identified factors.
Exploratory factor analysis grouped the dimensions as follows (Figure 2):
Factor analysis revealed an item organization that differs in certain aspects from the originally proposed factor structure, allowing for greater precision in the empirical representation of the data. While five theoretical factors were initially established (Cognitive, Psychological, Contextual, Sociocultural, and Collaborative), the factor analysis indicated the need to reorganize these factors into six dimensions: Coping in University Students, Academic Beliefs, Family Academic Support, University Socialization, Collaborative Learning Experience, and Gender Role Beliefs. This reorganization reflects how the items are empirically grouped, optimizing the clarity and internal consistency of each dimension. Although the items were distributed differently from the original proposal, these dimensions more accurately captured the constructs underlying the original factors, providing a better explanation of the variance and a more robust structure for measuring the factors influencing the gender gap in higher education. Therefore, restructuring does not invalidate the theoretical factors, but rather offers a more detailed and accurate representation of the observed empirical reality.
Table 1 displays the detailed results of the EFA, showing the standardized factor loadings, internal consistency (α), and item-total correlation for each of the 30 items in the instrument. Noteworthy are the significant factor loadings and acceptable internal consistency of the factors, which support the validity and reliability of the instrument for measuring the proposed constructs. After the analysis, items with low factor loadings were removed from the initial 50 items, resulting in a 30-item instrument (Table 1).
The heatmap shows the factor loadings of the items on the six retained factors after performing exploratory factor analysis (EFA) with varimax rotation. The results in Table 1 complemented the heatmap by showing the factor loadings, Cronbach’s alpha, and item-total correlation (r.drop). Items 39, 33, and 48, which had high factor loadings on their respective factors, also demonstrated adequate internal consistency within their scales. Items with low factor loadings or negative item-total correlations, such as Items 27 and 48, were identified for review or elimination, which will help improve the internal consistency and validity of the questionnaire.
Based on the EFA, the model was executed on a sample of N = 504. Model fit was evaluated using CFI, TLI, and RMSEA. A 30-item instrument was implemented in a different sample of N = 554 university students, considering the previously mentioned sociodemographic aspects. In this different sample, the demographic characteristics had a Mage = 21.14 years (SD = 2.67), and 63% were women. Regarding the gender of the participants, 58% were identified as female, 35% as male, and 5% as non-binary. Participants came from public (27.7%) and private universities (72.7%) in the Metropolitan Region of Santiago, Chile. The distribution by OECD disciplines was represented, but the majority participation was from Social Sciences (33%) and Engineering and Technology (29%) (Table 2).
To evaluate the quality of the models examined through both EFA and CFA, the following indicators were used: Comparative Fit Index (CFI), Tucker-Lewis Index (TLI), and Root Mean Square Error of Approximation (RMSEA), along with its 90% confidence interval. In the present study, the fit of a structural model was evaluated using a robust maximum likelihood estimator (MLR) because of the non-normal multivariate distribution of the data. The proposed model included six latent factors: Factor1 (items 14, 18, 19, 39), Factor2 (items 2, 4, 5, 7), Factor3 (items 43, 48, 49, 44), Factor4 (items 10, 22, 36, 23), Factor5 (items 11, 12, 13, 6), and Factor6 (items 32, 33, 34). Additional covariances were specified between items (item14 ~ ~ item18, item19 ~ ~ item39, item2 ~ ~ item4, item48 ~ ~ item49) based on theory and previous results. Table 3 presents descriptive statistics of the items.
The model fit indices indicated a good fit to the data: the Comparative Fit Index (CFI) was 0.911 (robust CFI: 0.916), the Tucker-Lewis Index (TLI) was 0.894 (robust TLI: 0.899), and the Root Mean Square Error of Approximation (RMSEA) was 0.049 (90% CI: 0.043–0.054, p-value = 0.640; robust RMSEA: 0.047, 90% CI: 0.041–0.053, p-value = 0.768). Additionally, the normed chi-squared (χ2/df) value of the model was 2.31.
To assess the improvement in the proposed model fit over a null independence model, a scaled chi-square difference test was performed using the Satorra-Bentler method. The results indicated a significant difference between the two models [χ2_diff(4) = 30.805, p < 0.001], with the adjusted model showing a chi-square of 488.13 (df = 211) and the null model showing a chi-square of 3383.611 (df = 253). This suggests that the proposed model provides a significantly better fit to the data than the null model. This indicates that the adjusted model not only fits the data well but is also statistically superior to the null model, corroborating its validity.
To evaluate the internal consistency of the latent factors, Cronbach’s alpha was calculated for each factor and the total set of items. Some items were negatively correlated with the first principal component and were reversed. The Cronbach’s alpha values for each factor were as follows: Factor 1 (α = 0.72), Factor 2 (α = 0.72), Factor 3 (α = 0.62), Factor 4 (α = 0.68), Factor 5 (α = 0.72), and Factor 6 (α = 0.78). Cronbach’s alpha for the total set of items was 0.74, indicating an overall acceptable internal consistency for a new model.
Meanwhile, McDonald’s omega coefficient values for each factor were as follows: Factor 1 (ω = 0.80), Factor 2 (ω = 0.76), Factor 3 (ω = 0.69), Factor 4 (ω = 0.73), Factor 5 (ω = 0.77), and Factor 6 (ω = 0.80). The omega coefficient for the total set of items was 0.77, indicating acceptable internal consistency.
The path diagram (Figure 3) represents the factor structure, relationships between latent factors, and observed variables using the robust maximum likelihood estimator (MLR). The model includes six latent factors (Factor 1 to Factor 6), each measured by several observed items. Standardized factor loadings indicate the strength of the relationship between each observed item and its respective latent factor. For example, i36 has a factor loading of 0.93 on Factor4, indicating a strong and significant relationship. All factor loadings were positive and significant, suggesting that the items were good indicators of their respective factors.
The residual covariances specified in the model, represented by the curved lines between certain items, indicate additional relationships between measurement errors. For example, the covariance between i14 and i18 was −0.37, suggesting a negative residual relationship between these items. The standardized correlations between latent factors, such as the correlation of 0.23 between Factor3 and Factor2, indicate moderate positive relationships, suggesting that the latent constructs are interrelated.
The global fit indices of the model indicated an adequate fit. The Comparative Fit Index (CFI) and the Tucker-Lewis Index (TLI) have values of 0.91 and 0.89, respectively, indicating an acceptable fit. Additionally, the Root Mean Square Error of Approximation (RMSEA) was 0.046, indicating a good fit of the model. Together, these results confirm the validity of the proposed model, showing significant and adequate relationships between the observed items and latent factors, as well as between the latent factors themselves. The high factor loadings and significant correlations between the factors support the theoretical structure of the model, suggesting that it is suitable for the analyzed data.
The findings of this study reveal critical insights into the specific indicators that perpetuate and fuel the gender gap in the university population, providing an analysis that identifies six dimensions: Coping in University Students (O’Rourke et al., 2022), Academic Beliefs (Yim, 2023), Family Academic Support (Flanagan-Bórquez and Soriano-Soriano, 2024), University Socialization (Demir and Leyendecker, 2018), Collaborative Learning Experience (Woolley and Gupta, 2024), and Gender Role Beliefs (Rucker et al., 2018), highlighting the relevance of a multidimensional perspective. The interpretation of these results not only enriches the existing literature on gender inequalities in higher education but also offers a solid empirical basis for designing effective, innovative, and targeted interventions for populations in STEM, Social Sciences, and Humanities.
One of the aspects highlighted by this study is that the model distinguished between the direct effect of family academic support and the effect of university socialization within contextual dimensions. Regarding the family factor, previous research has emphasized that the family context and the stereotypes that arise and are reinforced by parents influence students’ choices (Alam et al., 2021; Anaya et al., 2022; Cian et al., 2022; Ciftci and Erdogan, 2020; Li et al., 2022). These studies have shown that parents’ expectations and attitudes toward gender roles can limit students’ academic aspirations, especially those of women (Maloshonok et al., 2022), affecting their confidence and motivation to pursue careers in male-dominated fields (Salmela-Aro, 2020).
On the other hand, the educational context also plays a crucial role in shaping students’ academic and professional identities (Carvalho Silva et al., 2024). Interactions with teachers, peers, and the curriculum can reinforce or challenge established gender stereotypes (Stringer, et al., 2020). Similar to the family factor, the adverse effects of the educational context are particularly detrimental to women, limiting their opportunities and perpetuating gender inequalities (Merma-Molina et al., 2021). These negative effects can manifest in various ways, such as the lack of female representation in specific fields of study, implicit bias in teachers’ expectations (Woo et al., 2023), and the lack of institutional support for female students facing gender discrimination.
The consideration of the effects of the family and educational contexts is crucial for designing effective interventions (Fernández et al., 2023), as both aspects can contribute to gender disparities. While educational policies should focus on creating inclusive and supportive environments within educational institutions (Scala and Paterson, 2018), interventions at the family level should aim to educate parents about the impacts of gender stereotypes and promote more equitable attitudes and expectations toward their children’s abilities and aspirations (Starr et al., 2022). By addressing both contexts comprehensively, more effective strategies can be developed to close the gender gap in higher education.
Regarding the coping in university students dimension, the family can also influence the generation of emotional states such as anxiety (Chen et al., 2022) in university students. Anxiety induced by family expectations, pressure to meet high academic standards, and a lack of adequate emotional support can negatively affect students’ psychological well-being. These emotional states not only impact their mental health but also interfere with their academic performance, reducing their ability to concentrate, actively participate in class, and manage stress related to their studies (Barbayannis et al., 2022; Sumeera et al., 2024). Additionally, anxiety can limit students’ ability to form social support networks within the university environment, which is crucial for their personal and professional development (Pascoe et al., 2020). Therefore, it is essential that interventions designed to address the gender gap in higher education include strategies to mitigate the negative effects of family-induced anxiety, promoting a supportive environment both at home and at the university. It should be noted that global mental health post-pandemic has shown an increase in these issues among the university population (Lipson et al., 2022; Santomauro et al., 2021), with elevated levels of anxiety and depression (Ghanim et al., 2022; Hauck et al., 2022), suicidal ideation (Frajerman et al., 2022), stress, and fear (Azmi et al., 2022), as well as emotional impacts on learning (Pelucio et al., 2022), which affects students’ academic performance (El-Gabry et al., 2022).
The collaborative learning experience dimension in higher education also plays a crucial role. To thrive in these environments, it is essential to promote a sense of belonging and facilitate group interactions (Micari and Pazos, 2021). This not only fosters a more inclusive environment but also strengthens support networks among students (Schmiedl and Kauffeld, 2023; Wissing et al., 2022), thereby improving their educational experience and academic performance (Owoc, 2022; Rae and Blenker, 2023). These elements can counteract competitive dynamics (Al-Enazi and Jadea, 2022) and create a space where university students feel supported and motivated to actively participate in their careers. Collaboration in university careers is key to promoting diversity and equality in fields where women are traditionally underrepresented, such as STEM (Nielsen et al., 2017) and HSS (Wishkoski et al., 2022).
Gender-diverse teams promote innovation and creativity (Yang et al., 2022). Human beings depend on their peers to achieve collective goals, develop metacognitive skills, and interdependent processes that facilitate decision-making (Gupta and Woolley, 2020). Collaboration fosters collective intelligence and social skills such as empathy, self-control, efficacy, assertiveness, and effective communication (Woolley and Gupta, 2024). However, the gender gap limits these skills and self-perceptions, as in inequitable contexts, people may feel insecure and reluctant to express their opinions (Woolley et al., 2023).
The gender role beliefs dimension, such as exclusionary norms and beliefs, presents complex challenges to change (Leibnitz et al., 2022). These challenges require strategic and sustained interventions to dismantle entrenched structures and prejudices that limit full integration and participation in these fields (Parvazian et al., 2017). Sociocultural factors centered on biased beliefs that perpetuate unequal behaviors and do not promote inclusive environments significantly slow down the progress of discovery, creativity, and innovation. These factors artificially exclude individuals with valuable abilities and skills (Sulik et al., 2022), preventing them from making meaningful contributions to the national knowledge base.
Academic beliefs related to beliefs about individuals’ abilities and skills play a fundamental role in perpetuating the gender gap (Cvencek et al., 2020). These beliefs significantly influence self-perception and academic and professional expectations, restricting the development and participation of certain groups in various disciplines (Chaffee et al., 2024). Students who internalize these limiting beliefs are less likely to engage in challenging academic activities, seek leadership opportunities, or pursue advanced studies. This self-exclusion, in turn, reinforces the gender gap by perpetuating the under-representation of certain groups in specific academic and professional fields.
Understanding the gender gap from a multidimensional perspective is a comprehensive and necessary approach. It is crucial not only to analyze the individual impact of Coping in University Students, Academic Beliefs, Family Academic Support, University Socialization, Collaborative Learning Experience, and Gender Role Beliefs dimensions but also to understand how these factors interrelate and reinforce each other, exacerbating gender inequalities in higher education. Exclusionary sociocultural norms and beliefs create biased power dynamics that hinder equitable collaboration in the educational sphere, an effect that originates from family and educational contextual factors. A family environment that perpetuates gender stereotypes can limit women’s academic aspirations and negatively affect their performance and motivation. Similarly, an educational environment that does not foster inclusion and equity can amplify negative messages received at home, perpetuate gender inequalities, and foster self-limiting beliefs about one’s capabilities. These beliefs can trigger emotional states, such as anxiety and stress, especially in unequal environments. Therefore, an integrated approach is essential for developing strategies addressing the multiple dimensions of the gender gap and promoting greater equity in academia.
The decision to use predominantly positive items in the Family Academic Support and Collaborative Learning Experience factors was deliberate and aimed at capturing supportive and collaborative experiences more accurately. This strategy helped to avoid cognitive confusion among participants, which could have arisen from introducing negatively worded items into factors that are typically described positively (Schriesheim and Hill, 1981). However, for factors such as gender bias, using negatively worded items was considered more appropriate, as these aim to assess experiences of discrimination or perceived difficulties, aligning better with this approach (Tourangeau and Yan, 2007).
The practical implications of this study are particularly relevant to the utility of the developed psychometric instrument. This instrument provides educational institutions with a reliable and validated tool to measure the factors contributing to the gender gap in higher education. This allows educational policymakers and university administrators to identify specific areas for intervention and develop more effective and targeted support programs. Additionally, the psychometric instrument has the potential to assess the impact of existing initiatives aimed at reducing the gender gap, providing empirical data on their effectiveness and areas requiring improvement. This evaluation capability is crucial for formulating evidence-based policies that promote gender equality and inclusion in the university environment.
By delineating the factors that contribute to the gender gap in specific dimensions (Coping in University Students, Academic Beliefs, Family Academic Support, University Socialization, Collaborative Learning Experience, and Gender Role Beliefs), the instrument facilitates a more nuanced and comprehensive analysis. This enables the identification of female students’ specific needs, thereby allowing for the implementation of tailored strategies that support their academic and professional development. This instrument has the potential to transform how educational institutions address gender inequalities, providing a systematic approach to fostering a more equitable and inclusive educational environment.
A limitation of this study is the inherent biases associated with self-report measures, such as social desirability and biased self-assessment, which are well-documented challenges in educational and psychological research. Social norms or subjective perceptions may influence participants’ responses. To mitigate these biases, we implemented strategies such as ensuring participant anonymity and carefully constructing the questions to minimize socially guided responses. However, future research should incorporate objective measures, such as data on enrollment, retention, and graduation rates, to complement self-reports and provide a more comprehensive view of gender-related differences in educational contexts.
Additionally, this study did not include criterion analysis, which is crucial for assessing the predictive validity of the instrument and its correlation with other relevant constructs related to the gender gap in higher education. Since the primary aim of this study was to develop and validate the instrument, criterion analysis was beyond the scope of the present work. Future studies are encouraged to include this analysis to determine the instrument’s ability to predict outcomes related to the gender gap, such as academic and professional behaviors.
Moreover, the sample in this study was not evenly distributed across OECD disciplines, which may limit the generalizability of the findings. Future research should strive for a more balanced representation of fields to strengthen the validity of the results and enhance the instrument’s applicability in diverse academic and professional settings. By addressing these limitations, subsequent studies will be better positioned to offer a robust validation of the instrument and its capacity to predict gender-related outcomes.
The data that support the findings of this study are available from the corresponding author upon reasonable request. Due to privacy or ethical restrictions, certain portions of the data may be de-identified to protect participant confidentiality. The FACT-GÉN instrument is available to researchers conducting gender studies and can be accessed upon request to facilitate replication and further research on gender disparities in higher education.
The studies involving humans were approved by Ethics Committee of the Universidad de Santiago, Chile. The studies were conducted in accordance with the local legislation and institutional requirements. The participants provided their written informed consent to participate in this study.
FB-V: Conceptualization, Data curation, Formal analysis, Funding acquisition, Investigation, Methodology, Project administration, Resources, Software, Supervision, Validation, Visualization, Writing – original draft, Writing – review & editing. NS-G: Funding acquisition, Resources, Visualization, Writing – review & editing. DH: Funding acquisition, Resources, Writing – review & editing.
The author(s) declare financial support was received for the research, authorship, and/or publication of this article. This research was funded by the National Doctoral Scholarship Program 2022–2024 of the National Agency for Research and Development of Chile (ANID No. 21220061; GOP No. 242230011) and by Fondecyt No. 1231117, Project ‘El rol de los determinantes sociales de la salud en las habilidades socioemocionales en contextos socialmente vulnerables: una aproximación desde la neurociencia social utilizando EEG Móvil’. ANID, Chile.
The authors declare that the research was conducted in the absence of any commercial or financial relationships that could be construed as a potential conflict of interest.
All claims expressed in this article are solely those of the authors and do not necessarily represent those of their affiliated organizations, or those of the publisher, the editors and the reviewers. Any product that may be evaluated in this article, or claim that may be made by its manufacturer, is not guaranteed or endorsed by the publisher.
The Supplementary material for this article can be found online at: https://www.frontiersin.org/articles/10.3389/feduc.2024.1456085/full#supplementary-material
Adu, A., Yusif, B. D., Senyametor, F., Amponsah, M. O., and Sefa, F. O. (2023). Stereotyping, prejudice, collective self esteem and academic achievement of distance education and regular students. J. Int. Compar. Educ. 12, 103–121. doi: 10.14425/jice.2023.12.2.0612
Alam, M. S., Sajid, S., Kok, J. K., Rahman, M., and Amin, A. (2021). Factors that influence high school female students’ intentions to pursue science, technology, engineering and mathematics (STEM) education in Malaysia. Pertanika J. Soc. Sci. Human. 29, 839–867. doi: 10.47836/pjssh.29.2.06
Al-Enazi, A. A., and Jadea, W. M. A. (2022). The competitive behavior of university students and its relation to some variables. J. Educ. Teach. Train. 13, 260–269. doi: 10.47750/jett.2022.13.04.035
Alfarhan, U. F., and Dauletova, V. (2019). Revisiting the gender academic achievement gap: evidence from a unique environment. Gend. Educ. 31, 827–848. doi: 10.1080/09540253.2017.1324129
Ali, M., Shin, D., and Yang, Y. (2023). The impact of management gender diversity on productivity: the contextual role of industry gender composition. Aust. J. Manag :03128962231184664. doi: 10.1177/03128962231184664
Anaya, L., Stafford, F., and Zamarro, G. (2022). Gender gaps in math performance, perceived mathematical ability and college STEM education: the role of parental occupation. Educ. Econ. 30, 113–128. doi: 10.1080/09645292.2021.1974344
Ayuso, N., Fillola, E., Masia, B., Murillo, A. C., Trillo-Lado, R., Baldassarri, S., et al. (2021). Gender gap in STEM: a cross-sectional study of primary school students’ self-perception and test anxiety in mathematics. IEEE Trans. Educ. 64, 40–49. doi: 10.1109/TE.2020.3004075
Azmi, F. M., Khan, H. N., and Azmi, A. M. (2022). The impact of virtual learning on students’ educational behavior and pervasiveness of depression among university students due to the COVID-19 pandemic. Glob. Health 18:70. doi: 10.1186/s12992-022-00863-z
Barbayannis, G., Bandari, M., Zheng, X., Baquerizo, H., Pecor, K. W., and Ming, X. (2022). Academic stress and mental well-being in college students: correlations, affected groups, and COVID-19. Front. Psychol. 13:886344. doi: 10.3389/fpsyg.2022.886344
Barone, C., and Assirelli, G. (2020). Gender segregation in higher education: an empirical test of seven explanations. High. Educ. 79, 55–78. doi: 10.1007/s10734-019-00396-2
Berkowitz, T., Schaeffer, M. W., Maloney, E. A., Peterson, L., Gregor, C., Levine, S. C., et al. (2015). Math at home adds up to achievement in school. Science. 350, 196–198. doi: 10.1126/science.aac7427
Beroíza-Valenzuela, F., and Salas-Guzmán, N. (2024). Redefining academic trajectories: a comprehensive analysis of the factors and impacts of the gender gap in STEM higher education. Int. J. Sustain. High. Educ. doi: 10.1108/IJSHE-02-2024-0129
Bertoletti, A., Biagi, F., Di Pietro, G., and Karpiński, Z. (2023). The effect of the COVID-19 disruption on the gender gap in students’ performance: a cross-country analysis. Large Scale Assess. Educ. 11:6. doi: 10.1186/s40536-023-00154-y
Bian, L., Leslie, S.-J., and Cimpian, A. (2017). Gender stereotypes about intellectual ability emerge early and influence children’s interests. Science (New York, N.Y.) 355, 389–391. doi: 10.1126/science.aah6524
Borgonovi, F., Han, S. W., and Greiff, S. (2023). Gender differences in collaborative problem-solving skills in a cross-country perspective. J. Educ. Psychol. 115, 747–766. doi: 10.1037/edu0000788
Carvalho Silva, R., Vezzoli, M., Menesello, V., Meattini, M., Sartori, R., and Minelli, A. (2024). Everything changes but nothing changes: gender stereotypes in the Italian population. Arch. Womens Ment. Health 27, 537–545. doi: 10.1007/s00737-024-01437-1
Chaffee, K. E., Plante, I., Good, C., Aronson, J. M., Kinch, S.-B., and Gauvin, I. (2024). When stereotypes disadvantage boys: strength of stereotypes in mathematics and language arts and their relations with grades. J. Appl. Soc. Psychol. 54, 71–82. doi: 10.1111/jasp.13013
Chan, R. C. H. (2022). A social cognitive perspective on gender disparities in self-efficacy, interest, and aspirations in science, technology, engineering, and mathematics (STEM): the influence of cultural and gender norms. Int. J. STEM Educ. 9:37. doi: 10.1186/s40594-022-00352-0
Chen, G., Oubibi, M., Liang, A., and Zhou, Y. (2022). Parents? Educational anxiety under the? Double reduction? Policy based on the family and students personal factors. Psychol. Res. Behav. Manag. 15, 2067–2082. doi: 10.2147/PRBM.S370339
Chiu, M. (2011). Effects of a Women-in-Sciences/Men-in-Humanities Intervention on Taiwanese Adolescents’ Attitudes Towards Learning Science. The Asia-Pacific Education Researcher 20.
Cian, H., Dou, R., Castro, S., Palma-D’souza, E., and Martinez, A. (2022). Facilitating marginalized youths’ identification with STEM through everyday science talk: the critical role of parental caregivers. Sci. Educ. 106, 57–87. doi: 10.1002/sce.21688
Ciftci, A., and Erdogan, M. S. T. (2020). Gender gap and career choices in STEM education: Turkey sample. Int. J. Progress. Educ. 16, 53–66. doi: 10.29329/ijpe.2020.248.4
Cotner, S., Jeno, L. M., Walker, J. D., Jørgensen, C., and Vandvik, V. (2020). Gender gaps in the performance of Norwegian biology students: the roles of test anxiety and science confidence. Int. J. STEM Educ. 7:55. doi: 10.1186/s40594-020-00252-1
Cronbach, L. J. (1951). Coefficient alpha and the internal structure of tests. Psychometrika 16, 297–334. doi: 10.1007/BF02310555
Cvencek, D., Paz-Albo, J., Master, A., Herranz Llácer, C. V., Hervás-Escobar, A., and Meltzoff, A. N. (2020). Math is for me: a Field intervention to strengthen math self-concepts in Spanish-speaking 3rd grade children. Front. Psychol. 11:593995. doi: 10.3389/fpsyg.2020.593995
del Carmen Triana, M., Song, R., Um, C. T., and Huang, L. (2024). Stereotypical Perception in Management: A Review and Expansion of Role Congruity Theory. J. Manag. 50:188–215. doi: 10.1177/02311149206380836
Demir, M., and Leyendecker, B. (2018). School-related social support is associated with school engagement, self-competence and health-related quality of life (HRQoL) in Turkish immigrant students. Front. Educ. 3:83. doi: 10.3389/feduc.2018.00083
Demirtaş, C., Arıkan, S., and Muğaloğlu, E. Z. (2020). Adaptation of the self-efficacy beliefs in STEM education scale and testing measurement invariance across groups. J. Measure. Eval. Educ. Psychol. 11, 163–179. doi: 10.21031/epod.675240
Eagly, A. H., and Karau, S. J. (2002). Role congruity theory of prejudice toward female leaders. Psychol. Rev. 109, 573–598. doi: 10.1037/0033-295X.109.3.573
Eagly, A. H., and Wood, W. (2016). Social role theory of sex differences. In The Wiley Blackwell Encyclopedia of Gender and Sexuality Studies (Eds.) N. A. Naples, R. C. Hoogland, M. Wickramasinghe, & W. C. A. Wong. (John Wiley & Sons, Ltd., Chichester, UK.), 1–3.
Echavarren, J. M. (2023). The gender gap in environmental concern: support for an ecofeminist perspective and the role of gender egalitarian attitudes. Sex Roles 89, 610–623. doi: 10.1007/s11199-023-01397-3
ECLAC. (2019). The 2030 agenda and the sustainable development goals: An opportunity for Latin America and the Caribbean. Goals, targets and global indicators. Available at: https://hdl.handle.net/11362/40156 (Accessed January 7, 2019).
El-Gabry, D. A., Okasha, T., Shaker, N., Elserafy, D., Yehia, M., Aziz, K. A., et al. (2022). Mental health and wellbeing among Egyptian medical students: a cross-sectional study. Middle East Current Psychiatry 29:25. doi: 10.1186/s43045-022-00193-1
Fellegi, Z., Hrbková, L., and Dubrow, J. (2023). Women’s political power: global progress, persistent challenges. Women’s Stud. Int. Forum 101:102818. doi: 10.1016/j.wsif.2023.102818
Fernández, D. P., Ryan, M. K., and Begeny, C. T. (2023). Gender expectations, socioeconomic inequalities and definitions of career success: a qualitative study with university students. PLoS One 18:e0281967. doi: 10.1371/journal.pone.0281967
Field, A. (2017). Discovering statistics using IBM SPSS statistics: North American Edition. Thousand Oaks, CA: SAGE Publications.
Flanagan-Bórquez, A., and Soriano-Soriano, G. (2024). Family and higher education: developing a comprehensive framework of parents’ support and expectations of first-generation students. Front. Educ. 9, 1–11. doi: 10.3389/feduc.2024.1416191
Frajerman, A., Chaumette, B., Krebs, M.-O., and Morvan, Y. (2022). Mental health in medical, dental and pharmacy students: a cross-sectional study. J. Affect. Disord. Rep. 10:100404. doi: 10.1016/j.jadr.2022.100404
Ghanim, M., Rabayaa, M., Atout, S., Al-Othman, N., and Alqub, M. (2022). Prevalence of anxiety and depression among Palestinian university students: a cross-sectional study during COVID-19 pandemic. Middle East Curr. Psychiatry 29:71. doi: 10.1186/s43045-022-00238-5
Gupta, P., and Woolley, A. W. (2020). The Emergence of Collective Intelligence Behavior. In Proceedings of the Paper presented at the 8thACM Collective Intelligence (CI) Conference, Virtual Event, Zurich, Switzerland.
Gylfason, G., and Zoega, G. (2021). Um pilta og stúlkur í íslenska menntakerfinu. Tímarit Uppeldi Menntun 30, 135–165. doi: 10.24270/tuuom.2021.30.11
Hair, J. F., Black, W. C., Anderson, R. E., and Babin, B. J. (2014). Multivariate Data Analysis. (7th ed.). Pearson Education, Upper Saddle River, NJ, USA.
Hauck, A., Michael, T., and Ferreira de Sá, D. S. (2022). Fear learning and generalization during pandemic fear: how COVID-19-related anxiety affects classical fear conditioning with traumatic film clips. J. Psychiatr. Res. 155, 90–99. doi: 10.1016/j.jpsychires.2022.07.068
Hayes, A. F., and Coutts, J. J. (2020). Use omega rather than Cronbach’s alpha for estimating reliability. But…. Commun. Methods Meas. 14, 1–24. doi: 10.1080/19312458.2020.1718629
Huber, S. J., and Paule-Paludkiewicz, H. (2024). Gender norms and the gender gap in higher education. Labour Econ. 87:102491. doi: 10.1016/j.labeco.2023.102491
Kaya, E. (2023). Gender wage gap trends in Europe: the role of occupational skill prices. Int. Labour Rev. 162, 385–405. doi: 10.1111/ilr.12338
Kline, R. B. (2016). Principles and practice of structural equation modelling. New York, NY, USA: The Guilford Press.
Legewie, J., and DiPrete, T. A. (2014). The high school environment and the gender gap in science and engineering. Sociol. Educ. 87, 259–280. doi: 10.1177/0038040714547770
Leibnitz, G. M., Gillian-Daniel, D. L., Greenler, R. M., Campbell-Montalvo, R., Metcalf, H., Segarra, V. A., et al. (2022). The inclusive professional framework for societies: changing mental models to promote diverse, equitable, and inclusive STEM systems change. Front. Sociol. 6, 1–9. doi: 10.3389/fsoc.2021.784399
Levanon, A., and Grusky, D. B. (2016). The persistence of extreme gender segregation in the twenty-first century. Am. J. Sociol. 122, 573–619. doi: 10.1086/688628
Li, J., Faisal, E., and Al Hariri, A. (2022). Numbers for boys and words for girls? Academic gender stereotypes among Chinese parents. Sex Roles 87, 306–326. doi: 10.1007/s11199-022-01317-x
Lipson, S. K., Zhou, S., Abelson, S., Heinze, J., Jirsa, M., Morigney, J., et al. (2022). Trends in college student mental health and help-seeking by race/ethnicity: findings from the national healthy minds study, 2013-2021. J. Affect. Disord. 306, 138–147. doi: 10.1016/j.jad.2022.03.038
Liu, R. (2018). Gender-math stereotype, biased self-assessment, and aspiration in STEM careers: the gender gap among early adolescents in China. Comp. Educ. Rev. 62, 522–541. doi: 10.1086/699565
Maloshonok, N., Shcheglova, I., Vilkova, K., and Abramova, M. (2022). Gender stereotypes and the choice of an engineering undergraduate program. Educ. Stud. Moscow 3:3. doi: 10.17323/1814-9545-2022-3-149-186
Master, A., Cheryan, S., Moscatelli, A., and Meltzoff, A. N. (2017). Programming experience promotes higher STEM motivation among first-grade girls. J. Exp. Child Psychol. 160, 92–106. doi: 10.1016/j.jecp.2017.03.013
Merma-Molina, G., Ávalos-Ramos, M. A., and Martínez Ruiz, M. Á. (2021). Gender stereotypes: persistence and challenges. Equal. Divers. Inclusion 41, 1112–1135. doi: 10.1108/EDI-12-2018-0229
Micari, M., and Pazos, P. (2021). Beyond grades: improving college students’ social-cognitive outcomes in STEM through a collaborative learning environment. Learn. Environ. Res. 24, 123–136. doi: 10.1007/s10984-020-09325-y
Moè, A., Hausmann, M., and Hirnstein, M. (2021). Gender stereotypes and incremental beliefs in STEM and non-STEM students in three countries: relationships with performance in cognitive tasks. Psychol. Res. 85, 554–567. doi: 10.1007/s00426-019-01285-0
Moskos, M. (2020). Why is the gender revolution uneven and stalled? Gender essentialism and men’s movement into ‘women’s work’. Gender Work Organization 27, 527–544. doi: 10.1111/gwao.12406
Napp, C., and Breda, T. (2022). The stereotype that girls lack talent: a worldwide investigation. Science. Advances 8:eabm3689. doi: 10.1126/sciadv.abm3689
Nguyen, G. T., and Truong, D. H. (2024). Evaluating the importance of ecosystem Services in University Campus. Civil Engin. J. 10, 234–248. doi: 10.28991/CEJ-2024-010-01-015
Nielsen, M. W., Alegria, S., Börjeson, L., Etzkowitz, H., Falk-Krzesinski, H. J., Joshi, A., et al. (2017). Gender diversity leads to better science. Proc. Natl. Acad. Sci. 114, 1740–1742. doi: 10.1073/pnas.1700616114
O’Rourke, T., Vogel, C., John, D., Pryss, R., Schobel, J., Haug, F., et al. (2022). The impact of coping styles and gender on situational coping: an ecological momentary assessment study with the mHealth application TrackYourStress. Front. Psychol. 13, 1–9. doi: 10.3389/fpsyg.2022.913125
Olsson, M. I. T., van Grootel, S., Block, K., Schuster, C., Meeussen, L., Van Laar, C., et al. (2023). Gender gap in parental leave intentions: evidence from 37 countries. Polit. Psychol. 44, 1163–1192. doi: 10.1111/pops.12880
Owoc, M. L. (2022). Collective intelligence of honey bees for energy and sustainability. En E. Mercier-Laurent and G. Kayakutlu (Eds.), Artificial intelligence for knowledge management, energy, and sustainability. Springer International Publishing. pp 102–116.
Parvazian, S., Gill, J., and Chiera, B. (2017). Higher education, women, and sociocultural change: a closer look at the statistics. SAGE Open 7:2158244017700230. doi: 10.1177/2158244017700230
Pascoe, M. C., Hetrick, S. E., and Parker, A. G. (2020). The impact of stress on students in secondary school and higher education. Int. J. Adolesc. Youth 25, 104–112. doi: 10.1080/02673843.2019.1596823
Pelucio, L., Simões, P., Dourado, M. C. N., Quagliato, L. A., and Nardi, A. E. (2022). Depression and anxiety among online learning students during the COVID-19 pandemic: a cross-sectional survey in Rio de Janeiro, Brazil. BMC Psychol. 10:192. doi: 10.1186/s40359-022-00897-3
Prakasam, G. R., and Mukesh, R. G. (2019). Enrolment by academic discipline in higher education: differential and determinants. J. Asian Bus. Econ. Stud. 26, 265–285. doi: 10.1108/JABES-12-2018-0104
Presler-Marshall, E., Jones, N., Baird, S., Dutton, R., and Yadete, W. (2024). ‘Mind the gaps’: exploring regional and gender patterns in threats to Ethiopian adolescents’ bodily integrity. Eur. J. Dev. Res. 36, 306–326. doi: 10.1057/s41287-023-00602-z
Rae, D., and Blenker, P. (2023). The role of collective intelligence and collective agency in enterprising communities. J. Enterpris. Commun. 18, 7–28. doi: 10.1108/JEC-09-2022-0141
Risman, B. J., and Davis, G. (2013). From sex roles to gender structure. Curr. Sociol. 61, 733–755. doi: 10.1177/0011392113479315
Romero, I. M. V., and Blanco, Á. B. (2019). Factores sociocognitivos asociados a la elección de estudios científico-matemáticos. Un análisis diferencial por sexo y curso en la Educación Secundaria. Revist. Investig. Educ. 37, 269–286. doi: 10.6018/rie.37.1.303531
Rothermund, K., and de Paula Couto, M. C. P. (2024). Age stereotypes: dimensions, origins, and consequences. Curr. Opin. Psychol. 55:101747. doi: 10.1016/j.copsyc.2023.101747
Rucker, D. D., Galinsky, A. D., and Magee, J. C. (2018). The agentic–communal model of advantage and disadvantage: How inequality produces similarities in the psychology of power, social class, gender, and race. In Advances in Experimental Social Psychology, (Elsevier Academic Press, San Diego, CA, USA), 71–125.
Salmela-Aro, K. (2020). The role of motivation and academic wellbeing – the transition from secondary to further education in STEM in Finland. Eur. Rev. 28, S121–S134. doi: 10.1017/S1062798720000952
Santomauro, D. F., Herrera, A. M. M., Shadid, J., Zheng, P., Ashbaugh, C., Pigott, D. M., et al. (2021). Global prevalence and burden of depressive and anxiety disorders in 204 countries and territories in 2020 due to the COVID-19 pandemic. Lancet 398, 1700–1712. doi: 10.1016/S0140-6736(21)02143-7
Scala, F., and Paterson, S. (2018). Stories from the front lines: making sense of gender mainstreaming in Canada. Polit. Gend. 14, 208–234. doi: 10.1017/S1743923X17000526
Schmiedl, A., and Kauffeld, S. (2023). The positive effects of resource-oriented training on students’ perception and use of social support. Front. Educ. 8:1187319. doi: 10.3389/feduc.2023.1187319
Schriesheim, C. A., and Hill, K. D. (1981). Controlling acquiescence response Bias by item reversals: the effect on questionnaire validity. Educ. Psychol. Meas. 41, 1101–1114. doi: 10.1177/001316448104100420
Sczesny, S., Nater, C., and Eagly, A. H. (2018). Agency and communion: Their implications for gender stereotypes and gender identities. In Agency and Communion in Social Psychology, (Eds.) A. Abele & B. Wojciszke (Taylor & Francis, Abingdon, UK), 103–116. doi: 10.4324/9780203703663
Shin, S., Ha, M., and Lee, J.-K. (2016). The development and validation of instrument for measuring high school students’ STEM career motivation. J. Korean Assoc. Sci. Educ. 36, 75–86. doi: 10.14697/JKASE.2016.36.1.0075
Starr, C. R., Ramos Carranza, P., and Simpkins, S. D. (2022). Stability and changes in high school students’ STEM career expectations: variability based on STEM support and parent education. J. Adolesc. 94, 906–919. doi: 10.1002/jad.12067
Stringer, K., Mace, K., Clark, T., and Donahue, T. (2020). STEM focused extracurricular programs: Who’s in them and do they change STEM identity and motivation?. Res. Sci. Technol. Educ. 38, 507–522. doi: 10.1080/02635143.2019.1662388
Sulik, J., Bahrami, B., and Deroy, O. (2022). The diversity gap: when diversity matters for knowledge. Perspect. Psychol. Sci. 17, 752–767. doi: 10.1177/17456916211006070
Sumeera, K., Khan, M., Muhammad Ilyas,, Abro, P., and Ahmed, N. (2024). Exploring the factors of anxiety affecting students’ academic performance: a study of higher education institution of Larkana. Voyage J. Educ. Stud. 4, 68–82. doi: 10.58622/vjes.v4i1.117
Tanyildiz, G. S. (2023). Social reproduction, infrastructure, and the everyday. Dialogues in Human Geography, 13. doi: 10.1177/20438206231202820
Tourangeau, R., and Yan, T. (2007). Sensitive questions in surveys. Psychol. Bull. 133, 859–883. doi: 10.1037/0033-2909.133.5.859
van Aalderen-Smeets, S. I., Walma van der Molen, J. H., and Xenidou-Dervou, I. (2019). Implicit STEM ability beliefs predict secondary school students’ STEM self-efficacy beliefs and their intention to opt for a STEM field career. J. Res. Sci. Teach. 56, 465–485. doi: 10.1002/tea.21506
Vos, H., Marinova, M., Léon, S., Sasanguie, D., and Reynvoet, B. (2023). Gender differences in young adults’ mathematical performance: examining the contribution of working memory, math anxiety and gender-related stereotypes. Learn. Individ. Differ. 102:102255. doi: 10.1016/j.lindif.2022.102255
Wickham, H., Cetinkaya, M., and Grolemund, G. (2023). R for data science. O’Reilly Media, Sebastopol, CA, USA.
Wishkoski, R., Meter, D. J., Tulane, S., King, M. Q., Butler, K., and Woodland, L. A. (2022). Student attitudes toward research in an undergraduate social science research methods course. Higher Educ. Pedagog. 7, 20–36. doi: 10.1080/23752696.2022.2072362
Wissing, R. O., Hilverda, F., Scheepers, R. A., Nieboer, A. P., and Vollmann, M. (2022). Peer relationships buffer the negative association of online education with education satisfaction and subsequently with study engagement among undergraduate medical students. BMC Med. Educ. 22:276. doi: 10.1186/s12909-022-03337-3
Woo, H., Heo, N., Jang, H., and Jang, Y. (2023). Parental and school factors on American high school students’ academic and career intentions in STEM fields. Int. J. Educ. Vocat. Guid. 23, 19–42. doi: 10.1007/s10775-021-09498-9
Woolley, A. W., Chow, R. M., Mayo, A. T., Riedl, C., and Chang, J. W. (2023). Collective attention and collective intelligence: the role of hierarchy and team gender composition. Organ. Sci. 34, 1315–1331. doi: 10.1287/orsc.2022.1602
Woolley, A. W., and Gupta, P. (2024). Understanding collective intelligence: investigating the role of collective memory, attention, and reasoning processes. Perspect. Psychol. Sci. 19, 344–354. doi: 10.1177/17456916231191534
World Economic Forum. (2023). Future of jobs report. https://www3.weforum.org/docs/WEF_Future_of_Jobs_2023.pdf (accessed January 12, 2024).
Yang, Y., Tian, T. Y., Woodruff, T. K., Jones, B. F., and Uzzi, B. (2022). Gender-diverse teams produce more novel and higher-impact scientific ideas. Proc. Natl. Acad. Sci. 119:e2200841119. doi: 10.1073/pnas.2200841119
Keywords: gender gap, psychometric instrument, higher education, determinants of gender disparity, critical factors, educational interventions, gender policies
Citation: Beroíza-Valenzuela F, Salas-Guzmán N and Huepe D (2024) Gender disparities in higher education: development and validation of the FACT-GÉN instrument. Front. Educ. 9:1456085. doi: 10.3389/feduc.2024.1456085
Received: 28 June 2024; Accepted: 25 October 2024;
Published: 22 November 2024.
Edited by:
Elvira G. Rincon-Flores, Monterrey Institute of Technology and Higher Education (ITESM), MexicoReviewed by:
Maura Pilotti, Prince Mohammad Bin Fahd University, Saudi ArabiaCopyright © 2024 Beroíza-Valenzuela, Salas-Guzmán and Huepe. This is an open-access article distributed under the terms of the Creative Commons Attribution License (CC BY). The use, distribution or reproduction in other forums is permitted, provided the original author(s) and the copyright owner(s) are credited and that the original publication in this journal is cited, in accordance with accepted academic practice. No use, distribution or reproduction is permitted which does not comply with these terms.
*Correspondence: Francisca Beroíza-Valenzuela, ZnJhbmNpc2NhLmJlcm9pemFAdWcudWNoaWxlLmNs; David Huepe, ZGF2aWQuaHVlcGVAdWFpLmNs
Disclaimer: All claims expressed in this article are solely those of the authors and do not necessarily represent those of their affiliated organizations, or those of the publisher, the editors and the reviewers. Any product that may be evaluated in this article or claim that may be made by its manufacturer is not guaranteed or endorsed by the publisher.
Research integrity at Frontiers
Learn more about the work of our research integrity team to safeguard the quality of each article we publish.