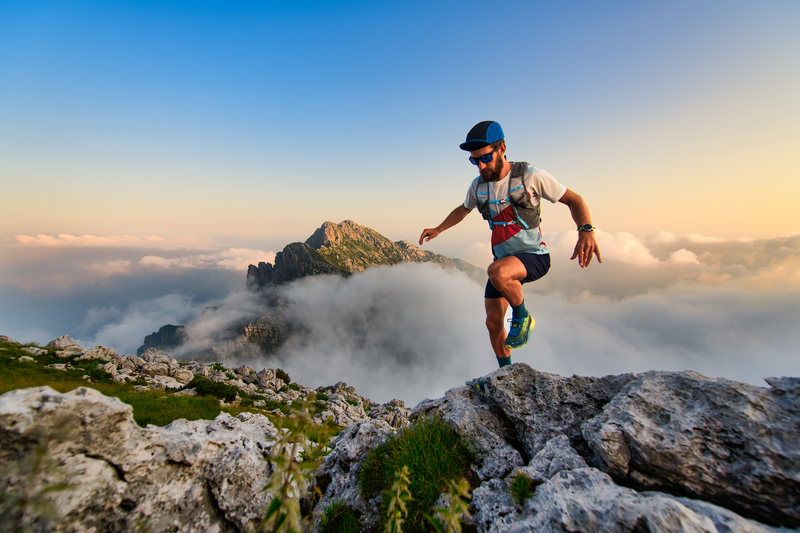
95% of researchers rate our articles as excellent or good
Learn more about the work of our research integrity team to safeguard the quality of each article we publish.
Find out more
REVIEW article
Front. Educ. , 06 November 2024
Sec. Assessment, Testing and Applied Measurement
Volume 9 - 2024 | https://doi.org/10.3389/feduc.2024.1442833
Background: Concept inventories (CIs) have become widely used tools for assessing students’ learning and assisting with educational decisions. Over the past three decades, CI developers have utilized various design approaches and methodologies. As a result, it can be challenging for those developing new CIs to identify the most effective and appropriate methods and approaches. This scoping review aimed to identify and map key design stages, summarize methodologies, identify design gaps and provide guidance for future efforts in the development and validation of CI tools.
Methods: A preliminary literature review combined theoretical thematic analysis (deductive, researcher-driven) focusing on specific data aspects, and inductive thematic analysis (data-driven), using emerging themes independent of specific research questions or theoretical interests. Expert discussions complemented the analysis process.
Results: The scoping review analyzed 106 CI articles and identified five key development stages: define the construct, determine and validate content domain; identify misconceptions; item formation and response processes design; test item selection and validation; and test application and refinement. A descriptive design model was developed using a mixed-method approach, incorporating expert input, literature review, student-oriented analysis, and statistical tests. Various psychometric assessments were employed to validate the test and its items. Substantial gaps were noted in defining and determining the validity and reliability of CI tools, and in the evidence required to establish these attributes.
Conclusion: The growing interest in utilizing CIs for educational purposes has highlighted the importance of identifying and refining the most effective design stages and methodologies. CI developers need comprehensive guidance to establish and evaluate the validity and reliability of their instruments. Future research should focus on establishing a unified typology of CI instrument validity and reliability requirements, as well as the types of evidence needed to meet these standards. This effort could optimize the effectiveness of CI tools, foster a cohesive evaluation approach, and bridge existing gaps.
In the dynamic landscape of education, improving comprehension is a primary goal, despite the difficulties associated with evaluating and enhancing students’ understanding (Black and Wiliam, 1998; Shepard, 2000). There is a notable emphasis on assessing comprehension in scientific fields, leading to the widespread use of concept inventories (CIs) for this purpose (Sands et al., 2018). CIs are designed to evaluate students’ conceptual understanding of and determine the probability that a student uses a specific conceptual model to approach the questions, thereby gauging deeper understanding (Klymkowsky et al., 2003; Klymkowsky and Garvin-Doxas, 2008).
CIs are designed based on learners’ misconceptions (Arthurs and Marchitto, 2011; Hestenes et al., 1992), and were developed to overcome the limitations of traditional, simple tests that often fail to diagnose students’ misunderstandings accurately. As highlighted by Sadler (1998), psychometric models and distractor-driven assessment instruments were designed to reconcile qualitative insights with more precise measurements of concept comprehension. CIs are essential in measuring conceptual understandings (Beichner, 1994; Hestenes et al., 1992), identifying misconceptions, and facilitating evidence-based instructional strategies (D'Avanzo, 2008; Adams and Wieman, 2011; Klymkowsky and Garvin-Doxas, 2008). Moreover, they serve as benchmarks for comparing interventions, assessing instructional effectiveness (Smith and Tanner, 2010), and contributing to educational research (Adams and Wieman, 2011) and curriculum development decisions (D'Avanzo, 2008).
The use of CIs has surged, with approximately 60% developed in the past decade. This growth has been attributed to CIs’ value in identifying misconceptions and providing insights into improving educational outcomes (Furrow and Hsu, 2019). Additionally, CIs can effectively assess the impact of various learning models by evaluating students’ conceptual understanding (Freeman et al., 2014; Adams and Wieman, 2011), and the effectiveness of teaching approaches (Bailey et al., 2012), thereby supporting enhanced educational outcomes (Sands et al., 2018). These tools employ systematic, theory-driven models rooted in construct validity (Messick, 1989a,b), cognitive psychology (Anderson, 2005), and educational measurement (Baker and Kim, 2004) to assess comprehension and learning outcomes (Sands et al., 2018; Furrow and Hsu, 2019).
Despite CIs offering various advantages, they also have limitations in capturing students’ critical thinking or understanding (Knight, 2010; Smith and Tanner, 2010). The multiple-choice question (MCQ) format, in particular, may lead to inflated scores due to guessing and varying student motivation (Furrow and Hsu, 2019; Sands et al., 2018). To address these issues, designing CIs with a construct-based approach (Cakici and Yavuz, 2010; Awan, 2013), applying multiple comparisons over time (Summers et al., 2018; Price et al., 2014), and utilizing multi-tier MCQs can help assess students’ understanding of propositional statements and their reasoning (Caleon and Subramaniam, 2010; Haslam and Treagust, 1987). Furthermore, integrating CIs with approaches like three-dimensional learning (3-DL) can enhance their effectiveness by providing a broader context for evaluating students’ application of concepts and offering a more comprehensive approach to addressing both specific and broader conceptual challenges (Cooper et al., 2024).
To effectively evaluate learning gains using CIs, these tools must meet specific criteria concerning validity, standardization, and longitudinal assessment (McGrath et al., 2015). However, the absence of a universally agreed-upon definition for what constitutes a CI (Epstein, 2013) has led to varied employment of theoretical models and approaches in their design and validation. These approaches differ in their emphasis at each stage, with some receiving more attention than others (Wren and Barbera, 2013). This variability highlights the challenge of identifying crucial development and validation stages and selecting appropriate methodologies. Consequently, differences arise in the development, utilization, and interpretation of CIs across test designers (Sands et al., 2018).
Over the past three decades, CI designers have adopted a variety of development models and have employed multiple approaches. These models, including those proposed by Adams and Wieman (2011), Treagust (1988), and Libarkin (2008), among others, involve diverse phases and patterns of development, such as formulating questions and responses through literature consultation, student essays and interview analysis, expert judgment, and pilot testing (Bailey et al., 2012; Sands et al., 2018). An early model by Wright et al. (2009) emphasized the value of identifying student misconceptions by analyzing their responses to MCQs. This model involved three main stages encompassing 10 steps to highlight concept description and validation, misconception identification, and design of test items as the core elements of CI development. The authors stressed that unstructured student interviews and free responses are important to uncover alternate conceptions. Many test developers have adopted this model, either fully or partially, in the design of their CIs (Jarrett et al., 2012; Anderson et al., 2002; Ngambeki et al., 2018).
By contrast, another model (Libarkin, 2008) presented an alternative data-driven method that integrated statistical analysis to refine tests and ensure psychometric reliability. This method helped to create reliable and valid CIs by analyzing student performance and item characteristics. The author underscored the pivotal roles of educators and students in crafting assessment tools, which are often overlooked by test development teams. Libarkin also stressed the importance of construct, content and communication validities in developing effective assessment tools. Identifying topics, exploring student misconceptions, generating items, administering tests, and selecting questions are all crucial elements in the design process (Wright et al., 2009).
Alternatively, developing CI can be completed in 3–12 steps, with feedback from both target populations and experts considered essential (Miller et al., 2011; Haladyna and Downing, 2006; Herman and Loui, 2011; Ngambeki et al., 2018; Julie and Bruno, 2020; Rowe and Smaill, 2007). Furthermore, certain authors (Adams and Wieman, 2011) have highlighted the need for item development and validation, emphasizing the alignment with specific learning objectives and assessing targeted concepts. They described four mandatory phases with six general steps to create assessment instruments, which other groups have utilized to develop various science inventory tools (Wasendorf et al., 2024; O’Shea et al., 2016). Overall, these articles demonstrate that there is no single agreed model for CI development, and each has its strengths and limitations.
Further progress in the use of CIs for educational decision-making necessitates a systematic analysis of the published design stages, methods and psychometric evaluations. This review also helps to highlight gaps in existing tools and guides for future research. The findings could optimize the instrument’s utilization in educational research, improve the effectiveness of teaching interventions, and support better identification of learners’ misconceptions and thereby refine STEM teaching practices (Sukarmin and Sarwanto, 2021; Freeman et al., 2014). Additionally, this review emphasizes leveraging the integration of technology and enhancing instruments to provide real-time feedback.
This scoping review aimed to identify key design stages, thematize patterns and trends and summarize the methods and approaches used in developing and validating CIs to guide future efforts. The goal was to characterize the psychometric properties employed in CI instrument validations and outline the evidence required to establish these attributes. Additionally, it aimed to identify gaps in the design, validity, and reliability aspects of CI tools. Ultimately, this review intended to provide resources that support CI tool design endeavors, enhance assessment practices and address existing design gaps in the field.
In line with our scoping review objectives and research questions, we followed the Arksey and O’Malley framework (Arksey and O'Malley, 2005) to structure our scoping review into five stages: (1) delineate the context and research questions; (2) identify pertinent studies; (3) select studies; (4) extract data; and (5) compile, summarize, and report results. Despite appearing as a sequence of linear phases, the process was iterative, allowing flexibility to revisit and refine steps to ensure systematic coverage of literature.
Our scoping review focused exclusively on CI instruments within the educational context and aimed to address the following research questions (RQs):
RQ1: What key stages and thematic trends are employed in the CI development process?
RQ2: What methods and approaches are employed during the development process?
RQ3: What psychometric properties (validity and reliability) are used in validating CIs?
RQ4: What gaps exist in the CI design and validation process?
Initially, we conducted a literature search to identify representative studies and map common themes and concepts. Our comprehensive search strategy included electronic databases: MedLine, EMBASE, PsycINFO, CINAHL Plus, Scopus, Web of Science, and ScienceDirect, supplemented by an advanced Google search. Research librarians guided our search terms and strategies. We employed a combination of keywords (e.g., “concept inventory”), Boolean operators (e.g., “AND,” “OR”), and truncation (e.g., asterisk*). This review did not restrict publication dates but excluded articles in languages other than English due to translation limitations and cost. We probed databases for new publications before data analysis (Supplementary Table S1).
This scoping review focused on qualitative and quantitative research concerning the development of CI tools in STEM disciplines, including medicine, nursing, and health sciences. Criteria for inclusion required CIs to measure conceptual understanding, identify misconceptions in a specific subject area, and aid instructional strategies. Emphasis was on assessing core concepts using standardized methods for consistent scoring.
Included studies presented original methodologies and demonstrated a focus on conceptual understanding, specific course content, and psychometric evidence of validity and reliability. Full-text conference proceedings were considered only if peer-reviewed, Exclusions encompassed non-English or non-peer-reviewed articles and preprints. Articles that were either not yet to be published in peer-reviewed journals or classified as gray literature were excluded. Additionally, tools that assess only computational tasks or basic factual knowledge of the subject (for instance, calculating work or memorizing formulas) were excluded. Tools specifically designed to evaluate courses or licensure exams that encompass a wide range of content, as well as those focused solely on validation and psychometrics were not considered. Quality assessments of the included tools were not conducted. Screening followed PRISMA guidelines (Page et al., 2021), with disagreements resolved through discussion. All citations were managed using EndNote® 20 and Covidence®. The full-text review involved three reviewers; again, any discrepancies were resolved through discussion. Finally, the reference lists of the included studies were searched.
Utilizing Covidence®, accessible to all reviewers, facilitated data extraction aligned with review questions and objectives. A thematic analysis framework, drawing from both theoretical and inductive approaches (Patton, 1990; Braun and Clarke, 2006), was employed to categorize extraction components and identify common vocabularies from literature searches. A pilot test on 25% of articles refined the thematic framework before the main extraction. Methodological components, employed in designing and validating test contents were mapped. Bibliographic details, development and validation methodologies, test characteristics and psychometric properties were extracted. Expert groups conducted independent coding to ensure unbiased data extraction. Initially, a group of two identified the main themes of pilot extraction. Subsequently, another team of three adjusted and refined the extraction process. Expert discussions further enriched methodologies and improved extraction format. A narrative data synthesis approach summarized the findings (Greenhalgh et al., 2005), identifying key themes and sub-themes (Figure 1).
Initial search strategies yielded 4,127 records, with 4,048 from databases and 79 from advanced searches. After removing 1,820 duplicates and ineligible articles, 2,307 citations underwent primary screening. Of these, 1,862 studies were excluded, leaving 445 for full-text retrieval. A total of 106 CI articles met the inclusion criteria for this scoping literature review (Figure 2). Approximately 20% were published in conference proceedings. Most developers (80%) used mixed methods, while 11% used quantitative and 9% used qualitative methods. Undergraduate students comprised 90% of the target population, with some representation from graduate and high school students (Supplementary Table S2). Reviewed CI tools were designed to evaluate several aspects: primarily measured conceptual understanding (80%, n = 85) and identified misconceptions (48%, n = 51), assessed both (40%, n = 42), evaluated learning gains (26%, n = 28) and determined the effectiveness of instructional approaches (22%, n = 23).
Thematic and content analysis unveiled five key stages of the CI development process. Each stage is characterized by a distinct thematic development approach, as outlined in Figure 3. These stages employed specific design methods, which could be qualitative, quantitative, or mixed. Moreover, various instruments were utilized throughout the development process, as depicted in Figure 4.
Figure 4. Methods, approaches, and instruments used in the initial design stages of concept inventory.
Stage 1: Define the construct, determine, select, and validate the contents domain.
Stage 2: Identify misconceptions and categorize distractors.
Stage 3: Test item construction, response format, and process defined.
Stage 4: Test item selection, testing process, and validation.
Stage 5: Application and refinement of CI.
Our study highlighted the initial stage in CI design, which involves delineating the target construct and selecting and validating contents. Designers employed mixed methods with diverse approaches. A literature review was used in most studies (94%), followed by expert input (83%). Additionally, about 45% of studies combined both expert input and literature review, while 8% adopted a more comprehensive approach by integrating expert input, literature review, and student interviews. Furthermore, 15% conducted student interviews to incorporate their perspectives in concept specification and validation.
This stage encompasses diagnosing and categorizing misconceptions, with researchers using various methods, including student interviews, which were conducted in 75% of studies. Different approaches such as cognitive and/or think-aloud interviews, as well as free-response methods, were utilized with structured open-ended questions (SOEQs), MCQs, and mixed-format questions. Additionally, 64% sought input from experts, while 79% utilized literature resources.
During the third stage, test items are constructed, responses formatted, and response processes defined. Approximately 75% of researchers opted for MCQ formats, while 16% chose a combination of open-ended questions (OEQs) and MCQs, and 5% used OEQs exclusively. Additionally, about 25% of CI items were in a two-tiered format, requiring students to answer MCQs first and then provide explanations along with feedback.
During this stage, the emphasis was placed on selecting and validating test items using a mixed-model approach. Item validity and reliability were established through an integrated process involving expert input, literature review, student responses, and standard statistical tests. Students were engaged through cognitive interviews (25%), think-aloud interviews (19%), and free-response surveys (20%). Figure 5 demonstrates the psychometric properties across various validity and reliability attributes. Additionally, many utilized both Classical Test Theory (CTT) and Item Response Theory (IRT) models, examining item statistics such as item difficulty (81%) and item discrimination (75%). Cronbach alpha (56%) and Kuder–Richardson (KR-20) tested item internal consistency (Figure 5).
Figure 5. Psychometric properties of concept inventory tools and methodological approaches to attain the tests.
During this phase of CI development, the test items and instruments undergo testing in real-world scenarios, with user feedback sought for further validation. This iterative model allows for the incorporation of new ideas and concepts from both developers and users in future iterations. Our scoping review found that 91% of researchers preferred the MCQs as the final test item format, with OEQ formats making up the remainder. Additionally, the majority of CI assessment tools utilized the MCQ format for real-world scenarios, with one-fourth (25%) incorporating items with multiple tiers.
Psychometric properties, including content validity (94%), face validity (15%), communication validity (32%), structural validity (42%), and criterion validity (23%), were determined in various CI instruments. Reliability measures encompassing internal consistency (69%) and reliability tests (26%) were applied. As illustrated in Figure 6, the psychometric tests have not been adequately described and limitations in utilization were noted. Test designers established these psychometric tests using a mixed-method approach that combined qualitative and quantitative methods such as expert reviews, analysis of student responses, gold-standard comparisons, and standard statistical tests (Supplementary Table S3).
This scoping review highlights various psychometric properties employed in the validation of CI tools, noting that certain types of validity are more critical than others (Wren and Barbera, 2013). Additionally, the evidence provided in the reviewed studies supporting these psychometric properties was inadequately described and requires further refinement.
This scoping review aimed to identify key design stages and summarize the methods and approaches used in developing and validating CI tools. Thematic and content analysis revealed five stages in the CI development process, each characterized by unique thematic approaches and specific design methods. This discussion will examine the implications of these findings, highlighting the psychometric properties necessary for effective validation and identifying gaps in design, validity, and reliability to support future development and enhance assessment practices.
The initial stage in CI development involves defining the construct, selecting the concept, and validating it, rooted in theories of construct validity, which emphasize that an assessment should accurately gauge the intended construct or concept (Messick, 1989a,b). Test developers employed various models and evidence-based frameworks (Messick, 1995; Cronbach and Meehl, 1955; American Educational Research Association (AERA), American Psychological Association (APA), and National Council on Measurement in Education (NCME), 1999), to define the dimensions of the test and assess content relevance (Treagust, 1988).
Test designers have used multiple approaches, each to varying extents (Stefanski et al., 2016; Perez et al., 2013; Peşman and Eryılmaz, 2010). Most developers relied on expert input or literature analysis, some integrated both expert input and literature and a smaller fraction also included student interviews (Wright et al., 2009; Abell and Bretz, 2019). Expert input, such as reflections on experiences, discussions, and interviews (Wasendorf et al., 2024; Nedungadi et al., 2021; Scribner and Harris, 2020), played a pivotal role in defining the target construct, aligning content specifications with standard procedures, and subjecting them to rigorous review processes (Caceffo et al., 2016; Moseley and Shannon, 2012; Adams and Wieman, 2011). In some instances, the Delphi process, another method for gathering expert knowledge on concepts, was utilized (Kirya et al., 2021; Nelson et al., 2007), albeit less frequently reported for concept selection (Nabutola et al., 2018; Nelson et al., 2007). These strategies are consistent with approaches demonstrating that key concepts can be identified and validated through an examination of literature, expert experiences, and student interviews (Klymkowsky et al., 2003). Insights from these approaches also aid in constructing CI distractors.
Previous studies (Solomon et al., 2021; Williamson, 2013), learning materials, and curricula (Wright et al., 2009; Bilici et al., 2011) were cited as essential resources by many authors. Combining approaches and conducting an inclusive analysis of various resources (White et al., 2023; Jarrett et al., 2012; Bardar et al., 2007) can effectively construct the underlying content structures necessary for designing CI tools that efficiently measure student performance, validate misconceptions, and mitigate potential biases, rather than relying solely on a single source of evidence (Bretz and Linenberger, 2012). This holistic approach contributes to ensuring the accurate measurement of assessment objectives and outcomes (Brandriet and Bretz, 2014; Abraham et al., 2014).
This stage focuses on identifying student misconceptions within the defined scope and test construct, using cognitive psychology principles to address students’ misunderstandings and thought processes in formulating questions and responses (Anderson and Rogan, 2010). Misconceptions often stem from informal learning experiences and interactions with others (Driver et al., 1994; Driver, 1983). It is also important to recognize that educators, religious beliefs, parental influences, textbooks and media can further contribute to these misconceptions (Yates and Marek, 2014; Abraham et al., 1992). Moreover, ineffective teaching strategies (Gunyou, 2015; Köse, 2008) and daily experiences often perpetuate these flawed understandings (Driver, 1983; Driver et al., 1994). According to the National Research Council (2005), new understanding builds on existing knowledge and experiences. If students’ prior ideas are not identified, new information can be integrated into their existing framework, thereby reinforcing incorrect concepts and complicating future learning (Karpudewan et al., 2017).
To effectively address and correct misconceptions, it is essential to first identify them (Karpudewan et al., 2017). This can be achieved through formative assessments, concept mapping, classroom observations, various questioning techniques, student reflections, discussions, diagnostic tests, and peer interactions. This constructivist-based model, which emphasizes understanding students’ prior knowledge and facilitating conceptual change (Driver et al., 1994; McCaffrey and Buhr, 2008), is particularly effective in addressing misconceptions and enhancing scientific understanding (Cakici and Yavuz, 2010; Awan, 2013). This approach is pivotal for recognizing common misconceptions within a specific domain, aiding in content selection and establishing construct validity (Driver and Oldham, 1986; Brooks and Brooks, 1999).
Combining strategies involving literature reviews, expert feedback, and learners’ responses, misconceptions were constructed and validated to establish test items (Jarrett et al., 2012; McGinness and Savage, 2016). About 80% of studies employed literature to validate student alternative conceptions. Additionally, more than three-fourths of the studies actively involved learners through cognitive and think-aloud interviews, as well as written responses. This approach enabled the use of students’ language to characterize and construct distractors (Hicks et al., 2021; Kirya et al., 2021). About two-thirds incorporated expert input through panel discussions, the Delphi process, and drawing from experiences. Approximately 80% of CI developers used structured OEQs followed by MCQs and mixed-format interviews in diagnosing misconceptions (Corkins et al., 2009; Martin et al., 2003; Scribner and Harris, 2020).
During the third stage of development, items are generated, and formats are determined, with an emphasis on predicting psychometric properties and conducting statistical analysis. This phase also involves specifying test procedures, defining the target population, and selecting appropriate test administration platforms. It is an essential step in producing the initial versions of test items, allowing for the optimization of the CI efficiency. For optimal effectiveness, test items should be succinct and well-crafted (Crocker and Algina, 1986; Taskin et al., 2015).
MCQs are primarily used due to their ease of administration, consistent grading and suitability for large-scale assessments across different instructors or institutions (Haladyna and Rodriguez, 2013; Nedeau-Cayo et al., 2013; Vonderheide et al., 2022; Bardar et al., 2007). Beyond these practical benefits, evidence suggests that MCQs can match or even surpass OEQs in assessing higher-order cognitive skills and providing valid results, particularly in exit-level summative assessments (Hift, 2014). Additionally, the better reliability and cost-effectiveness of MCQs make them a viable alternative to OEQs for summative purposes, enhancing the standardization of CI tools. Current research suggests that well-constructed MCQs can provide evidence comparable to OEQs, enhancing the structure and standardization of CI tools (Sherman et al., 2019). This indicates that MCQs may be more effective than commonly assumed.
About one-quarter of CI tool items were in two-tiered formats, requiring students to answer questions and provide explanations and feedback. This model mandates precise answers in the first tier and asks students to confidently rate their responses in the second tier. The two primary purposes of confidence scales are to mitigate random guessing, aid in assessing the depth of students’ understanding, and help to investigate learning challenges by analyzing incorrect answers. This approach is crucial for identifying misconceptions or learning difficulties (Bitzenbauer et al., 2022; Luxford and Bretz, 2014), resembles the Formative Assessment of Instruction (FASI), and is essential for maintaining a clear test structure, saving time, and ensuring objective assessment (Adams and Wieman, 2011). Conversely, a single-tier test model is essential to preserve a clear test layout, streamline test administration, and ensure a prompt and unbiased evaluation of students’ responses (Wörner et al., 2022).
In the fourth stage of test development, the focus is ensuring the accuracy, relevance, and consistency of inventory items. We identified the validity and reliability parameters specifically for test items. The psychometric properties of CI instruments encompass various components described in a separate section below. Relevance and representativeness were assessed through integrated approaches, including expert panels, student interviews, pilot testing, curriculum analysis, and literature reviews (O’Shea et al., 2016; Haynes et al., 1995; Villafañe et al., 2011). Internal consistency and correlations were evaluated using factor analysis (Messick, 1989b) and reliability tests (Cronbach, 1951). More than half of the approaches to tool development used techniques like Cronbach’s alpha and/or the KR-20 to measure internal consistency (Eshach, 2014; Jarrett et al., 2012).
Reliability ensures the consistency of scores across items measuring the same construct, leading to reproducible outcomes (Villafañe et al., 2011). About 30% of the studies assess item reliability using test–retest, split-half, parallel-forms, and inter-rater methods (Veith et al., 2022; Bristow et al., 2011). Most tests employed the CTT and IRT models to examine item statistics such as item difficulty and discrimination. While not all test designers utilized these models, all elements within an item pool should meet these criteria (Haladyna and Rodriguez, 2013). This analysis aids in identifying items requiring revision, removal, or further consideration (Flynn et al., 2018; Brandriet and Bretz, 2014).
During this phase, CI tools are assessed within real-world settings, and feedback from users utilized to iteratively refine and modify the tools. Test outcomes are methodically assessed, and user feedback is integrated to guide crucial adjustments based on user perspectives. Moreover, while design approaches are rooted in various theories, the dynamic nature of the design model and evolving concept domains may pose challenges to testing relevance over time (Haynes et al., 1995; Haynes and O’brien, 2000). Ongoing modifications and validations through consistent evaluation and testing (McFarland et al., 2017; Jarrett et al., 2012; Savinainen and Scott, 2002) ensure alignment with existing theories.
The majority of CI assessments employed MCQ formats and incorporated multi-tiered items, which enable efficient large-scale evaluations of chosen concepts (Vonderheide et al., 2022; Bardar et al., 2007). These multi-tiered items not only evaluate conceptual understanding but also prompt students to articulate their reasoning process, assisting in identifying misconceptions (Rosenblatt and Heckler, 2017; Luxford and Bretz, 2014). This approach also helps in evaluating learners’ cognitive skills related to specific constructs and aids in exploring methods to address misconceptions while controlling parameters associated with guessing. Despite their benefits, variation exists in the designing process and methodological approaches used in CI development. This scoping review identified key development stages, methods, and approaches, providing insights for future CI tool creation and validation.
This scoping review identified the psychometric properties of CIs required in the design process (Libarkin, 2008; Lopez, 1996). Most CI designers have utilized mixed-method approaches grounded in theories of construct validity, cognitive psychology, and educational research methodology to gather the validity evidence (Villafañe et al., 2011; Wren and Barbera, 2013; Anderson and Rogan, 2010). While validation is crucial in CI development, this review uncovered inadequacies in describing necessary psychometric properties, and types of validity evidence required to establish them. As an example, only 42% of the studies reported structural validity, and 23% addressed criterion validity. Also, 31% employed internal consistency measures and only 26% included reliability testing. The extent to which CIs have been validated varies considerably and is contingent on factors such as design stage, aim, and interpretations and uses of test scores (Wren and Barbera, 2013; Flynn et al., 2018). Despite some tools lacking sufficient validity evidence, certain inventories can still be utilized with minimal validation (Furrow and Hsu, 2019).
For example, content validity plays a crucial role in ensuring item relevance and representativeness within the intended construct (Haynes et al., 1995; Kline, 2013). However, instances were identified in which assessment tools lacked full validation on target populations (Wright et al., 2009; Sherman et al., 2019; Luxford and Bretz, 2014), and instructors may not agree on alignment with learning priorities (Solomon et al., 2021). Furthermore, internal consistency was assessed to ensure that items accurately reflected the test dimensionality (Kline, 2013; Haynes et al., 1995; Mokkink et al., 2010). If the obtained scores do not reflect the expected concept, adjustments to items may be necessary (Villafañe et al., 2011). However, few CI tools addressed these aspects (Paustian et al., 2017; McFarland et al., 2017).
Despite recommendations in the literature (Messick, 1995; Cook and Beckman, 2006) that assessment instruments should employ various psychometric tests to strengthen validity evidence, designers have addressed these tests to varying extents and some tests are considered more critical than others (Wren and Barbera, 2013). Additionally, this review highlighted a significant gap in describing the sources of validity evidence supporting the claimed psychometric tests (Bristow et al., 2011). This underscores the need for more comprehensive refinement and documentation in future research.
Our study found that 80% of the CI instruments primarily measured the students’ conceptual understanding, with 48% identifying misconceptions and 40% assessing both conceptual understanding and misconceptions. The remaining inventories assessed learning gains and evaluated the effectiveness of instructional approaches. More than half (53%) of authors utilized a pre-post-test approach to evaluate learning outcomes. This approach allows educators to compare students’ learning gains over time, which might improve conceptual understanding, as compared to relying on a single assessment score (Price et al., 2014). However, the pre-post method may have limitations, particularly if the educator is aware of the test items and teaches to the questions. Nevertheless, using the CI tool provides a deeper and more nuanced evaluation of student knowledge, enabling educators to design targeted interventions to address misunderstandings. For example, a CI tool discovered a common misconception among high school physics students about Newton’s laws. This finding enabled educators to design focused lessons that improve students’ understanding (Rusilowati et al., 2021). Likewise, educators utilized inventories to diagnose and address common misconceptions, allowing them to adjust the curriculum effectively. The analysis of CI results can guide curriculum changes and enrich students’ academic success (Rennpferd et al., 2023).
We conducted a scoping review to systematically examine CI development processes and methodological approaches. Despite our rigorous approach, caution should be exercised in interpreting our findings. Our goal was to identify key stages, summarize thematic trends, and map methods and approaches used in CI instrument development and evaluation. We refrained from assessing the quality of assessment instruments due to diverse design methods, precluding statistical comparisons. Instead, we described and summarized methods to develop a consensus model for quality CI instrument practice. Our review focused on original articles detailing CI development methods, potentially excluding papers evaluating psychometric tests or teaching interventions. Additionally, our review only encompassed English-language studies, potentially overlooking relevant research in other languages.
This review identified and refined the key design stages involved in the development and validation of CI tools. It also highlighted the patterns and trends while summarizing the methodological approaches that can inform future research. Despite the growing interest in using CIs for educational assessment, the variability in design and validation processes underscores the need for ongoing evaluation. A thorough understanding of the CI development stages and methods, particularly those that utilized mixed-method approaches incorporating expert input, literature reviews and student response analysis, can guide researchers in selecting effective design models.
Test designers employed diverse approaches, integrating construct validity theories to ensure accurate assessments and cognitive psychology principles to understand and address students’ misconceptions and thought processes in formulating questions and responses. Additionally, they applied educational research methodology principles, including iterative development, piloting, and validation through expert review and statistical analysis across different stages. To emphasize the holistic nature of CI design, a descriptive, iterative, and dynamic model was constructed, highlighting five key stages identified through thematic analysis.
Additionally, identifying and characterizing the psychometric properties at both instrument and test item levels is crucial for ensuring the practical applicability of validated CI tools. Validity and reliability requirements are highly linked to specific constructs, development stages, and intended uses of these instruments. Moreover, the importance of certain validity types varies depending on the context, leading to variability in their applications. This review provides a characterization of the psychometric properties used to establish the validity and reliability aspects of CI tools. However, the evidence to establish the claimed psychometric properties is often inadequately described, indicating a need for further refinement.
Future research should establish a unified typology for validity and reliability test requirements and types of validity evidence to establish these requirements. The findings of this review will be complemented with expert opinions, educational guidelines and standards to guide the development of an analytical tool for refining the psychometric properties of CI tools. This effort could further optimize the effectiveness of CI tools, foster a cohesive evaluation approach, and bridge existing gaps.
AKN: Conceptualization, Data curation, Formal analysis, Investigation, Methodology, Project administration, Software, Supervision, Validation, Visualization, Writing – original draft, Writing – review & editing. A-MB: Formal analysis, Methodology, Writing – original draft, Writing – review & editing, Investigation. RK-L: Formal analysis, Investigation, Methodology, Writing – original draft, Writing – review & editing. TA: Formal analysis, Investigation, Methodology, Writing – original draft, Writing – review & editing. PW: Formal analysis, Methodology, Writing – original draft, Writing – review & editing, Conceptualization, Supervision.
The author(s) declare that no financial support was received for the research, authorship, and/or publication of this article.
The authors extend their gratitude to the CI developers and expert group members who generously contributed their expertise to this review. Additionally, we acknowledge the valuable assistance provided by Monash University librarians in preparing and formulating the literature search protocol.
The authors declare that the research was conducted in the absence of any commercial or financial relationships that could be construed as a potential conflict of interest.
All claims expressed in this article are solely those of the authors and do not necessarily represent those of their affiliated organizations, or those of the publisher, the editors and the reviewers. Any product that may be evaluated in this article, or claim that may be made by its manufacturer, is not guaranteed or endorsed by the publisher.
The Supplementary material for this article can be found online at: https://www.frontiersin.org/articles/10.3389/feduc.2024.1442833/full#supplementary-material
CIs, Concept Inventories; CTT, Classical Test Theory; IRT, Item Response Theory; MCQs, Multiple Choice Questions; OEQs, Open-Ended Questions; SOEQs, Structured Open-Ended Questions; STEM, Science, Technology, Engineering, and Mathematics.
Abell, T. N., and Bretz, S. L. (2019). Development of the enthalpy and entropy in dissolution and precipitation inventory. J. Chem. Educ. 96, 1804–1812. doi: 10.1021/acs.jchemed.9b00186
Abraham, M. R., Grzybowski, E. B., Renner, J. W., and Marek, E. A. (1992). Understandings and misunderstandings of eighth graders of five chemistry concepts found in textbooks. J. Res. Sci. Teach. 29, 105–120. doi: 10.1002/tea.3660290203
Abraham, J. K., Perez, K. E., and Price, R. M. (2014). The dominance concept inventory: a tool for assessing undergraduate student alternative conceptions about dominance in Mendelian and population genetics. Cbe—Life Sci. Educ. 13, 349–358. doi: 10.1187/cbe.13-08-0160
Adams, W. K., and Wieman, C. E. (2011). Development and validation of instruments to measure learning of expert-like thinking. Int. J. Sci. Educ. 33, 1289–1312. doi: 10.1080/09500693.2010.512369
American Educational Research Association (AERA), American Psychological Association (APA), and National Council on Measurement in Education (NCME) (1999). Standards for educational and psychological testing. New York: American Educational Research Association.
Anderson, J. R. (2005). Cognitive psychology and its implications. San Fransisco, CA: Worth Publishers.
Anderson, D. L., Fisher, K. M., and Norman, G. J. (2002). Development and evaluation of the conceptual inventory of natural selection. J. Res. Sci. Teach. 39, 952–978. doi: 10.1002/tea.10053
Anderson, T. R., and Rogan, J. M. (2010). Bridging the educational research-teaching practice gap. Biochem. Mol. Biol. Educ. 38, 51–57. doi: 10.1002/bmb.20362
Arksey, H., and O'Malley, L. (2005). Scoping studies: towards a methodological framework. Int. J. Soc. Res. Methodol. 8, 19–32. doi: 10.1080/1364557032000119616
Arthurs, L., and Marchitto, T. (2011). “Qualitative methods applied in the development of an introductory oceanography concept inventory survey” in A. D. Feig & A. Stokes (Eds.), Qualitative inquiry in geoscience education research (Boulder, CO: Geological Society of America Special Paper 474), 97–111.
Awan, A. S. (2013). Comparison between traditional text-book method and constructivist approach in teaching the concept ‘Solution’. J. Res. Reflections Educ. 7, 41–51.
Bailey, J. M., Johnson, B., Prather, E. E., and Slater, T. F. (2012). Development and validation of the star properties concept inventory. Int. J. Sci. Educ. 34, 2257–2286. doi: 10.1080/09500693.2011.589869
Baker, F. B., and Kim, S.-H. (2004). Item response theory: Parameter estimation techniques. Boca Raton: CRC Press.
Bardar, E. M., Prather, E. E., Brecher, K., and Slater, T. F. (2007). Development and validation of the light and spectroscopy concept inventory. Astron. Educ. Rev. 5, 103–113.
Beichner, R. J. (1994). Testing student interpretation of kinematics graphs. Am. J. Phys. 62, 750–762. doi: 10.1119/1.17449
Bilici, S. C., Armagan, F. O., Cakir, N. K., and Yuruk, N. (2011). The development of an astronomy concept inventory (ACI). Procedia Soc. Behav. Sci. 15, 2454–2458. doi: 10.1016/j.sbspro.2011.04.127
Bitzenbauer, P., Veith, J. M., Girnat, B., and Meyn, J.-P. (2022). Assessing engineering students’ conceptual understanding of introductory quantum optics. Physics 4, 1180–1201. doi: 10.3390/physics4040077
Black, P., and Wiliam, D. (1998). Inside the black box: Raising standards through classroom assessment. Granada Learn. 5, 7–74. doi: 10.1080/0969595980050102
Brandriet, A. R., and Bretz, S. L. (2014). The development of the redox concept inventory as a measure of students’ symbolic and particulate redox understandings and confidence. J. Chem. Educ. 91, 1132–1144. doi: 10.1021/ed500051n
Braun, V., and Clarke, V. (2006). Using thematic analysis in psychology. Qual. Res. Psychol. 3, 77–101. doi: 10.1191/1478088706qp063oa
Bretz, S. L., and Linenberger, K. J. (2012). Development of the enzyme-substrate interactions concept inventory. Biochem. Mol. Biol. Educ. 40, 229–233. doi: 10.1002/bmb.20622
Bristow, M., Erkorkmaz, K., Huissoon, J. P., Jeon, S., Owen, W. S., Waslander, S. L., et al. (2011). A control systems concept inventory test design and assessment. IEEE Trans. Educ. 55, 203–212. doi: 10.1109/TE.2011.2160946
Brooks, J. G., and Brooks, M. G. (1999). In search of understanding: The case for constructivist classrooms. Alexandria, Virginia, USA: Association for Supervision and Curriculum Development.
Caceffo, R., Wolfman, S., Booth, K. S., and Azevedo, R. (2016). Developing a computer science concept inventory for introductory programming. Proceedings of the 47th ACM technical symposium on computing science education, 364–369.
Cakici, Y., and Yavuz, G. (2010). The effect of constructivist science teaching on 4th grade students' understanding of matter. Asia-Pac. Forum Sci. Learn. Teach. 11, 1–19.
Caleon, I., and Subramaniam, R. (2010). Development and application of a three-tier diagnostic test to assess secondary students’ understanding of waves. Int. J. Sci. Educ. 32, 939–961. doi: 10.1080/09500690902890130
Cook, D. A., and Beckman, T. J. (2006). Current concepts in validity and reliability for psychometric instruments: theory and application. Am. J. Med. 119:166.e7-16. doi: 10.1016/j.amjmed.2005.10.036
Cooper, M. M., Caballero, M. D., Carmel, J. H., Duffy, E. M., Ebert-May, D., Fata-Hartley, C. L., et al. (2024). Beyond active learning: using 3-dimensional learning to create scientifically authentic, student-centered classrooms. PLoS One 19:e0295887. doi: 10.1371/journal.pone.0295887
Corkins, J., Kelly, J., Baker, D., Kurpius, S. R., Tasooji, A., and Krause, S. (2009). Determining the factor structure of the materials concept inventory. Annual Conference & Exposition, 14.436.1–14.436.19.
Crocker, L., and Algina, J. (1986). Introduction to classical and modern test theory. New York, NY: ERIC.
Cronbach, L. J. (1951). Coefficient alpha and the internal structure of tests. Psychometrika 16, 297–334. doi: 10.1007/BF02310555
Cronbach, L. J., and Meehl, P. E. (1955). Construct validity in psychological tests. Psychol. Bull. 52, 281–302. doi: 10.1037/h0040957
D'Avanzo, C. (2008). Biology concept inventories: overview, status, and next steps. Bioscience 58, 1079–1085. doi: 10.1641/B581111
Driver, R., Asoko, H., Leach, J., Scott, P., and Mortimer, E. (1994). Constructing scientific knowledge in the classroom. Educ. Res. 23, 5–12. doi: 10.3102/0013189X023007005
Driver, R., and Oldham, V. (1986). A constructivist approach to curriculum development in science. Stud. Sci. Educ. 13, 105–122. doi: 10.1080/03057268608559933
Epstein, J. (2013). The calculus concept inventory-measurement of the effect of teaching methodology in mathematics. Not. Am. Math. Soc. 60, 1018–1027. doi: 10.1090/noti1033
Eshach, H. (2014). Development of a student-centered instrument to assess middle school students’ conceptual understanding of sound. Phys. Rev. Spec. Top. Phys. Educ. Res. 10:010102. doi: 10.1103/PhysRevSTPER.10.010102
Flynn, C. D., Davidson, C. I., and Dotger, S. (2018). Development and psychometric testing of the rate and accumulation concept inventory. J. Eng. Educ. 107, 491–520. doi: 10.1002/jee.20226
Freeman, S., Eddy, S. L., Mcdonough, M., Smith, M. K., Okoroafor, N., Jordt, H., et al. (2014). Active learning increases student performance in science, engineering, and mathematics. Proc. Natl. Acad. Sci. 111, 8410–8415. doi: 10.1073/pnas.1319030111
Furrow, R. E., and Hsu, J. L. (2019). Concept inventories as a resource for teaching evolution. Evol.: Educ. Outreach 12, 1–11. doi: 10.1186/s12052-018-0092-8
Greenhalgh, T., Robert, G., Macfarlane, F., Bate, P., Kyriakidou, O., and Peacock, R. (2005). Storylines of research in diffusion of innovation: a meta-narrative approach to systematic review. Soc. Sci. Med. 61, 417–430. doi: 10.1016/j.socscimed.2004.12.001
Gunyou, J. (2015). I flipped my classroom: one teacher’s quest to remain relevant. J. Public Aff. Educ. 21, 13–24. doi: 10.1080/15236803.2015.12001813
Haladyna, T. M., and Downing, S. M. (Eds.) (2006). Handbook of test development. 1st Edn. Mahwah, NJ, US: Routledge.
Haladyna, T. M., and Rodriguez, M. C. (2013). Developing and validating test items. New York: Routledge.
Haslam, F., and Treagust, D. F. (1987). Diagnosing secondary students' misconceptions of photosynthesis and respiration in plants using a two-tier multiple-choice instrument. J. Biol. Educ. 21, 203–211. doi: 10.1080/00219266.1987.9654897
Haynes, S. N., and O’brien, W. H. (2000). “Psychometric foundations of behavioral assessment” in Principles and practice of behavioral assessment, eds. Alan S. Bellack and Michel Hersen (Boston, MA: Springer US), 199–222.
Haynes, S. N., Richard, D., and Kubany, E. S. (1995). Content validity in psychological assessment: a functional approach to concepts and methods. Psychol. Assess. 7, 238–247. doi: 10.1037/1040-3590.7.3.238
Herman, G. L., and Loui, M. C. (2011). Administering a digital logic concept inventory at multiple institutions. ASEE Annual Conference & Exposition, 22.142.1–22.142.12.
Hestenes, D., Wells, M., and Swackhamer, G. (1992). Force concept inventory. Phys. Teach. 30, 141–158. doi: 10.1119/1.2343497
Hicks, M., Divenuto, A., Morris, L., and Demarco, V. (2021). Active drawing of mechanisms of genetics and molecular biology as an undergraduate learning tool. FASEB J. 35. doi: 10.1096/fasebj.2021.35.S1.04715
Hift, R. J. (2014). Should essays and other “open-ended”-type questions retain a place in written summative assessment in clinical medicine? BMC Med. Educ. 14, 1–18. Available at: http://www.biomedcentral.com/1472-6920/14/249
Jarrett, L., Ferry, B., and Takacs, G. (2012). Development and validation of a concept inventory for introductory-level climate change science. Int. J. Innovation Sci. Math. Educ. 20, 25–41. Available at: https://ro.uow.edu.au/eispapers/723.
Julie, H., and Bruno, D. (2020). Approach to develop a concept inventory informing teachers of novice programmers’ mental models. IEEE Frontiers in Education Conference (FIE), 1–9.
Karpudewan, M., Zain, A. N. M., and Chandrasegaran, A. (2017). Overcoming Students' misconceptions in science. Singapore: Springer Nature Singapore Pte Limited.
Kirya, K. R., Mashood, K. K., and Yadav, L. L. (2021). A methodological analysis for the development of a circular-motion concept inventory in a Ugandan context by using the Delphi technique. Int. J. Learn. Teach. Educ. Res. 20, 61–82. doi: 10.26803/ijlter.20.10.4
Klymkowsky, M. W., and Garvin-Doxas, K. (2008). Recognizing student misconceptions through Ed's tools and the biology concept inventory. PLoS Biol. 6:e3. doi: 10.1371/journal.pbio.0060003
Klymkowsky, M. W., Garvin-Doxas, K., and Zeilik, M. (2003). Bioliteracy and teaching efficacy: what biologists can learn from physicists. Cell Biol. Educ. 2, 155–161. doi: 10.1187/cbe.03-03-0014
Knight, J. (2010). Biology concept assessment tools: design and use. Microbiol. Australia 31, 5–8. doi: 10.1071/MA10005
Köse, S. (2008). Diagnosing student misconceptions: using drawings as a research method. World Appl. Sci. J. 3, 283–293.
Luxford, C. J., and Bretz, S. L. (2014). Development of the bonding representations inventory to identify student misconceptions about covalent and ionic bonding representations. J. Chem. Educ. 91, 312–320. doi: 10.1021/ed400700q
Martin, J., Mitchell, J., and Newell, T. (2003). Development of a concept inventory for fluid mechanics. 33rd Annual Frontiers in Education, 2003. FIE 2003., 1, T3D-T3D.
McCaffrey, M. S., and Buhr, S. M. (2008). Clarifying climate confusion: addressing systemic holes, cognitive gaps, and misconceptions through climate literacy. Phys. Geogr. 29, 512–528. doi: 10.2747/0272-3646.29.6.512
Mcfarland, J. L., Price, R. M., Wenderoth, M. P., Martinková, P., Cliff, W., Michael, J., et al. (2017). Development and validation of the homeostasis concept inventory. Cbe—Life Sci. Educ. 16:ar35. doi: 10.1187/cbe.16-10-0305
Mcginness, L. P., and Savage, C. (2016). Developing an action concept inventory. Phys. Rev. Phys. Educ. Res. 12:010133. doi: 10.1103/PhysRevPhysEducRes.12.010133
McGrath, C., Guerin, B., Harte, E., Frearson, M., and Manville, C. (2015). Learning gain in higher education. Santa Monica, CA: Rand Corporation.
Messick, S. (1989a). Meaning and values in test validation: the science and ethics of assessment. Educ. Res. 18, 5–11. doi: 10.3102/0013189X018002005
Messick, S. (1989b). “Validity” in Educational measurement. ed. R. L. Linn . 3rd ed. (New York, NY: Macmillan Publishing Co, Inc.; American Council on Education), 13–103.
Messick, S. (1995). Validity of psychological assessment: validation of inferences from persons' responses and performances as scientific inquiry into score meaning. Am. Psychol. 50, 741–749. doi: 10.1037/0003-066X.50.9.741
Miller, R. L., Streveler, R. A., Yang, D., and Santiago Román, A. I. (2011). Identifying and repairing student misconceptions in thermal and transport science: concept inventories and schema training studies. Chem. Eng. Educ. 45, 203–2010.
Mokkink, L. B., Terwee, C. B., Patrick, D. L., Alonso, J., Stratford, P. W., Knol, D. L., et al. (2010). The Cosmin study reached international consensus on taxonomy, terminology, and definitions of measurement properties for health-related patient-reported outcomes. J. Clin. Epidemiol. 63, 737–745. doi: 10.1016/j.jclinepi.2010.02.006
Moseley, S. S., and Shannon, M. (2012). Work-in-Progress: initial investigation into the effect of homework solution media on fundamental statics comprehension. Association for Engineering Education – Engineering Library Division Papers, 25.1491.1.
Nabutola, K., Steinhauer, H., Nozaki, S., and Sadowski, M. (2018). Engineering graphics concept inventory: instrument development and item selection. 4th international conference on higher education advances (Head'18), 1317–1324.
National Research Council (2005). How students learn: Science in the classroom. Washington, DC: The National Academies Press.
Nedeau-Cayo, R., Laughlin, D., Rus, L., and Hall, J. (2013). Assessment of item-writing flaws in multiple-choice questions. J. Nurses Prof. Dev. 29, 52–57. doi: 10.1097/NND.0b013e318286c2f1
Nedungadi, S., Mosher, M. D., Paek, S. H., Hyslop, R. M., and Brown, C. E. (2021). Development and psychometric analysis of an inventory of fundamental concepts for understanding organic reaction mechanisms. Chem. Teach. Int. 3, 377–390. doi: 10.1515/cti-2021-0009
Nelson, M. A., Geist, M. R., Miller, R. L., Streveler, R. A., and Olds, B. M. (2007). How to create a concept inventory: the thermal and transport concept inventory. Annual Conference of the American Educational Research Association, 9–13.
Ngambeki, I., Nico, P., Dai, J., and Bishop, M. (2018). Concept inventories in cybersecurity education: an example from secure programming. IEEE Frontiers in Education Conference (FIE), 1–5.
O’shea, A., Breen, S., and Jaworski, B. (2016). The development of a function concept inventory. Int. J. Res. Undergrad. Math. Educ. 2, 279–296. doi: 10.1007/s40753-016-0030-5
Page, M. J., Mckenzie, J. E., Bossuyt, P. M., Boutron, I., Hoffmann, T. C., Mulrow, C. D., et al. (2021). The Prisma 2020 statement: an updated guideline for reporting systematic reviews. Int. J. Surg. 88:105906. doi: 10.1016/j.ijsu.2021.105906
Patton, M. Q. (1990). Qualitative evaluation and research methods. Thousand Oaks, CA, US: Sage Publications, Inc.
Paustian, T. D., Briggs, A. G., Brennan, R. E., Boury, N., Buchner, J., Harris, S., et al. (2017). Development, validation, and application of the microbiology concept inventory. J. Microbiol. Biol. Educ. 18, 1–10. doi: 10.1128/jmbe.v18i3.1320
Perez, K. E., Hiatt, A., Davis, G. K., Trujillo, C., French, D. P., Terry, M., et al. (2013). The EvoDevoci: a concept inventory for gauging students’ understanding of evolutionary developmental biology. Cbe—Life Sci. Educ. 12, 665–675. doi: 10.1187/cbe.13-04-0079
Peşman, H., and Eryılmaz, A. (2010). Development of a three-tier test to assess misconceptions about simple electric circuits. J. Educ. Res. 103, 208–222. doi: 10.1080/00220670903383002
Price, R. M., Andrews, T. C., Mcelhinny, T. L., Mead, L. S., Abraham, J. K., Thanukos, A., et al. (2014). The genetic drift inventory: a tool for measuring what advanced undergraduates have mastered about genetic drift. Cbe—Life Sci. Educ. 13, 65–75. doi: 10.1187/cbe.13-08-0159
Rennpferd, M. J., Schroeder, M. V., Nguyen, J. J., Lund-Peterson, M. A., Lancaster, O., and Condry, D. L. J. (2023). Application of the microbiology concept inventory to improve programmatic curriculum. J. Microbiol. Biol. Educ. 24, e00110–e00122. doi: 10.1128/jmbe.00110-22
Rosenblatt, R., and Heckler, A. F. (2017). The development process for a new materials science conceptual evaluation. IEEE Frontiers in Education Conference (FIE), 1–9.
Rowe, G., and Smaill, C. (2007). Development of an electromagnetic course—concept inventory—a work in progress. Proceedings of the eighteenth conference of Australian Association for Engineering. Department of Computer Science and Software Engineering, the University of Melbourne, Melbourne, Australia, 7, 1–7.
Rusilowati, A., Susanti, R., Sulistyaningsing, T., Asih, T., Fiona, E., and Aryani, A. (2021). Identify misconception with multiple choice three tier diagnostik test on newton law material. J. Phys. Conf. Ser. 1918:052058. doi: 10.1088/1742-6596/1918/5/052058
Sadler, P. M. (1998). Psychometric models of student conceptions in science: reconciling qualitative studies and distractor-driven assessment instruments. J. Res. Sci. Teach. 35, 265–296. doi: 10.1002/(SICI)1098-2736(199803)35:3<265:AID-TEA3>3.0.CO;2-P
Sands, D., Parker, M., Hedgeland, H., Jordan, S., and Galloway, R. (2018). Using concept inventories to measure understanding. High. Educ. Pedagog. 3, 173–182. doi: 10.1080/23752696.2018.1433546
Savinainen, A., and Scott, P. (2002). The force concept inventory: a tool for monitoring student learning. Phys. Educ. 37, 45–52. doi: 10.1088/0031-9120/37/1/306
Scribner, E. D., and Harris, S. E. (2020). The mineralogy concept inventory: a statistically validated assessment to measure learning gains in undergraduate mineralogy courses. J. Geosci. Educ. 68, 186–198. doi: 10.1080/10899995.2019.1662929
Shepard, L. A. (2000). The role of assessment in a learning culture. Educ. Res. 29, 4–14. doi: 10.3102/0013189X029007004
Sherman, A. T., Oliva, L., Golaszewski, E., Phatak, D., Scheponik, T., Herman, G. L., et al. (2019). The cats hackathon: creating and refining test items for cybersecurity concept inventories. IEEE Secur. Priv. 17, 77–83. doi: 10.1109/MSEC.2019.2929812
Smith, J. I., and Tanner, K. (2010). The problem of revealing how students think: concept inventories and beyond. Cbe—Life Sci. Educ. 9, 1–5. doi: 10.1187/cbe.09–12–0094
Solomon, E. D., Bugg, J. M., Rowell, S. F., Mcdaniel, M. A., Frey, R. F., and Mattson, P. S. (2021). Development and validation of an introductory psychology knowledge inventory. Scholarsh. Teach. Learn. Psychol. 7, 123–139. doi: 10.1037/stl0000172
Stefanski, K. M., Gardner, G. E., and Seipelt-Thiemann, R. L. (2016). Development of a lac operon concept inventory (loci). Cbe—Life Sci. Educ. 15:24. doi: 10.1187/cbe.15-07-0162
Sukarmin, Z. A., and Sarwanto, D. M. S. (2021). The development of concept inventory assessment integrated with stem literacy to measure students’ creative thinking skills: a need analysis. J. Hunan Univ. Nat. Sci. 48, 405–412.
Summers, M. M., Couch, B. A., Knight, J. K., Brownell, S. E., Crowe, A. J., Semsar, K., et al. (2018). EcoEvo-maps: an ecology and evolution assessment for introductory through advanced undergraduates. Cbe—Life Sci. Educ. 17:ar18. doi: 10.1187/cbe.17-02-0037
Taskin, V., Bernholt, S., and Parchmann, I. (2015). An inventory for measuring student teachers' knowledge of chemical representations: design, validation, and psychometric analysis. Chem. Educ. Res. Pract. 16, 460–477. doi: 10.1039/C4RP00214H
Treagust, D. F. (1988). Development and use of diagnostic tests to evaluate students’ misconceptions in science. Int. J. Sci. Educ. 10, 159–169. doi: 10.1080/0950069880100204
Veith, J. M., Bitzenbauer, P., and Girnat, B. (2022). Assessing learners’ conceptual understanding of introductory group theory using the Ci2gt: development and analysis of a concept inventory. Educ. Sci. 12:376. doi: 10.3390/educsci12060376
Villafañe, S. M., Bailey, C. P., Loertscher, J., Minderhout, V., and Lewis, J. E. (2011). Development and analysis of an instrument to assess student understanding of foundational concepts before biochemistry coursework. Biochem. Mol. Biol. Educ. 39, 102–109. doi: 10.1002/bmb.20464
Vonderheide, A., Sunny, C., and Koenig, K. (2022). Development of a concept inventory for the nursing general, organic and biochemistry course. J. Stem Educ. Innovations Res. 23, 15–22.
Wasendorf, C., Reid, J. W., Seipelt-Thiemann, R., Grimes, Z. T., Couch, B., Peters, N. T., et al. (2024). The development and validation of the mutation criterion referenced assessment (Mucra). J. Biol. Educ. 58, 651–665. doi: 10.1080/00219266.2022.2100451
White, P. J., Guilding, C., Angelo, T., Kelly, J. P., Gorman, L., Tucker, S. J., et al. (2023). Identifying the core concepts of pharmacology education: a global initiative. Br. J. Pharmacol. 180, 1197–1209. doi: 10.1111/bph.16000
Williamson, K. E. (2013). Development and calibration of a concept inventory to measure introductory college astronomy and physics students' understanding of Newtonian gravity, Montana State University.
Wörner, S., Becker, S., Küchemann, S., Scheiter, K., and Kuhn, J. (2022). Development and validation of the ray optics in converging lenses concept inventory. Phys. Rev. Phys. Educ. Res. 18:020131. doi: 10.1103/PhysRevPhysEducRes.18.020131
Wren, D., and Barbera, J. (2013). Gathering evidence for validity during the design, development, and qualitative evaluation of thermochemistry concept inventory items. J. Chem. Educ. 90, 1590–1601. doi: 10.1021/ed400384g
Wright, T., Hamilton, S., Rafter, M., Howitt, S., Anderson, T., and Costa, M. (2009). Assessing student understanding in the molecular life sciences using a concept inventory. FASEB J. 23. doi: 10.1096/fasebj.23.1_supplement.Lb307
Keywords: concept inventory, design stages, methodology, psychometric properties, scoping review
Citation: Netere AK, Babey A-M, Kelly-Laubscher R, Angelo TA and White PJ (2024) Mapping design stages and methodologies for developing STEM concept inventories: a scoping review. Front. Educ. 9:1442833. doi: 10.3389/feduc.2024.1442833
Received: 12 June 2024; Accepted: 17 October 2024;
Published: 06 November 2024.
Edited by:
Janet Clinton, The University of Melbourne, AustraliaReviewed by:
Michael W. Klymkowsky, University of Colorado Boulder, United StatesCopyright © 2024 Netere, Babey, Kelly-Laubscher, Angelo and White. This is an open-access article distributed under the terms of the Creative Commons Attribution License (CC BY). The use, distribution or reproduction in other forums is permitted, provided the original author(s) and the copyright owner(s) are credited and that the original publication in this journal is cited, in accordance with accepted academic practice. No use, distribution or reproduction is permitted which does not comply with these terms.
*Correspondence: Paul J. White, UGF1bC53aGl0ZUBtb25hc2guZWR1
†ORCID: Adeladlew Kassie Netere, http://orcid.org/0000-0003-0519-509X
Anna-Marie Babey, http://orcid.org/0000-0003-3454-5204
Roisin Kelly-Laubscher, http://orcid.org/0000-0002-6379-9943
Paul J. White, http://orcid.org/0000-0002-7524-1808
Disclaimer: All claims expressed in this article are solely those of the authors and do not necessarily represent those of their affiliated organizations, or those of the publisher, the editors and the reviewers. Any product that may be evaluated in this article or claim that may be made by its manufacturer is not guaranteed or endorsed by the publisher.
Research integrity at Frontiers
Learn more about the work of our research integrity team to safeguard the quality of each article we publish.