- 1Faculty of Education, Simon Fraser University, Burnaby, BC, Canada
- 2JSPS Overseas Research Fellow, Tokyo, Japan
Although scientific inquiry with simulations may enhance learning, learners often face challenges creating high demand for self-regulation due to an abundance of information in simulations and supplementary instructional texts. In this research, participants engaged in simulation-based inquiry about principles of electric circuits supplemented by domain-specific expository text provided on-demand. They received just-in-time inquiry prompts for inquiry behaviors, guidance to self-explain electrical principles, both, or neither. We examined how these interventions influenced participants’ access of text information and achievement. Undergraduates (N = 80) were randomly assigned to one of four groups: (1) inquiry prompts and principle-based self-explanation (SE) guidance, (2) inquiry prompts without principle-based SE guidance, (3) principle-based SE guidance without inquiry prompts, or (4) control. Just-in-time inquiry prompts facilitated learning rules. However, there was no main effect of principle-based self-explanation guidance nor an interaction involving both interventions. Effects of just-in-time inquiry prompts were moderated by prior knowledge. Although principle-based self-explanation guidance promoted re-examination of text-based domain information, reading time did not affect posttest scores. These findings have important implications for instructional design of computer-based adaptive guidance in simulation-based inquiry learning.
1 Introduction
Alongside the widespread introduction of computers in classrooms, simulation-based inquiry learning has garnered much attention in STEM education. Simulations are interactive software that dynamically model phenomena, enable learners to manipulate variables and observe results (Banda and Nzabahimana, 2023; Smetana and Bell, 2012). Meta-analyses reported STEM-related simulations enhance learning (Antonio and Castro, 2023; D’Angelo et al., 2014). However, two characteristics of simulation-based inquiry learning present challenges for learners.
First, learners are exposed to a plethora of information, a characteristic common in multimedia learning (Mayer, 2014). Unlike pre-made images or animations, models presented by simulations dynamically update representations as a learner sets each new value for variables. Learners are required to process and integrate such dynamic representations continuously with their prior knowledge (Blake and Scanlon, 2007; Kabigting, 2021; Rutten et al., 2012).
In addition to the simulation itself, simulations often incorporate supplementary information about the target learning domain in the form of expository texts or other media (see simulations used in Chang et al., 2008; Eckhardt et al., 2013; Swaak and de Jong, 2001). Although these information supplements align with instructional design principles (van Merriënboer and Kester, 2014; van Merriënboer and Kirschner, 2019) and offer potential to improve outcomes (Hulshof and de Jong, 2006; Kuang et al., 2023; Lazonder et al., 2010; Wecker et al., 2013), learners face additional work integrating them with the primary information source (i.e., simulation) and experience increased cognitive load (de Jong and Lazonder, 2014). Previous studies, however, rarely examined how often simulation-based learning environments incorporate expository texts (and other media) and how to support effective utilization of the information they can provide.
Second, learners need to be productively self-regulating. Inquiry learning, a form of constructivist learning (de Jong and van Joolingen, 1998; Mayer, 2004; White and Frederiksen, 1998), requires learners exercise agency for their learning to discover principles through self-directed investigation (Alfieri et al., 2011; de Jong, 2019; de Jong et al., 2023; Lazonder, 2014). It typically involves multiple phases, including generating questions and hypotheses, conducting experiments where variables are manipulated, observing findings and drawing conclusions based on that evidence. Throughout an inquiry activity, learners regulate learning through planning and monitoring (de Jong and Njoo, 1992; de Jong and Lazonder, 2014; Pedaste et al., 2015; Zimmerman and Klahr, 2018). This complexity poses challenges for learners in procedural execution, higher-order thinking, and coordinating those complementary processes (de Jong and van Joolingen, 1998; Klahr and Nigam, 2004; Kranz et al., 2023; Njoo and de Jong, 1993; Wu and Wu, 2011; Zimmerman, 2007).
Instructors and some software systems often provide guidance to help learners manage this complexity (Lazonder and Harmsen, 2016; Sun et al., 2022; Vorholzer and von Aufschnaiter, 2019). Guided-inquiry learning can be considered a midpoint along a continuum varying from extensive guidance in direct instruction to nil guidance in discovery (Furtak et al., 2012). In this conceptualization, a key to learning effectiveness is tailoring guidance to each learner while assessing their performance during inquiry as contrasted to providing uniform guidance to all learners (de Jong and Lazonder, 2014; Fukuda et al., 2022). While AI may offer potential for effectively adapting guidance (Dai and Ke, 2022; de Jong et al., 2023; Linn et al., 2018), empirical evidence on the effectiveness of adaptive guidance for individual learners in simulation-based inquiry learning is meager.
In this study, we investigated effects of two types of guidance potentially addressing these challenges in simulation-based inquiry learning. One intervention is adaptive guidance in the form of just-in-time prompts designed to promote a learner’s self-regulated learning behaviors—effective use of inquiry tactics. The second is principle-based self-explanation guidance aimed at facilitating synthesis of information—a learner’s integration of information from two different sources, the simulation and accompanying expository text.
Guidance is “any form of assistance offered before and/or during the inquiry learning process that aims to simplify, provide a view on, elicit, supplant, or prescribe the scientific reasoning skills involved” (Lazonder and Harmsen, 2016, p. 687). Six types of guidance can be distinguished by explicitness. For instance, the least explicit guidance sets process constraints, which limit the number of elements the learner considers. Moderately explicit guidance takes the form of prompts which tell the learner what to do, while the most explicit guidance involves explanations that teach the learner how to take a specific action. Lazonder and Harmsen (2016) meta-analysis found explicit guidance was more effective for successful performance in inquiry, while all types of guidance were equally effective for post-inquiry learning outcomes.
Guidance in inquiry learning can also be characterized by adaptiveness, i.e., providing learners with information they can use to make decisions when engaging with computer-based environments they control (Bell and Kozlowski, 2002). Adaptive guidance in computer-based learning has been extensively studied in Intelligent Tutoring Systems for learning from texts and solving well-defined problems (Graesser and McNamara, 2010; Kulik and Fletcher, 2016). Recent research has investigated adaptive guidance in inquiry learning, which can address problems about implementation of individualized support where there is wide variation in learning trajectories and self-regulatory abilities among students (Kerawalla et al., 2013). One example is the Web-based Inquiry Science Environment (WISE; Slotta and Linn, 2009). Gerard et al. (2016) graded students’ essays submitted during an inquiry learning unit in WISE and provided guidance based on teacher’s assessments in a computer system to enhance students’ knowledge integration. Another example is the Inquiry Intelligent Tutoring System (Inq-ITS; Gobert et al., 2013, 2018), which allows participants to enter facets on worksheets designed for each phase of inquiry learning, such as collecting and analyzing data. The system provides various levels of guidance to promote using effective inquiry behaviors depending on learners’ input.
Designing systems to develop transferable scientific thinking skills and conceptual understanding requires close attention to two issues. First, students should be guided to acquire conditional knowledge of when and how to use inquiry strategies (Winne, 1996, 2022; Veenman, 2011). Providing adaptive guidance responsive to the learner’s needs and progress, like a just-in-time question or suggestion provided by human tutors (Chi et al., 2001; VanLehn et al., 2003), models for learners when and how to apply strategies under appropriate conditions. Second, to obtain substantial learning gains students should be guided according to their current level of inquiry skills and their understanding of the learning content (Fukuda et al., 2022).
In this study, we formulate just-in-time inquiry prompts for facilitating effective inquiry behaviors within tasks as adaptive guidance (Fukuda et al., 2022; Hajian et al., 2021). We address the aforementioned issues by having an experimenter evaluate learning performance and provide pre-developed recorded voice and text prompts in real time to learners through a computer interface. Essentially, the experimenter mimics an AI-based adaptive guidance system in a Wizard of Oz design (Kelley, 1983). Our inquiry prompts (1) adjust to conditions in the moment, (2) tailor guidance to match specific issues the learner encounters in inquiry skills or content understanding, and (3) adapt to the learner’s progress by gradually fading support as they gain proficiency. From the perspective of self-regulated learning theory (Winne, 1995, 2022), guidance scaffolds self-monitoring of learning by managing cognitive load to allow cognitive resources to be assigned to learning content and developing inquiry skills. As the learner develops competence, they gradually increase responsibility for self-monitoring and other self-regulatory functions which theoretically also promotes autonomy.
Since it is challenging to engage in inquiry without some initial knowledge (Lazonder et al., 2009; van Riesen et al., 2018a), supplementary information about the target domain is often provided in various formats, including lectures by teachers/experimenters (Weaver et al., 2018; Wecker et al., 2013) or access to expository texts (Hulshof and de Jong, 2006; Lazonder et al., 2010). Although not many studies have examined the effect of providing domain-specific information in inquiry learning, some previous studies showed that supplementary information can enhance inquiry outcomes (e.g., Hulshof and de Jong, 2006; Kuang et al., 2023; Lazonder et al., 2010; Leutner, 1993; Wecker et al., 2013).
In particular, expository texts available on demand may strengthen learners’ understanding of domain-specific terms and concepts by supplying information when it is needed. However, merely providing on-demand expository resources does not ensure they will be productively used by learners, and there is evidence such resources are often underused by students engaged in inquiry learning (Swaak and de Jong, 2001). Hulshof and de Jong (2006) provided on-demand information tips and reported a positive correlation between prior knowledge and information use. These studies suggest learners with less prior knowledge, who should need more information, were not able to use the information effectively.
van der Graaf et al. (2020) designed a learning environment divided into two spaces side by side: one for informational texts—domain content and instructions for inquiry behaviors (hypothesizing, experimenting, and concluding)—and the other for interacting with a simulation. Increased gaze transitions across these spaces, indicating integration of information developed in the simulation and presented in texts, was associated with a greater number of correct experiments. Additionally, integration moderated the impact of low prior knowledge on correct experiments. However, the increased gaze transitions between the simulation and instructional texts apparently improved neither the quality of inquiry learning nor posttest performance. The texts included information on not only the domain but also inquiry procedures, and they required participants to follow them sequentially rather than on demand. Nonetheless, the results suggest the necessity for further research on interventions to shape learners’ effective use of texts.
To facilitate learners’ access to on-demand expository text and integration of information in the simulation and texts, we draw on a framework of example-based learning in which learners first read instructional text providing basic knowledge, such as principles and concepts, followed by studying examples illustrating those principles and concepts (Renkl, 2014; Roelle and Renkl, 2020; Wittwer and Renkl, 2010). This learning process emphasizes generating principle-based self-explanations in which learners use the knowledge elements in generated explanations to describe or justify the provided examples (Berthold et al., 2009; Renkl, 1997; Roelle et al., 2017; Roelle and Renkl, 2020).
While many studies have examined the effectiveness of prompting self-explanation in the second step (e.g., Atkinson et al., 2003; Hefter et al., 2015; Schworm and Renkl, 2007), only a few focused on the initial step, studying instructional text (e.g., Hiller et al., 2020; Roelle et al., 2017; Roelle and Renkl, 2020). For example, Hiller et al. (2020) examined effects of facilitating learners’ active processing of basic instructional texts and accessibility of the texts during self-explanation (open-book format vs. closed-book format) on posttest performance in chemistry example-based learning. They found that active process prompts, which asked learners to explain the content after reading instructional text, promoted reference to important content in their self-explanations.
The effects of self-explanation have also been studied in inquiry learning (e.g., Elme et al., 2022; Li et al., 2023; van der Meij and de Jong, 2011). van der Meij and de Jong (2011) compared effects of general self-explanation prompts, which only ask participants to explain their answers, with directive self-explanation prompts, which explicitly instruct participants to explain relationships between two or more representations (such as diagrams, equations, graphs, and tables) provided by physics simulations. Directive self-explanation led to higher scores on the posttest despite incurring higher cognitive load. In a quasi-experimental study, Elme et al. (2022) investigated effects of self-explanation prompts while learners interacted with a simulation-based learning environment which followed the PSEC framework (prediction, simulation, explanation, and conclusion). For instance, during the prediction phase, participants were prompted to predict the result by describing the relationship between variables. Compared to a control group experiencing the PSEC process without self-explanation prompts, the group receiving the self-explanation prompts showed higher posttest scores.
Previous research on inquiry learning studied various types of self-explanation ranging from prompting explanations that integrate representations obtained within a simulation to facilitating an inquiry process, such as predicting and justifying answers. There are few studies focusing on self-explanation that integrates simulation outcomes and informational texts. In one, Wecker et al. (2013) investigated effects of providing instruction about theory. That study found inquiry alone may not lead to acquiring theoretical-level knowledge. This raises the question of whether self-explanation prompts to integrate instructional text and simulation outcomes facilitate knowledge acquisition, including the understanding the theory behind the rules.
In the present study, throughout an inquiry learning session participants were able to access expository, domain-specific text which presented basic terms and concepts related to the task of inquiring about Ohm’s law. However, to maintain the constructive nature of inquiry learning, text directly describing Ohm’s law formula, which governed the behavior of the simulation, was available only after inquiry tasks were completed in the simulation. Principle-based self-explanation linked instructional texts accessed on demand with simulation outcomes via two types of prompts. First, before engaging in the inquiry task, learners were asked to access expository texts and explain terms about electricity included in the simulation space (i.e., active process prompts). To increase learners’ awareness of the need to consult informational texts, participants were asked to explain basic terms without referring to expository texts before accessing expository texts. We aimed to enhance their metacomprehension (Fukaya, 2013; Griffin et al., 2008). Note that these active process prompts do not qualify as a type of self-explanation prompt since they do not require participants to explain beyond the texts themselves (Hiller et al., 2020). As the second component, after completing inquiry tasks in a simulation, participants were asked to write rules they had discovered via inquiry and then to explain the rules based on the laws behind the simulation model (i.e., principle-based self-explanation prompts). Participants assigned to groups without principle-based self-explanation prompts were also asked to write the rules. However, they were not asked explicitly to integrate information from expository texts, only asked to “explain your rules” (i.e., received general self-explanation prompts). We referred to the intervention consisting of active process prompts and principle-based self-explanation prompts as principle-based self-explanation guidance.
The focus of this study was to examine effects of two interventions, just-in-time inquiry prompts (hereafter referred to as inquiry prompts, abbreviated Inq) and principle-based self-explain guidance (hereafter referred to as principle-based SE guidance, abbreviated SE), on learners’ acquisition of knowledge about rules through guided inquiry learning and information use behavior. Table 1 illustrates the conceptualization and differences between the two interventions. The inquiry prompts ask participants to input predictions, plans, and discovered rules into a textbox but not to justify rules or integrate them with expository texts. Following the inquiry phases outlined by Pedaste et al. (2015), inquiry prompts support generating hypotheses, predicting, experimenting, and deriving relationships based on gathered data (i.e., conceptualization and investigation). On the other hand, principle-based SE guidance supports two phases: orientation, which involves learning basic information, and conclusion, which entails explaining findings. During the interventions of principle-based SE guidance, learners do not interact with the simulation.
We investigated three research questions:
1. Do inquiry prompts (Inq) and principle-based guidance (SE) promote learning rules related to inquiry tasks about series circuits?
2. Are effects of inquiry prompts (Inq) and principle-based guidance (SE) on learning outcomes moderated by learners’ prior knowledge?
3. Does information use mediate the impact of principle-based guidance (SE) on learning outcomes?
A posttest included multiple-choice items plus a requirement to explain the answer. These items assessed qualitative understanding of relationships among variables as well as the underlying principles.
Learners were expected to benefit from both inquiry activities within the simulation and access to supplementary expository texts, resulting in improved learning outcomes. Therefore, we offer three hypotheses. First, we expected both inquiry prompts (Hypothesis 1a) and principle-based self-explanation guidance (Hypothesis 1b) would positively influence posttest performance. Moreover, as our interventions address different aspects of learners’ challenges in inquiry learning, we hypothesized the group receiving both types of guidance would demonstrate the highest performance (Hypothesis 1c). Regarding the influence of prior knowledge, previous studies revealed prior knowledge influences the effect of guidance (e.g., Roll et al., 2018; van Dijk et al., 2016; van Riesen et al., 2018a,2018b). However, since supplementary texts might compensate for low prior knowledge, we expected a moderation effect of prior knowledge would be only observed for the inquiry prompts group, where participants did not receive principle-based self-explanation guidance (Hypothesis 2). Lastly, previous research has produced inconsistent findings regarding the relationship between access to expository texts and performance in inquiry learning (Manlove et al., 2007, 2009; van der Graaf et al., 2020). Since expository texts in this study exclusively pertain to domain knowledge, we can distinguish access to the expository text from a response to difficulties related to the inquiry procedure, which would interfere with learning. Therefore, we expected the effect of principle-based self-explanation guidance on learning outcomes would be mediated by the increased reading time of expository texts (Hypothesis 3).
2 Methodology
2.1 Participants
Participants (N = 83; Mage = 19.73, SD = 1.40, range = 18–24, 52 women) were volunteers recruited at nine universities in Japan and the mailing list for the Japanese community at a university in Canada enrolled in various faculties, such as Education, Social Science, and Economics. Students enrolled in the Faculty of Science or pursuing science education majors in the Faculty of Education, as well as individuals with experience tutoring science, were not eligible to participate. The protocol for this research was approved by the Ethics Review Board of Authors’ affiliation. All participants provided informed consent prior to participating and received monetary compensation of 1,200 JPY per hour.
Participants were randomly assigned to one of four groups using a 2 × 2 between-subjects factorial design: (1) inquiry prompts and principle-based self-explanation guidance (Inq+SE), (2) inquiry prompts and no principle-based self-explanation guidance (Inq), (3) no inquiry prompts with principle-based self-explanation guidance (SE), or (4) control. Based on a boxplot of pretest scores, three outliers were identified using the criterion Q3 + 3 times the interquartile range (Dawson, 2011) and excluded from analyses. Therefore, the final sample size for analyses was N = 80 (n = 20 for each group), which a power analysis1 suggested was adequate to test our hypotheses.
2.2 Learning environment
Participants engaged in inquiry learning using an interactive website developed for this study (see Figure 1).
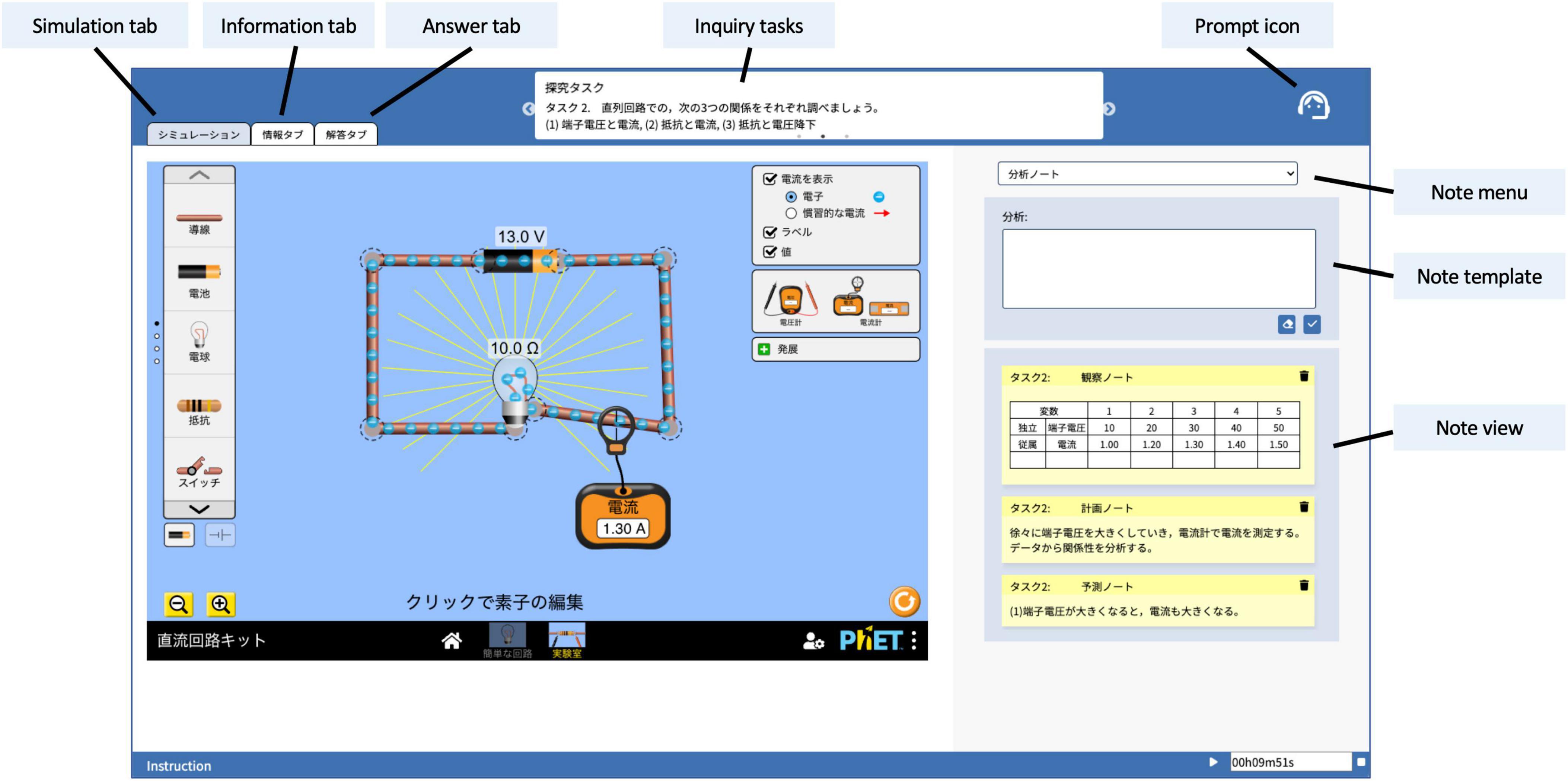
Figure 1. Screenshot of learning website [including a simulation by PhET Interactive Simulations, University of Colorado Boulder, licensed under CC-BY-4.0 (https://phet.colorado.edu)].
2.2.1 Simulation tab
The simulation tab presented a DC circuit simulation developed by PhET Interactive Simulations (University of Colorado Boulder2, licensed under CC-BY-4.0). The simulation, shown in Figure 1, featured electronic components and instruments to measure voltage and current. Participants configured circuits and observed changes in values for voltage and current displayed on measuring devices and changes in the brightness of the bulb by adjusting resistance and voltage of the battery. To encourage focusing on learning, participants were instructed not to use less relevant components such as coins.
2.2.2 Information tab
The information tab presented hyperlinked texts about electricity based on high school physics textbooks. Five types of information were available (Supplementary Appendix A): (a) terms central to inquiry tasks (e.g., resistance), (b) measurement tools (e.g., voltmeter), (c) fundamental disciplinary concepts used to explain terms (e.g., electric potential), (d) peripheral disciplinary concepts (e.g., Coulomb force) and (e) laws and models of relationships among variables in electric circuits (e.g., Ohm’s law).
By clicking on the menu button, then clicking on a term in the menu that appeared, participants could open a text page showing a one- or two-line summary of the term. Clicking a “Read more” button revealed detailed information with a diagram. Information types (a) to (d) were accessible throughout the inquiry tasks. Laws and models (e) which were the targets of inquiry tasks were accessible only after participants completed all inquiry tasks and switched to the answer tab.
2.2.3 Answer tab
In the answer tab, participants entered answers to inquiry tasks and self-explanations. First, all participants were asked to write the rules of voltage and current they had discovered in the inquiry session. Next, participants were asked to explain their rules, but prompts were different depending on the group, as described in section “2.3.2 Principle-based self-explanation guidance.”
When the answer tab was open, interaction with the simulation was blocked. While writing answers, all participants could refer to all the texts in the information tab and notes they wrote during inquiry tasks.
2.2.4 Note templates
The learning system provided an area where participants could choose among six note templates: variable, prediction, planning, observation, analysis, and free note for text fields: The observation note template included a table for recording data. Other templates had blank text fields for making a note. Submitted notes were posted in the note view area below. Participants could edit and delete note text.
2.2.5 Inquiry tasks
There were three tasks created based on previous studies (Fukuda et al., 2022; Hajian et al., 2021). In Task 1, participants were oriented to the simulation by freely manipulating features to create a series circuit. In Task 2, participants were asked to investigate each of three relationships in a series circuit: (1) terminal voltage and current, (2) resistance and current, and (3) resistance and voltage drop. In Task 3, participants were asked to construct a series circuit containing two or more resistors or light bulbs and, while keeping the battery value constant, to examine relationships between (1) resistance and current and (2) resistance and voltage drop to find the law of current and voltage drop, respectively.
2.2.6 Prompt icon
A prompt icon was only operational for participants in the Inq+SE and Inq groups. When they clicked on the icon, the text of the mostly recently played prompt was displayed in the balloon.
2.3 Interventions
2.3.1 Just-in-time inquiry prompts
The experimenter continuously monitored the learner’s behavior, speech, and notes. Based on a prompt decision map, just-in-time inquiry prompts were issued to encourage effective inquiry strategies coordinated to the learner’s behavior and comprehension status. The just-in-time, adaptive nature of prompts meant that not all prompts were given to each participant. For example, if a participant spontaneously stated a relationship, they were not given a prompt for that behavior. Inquiry prompts were pre-recorded using software that converted text to synthesized but natural-sounding speech. The experimenter activated playback of a prompt which sounded on the participant’s computer. Inquiry prompts were adopted and modified from previous research (Fukuda et al., 2022; Hajian et al., 2021) to form two categories based on Pedaste et al. (2015) framework of phases of inquiry: conceptualization and investigation (Supplementary Appendix B). Conceptualization is a process of understanding concepts belonging to an inquiry task leading to forming a hypothesis (Pedaste et al., 2015). For conceptualization, prompts targeted variables and predictions, e.g., “Before the experiment, please predict the relationship between terminal voltage and current.”
Investigation is the phase in which experimental actions are taken to examine the research question and hypothesis, and the outcome of this phase is interpreting the data and formulating relationships among the variables (Pedaste et al., 2015). To help this process, prompts for planning, testing, and rule generation were provided, e.g., “What is the relationship between resistance and current?”
Due to the different nature of tasks 2 and 3, the types of prompts received somewhat differed. In task 2, no evaluation or application prompts were given because the goal was to identify a relationship between two variables.
2.3.2 Principle-based self-explanation guidance
The Inq+SE and SE groups received interventions at two points. Before the inquiry task began, the Inq+SE and SE participants were instructed to understand all the terms involved in the simulation and inquiry tasks. To encourage monitoring prior knowledge of terms and raise awareness of the need to look up information, they were asked to explain a term without access to any texts. They were then directed to the information tab to check their explanation and explain the term again based on that information (i.e., active process prompts). This cycle repeated for all terms. No feedback was given on participants’ explanations, and no specific instructions were given about pages to examine although participants were encouraged to explore other pages if they did not find information they sought. After all terms were explained, participants engaged in the inquiry tasks.
After completing the inquiry tasks and moving to the answer tab, all participants described the rules of current and voltage they generated within the inquiry tasks. Then, the Inq+SE and SE groups provided explanations in response to principle-based self-explanation prompts encouraging them to use the laws and principles provided in the information tab: “Please explain the rules you found based on the text about laws and principles of electricity in the information tab.” The Inq and control groups also provided explanations in response to general SE prompts, which only asked, “Explain your rules.” Separate text boxes were provided for descriptions of current and voltage.
2.4 Pre and posttest
A pretest and identical posttest were administered to evaluate participants’ knowledge of electric circuits before and after the inquiry learning session3 (Supplementary Appendix C). All test items were reviewed by a high school physics teacher.
Nine items in a 4-option multiple-choice format assessed application of rules to electrical circuits. For each item, participants also were required to explain the reason for their response. One point was given for a correct choice. The explanation of the reason for each choice was rated 0–2 points based on high school physics standards (Supplementary Appendix D). Thus, the score for each item ranged from 0 to 3 points.
First and second coders independently coded pre and posttest items for 16 randomly selected participants (20% of the total sample). Mean percent item score agreement was 93.6% (76.9–100%) for the pretest, and 85.9% (76.9–100%) for rule application on the posttest. Disagreements were discussed and resolved before remaining data were coded by the first coder.
Cronbach’s alpha coefficients for rule application items were α = 0.42 for the pretest and α = 0.83 for the posttest. The low reliability of the pretest was also considered to be due to a floor effect. Given the α coefficient for the posttest, we determined items were internally consistent, so we formed a total score for the pre- and posttest, respectively (maximum 27 points for each).
2.5 Procedure
First, participants completed an online pretest within a week before their individual experimental session to avoid participant fatigue. The pretest required approximately 30 min.
The individual experimental sessions were conducted online using Zoom conferencing software. All sessions were recorded and learners’ manipulations in the learning system were logged. The session was designed to span 2 h, but the actual time depended on the intervention condition and participants’ learning pace.
The experimental session consisted of five activities: orientation, the active process prompts for the Inq+SE and SE groups, inquiry tasks within the simulation, post-task self-explanation in the answer tab, and posttest (Figure 2). Participants were told the purpose of the experiment was to engage them in scientific inquiry learning using effective inquiry strategies. Participants then read 11 short explanatory texts describing each strategy that the study aimed to promote (Supplementary Appendix E). Next, participants viewed a 3-min video explaining how to use the learning system and were given approximately one minute to operate a demo version of the system which provided no information about electricity. Participants then viewed a 3-min video explaining how to use the electrical circuit simulation.
Next, the Inq+SE and SE groups were given the active process prompts and access and explain terms in the information tab for about 20 min. The control and Inq groups moved directly to the inquiry tasks without this phase.
Before beginning the inquiry task, all participants were given instructions for the think-aloud protocol, asking them to “Please verbalize your thoughts while learning.” They were informed there were three tasks, that they could use the information tab as needed while completing the inquiry tasks and while filling in the answers tab, and that new text would be added to the information tab after they moved to the answers tab. Participants were also told they had 1.5 h to work but that could be extended if necessary to complete all tasks. Only the Inq+SE and Inq groups received inquiry prompts during inquiry tasks 2 and 3. After completing all tasks, participants moved to the answer tab to describe and explain rules they had discovered without interacting with the simulation. The Inq+SE+ and SE groups received principle-based SE prompts and were instructed to relate the results to the underlying laws and models, referring to the information tab. The control and Inq groups received general SE prompts. Finally, participants completed the posttest.
2.6 Data analyses
Data were analyzed using IBM SPSS Statistics Version 27. To conduct moderation and mediation analyses, we used the PROCESS macro (Hayes, 2022).
3 Results
3.1 Preliminary analyses
3.1.1 Pretest score
To investigate whether participants’ limited prior knowledge about rules in series circuits was homogeneous among groups, a one-way ANOVA was conducted with groups as the independent variable and pretest scores as outcome variables. There was no statistically detectable difference in the pretest scores among groups [F(3, 76) = 2.03, p = 0.12, partial η2 = 0.074, Table 2]. Also, there was no statistically detectable correlation between pretest and posttest scores (r = 0.19, p = 0.10).
The correlation coefficients per group were r = 0.18 (p = 0.44) for the control group, r = 0.72 (p < 0.001) for the Inq group, r = 0.18 (p = 0.45) for the SE group, and r = 0.19 (p = 0.42) for the Inq+SE group.
3.1.2 Frequency of prompts (P+ groups)
Next, to examine equivalence of prompts provided, an independent t-test compared the total frequency of prompts between the Inq and Inq+SE groups. There was no statistically detectable difference [t(38) = 0.34, p = 0.73, d = 0.11] regardless of receiving directions to explain. Means and SDs of frequency of prompts are showed in Supplementary Appendix F.
To investigate the adaptive nature of the prompts, Pearson’s correlations were calculated between each type of prompt and total prompt frequency and pretest scores. As expected, there were statistically detectable negative correlations between pretest scores and the number of prompts (r = −0.36, p < 0.05). Participants with lower prior knowledge were generally prompted more often, indicating they needed guidance to compensate for less knowledge about the rules of series circuits.
3.2 Effect of interventions on the posttest scores (RQ1)
We conducted a two-way ANCOVA to examine effects of interventions on posttest scores. The two interventions were independent variables, time spent in the simulation tab was a covariate, and the posttest score was the dependent variable. Pretest scores were not used as a covariate because they interacted with groups [see section “3.3 Effect of prior knowledge on intervention effectiveness (RQ2)”] and thus violated the ANCOVA assumptions (Meyers et al., 2016). The result revealed a statistically detectable main effect of inquiry prompts [F(1, 75) = 5.614, p = 0.02, partial η2 = 0.07]. However, there was neither a statistically detectable main effect of principle-based self-explanation guidance [F(1, 75) = 1.451, p = 0.23, partial η2 = 0.02] nor an interaction involving both interventions [F(1, 75) = 0.19, p = 0.66, partial η2 = 0.003]. The results suggest that only inquiry prompts enhance learning outcomes independently of learning time in the simulation tab.
3.3 Effect of prior knowledge on intervention effectiveness (RQ2)
To investigate whether learners’ prior knowledge moderated intervention effects, we conducted a moderation analysis (Hayes, 2022) using posttest scores as a dependent variable. Independent variables were three group dummy vectors comparing the control group (0) to each of the Inq group (Group 1 dummy), SE group (Group 2 dummy), and Inq+SE group (Group 3 dummy). The pretest score served as a moderator.
Only the interaction for the vector identifying Inq by pretest score was statistically detectable (Table 3), with the effect size for the regression being f2 = 0.408 (large effect; Cohen, 1992). The moderation effect of pretest score for the intervention effect was statistically detectable for participants who scored relatively medium and high on the pretest (Table 4 and Figure 3). It suggests that prompts were not effective for participants without some degree of prior knowledge. However, we did not find a statistically detectable moderation effect of pretest scores on Inq+SE group compared to the control group.
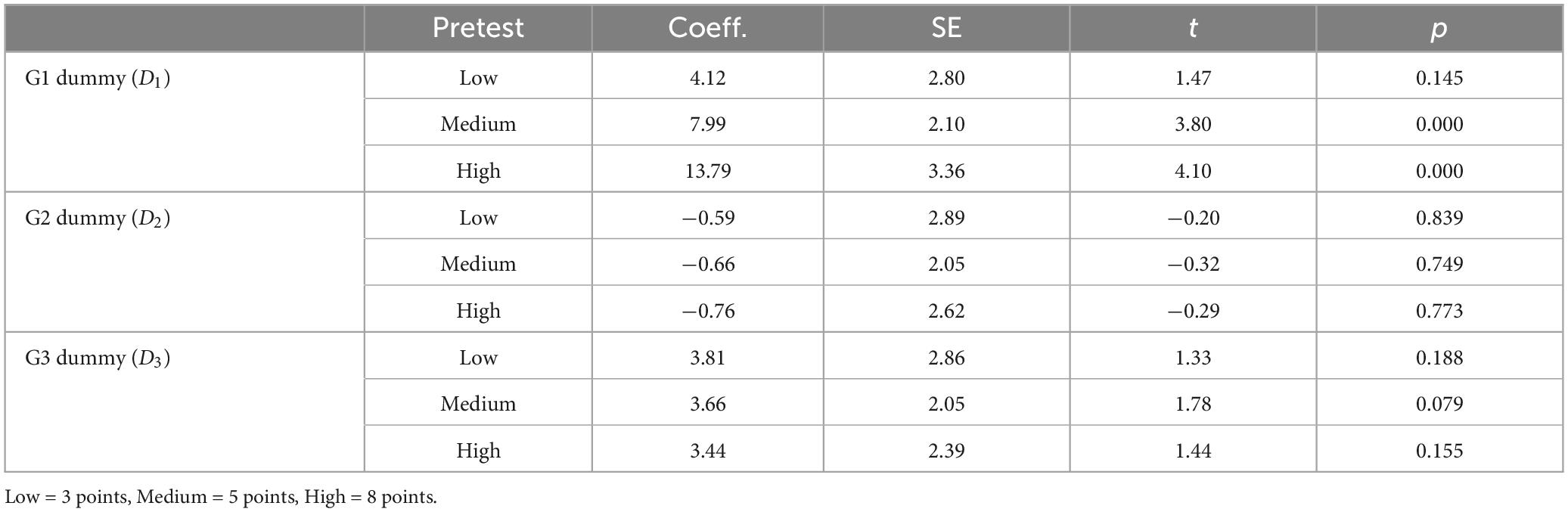
Table 4. Moderating effects of pretest scores on the relationship between the interventions and posttest scores.
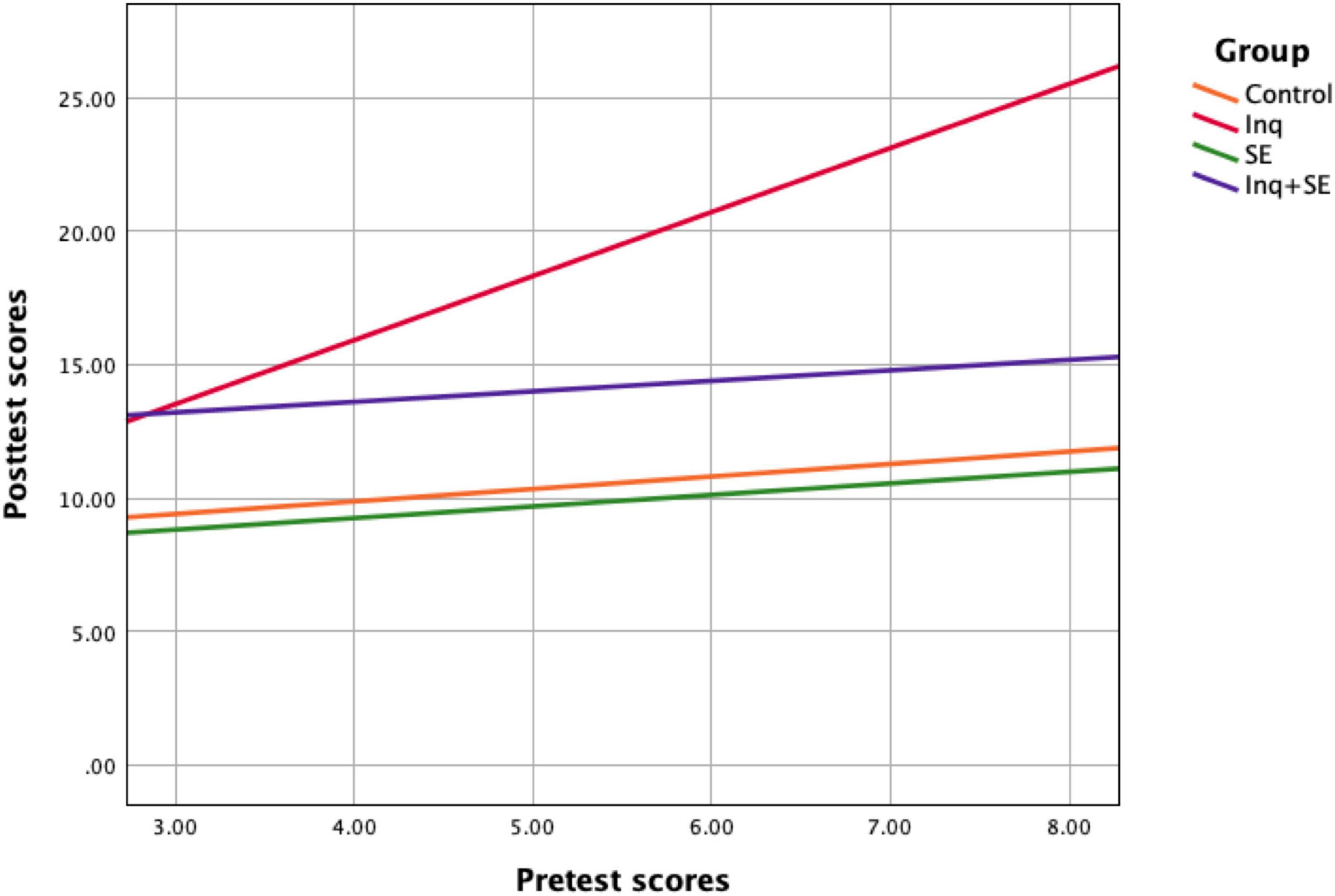
Figure 3. Moderating effects of pretest scores on the relationship between the interventions and posttest scores.
3.4 Mediation effect of information use on the relationship between interventions and posttest scores (RQ3)
To investigate whether time spent on the information text tab mediated relationships between the interventions and posttest scores, a mediation analysis was conducted with posttest scores as a dependent variable, three group dummy vectors as before comparing Inq, SE and Inq+SE, to the control group and time spent in the information text tab as a mediator. Pretest scores and time spent in the simulation tab were included as covariates (Figure 4).
There were positive associations between the SE and the Inq+SE dummy vectors, both of which received principle-based SE guidance, and time spent in the information text tab (Table 5). Similarly, in line with the mediation analysis, we found relative direct and total effects of the Inq dummy on posttest scores. However, we did not find statistically detectable indirect effects of group dummies on posttest scores, mediated by time spent in the information text tab (Table 6). These results suggest principle-based self-explanation guidance did enhance access and reading time in the information text tab, but this did not translate into higher posttest scores.

Table 6. Indirect effects of group dummies on posttest scores, mediated by time spent in the information tab.
4 Discussion
This study investigated how two interventions, just-in-time inquiry prompts and principle-based self-explanation guidance, affect learners’ acquisition of scientific rules when they participate in inquiry learning using a simulation and can access information on-demand in expository texts.
4.1 Effect of interventions on the posttest scores
Just-in-time inquiry prompts had positive effects on rule learning. Participants who received inquiry prompts demonstrated increased ability to apply rules. That this effect was observed even when learning time with the simulation was controlled suggests inquiry prompts promoted more strategically effective interactions with the simulation which led to rule discovery, Hypothesis 1-a was supported.
When learners answered multiple-choice questions about rules, they were asked to provide explanations for answers. We examined those explanations to evaluate how well they understood underlying principles. Inquiry prompts boosted understanding rules relating fundamental principles represented in the simulation. In this context, it is important to note rule application items did not specifically measure understanding of terms or formulas for laws commonly presented in expository texts. The analysis using knowledge clusters revealed in the group that only received the prompts, more participants than expected were unable to acquire knowledge of basic terms related to the tasks (i.e., current, voltage, and voltage drop) and Ohm’s law. These results suggest that, while participants may have understood underlying principles of the simulation model, the intervention did not necessarily facilitate acquiring conceptual knowledge available in expository texts.
However, there was no effect observed for principle-based self-explanation guidance nor an additional effect of combining inquiry prompts and principle-based self-explanation guidance. Hypotheses 1-b and 1-c were not supported. There are several possible interpretations for why principle-based SE prompts were not effective. First, we interpret this from the perspective of cognitive load and resource allocation. A previous study reported an increase in cognitive load due to self-explanation prompts that asked participants to integrate multiple representations (van der Meij and de Jong, 2011). Learners who read explanations of terms before engaging in inquiry learning may have attempted to recall and relate what they learned from that early access as they worked with the simulation. Resources dedicated to that cognitive work rather than learning from the simulation might depress knowledge construction.
A second possibility is some learners failed to discover rules within the simulation in the first place. Unlike example-based learning where learners are provided with correct concrete examples to explain, in inquiry learning, the subject of explanation—the discovered rules—may contain errors. Incorrect self-explanation can have no effect or even hinder learning outcomes (Rittle-Johnson et al., 2017; Berthold and Renkl, 2009). However, in inquiry learning rooted in the constructivist approach (de Jong and van Joolingen, 1998; Mayer, 2004; White and Frederiksen, 1998), the correct answer is not provided when learners self-explain answers. One way to avoid adverse effects of such incorrect self-explanation may be to teach correct rules after learners have completed inquiry tasks in the simulation and before they are asked to integrate ideas from expository texts in their explanations of theories underlying the rules. Further research is warranted to improve effects of self-explanation in inquiry learning when learners strive to integrate results of a simulation and expository texts.
Lastly, even when learners successfully discover rules by working with the simulation, they might struggle to integrate them effectively with information presented in supplemental text. For example, in the case of a series circuit with only one resistor, increasing resistance does not change voltage drop. According to Ohm’s law, increased resistance would produce decreased current and a constant voltage drop. However, if learners only understood “resistance and voltage are proportional,” they might have mistakenly understood that an increase in resistance would increase voltage drop, despite discovering in the simulation that voltage drop remained constant. Insufficient integration with rules based on the text may interfere with potentially beneficial effects of the interventions.
4.2 Moderation effect of prior knowledge
The impact of our interventions on learning rules was moderated by learners’ prior knowledge. As expected, the effect of inquiry prompts was stronger for learners with more prior knowledge in situations where no guidance was provided to encourage access to information to compensate for the low level of prior knowledge. This suggests Hypothesis 2 is supported and is consistent with previous research findings that directive guidance is effective for learners with higher knowledge (Roll et al., 2018), and that there is a positive correlation between prompt viewing and knowledge gain (van Dijk et al., 2016). However, learners with low prior knowledge exhibited similar posttest performance to the group that received principle-based self-explanation guidance, while the effect of the inquiry prompts on learners with relatively high prior knowledge was pronounced. The inquiry prompts in this study were designed to promote understanding not only of bivariate relationships, such as terminal voltage and current being proportional, but also of more complex rules such as the sum of the voltage drops across multiple resistors matching the value of terminal voltage. For example, the application prompt was “What is the relationship between the voltage drop across each resistor and the terminal voltage?” To answer this correctly, learners were required to correctly measure the value of voltage drops, notice that as the resistor was manipulated the value of one voltage drop changed in the exact opposite direction from the value of the other voltage drop, and notice the pattern by summing the two values. With little prior knowledge, students stumbled at one of these multiple steps, which led to low posttest performance. These results suggest prompts worked only when learners had some degree of prior knowledge to take intended actions.
4.3 Information use and learning outcomes
In this study, principle-based self-explanation guidance increased access to and reading time of expository texts, but there was no mediating effect through which it enhanced posttest performance. This suggests Hypothesis 3 was not supported. However, principle-based self-explanation guidance overcame the problem of learners not accessing on-demand information texts during inquiry learning (Swaak and de Jong, 2001; Hulshof and de Jong, 2006). Indeed, in the answering activity, learners who received principle-based self-explanation guidance spent more time, an average of 5 min, on the expository text; thus setting the stage to interpret rules discovered during inquiry tasks. In contrast, learners without the intervention spent on average less than one minute on the expository text, and 34 out of 40 learners never accessed it during the answering activity. In previous research, rule discovery has been commonly regarded as the goal of inquiry learning. The importance of principle-based self-explanation to construct integrated conceptual knowledge should also be emphasized.
The association between time spent reading domain information and posttest performance was not confirmed, which is consistent with van der Graaf et al. (2020), where informational texts included advice on inquiry processes as well as domain information. We did not find that the more the informational text was reviewed, the more positive the effect on learning, as reported by (Manlove et al. 2007; 2009). The coefficient relating posttest scores to time spent reading the text was effectively zero (b = −0.01, SE = 0.12). To understand the absence of an effect of reading time and explain the low achievement observed in some participants, we might consider four plausible patterns of learner behavior mapped by a 2 × 2 table representing (a) metacognitively judged need to consult domain information and (b) reading time, In the first pattern, both perceived need and reading time are low. These learners rarely used expository texts because their prior knowledge was relatively high. Their posttest performance could be high. In the second pattern, the need was high but the reading time was low. Learners did not sufficiently engage with expository texts despite their limited prior knowledge. As a result, these learners tackled inquiry tasks without a thorough grasp of the basic knowledge, leading to lower posttest performance. In the third pattern, the need was low but the reading time was high. Learners read expository texts carefully even though they were already familiar with the terms. They might have felt uncertain about their understanding and read the texts to confirm correctness, resulting in high posttest performance. In the final pattern, both the need and reading time were high. In this case, learners were aware of their lack of knowledge and actively used expository texts. However, if they could not attain a sufficient understanding after reading the texts, their posttest scores could still be low. To explore these distinct patterns further, a detailed analysis of learning behavior is necessary.
4.4 Implications for system development of adaptive guidance
Findings of this study have implications for researchers who develop adaptive guidance in simulation-based inquiry learning. For just-in-time inquiry prompts to be effective, some prior knowledge is required. However, promoting use of on-demand supplementary texts by self-explanation prompts may increase cognitive load which hinders their effectiveness. In other words, when trying to discover relationships among variables by inquiring in a simulation and examining conceptual information in text, interventions that directly contribute to each goal may sometimes mutually interfere. To avoid this, we predict it would be beneficial for teachers or video lecturers in simulation-based learning environments to present essential concepts related to the simulation beforehand. This should minimize learners’ need to consult supplemental information. This conjecture requires further investigation to determine whether it is more effective to teach only key concepts or, as Wecker et al. (2013) suggested, to include the background theory as well. During inquiry, the focus should be on identifying relationships between variables, without providing detailed information about terms, and developing a comprehensive understanding of relationships within the simulation. After completing inquiry tasks, to increase correct self-explanation, answers to inquiry tasks should be provided. Subsequently, teachers or video lectures can instruct the principle and theory behind the simulation model (e.g., Ohm’s law). Subsequently, principle-based self-explanation (Renkl, 2014) can be encouraged so students connect rules among variables discovered in the simulation and the theory. Further research is needed to determine how best to combine inquiry learning and direct instruction.
4.5 Limitations and future directions
In this study, we focused on effects of just-in-time inquiry prompts in facilitating spontaneous use of inquiry learning strategies and principle-based self-explanation guidance in promoting integration of different types of information. However, changes in inquiry behavior during learning and learners’ information use and integration process were not directly examined. For instance, the reduction in the number of inquiry prompts between tasks 2 and 3 suggests that learners may have started using some inquiry learning skills spontaneously. A detailed analysis of learning videos, including their think-aloud protocols and manipulations on the learning website could provide insights into these behavioral changes and should be considered in future research.
The learning webpage used in this study was limited in the types of behavior it could log, such as accessing the information tab, and could not log other potentially beneficial data, such as participants’ manipulation of the simulation and highlighting of text in the information tab. Previous studies have argued for detailed logging of participants’ interactions (Winne, 2020, 2022), and that will be necessary to support more complete and precise process analyses in future research.
Also, the experimenter monitored the learning situation and made judgments about providing just-in-time inquiry prompts, resulting in some variation even though a pre-constructed prompt list was followed. Future research on intelligent support systems able to tailor just-in-time inquiry prompts is necessary.
Related to the previous point, in this study, think-aloud training (Noushad et al., 2024) was not conducted due to the time constraints of the experiment. However, since the provision of just-in-time inquiry prompts was based on both participants’ manipulations on the learning webpage and their utterances, factors such as the frequency of utterances may have influenced the experimenter’s decision to provide prompts. To minimize the variation of guidance described above, it may be beneficial to conduct think-aloud training before engaging in tasks in future studies.
Data availability statement
The raw data supporting the conclusions of this article will be made available by the authors, without undue reservation.
Ethics statement
The studies involving humans were approved by the Research Ethics Board at Simon Fraser University (#30001138). The studies were conducted in accordance with the local legislation and institutional requirements. The participants provided their written informed consent to participate in this study.
Author contributions
MF: Conceptualization, Data curation, Formal analysis, Funding acquisition, Investigation, Methodology, Project administration, Resources, Software, Visualization, Writing – original draft. JN: Conceptualization, Funding acquisition, Methodology, Supervision, Writing – review & editing. PW: Conceptualization, Funding acquisition, Methodology, Supervision, Writing – review & editing.
Funding
The authors declare that financial support was received for the research, authorship, and/or publication of this article. This research was supported by the Social Sciences and Humanities Research Council of Canada (SSHRC) [grant number: SSHRC 435-2019-0458].
Conflict of interest
The authors declare that the research was conducted in the absence of any commercial or financial relationships that could be construed as a potential conflict of interest.
Publisher’s note
All claims expressed in this article are solely those of the authors and do not necessarily represent those of their affiliated organizations, or those of the publisher, the editors and the reviewers. Any product that may be evaluated in this article, or claim that may be made by its manufacturer, is not guaranteed or endorsed by the publisher.
Supplementary material
The Supplementary Material for this article can be found online at: https://www.frontiersin.org/articles/10.3389/feduc.2024.1446941/full#supplementary-material
Footnotes
- ^ We conducted a preliminary power analysis using G*Power version 3.1.9.6 (Faul et al., 2009) to estimate the required sample size. The analysis was based on data from Hajian et al. (2021; N = 13), the most similar previous study to the intervention in this study, in which they compared the just-in-time prompts group to the control group. The effect size observed by Hajian et al. (2021) was 1.72, which is considered a large effect according to Cohen (1992) criteria. With a significance criterion of α = 0.05, power of 0.80, and f = 0.4 (large effect), the minimum sample size required to detect this effect size in an ANCOVA analysis is N = 52.
- ^ https://phet.colorado.edu
- ^ We administered a four-item test measuring participants’ knowledge of basic terms (current, voltage, voltage drop, Ohm’s law), but we could not confirm sufficient internal consistency reliability. Therefore we do not analyze these data here.
References
Alfieri, L., Brooks, P. J., Aldrich, N. J., and Tenenbaum, H. R. (2011). Does discovery-based instruction enhance learning? J. Educ. Psychol. 103, 1–18. doi: 10.1037/a0021017
Antonio, R. P., and Castro, R. R. (2023). Effectiveness of virtual simulations in improving secondary students’ achievement in physics: A meta-analysis. Int. J. Instruct. 16, 533–556. doi: 10.29333/iji.2023.16229a
Atkinson, R. K., Renkl, A., and Merrill, M. M. (2003). Transitioning from studying examples to solving problems: Combining fading with prompting fosters learning. J. Educ. Psychol. 95, 774–785.
Banda, H. J., and Nzabahimana, J. (2023). The impact of physics education technology (PhET) interactive simulation-based learning on motivation and academic achievement among Malawian physics students. J. Sci. Educ. Technol. 32, 127–141. doi: 10.1007/s10956-022-10010-3
Bell, B. S., and Kozlowski, S. W. (2002). Adaptive guidance: Enhancing self-regulation, knowledge, and performance in technology-based training. Pers. Psychol. 55, 267–306. doi: 10.1111/j.1744-6570.2002.tb00111.x
Berthold, K., and Renkl, A. (2009). Instructional AIDS to support a conceptual understanding of multiple representations. J. Educ. Psychol. 101, 70–87. doi: 10.1037/a0013247
Berthold, K., Eysink, T. H. S., and Renkl, A. (2009). Assisting self-explanation prompts are more effective than open prompts when learning with multiple representations. Instruct. Sci. 37, 345–363. doi: 10.1007/s11251-008-9051-z
Blake, C., and Scanlon, E. (2007). Reconsidering simulations in science education at a distance: Features of effective use. J. Comput. Assist. Learn. 23, 491–502. doi: 10.1111/j.1365-2729.2007.00239.x
Chang, K. E., Chen, Y. L., Lin, H. Y., and Sung, Y. T. (2008). Effects of learning support in simulation-based physics learning. Comput. Educ. 51, 1486–1498. doi: 10.1016/j.compedu.2008.01.007
Chi, M. T. H., Siler, S. A., Jeong, H., Yamauchi, T., and Hausmann, R. G. (2001). Learning from human tutoring. Cogn. Sci. 25, 471–533. doi: 10.1207/s15516709cog2504_1
D’Angelo, C., Rutstein, D., Harris, C., Bernard, R., Borokhovski, E., and Haertel, G. (2014). Simulations for STEM learning: Systematic review and meta-analysis. Menlo Park, CA: SRI International.
Dai, C. P., and Ke, F. (2022). Educational applications of artificial intelligence in simulation-based learning: A systematic mapping review. Comput. Educ. Artif. Intell. 3:100087. doi: 10.1016/j.caeai.2022.100087
Dawson, R. (2011). How significant is a boxplot outlier? J. Stat. Educ. 19:9610. doi: 10.1080/10691898.2011.11889610
de Jong, T. (2019). Moving towards engaged learning in STEM domains; there is no simple answer, but clearly a road ahead. J. Comput. Assist. Learn. 35, 153–167. doi: 10.1111/jcal.12337
de Jong, T., and Lazonder, A. W. (2014). “The guided discovery learning principle in multimedia learning,” in The Cambridge handbook of multimedia learning, ed. R. Mayer (Cambridge: Cambridge University Press), 371–390. doi: 10.1017/CBO9781139547369.019
de Jong, T., and Njoo, M. (1992). “Learning and instruction with computer simulations: Learning processes involved,” in Computer-based learning environments and problem solving, eds E. De Corte, M. C. Linn, H. Mandl, and L. Verschaffel (Berlin: Springer), 411–427. doi: 10.1007/978-3-642-77228-3_19
de Jong, T., and van Joolingen, W. R. (1998). scientific discovery learning with computer simulations of conceptual domains. Rev. Educ. Res. 68, 179–201. doi: 10.3102/00346543068002179
de Jong, T., Lazonder, A. W., Chinn, C. A., Fischer, F., Gobert, J., Hmelo-Silver, C. E., et al. (2023). Let’s talk evidence – The case for combining inquiry-based and direct instruction. Educ. Res. Rev. 39:100536. doi: 10.1016/j.edurev.2023.100536
Eckhardt, M., Urhahne, D., Conrad, O., and Harms, U. (2013). How effective is instructional support for learning with computer simulations? Instruct. Sci. 41, 105–124. doi: 10.1007/s11251-012-9220-y
Elme, L., Jørgensen, M. L. M., Dandanell, G., Mottelson, A., and Makransky, G. (2022). Immersive virtual reality in STEM: Is IVR an effective learning medium and does adding self-explanation after a lesson improve learning outcomes? Educ. Technol. Res. Dev. 70, 1601–1626. doi: 10.1007/s11423-022-10139-3
Faul, F., Erdfelder, E., Buchner, A., and Lang, A.-G. (2009). Statistical power analyses using G*Power 3.1: Tests for correlation and regression analyses. Behav. Res. Methods 41, 1149–1160. doi: 10.3758/BRM.41.4.1149
Fukaya, T. (2013). Explanation generation, not explanation expectancy, improves metacomprehension accuracy. Metacogn. Learn. 8, 1–18. doi: 10.1007/s11409-012-9093-0
Fukuda, M., Hajian, S., Jain, M., Liu, A. L., Obaid, T., Nesbit, J. C., et al. (2022). Scientific inquiry learning with a simulation: Providing within-task guidance tailored to learners’ understanding and inquiry skill. Int. J. Sci. Educ. 44, 1021–1043. doi: 10.1080/09500693.2022.2062799
Furtak, E. M., Shavelson, R. J., Shemwell, J. T., and Figueroa, M. (2012). To teach or not to teach through inquiry. J. Child Sci. Integr. Cogn. Dev. Educ. Sci. 2001, 227–244. doi: 10.1037/13617-011
Gerard, L. F., Ryoo, K., McElhaney, K. W., Liu, O. L., Rafferty, A. N., and Linn, M. C. (2016). Automated guidance for student inquiry. J. Educ. Psychol. 108, 60–81. doi: 10.1037/edu0000052
Gobert, J. D., Moussavi, R., Li, H., Sao Pedro, M., and Dickler, R. (2018). “Real-time scaffolding of students’ online data interpretation during inquiry with inq-its using educational data mining,” in Cyber-physical laboratories in engineering and science education, eds A. K. M. Azad, M. Auer, A. Edwards, and T. de Jong (Cham: Springer International Publishing), 191–217. doi: 10.1007/978-3-319-76935-6_8
Gobert, J. D., Sao Pedro, M., Raziuddin, J., and Baker, R. S. (2013). From log files to assessment metrics: Measuring students’ science inquiry skills using educational data mining. J. Learn. Sci. 22, 521–563. doi: 10.1080/10508406.2013.837391
Graesser, A., and McNamara, D. (2010). Self-regulated learning in learning environments with pedagogical agents that interact in natural language. Educ. Psychol. 45, 234–244. doi: 10.1080/00461520.2010.515933
Griffin, T. D., Wiley, J., and Thiede, K. W. (2008). Individual differences, rereading, and self-explanation: Concurrent processing and cue validity as constraints on metacomprehension accuracy. Mem. Cogn. 36, 93–103. doi: 10.3758/MC.36.1.93
Hajian, S., Jain, M., Liu, A. L., Obaid, T., Fukuda, M., Winne, P. H., et al. (2021). Enhancing scientific discovery learning by just-in-time prompts in a simulation-assisted inquiry environment. Eur. J. Educ. Res. 10, 989–1007. doi: 10.12973/EU-JER.10.2.989
Hayes, A. F. (2022). Introduction to mediation, moderation, and conditional process analysis: A regression-based approach, 3rd Edn. New York, NY: The Guilford Press.
Hefter, M. H., Renkl, A., Riess, W., Schmid, S., Fries, S., and Berthold, K. (2015). Effects of a training intervention to foster precursors of evaluativist epistemological understanding and intellectual values. Learn. Instruct. 39, 11–22. doi: 10.1016/j.learninstruc.2015.05.002
Hiller, S., Rumann, S., Berthold, K., and Roelle, J. (2020). Example-based learning: Should learners receive closed-book or open-book self-explanation prompts? Instruct. Sci. 48, 623–649. doi: 10.1007/s11251-020-09523-4
Hulshof, C. D., and de Jong, T. (2006). Using just-in-time information to support scientific discovery learning in a computer-based simulation. Interact. Learn. Environ. 14, 79–94. doi: 10.1080/10494820600769171
Kabigting, L. D. C. (2021). Computer simulation on teaching and learning of selected topics in physics. Eur. J. Interact. Multimedia Educ. 2:e02108. doi: 10.30935/ejimed/10909
Kelley, J. F. (1983). Natural language and computers: Six empirical steps for writing an easy-to-use computer application [Ph.D. thesis]. Baltimore, MD: Johns Hopkins University.
Kerawalla, L., Littleton, K., Scanlon, E., Jones, A., Gaved, M., Collins, T., et al. (2013). Personal inquiry learning trajectories in geography: Technological support across contexts. Interact. Learn. Environ. 21, 497–515. doi: 10.1080/10494820.2011.604036
Klahr, D., and Nigam, M. (2004). The equivalence of learning paths in early science instruction. Psychol. Sci. 15, 661–667. doi: 10.1111/j.0956-7976.2004.00737.x
Kranz, J., Baur, A., and Möller, A. (2023). Learners’ challenges in understanding and performing experiments: A systematic review of the literature. Stud. Sci. Educ. 59, 321–367. doi: 10.1080/03057267.2022.2138151
Kuang, X., Eysink, T. H. S., and de Jong, T. (2023). Presenting domain information or self-exploration to foster hypothesis generation in simulation-based inquiry learning. J. Res. Sci. Teach. 61, 70–102. doi: 10.1002/tea.21865
Kulik, J. A., and Fletcher, J. D. (2016). Effectiveness of intelligent tutoring systems: A meta-analytic review. Rev. Educ. Res. 86, 42–78. doi: 10.3102/0034654315581420
Lazonder, A. W. (2014). “Inquiry learning,” in Handbook of research on educational communications and technology, 4th Edn, eds J. M. Spector, M. D. Merrill, J. Elen, and M. J. Bishop (New York, NY: Springer), 453–464. doi: 10.1007/978-1-4614-3185-5
Lazonder, A. W., and Harmsen, R. (2016). Meta-analysis of inquiry-based learning. Rev. Educ. Res. 86, 681–718. doi: 10.3102/0034654315627366
Lazonder, A. W., Hagemans, M. G., and de Jong, T. (2010). Offering and discovering domain information in simulation-based inquiry learning. Learn. Instruct. 20, 511–520. doi: 10.1016/j.learninstruc.2009.08.001
Lazonder, A. W., Wilhelm, P., and van Lieburg, E. (2009). Unraveling the influence of domain knowledge during simulation-based inquiry learning. Instruct. Sci. 37, 437–451. doi: 10.1007/s11251-008-9055-8
Leutner, D. (1993). Guided discovery learning with computer-based simulation games: Effects of adaptive and non-adaptive instructional support. Learn. Instruct. 3, 113–132. doi: 10.1016/0959-4752(93)90011-N
Li, Y. H., Su, C. Y., and Ouyang, F. (2023). Integrating self-explanation into simulation-based physics learning for 7th graders. J. Sci. Educ. Technol. 10:0123456789. doi: 10.1007/s10956-023-10082-9
Linn, M. C., Eylon, B. S., Kidron, A., Gerard, L., Toutkoushian, E., Ryoo, K. K., et al. (2018). Knowledge integration in the digital age: Trajectories, opportunities and future directions. Proc. Int. Conf. Learn. Sci. 2, 1259–1266.
Manlove, S., Lazonder, A. W., and De Jong, T. (2007). Software scaffolds to promote regulation during scientific inquiry learning. Metacogn. Learn. 2, 141–155. doi: 10.1007/s11409-007-9012-y
Manlove, S., Lazonder, A. W., and de Jong, T. (2009). Trends and issues of regulative support use during inquiry learning: Patterns from three studies. Comput. Hum. Behav. 25, 795–803. doi: 10.1016/j.chb.2008.07.010
Mayer, R. E. (2004). Should there be a three-strikes rule against pure discovery learning? Am. Psychol. 59, 14–19. doi: 10.1037/0003-066X.59.1.14
Mayer, R. E. (2014). “Cognitive theory of multimedia learning,” in The Cambridge handbook of multimedia learning, 2nd Edn, ed. R. E. Mayer (Cambridge: Cambridge University Press), 43–71. doi: 10.1017/CBO9781139547369.005
Meyers, L. S., Gamst, G., and Guarino, A. J. (2016). Applied multivariate research: Design and interpretation. Sage publications. Thousand Oaks, CA.
Njoo, M., and de Jong, T. (1993). Exploratory learning with a computer simulation for control theory: Learning processes and instructional support. J. Res. Sci. Teach. 30, 821–844. doi: 10.1002/tea.3660300803
Noushad, B., Van Gerven, P. W. M., and de Bruin, A. B. H. (2024). Twelve tips for applying the think-aloud method to capture cognitive processes. Med. Teach. 46, 892–897. doi: 10.1080/0142159X.2023.2289847
Pedaste, M., Mäeots, M., Siiman, L. A., de Jong, T., van Riesen, S. A. N., Kamp, E. T., et al. (2015). Phases of inquiry-based learning: Definitions and the inquiry cycle. Educ. Res. Rev. 14, 47–61. doi: 10.1016/j.edurev.2015.02.003
Renkl, A. (1997). Learning from worked-out examples: A study on individual differences. Cogn. Sci. 21, 1–29. doi: 10.1016/S0364-0213(99)80017-2
Renkl, A. (2014). Toward an instructionally oriented theory of example-based learning. Cogn. Sci. 38, 1–37. doi: 10.1111/cogs.12086
Rittle-Johnson, B., Loehr, A. M., and Durkin, K. (2017). Promoting self-explanation to improve mathematics learning: A meta-analysis and instructional design principles. ZDM 49, 599–611. doi: 10.1007/s11858-017-0834-z
Roelle, J., and Renkl, A. (2020). Does an option to review instructional explanations enhance example-based learning? It depends on learners’ academic self-concept. J. Educ. Psychol. 112, 131–147. doi: 10.1037/edu0000365
Roelle, J., Hiller, S., Berthold, K., and Rumann, S. (2017). Example-based learning: The benefits of prompting organization before providing examples. Learn. Instruct. 49, 1–12. doi: 10.1016/j.learninstruc.2016.11.012
Roll, I., Butler, D., Yee, N., Welsh, A., Perez, S., Briseno, A., et al. (2018). Understanding the impact of guiding inquiry: The relationship between directive support, student attributes, and transfer of knowledge, attitudes, and behaviours in inquiry learning. Instruct. Sci. 46, 77–104. doi: 10.1007/s11251-017-9437-x
Rutten, N., Van Joolingen, W. R., and Van Der Veen, J. T. (2012). The learning effects of computer simulations in science education. Comput. Educ. 58, 136–153. doi: 10.1016/j.compedu.2011.07.017
Schworm, S., and Renkl, A. (2007). Learning argumentation skills through the use of prompts for self-explaining examples. J. Educ. Psychol. 99, 285–296. doi: 10.1037/0022-0663.99.2.285
Slotta, J. D., and Linn, M. C. (2009). WISE Science: Web-based Inquiry in the Classroom. Technology, education–connections. New York, NY: Amsterdam Avenue, 10027.
Smetana, L. K., and Bell, R. L. (2012). Computer simulations to support science instruction and learning: A critical review of the literature. Int. J. Sci. Educ. 34, 1337–1370. doi: 10.1080/09500693.2011.605182
Sun, Y., Yan, Z., and Wu, B. (2022). How differently designed guidance influences simulation-based inquiry learning in science education: A systematic review. J. Comput. Assist. Learn. 38, 960–976. doi: 10.1111/jcal.12667
Swaak, J., and de Jong, T. (2001). Learner vs. system control in using online support for simulation-based discovery learning. Learn. Environ. Res. 4, 217–241. doi: 10.1023/A:1014434804876
van der Graaf, J., Segers, E., and de Jong, T. (2020). Fostering integration of informational texts and virtual labs during inquiry-based learning. Contemp. Educ. Psychol. 62:101890. doi: 10.1016/j.cedpsych.2020.101890
van der Meij, J., and de Jong, T. (2011). The effects of directive self-explanation prompts to support active processing of multiple representations in a simulation-based learning environment. J. Comput. Assist. Learn. 27, 411–423. doi: 10.1111/j.1365-2729.2011.00411.x
van Dijk, A. M., Eysink, T. H. S., and de Jong, T. (2016). Ability-related differences in performance of an inquiry task: The added value of prompts. Learn. Individ. Differ. 47, 145–155. doi: 10.1016/j.lindif.2016.01.008
van Merriënboer, J. J. G., and Kester, L. (2014). “The four-component instructional design model: Multimedia principles in environments for complex learning,” in The Cambridge handbook of multimedia learning, ed. R. E. Mayer (Cambridge: Cambridge University Press), 104–148.
van Merriënboer, J. J. G., and Kirschner, P. A. (2019). 4C/ID in the context of instructional design and the learning sciences. Int. Handb. Learn. Sci. 10144, 169–179. doi: 10.4324/9781315617572-17
van Riesen, S., Gijlers, H., Anjewierden, A., and de Jong, T. (2018a). Supporting learners’ experiment design. Educ. Technol. Res. Dev. 66, 475–491. doi: 10.1007/s11423-017-9568-4
van Riesen, S., Gijlers, H., Anjewierden, A., and de Jong, T. (2018b). The influence of prior knowledge on experiment design guidance in a science inquiry context. Int. J. Sci. Educ. 40, 1327–1344.
VanLehn, K., Siler, S., Murray, C., Yamauchi, T., and Baggett, W. B. (2003). Why do only some events cause learning during human tutoring? Cogn. Instruct. 21, 209–249. doi: 10.1207/S1532690XCI2103_01
Veenman, M. V. J. (2011). “Learning to self-monitor and self-regulate,” in Handbook of research on learning and instruction, eds R. Mayer and P. Alexander (New York, NY: Routledge), 197–218.
Vorholzer, A., and von Aufschnaiter, C. (2019). Guidance in inquiry-based instruction–an attempt to disentangle a manifold construct. Int. J. Sci. Educ. 41, 1562–1577. doi: 10.1080/09500693.2019.1616124
Weaver, J. P., Chastain, R. J., DeCaro, D. A., and DeCaro, M. S. (2018). Reverse the routine: Problem solving before instruction improves conceptual knowledge in undergraduate physics. Contemp. Educ. Psychol. 52, 36–47. doi: 10.1016/j.cedpsych.2017.12.003
Wecker, C., Rachel, A., Heran-Dörr, E., Waltner, C., Wiesner, H., and Fischer, F. (2013). Presenting theoretical ideas prior to inquiry activities fosters theory-level knowledge. J. Res. Sci. Teach. 50, 1180–1206. doi: 10.1002/tea.21106
White, B. Y., and Frederiksen, J. R. (1998). Inquiry, modeling, and metacognition: Making science accessible to all students. Cogn. Instruct. 16, 3–118. doi: 10.1207/s1532690xci1601_2
Winne, P. H. (1995). Inherent details in self-regulated learning. Educ. Psychol. 30, 173–187. doi: 10.1207/s15326985ep3004_2
Winne, P. H. (1996). A metacognitive view of individual differences in self-regulated learning. Learn. Individ. Differ. 8, 327–353. doi: 10.1016/S1041-6080(96)90022-9
Winne, P. H. (2020). Construct and consequential validity for learning analytics based on trace data. Comput. Hum. Behav. 112:106457. doi: 10.1016/j.chb.2020.106457
Winne, P. H. (2022). Modeling self-regulated learning as learners doing learning science: How trace data and learning analytics help develop skills for self-regulated learning. Metacogn. Learn. 17, 773–791. doi: 10.1007/s11409-022-09305-y
Wittwer, J., and Renkl, A. (2010). How effective are instructional explanations in example-based learning? A meta-analytic review. Educ. Psychol. Rev. 22, 393–409. doi: 10.1007/s10648-010-9136-5
Wu, H.-K., and Wu, C.-L. (2011). Exploring the development of fifth graders’ practical epistemologies and explanation skills in inquiry-based learning classrooms. Res. Sci. Educ. 41, 319–340. doi: 10.1007/s11165-010-9167-4
Zimmerman, C. (2007). The development of scientific thinking skills in elementary and middle school. Dev. Rev. 27, 172–223. doi: 10.1016/j.dr.2006.12.001
Keywords: simulation-based inquiry learning, expository texts, adaptive guidance, just-in-time prompts, self-explanation
Citation: Fukuda M, Nesbit JC and Winne PH (2024) Effects of just-in-time inquiry prompts and principle-based self-explanation guidance on learning and use of domain texts in simulation-based inquiry learning. Front. Educ. 9:1446941. doi: 10.3389/feduc.2024.1446941
Received: 10 June 2024; Accepted: 27 August 2024;
Published: 25 September 2024.
Edited by:
Niwat Srisawasdi, Khon Kaen University, ThailandReviewed by:
Rosanda Pahljina-Reinić, University of Rijeka, CroatiaKeiichi Kobayashi, Shizuoka University, Japan
Copyright © 2024 Fukuda, Nesbit and Winne. This is an open-access article distributed under the terms of the Creative Commons Attribution License (CC BY). The use, distribution or reproduction in other forums is permitted, provided the original author(s) and the copyright owner(s) are credited and that the original publication in this journal is cited, in accordance with accepted academic practice. No use, distribution or reproduction is permitted which does not comply with these terms.
*Correspondence: Mari Fukuda, ZnVrdWRhLm1hcmkuNnlAa3lvdG8tdS5hYy5qcA==
†Present address: Mari Fukuda, JSPS Research Fellow, Graduate School of Education, Kyoto University, Kyoto, Japan