- 1Keck School of Medicine, Stevens Neuroimaging and Informatics Institute, University of Southern California, Los Angeles, CA, United States
- 2Bravo Medical Magnet High School, Los Angeles United School District, Los Angeles, CA, United States
- 3Division of Engineering Education, Viterbi School of Engineering, University of Southern California, Los Angeles, CA, United States
Introduction: Recent efforts including the U.S. Department of Education’s Raise the Bar: STEM Excellence for All Students, designed to strengthen Science, Technology, Engineering and Mathematics (STEM) education, typify the development of effective outreach programs implemented in high school settings to increase STEM achievement and literacy and to promote future careers in STEM. Specifically, artificial intelligence (AI) and machine learning (ML) are topics of great importance and interest but are often reserved for higher-level education. Introductions of complex subjects in high school promotes student efficacy, enthusiasm, and skill-development for STEM careers. Establishing strong partnerships between universities and high schools is mutually beneficial for the professional development of students, teachers, and professors. In this paper, we detail immersive outreach efforts and their effectiveness in a high school setting.
Methods: From Spring 2021 to Spring 2024, we conducted eight data-science and analysis-coding style workshops along with two data science units, with 302 students participating in the data science workshops and 82 students in the data science units. All students who participated in the data science lessons completed a comprehensive final project. Surveys measuring knowledge and appeal to data science and coding were conducted both retrospectively and prospectively, before and after each workshop and the data science units. A 1 year follow up survey was conducted for students in the 2023 data science lessons (n = 23).
Results: Overall, average student interest significantly increased from 2.72 ± 1.08/5.0 (n = 205) to 3.15 ± 1.18/5.0 (n = 181, p = 0.001) during the data science workshops, while 70% of students expressed desire to continue with coding. Interest modestly increased in the data science lessons from 3.15 ± 0.65/4.0 to 3.17 ± 0.77/4.0 (n = 82, p = 0.8571), while knowledge significantly increased from 64.16% to 88.5% (% correct out of six questions) in the 2023 data science lessons and from 52.62% to 60.79% (% correct out of 29 questions) in the 2024 data science lessons.
Discussion: Increasing STEM exposure through outreach programs and a modified curriculum can positively alter students’ career trajectory and prepare them for the evolving technologically advanced world and the careers within it.
1 Introduction
While artificial intelligence (AI), machine learning (ML), and data science (DS) are becoming ubiquitous in applications globally, there has been a recent push to incorporate AI and ML topics into the curriculum of undergraduate, graduate, and medical students, especially for those who are not specializing in computational subjects (Brouillette, 2019; James et al., 2021; Pucchio et al., 2021; Rajkomar et al., 2019; Shapiro et al., 2018). Introducing DS methods to pre-college students is becoming increasingly relevant, as several professions depend on DS skills and therefore providing such content to high school students provides them with necessary understanding prior to entering college (Srikant and Aggarwal, 2017). National and international content frameworks are evolving to emphasize the importance of DS experiences in K-12 education (Drozda et al., 2022). Yet, unmet needs remain in these learning frameworks, such as a limited emphasis on technology and digital literacy (the ability to “access, manage, understand, integrate, communicate, and create information safely and appropriately; Brata et al., 2022; UNESCO, 2018) data ethics, and relevancy (Drozda et al., 2022). To understand and navigate the contemporary world, it is imperative for students to understand AI, ML, and DS, along with its applications, implications, and limitations in everyday life, beyond the classroom. While K-12 computational programs are becoming increasingly prevalent (Master et al., 2017; Vartiainen et al., 2020), much work is necessary to prepare students for a continuously technologically evolving landscape. These disparities are pronounced on a large scale, as only 45% of public high schools in the U.S. offered computer science (CS) courses in 2019, whereas 57.5% offered a CS course in 2023 (Code.org, 2019; Gifford, 2023). This prevalence is sparser in schools with greater proportions of diverse students. In 2019, it was reported that 42% of schools with a 0–25% minority population offer a CS course compared to only 27% of schools with 75–100% minority population offering a CS course (Code.org, 2019). It is therefore vital to bridge the gap of digital literacy. Thus, the present study aims to address this gap.
An integrated STEM education is essential to prepare students with skills necessary to succeed in the current workforce, requiring improved instruction in K-12 settings (Sen et al., 2018; Herro et al., 2019). Accordingly, a President’s Council of Advisors on Science and Technology (PCAST) report (Executive Office of the President, 2011) recommended recruitment of science and health students and corresponding increased attention to strategic STEM-related instruction and teacher professional development. Since then, several teacher professional development programs have been implemented to bridge the gaps present in contemporary STEM education (Huang et al., 2022). Modern techniques such as immersive collaboration, or the ability to collaborate through digital technologies while not physically together may help boost STEM engagement, which in turn may increase interest and knowledge to better prepare students for STEM careers. The growing national concern over decreases in STEM achievement in high school, which contributes to workforce declines in STEM-related careers, must be addressed to improve educational and career trajectories. With digital literacy comes more and better career opportunities, along with an advanced, nuanced understanding of the contemporary world (National Skills Coalition, 2023). As evidenced by recent national initiatives such as CHIPS for America–a $280 billion act signed in 2022 to advance science and technology–the landscape of the workforce is shifting towards technology-based careers (The White House, 2022).
Despite the growing prevalence of computer science courses in the U.S., issues remain that must be addressed to bridge the gap between levels of digital literacy in high schools and the growing demand for STEM related careers. A primary theory attributed to this gap is the lack of personal and cultural relevance to the students, as well as a lack of sustained engagement with the material (Ladson-Billings, 1995; Maltese et al., 2014). However, despite previous research demonstrating the potential to increase engagement and interest in STEM fields by providing personally and culturally relevant instruction, many programs fail to achieve this (Harackiewicz and Hulleman, 2010; Tai et al., 2006). It is therefore important to address these shortcomings to improve digital literacy and increased interest and motivation for STEM careers.
Another reason posited for poor student achievement in DS and technology related subjects is connected to teachers’ under preparedness (Lewis et al., 1999). One study found high school teachers to have limited content knowledge regarding DS and computational frameworks (Wenglinsky, 2000). Similarly, a review of 17 studies established that providing support for teachers’ professional development in STEM education would help to increase confidence and subsequently performance in the classroom, especially in diverse settings (Margot and Kettler, 2019).
Thus, our collaborative efforts aim to ameliorate the gap between students’ digital literacy and the growing demand for STEM careers by addressing the research question of how university-high school collaborations can increase students’ digital literacy and motivation for a career in STEM.
To address this question, a neuroimaging research center in a large research university in the western U.S. teamed up with a high school in a large urban area in the western U.S to teach data science to 9th and 11th grade students. Research has revealed that collaboration between higher education and high school teachers promotes faculty professional development both for teachers and higher education faculty (Knowlton et al., 2015). Socio-demographically, the partnering school district for this outreach program is the second largest in the U.S., serving approximately 430,000 students, and is composed of a diverse student body (See Supplementary material for 2023–2024 demographic data). As prior empirical data has illustrated that schools with more diverse and higher minority populations tend to be under-resourced and therefore experience less technological-related courses like CS, the targeted partnership between the university and the high school was intended to ameliorate the disparities of STEM education. Previous studies show that immersive workshops had positive impacts on students and teachers, especially when scientists interacted with students inside the classroom (Fitzakerley et al., 2013; Toledo et al., 2020). The hope is that this work can extend findings of positive impacts from previous research as well as introduce methods to address current gaps in STEM achievement in K-12 students, such as the current national learning frameworks described in Drozda et al. (e.g., limited emphasis on technology and digital literacy, data ethics, and relevancy; 2022). Furthermore, the collaboration also aims to translate this work into regional, state, national, and international success, helping improve STEM prevalence in K-12 settings.
The collaborative efforts entailed eight, one-day DS workshops from the spring of 2021–2023 and two, 3-week introduction to DS course units taught in the spring of 2023 and 2024. The DS unit consisted of five unified lessons of: (1)-an introduction to DS, (2)-using code to conduct DS and data analysis (DA), (3)-using code to produce data visualizations, (4)-using code to create regression models and make predictions (ML applications), and (5)-data ethics. By offering students hands-on ways of learning about data science through guided instruction as well as independent and group work, the aim is to provide students with technical data science fundamentals that translate into career-based skills, while acknowledging the importance of ethics. As a culmination of all lessons (the unit of study), students created and presented a team group project to the class in spring 2023, while students created a final individual project in spring 2024, based on relevant, real-world data sets. The final projects served as a means for culminating reinforcement of what was learned over the three-week unit and an avenue to improve public speaking and allow for the interpretation and ability to convey scientific information while working as a team (in 2023) on a real-world challenge. Additionally, the outreach model increased teachers’ knowledge of DS and computational skills using challenge-based pedagogy for workshops and tutorials.
Through this collaboration, we were able to quantify students’ digital literacy and quantitative affect and motivation relative to each workshop and unit, along with a 1-year longitudinal affect and motivation analysis of students who participated in the spring 2023 lessons. In what follows, the outreach conducted in 2021–2024 attempts to answer the questions of how university-high school collaborations can increase digital literacy and motivation for a career in STEM.
2 Pedagogical frameworks, pedagogical principles, competencies/standards
2.1 Competencies/standards
The unit of study lessons were guided by NGSS (Next Generation Science Standards, 2022). HS-ETS1-1, 1-2 Engineering and California Career Technical Education [CTE] (2021) Model Curriculum Standards for the Information and Communication Technologies Sector. The following primary standards from CTE were incorporated into the DS lessons:
• Statistics and Probability-Interpreting Categorical and Quantitative Data (S-ID)
• Statistics and Probability-Advanced Placement Probability and Statistics (APPS)
• Engineering, Technology, and the Applications of Science (ETS)
• 4.0 Technology
• 5.0 Problem Solving and Critical Thinking
The following secondary standards from CTE were implemented into the DS lessons:
• Functions-Building Functions (F-BF)
• Number and quantity-Quantities (N-Q)
• Statistics and Probability-Making Inferences and Justify Conclusions (S-IC)
• 8.0 Ethics and Legal Responsibilities
• 9.0 Leadership and Teamwork
• A2.0 Understand the ethical, moral, legal, and cultural issues related to the use of biotechnology research and product development
• A5.0 Integrate computer skills into program components
The standards aim to address the shifting landscape of education and technology (NSF, 2021), preparing students for the influx of thousands of technology-based careers (The White House, 2022).
2.2 Pedagogical frameworks, pedagogical principles
2.2.1 Workshops
The aim of the workshops was to introduce students to advanced techniques to analyze public health data, equipping them with skills needed to break through in the AI/ML/neuroinformatics fields. Each workshop lasted 50 minutes and consisted of four sections: (1)-understanding the basics and foundation of data and the Python coding language, (2)-learning how to explore and analyze data, (3)-learning how to visualize data and (4)-critical thinking: tying DA and visualization together to form interpretations. The workshops were centered around an integrative pedagogical approach. The integrative approach describes how students integrate knowledge from multiple disciplines to work towards approaching new problems which require critical thinking (Edith Cowan University, 2021). As COVID-19 was near its peak relevance during the time of instruction of workshops, students were required to reflect and answer critical public health questions relevant to COVID-19 using coding and math skills. Through guided workshops that integrated math, programming, and public health, not only did students develop their technical skills, but they also applied these skills to a relevant real-world phenomenon such as COVID-19, which has been directly affecting their lives and their community. Through the workshop’s interactive nature of posing vital, complex questions about COVID-19, students were guided through how to develop evidence-based conclusions using technological tools using mathematics and programming. The guided workshop promoted critical thinking and curiosity, leading students to gain a deeper holistic and quantitative understanding about current events, such as COVID-19.
2.2.2 Units of study
The aim of the unit of study was similar to the workshops, with an aim of introducing students to advanced techniques to collect, analyze, interpret, and communicate information related to real-world problems. The unit of study lasted three weeks and allowed for a more critical and nuanced understanding of the following five lesson topics: (1)-introduction to DS and DA and their applications, (2)-using code to conduct DS and DA, (3)-using code to visualize data, (4)-using code to make regression models and conduct regression analysis, and (5)-understanding data ethics and implications of using data. Throughout the lessons, the constructivist approach (von Glasersfeld, 1995), collaborative approach (Hmelo-Silver et al., 2013), integrative approach (Edith Cowan University, 2021), and inquiry-based approach (Khalaf and Zin, 2018) were all applied, while students collaborated to solve real-world problems, preparing them for careers in technology (Ed.gov, 2024). The unit of study included a pre-evaluative assessment of knowledge, followed by guided instruction of the five lesson topics, each with ample time for guided and independent exploration of applications of lesson concepts in various contexts. The in-class time also consisted of independent work, group discussions, question and answer time, critical thinking opportunities, reflection, and collaboration under these pedagogical frameworks. Students also were instructed to coalesce all the skills gained in the lessons to deliver a culminating presentation about analyzing data to draw conclusions about a real-world problem. In 2023, students created and presented the final project to the class as a team, whereas time constraints in spring 2024 led to the completion of an individual project for each student. Through the final projects, students were able to investigate student identified, open-ended problems (constructivist and inquiry-based approach) and collaborate (collaborative approach) with their peers (in 2023) to apply a myriad of skills to solve these interdisciplinary problems (integrative approach). These final projects aimed at engaging students and increasing their motivation through relevant content that were of interest to students, such as analyzing housing data, heart-disease data, and nutrition data. Furthermore, through pre- and post-assessments, learning and affect/motivation of students were gauged, which allowed the instructors to reflect on the effectiveness, strengths, and weaknesses of instruction. The students in the 2023 lesson cohort completed a 1 year follow up survey in 2024. Overall, by introducing students to the learning objectives prior to each lesson, providing an environment for applying learned-material, and assessing students with pre- and post-surveys as well as final projects, constructive alignment was established.
3 Learning environment, learning objectives, pedagogical format
3.1 Learning environment
A neuroimaging research center in a large research university in the western U.S. collaborated with a high school in a large urban area in the western U.S., to teach students about DS and DA. The public school district is the second largest in the U.S., serving more than 430,000 students (see Supplementary material for demographic data). To see more information about the outreach program, see Duncan Lab, 2024; Duncan et al., 2022.
The DS workshops were conducted in a grade nine health class in spring 2021–2023. There were eight total workshops, with two recorded sessions.
The DS unit of study lessons were conducted in a grade 11 biotechnology elective class for three weeks each, in Spring 2023/2024.
3.2 Workshops
3.2.1 Workshop participants
The workshops were taught to 9th grade students (age 14–15) enrolled in a health class. Although no demographic data were available for the workshops, all students at the high school take the 9th grade health class, so this classroom demographic is representative of the school population.
In the six live, remote workshops, 115 students participated. Ninety-six of these students completed both pre- and post-surveys. In the two recorded workshops, 187 students participated, and 85 students completed both pre- and post-surveys. Some student responses were unaccounted for due to insurmountable technical difficulties.
3.2.2 Workshop instructors
The workshops were instructed by a university researcher over Zoom, either live or through recorded sessions. The workshops were presented in the presence of the high school teacher of the class.
3.3 Unit of study
3.3.1 Unit of study participants
The units of study were taught in a biotechnology elective course for grade 11 students (age 16–17) in 2023 and 2024, taking place during regular school hours. Forty-three students in a biotechnology elective course participated in the DS lessons in 2023 and 46 students participated in 2024, all taking place in a live high school classroom setting. Thirty-three students completed the pre-survey, and 36 students completed the post-survey in 2023 whereas 46 students completed the pre-survey and 46 students completed the post survey in 2024. The unit of study participants were representative of the school population with respect to racial/ethnic background. See Supplementary material for a breakdown of the demographic data. Twenty-three students who participated in the 2023 lessons completed a follow-up survey 1 year later in 2024.
3.3.2 Unit of study instructors
Two university researchers and two graduate students in DS led the creation of the DS unit, which consisted of five lessons. In 2023, one university researcher and a graduate student delivered live, in-class instruction to the class while one university researcher observed the classes over Zoom. In 2024, two graduate students delivered live, in class instruction. The university’s researchers and supervisors also attended numerous meetings to help guide the creation of the unit of study’s lesson plans, ensuring their compliance to curriculum standards.
3.4 Teacher involvement
Three high school faculty members also participated in the development and implementation of DS workshops and lessons, strengthening their professional development and content knowledge. Weekly meetings between the university and the high school faculty were held to ensure the workshops and lessons were adequately aligned with science and math curricular standards and were suitable for instruction in classrooms. During these meetings, the researcher-teacher relationship was strengthened as both parties gained valuable insight into each other’s fields; teachers gained content-knowledge of DS and technological prowess, while the university researchers obtained pedagogical expertise and received coaching, mentoring, and modeling of how to deliver classroom lessons. Ultimately, these meetings helped both parties become more confident in their roles, while serving as an environment to collaboratively discuss future innovations in high school classrooms.
Prior to live classroom instruction from the university researchers, the high school teachers were involved in reviewing and approving the workshops and the unit of study (and its lessons). The participating teachers also suggested appropriate modifications to ensure material aligned with math and science standards and was suitable for students. During live instruction provided by the university researchers, the teachers oversaw the workshops and lessons, helped facilitate and guide discussions, and asked the class questions to facilitate ongoing student engagement. Additionally, the teachers made copies of worksheets, distributed online materials, and helped plan the workshop and lesson schedules in alignment with the constraints of the school’s schedule.
3.5 Learning objectives
Table 1 provides a listing of the learning objectives for the workshops and unit of study lessons. The learning objectives directly address the gap between students’ digital literacy and readiness for a career in STEM. The lessons address each aspect of digital literacy (accessing, managing, understanding, integrating, communicating, and creating information safely and appropriately), providing students with a solid foundation for a career in STEM. Furthermore, by integrating the constructivist, collaborative, integrative, and inquiry-based approach into the workshops and lessons, students become better equipped to approach problems with an adaptive mindset, whether independently or with teams.
3.6 Pedagogical format
3.6.1 Workshop pedagogical format
The workshops consisted primarily of a direct instruction, follow along style of integrative-based learning. That is, the instructor prepared incomplete templates of code in Google Colab (Google Colaboratory, 2024) regarding topics of data collection, cleaning, analysis, and visualizations for the students to fill in as the instructor was explaining these concepts. As these workshops were focused on exploring a COVID-19 dataset, the students were periodically asked to pause in between concepts and reflect on what each step in the code meant and its real-world implications. By building foundational skills in programming and statistics, students were able to integrate this learning to apply it to topics such as public health. At the end of each workshop, the participating students were asked to critically reflect on what they had just learned and to think of ways to use newly learned skills to solve novel questions. Through these workshops, the students developed skills that are applicable to virtually any field involving data. Surveys (with quantitative and open-ended feedback) were also administered (retrospectively and prospectively) to gauge students’ appeal of the material learned.
3.6.2 Unit of study pedagogical format
Through a combined constructivist, collaborative, integrative, and inquiry based pedagogical approach, students participated in five lessons during a three-week span which culminated into a final project. Prior to the commencement of the unit of study, the students completed pre-assessments to gauge their baseline understanding of the unit’s content. Each lesson was taught by a university researcher and a graduate student guest instructor, while another university researcher observed through Zoom. The high school teacher of the class also was present in the classroom. At the beginning of every class period, the participating students were posed with relevant, content-related questions to think about during the lessons. The instructors provided instruction, while the students were given the opportunity to ask questions and discuss topics in groups posed by the instructors. After guided instruction, the students were tasked with completing an in-class assignment with their peers to respond to inquiries that required skills learned in the guided practice. Each lesson ended with a recapitulation of key points, along with follow-up questions that the students were encouraged to contemplate before the next day’s lesson. In the 2023 unit of study, upon completion of the five core concepts taught in the DS lessons, students were placed into groups of 4–6 and were tasked with researching a relevant real-world problem by researching its background, analyzing a provided data set with code and statistics, making visualizations of these findings, interpreting these findings, and presenting their work to their peers in class. Furthermore, the students were asked to reflect on the ethical, social, and economic implications their findings had on their everyday life and how their findings help them perceive the world in a quantitative way. The students presented their work in an approximately 10-minute presentation to the class, developing their public speaking skills. By providing the students with an opportunity to apply their skills in a culturally and personally relevant context, the goal was to advance their skills while increasing their motivation for STEM applications. Finally, along with providing open-ended feedback and completing affect and motivation based surveys, the students completed a post-assessment to assess their understanding of the material. Twenty-three students from the 2023 workshops completed a 1 year follow up survey in 2024. In 2024, the students completed a similar, individual final project but did not present it to the class because of time constraints. Ultimately, the pedagogical principles that underlaid the development of the unit’s lessons helped students gain relevant mathematical, coding, inquiry, presentation, scientific writing, and communication skills in the technological, critical thinking, collaborative, and public speaking realms.
4 Results
4.1 2021–2023 workshop data
Analytically, paired sample t-tests were used to determine significant changes in interest and desire to continue DA/DS related activities from students before and after the data-science workshops. In the two live sessions on May 12, 2022, the post-evaluative survey was missing two responses (before: n = 13, after n = 11), so the average response value of interest was imputed for two missing responses (Jamshidian and Mata, 2007). Similarly, in the March 7, 2023 recorded session, the post-evaluative survey was missing five responses (before: n = 90, after n = 85), so the average response value of interest was imputed for five missing responses. Overall change in interest was measured using an unpaired t-test (pre-interest n = 205, post-interest n = 181). The tables that follow (Tables 2, 3) represent the full analytical results of the workshops.

Table 2. Responses from 2021 Data Science Workshops – quantitative affect and motivation-based results.
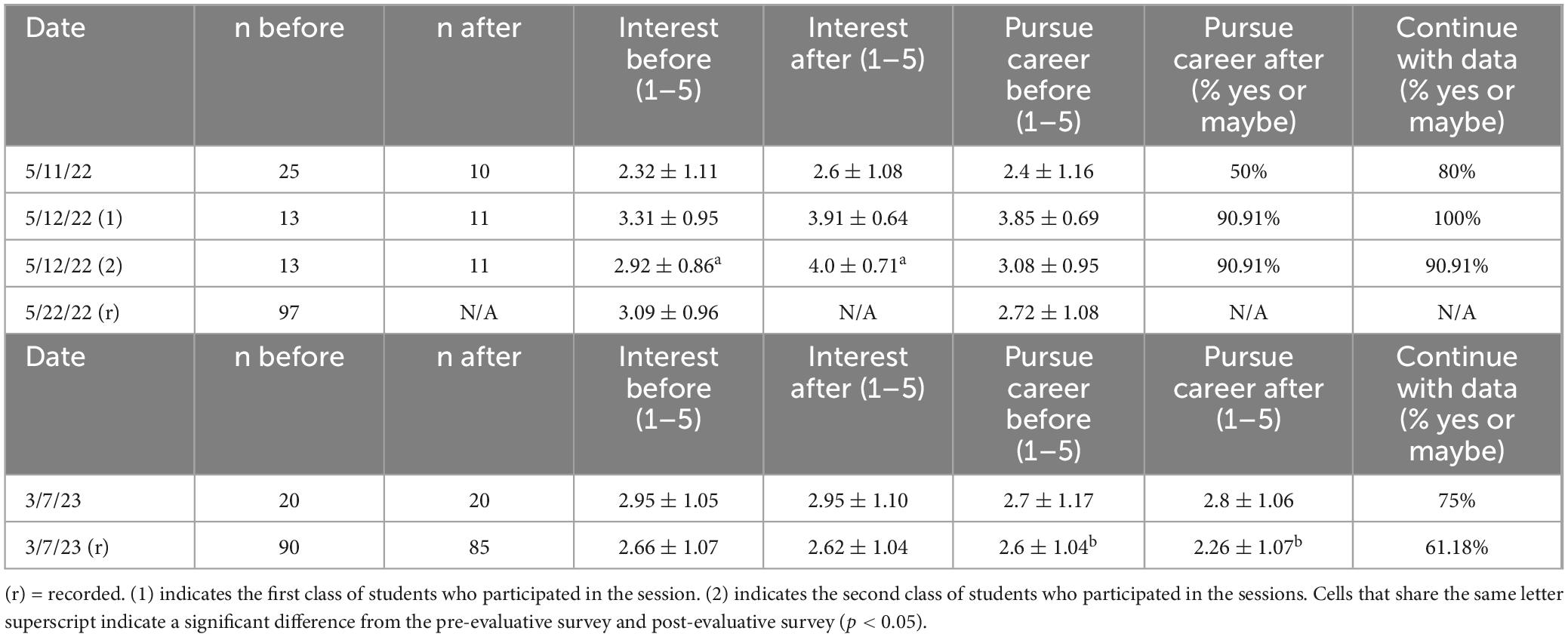
Table 3. Responses from 2022 to 2023 Data Science Workshops – quantitative affect and motivation based results.
Interest significantly increased from 2.72 ± 1.08/5.0 (n = 205) to 3.15 ± 1.18/5.0 (n = 181, p = 0.001) during the DS workshops, while 70% of students wanted to continue with coding.
4.2 2023–2024 units of study data
Paired sample t-tests were conducted to calculate pre- and post-survey differences resulting from completing the units of study (one unit delivered twice over two academic years). The average response was imputed for three data points in the 2023 pre-survey as there were 36 responses in the post-survey and only 33 in the pre-survey. Paired sample t-tests were also conducted to calculate overall change scores for interest, with the imputation of 3 responses (average of all responses) as three students who took the 2023 post-survey did not take the 2023 pre-survey. When stratified by gender, unpaired t-tests were conducted to calculate 2023 changes (due to differences between the number of males who took the pre and post survey), whereas paired t-tests were conducted to calculate 2024 changes. Table 4 represents pre-post comparisons. The table values represent score ± standard deviation out of 4.0.
While Table 4 shows that none of the results were statistically significant when compared from 2023 to 2024, it instead remained relatively the same with an increasing general trend. This indicates the lessons’ lasting impact on the students 1 year after completing the lessons. There was also a high desire to continue endeavors in DS, as evidenced by the unmatched 2024 follow up questions.
Table 5 illustrates pre-post comparisons of content knowledge.
The pre- and post-content knowledge measures from 2023 to 2024 indicate significant increases in knowledge gained from before to after the DS lessons. In 2023, only six questions were asked of moderate difficulty, whereas in 2024, 29 questions were asked: 10 of low difficulty, 10 of medium difficulty, and 10 of significant difficulty (1 question omitted due to a typo). Interest marginally increased in the DS lessons from 3.15 ± 0.65/4.0 to 3.17 ± 0.77/4.0 (n = 82, p = 0.8571), while knowledge significantly increased from 64.16% to 88.5% (six questions) in the 2023 DS lessons and from 52.62% to 60.79% (29 questions) in the 2024 DS lessons.
Table 6 provides the results of the open-ended information from the assessment.
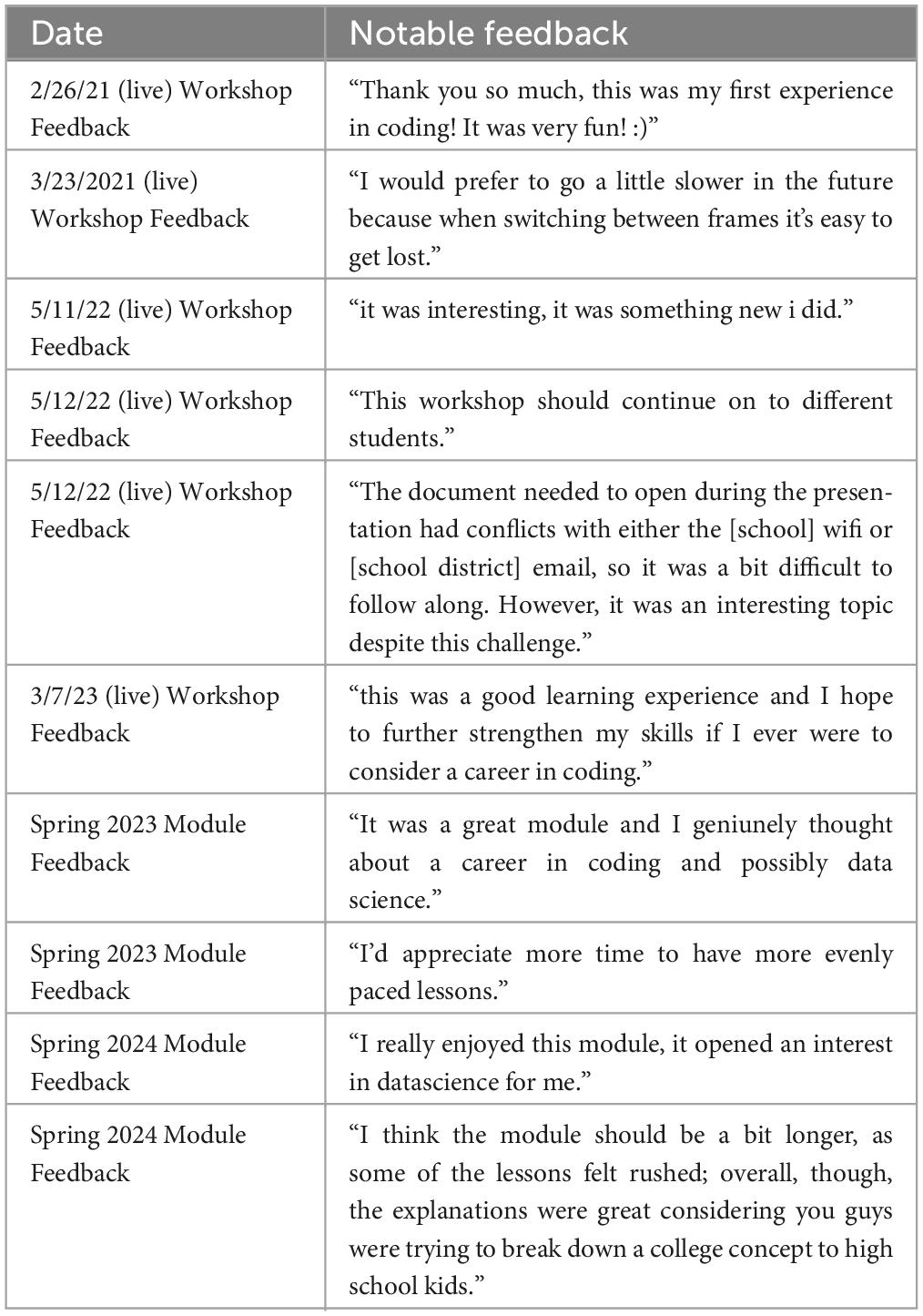
Table 6. Sample of open-ended feedback results from the 2021–2023 workshops and 2023, 2024 units of study.
The results from Table 6 show positive and negative constructive feedback from students who completed the workshops and lessons.
5 Discussion
Engagement with DS, AI, and ML at the high school level is currently limited across the United States, often leaving students under-prepared for careers in contemporary DS and other CS professions. With direct implementation of DS workshops and tutorials, the authors aimed to make DS knowledge more accessible through bringing ground-breaking technologies and innovative methods to urban high school students who may not have otherwise had such educational opportunities. The lessons were intended to help students break through a DS related field, such as ML/AI. The knowledge and skills gained in the workshops/units of study are precursors and essential prerequisites for engaging formally in ML/AI related fields. By collaborating with high school teachers and providing relevant and engaging instruction, increases in digital literacy and interest in STEM were observed.
Generally, students demonstrated a greater interest in DS from before to after completing the workshops. Five out of seven workshop sessions with post-evaluative data showed a general increase in interest, with live sessions showing stronger results. Additionally, in all sessions, most students either wanted to or maybe wanted to continue coding. With respect to 2023, interest stayed about the same and a decrease in interest to pursue a career was observed. This outlier may be because of a few reasons. One, this session was the only recorded workshop where post-evaluative survey results were collected. Students may feel disengaged with an asynchronous model rather than a live model, in which the students desire to pursue a career marginally increased (Table 3). Additionally, in the recording of this workshop, there were technical difficulties for about the first ten minutes, which may have contributed to students’ loss of interest. Finally, this is the final workshop that was conducted and thus the furthest from COVID-19’s relevance. The workshop was centered around COVID-19, which was more relevant in 2021 and 2022, which may suggest that students become more interested when they learn something that is relatable or relevant to them. Practically, taken as a whole, these results may suggest that students’ interest in classroom material may be higher when engaging with temporally and personally relevant material (Ragusa and Leung, 2023; Harackiewicz and Hulleman, 2010; Tai et al., 2006) while also suggesting live learning may enhance motivation compared to asynchronous learning. Since the course content remained virtually the same throughout all of the workshops, these explanations may be the most likely (with the possibility of technical difficulties affecting students’ motivation and interest in the recorded workshops). Nonetheless, the qualitative results from the March 7, 2023, workshop sessions were markedly more positive than negative and students’ learning greatly increased, which suggests positive effects from this workshop.
For the units of study, success was reflected through the pre-and post-assessments, the quality of students’ final group projects, as well as their feedback. Although interest remained relatively constant throughout the lessons as well as the 1-year follow up (yet marginally increased), the students’ experience in these fields profoundly increased (Table 4). In both the 2023 and 2024 lessons, knowledge of DS/DA significantly increased among both females and males. With regard to interest, the units of study were structured so that students were able to engage more with the instructors and peers and have more time to reflect and apply what they learned in class into projects. Engagement in STEM material is vital for long-term interest in STEM careers (Maltese et al., 2014), especially through real-world, hands-on projects (Krajcik and Czerniak, 2014). Furthermore, as described in Johnson et al., 2007, collaborative learning, especially in diverse settings, boasts success and sustained engagement in learning environments. Contrasted with the 2023 workshop results, this upward turn-around in interest is likely due to the aforementioned factors. Similarly, the significant increase in content knowledge in both the 2023 and 2024 units of study can be attributed to such factors, as performance generally increases with factors that increase motivation (Maranan, 2018). However, there was a gap between percentage scores in 2023/2024, which may be due to the increased difficulty and number of questions in the 2024 unit of study. Open-ended feedback also suggested some students felt the lessons helped them better understand the field of DS/computer programming, with some students expressing the desire to pursue a related career (Table 6). Generally, the motivation and affect based results observed in the lessons were more consistently positive compared to the workshops. Although mostly marginal, every question related to interest, familiarity, desire to pursue a career in a data-related field (with the exception of the question related to desire to pursue a career in 2023) demonstrated an increased level of interest and familiarity among the students. These consistent results may be explained by the workshops’ more structured approach, which involved greater levels of interaction, more team-building exercises, and in-person format.
Overall, despite limited access to educational programs for DS in high schools, there remains strong interest and motivation, and increased digital literacy, as evidenced by several workshops and lessons conducted throughout 2021–2024. The success described in the present study may be attributed to two main factors. First, the implementation of collaboration between high-school teachers and university researchers, which fosters a mutually beneficial relationship in which teachers are better prepared to teach STEM content and university researchers gain pedagogical skills. Second, the focus on implementing engaging and personally relevant material into the workshops and units of study has shown to increase learning and motivation. In terms of content knowledge, the pre- and post-content assessment results in 2023 and 2024 highlight that collaboration between higher-learning institutions and high schools provide a pathway of increased digital literacy, preparing students for careers and lives in STEM. By integrating the constructivist, collaborative, integrative, and inquiry-based approaches into the workshops and lessons, along with content expertise from university researchers, high-school students can develop increased digital literacy and motivation for STEM careers. By bridging the gap between a lack of exposure to data-related topics in the high-school setting and the demand for such careers, students can be better prepared in their pursuit of higher education as well as a career in STEM.
6 Limitations
Along with some missing data during the May 11/May 12 workshops, during the last workshop in 2022, post-assessments were not collected due to technological difficulties, the COVID-19 pandemic access limits, and students not completing the survey, which limited data analysis capabilities. These missing data may affect interpretability, as not all data are perfectly matched among sessions. However, every reasonable attempt to ameliorate missing data was taken. Future workshops and unit of study adaptations should ensure pre- and post-assessments are collected for all participant, which may provide more accurate and reliable data. These assessments could also include additional content-related questions to help measure the growth of each student’s DS knowledge with specificity. Results from the workshops also may have been influenced by students’ participation medium, i.e., whether the students participated via online (live/recorded) or in-person. Finally, the decision to convert the team project to an individual project in 2024 was required due to time-constraints associated with the participating high school’s schedule and may have changed students’ perceptions and experiences relative to 2023.
Data availability statement
The raw data supporting the conclusions of this article will be made available by the authors, without undue reservation.
Ethics statement
The studies involving humans were approved by the University of Southern California (USC) IRB. The studies were conducted in accordance with the local legislation and institutional requirements. Written informed consent for participation was not required from the participants or the participants’ legal guardians/next of kin because instructors taught students curriculum-related material during regular class hours in the presence of a teacher.
Author contributions
AAB: Conceptualization, Data curation, Formal analysis, Investigation, Methodology, Project administration, Writing – Original Draft, Writing – Review and Editing. AB: Writing – Original Draft, Writing – Review and Editing. MB-C: Investigation, Writing – Original Draft, Writing – Review and Editing. MS: Supervision, Writing – Original Draft Preparation, Writing – Review and Editing. GR-D: Supervision, Writing – Original Draft, Writing – Review and Editing. GR: Supervision, Conceptualization, Funding acquisition, Writing – Original Draft, Writing – Review and Editing. DD: Funding acquisition, Supervision, Writing – Original Draft, Writing – Review and Editing.
Funding
The author(s) declare financial support was received for the research, authorship, and/or publication of this article. This study was conducted with the support of the National Science Foundation (NSF) under Award Number 2027456 (COVID-ARC) and the Engineer’s Foundation.
Acknowledgments
Thank you to the teachers, instructors, and supervisors at the high school for making these workshops and lessons possible.
Conflict of interest
The authors declare that the research was conducted in the absence of any commercial or financial relationships that could be construed as a potential conflict of interest.
Publisher’s note
All claims expressed in this article are solely those of the authors and do not necessarily represent those of their affiliated organizations, or those of the publisher, the editors and the reviewers. Any product that may be evaluated in this article, or claim that may be made by its manufacturer, is not guaranteed or endorsed by the publisher.
Supplementary material
The Supplementary Material for this article can be found online at: https://www.frontiersin.org/articles/10.3389/feduc.2024.1429893/full#supplementary-material
References
Brata, W., Padang, R. Y., Suriani, C., Prasetya, E., and Pratiwi, N. (2022). Student’s digital literacy based on students’ interest in digital technology, internet costs, gender, and learning outcomes. Int. J. Emerg. Technol. Learn. 17, 138–151.
Brouillette, M. (2019). Ai added to the curriculum for doctors-to-be. Nat. Med. 25, 1808–1809. doi: 10.1038/s41591-019-0648-3
California Career Technical Education [CTE] (2021). California career technical education model curriculum standards. Sacramento, CA: California Career Technical Education.
Drozda, Z., Johnstone, D., and Van Horne, B. (2022). Previewing the National Landscape of K-12 Data Science Implementation: Workshop on foundations of data science for students in grades K-12. Washington, DC: National Center for Education Research.
Duncan Lab (2024). Informatics and computing in neuroscience (ICON) lab. Outreach. Los Angeles, CA: University of Southern California.
Duncan, D., Garner, R., Bennett, A., Sinclair, M., Ramirez-De La Cruz, G., and Pasik-Duncan, B. (2022). “Inter-disciplinary K-12 control education in biomedical and public health applications,” in Proceedings of the 13th symposium on advances in control education, (Berlin).
Ed.gov (2024). Work-based learning. Available online at: https://cte.ed.gov/unlocking-career-success/our-keys/work-based-learning (accessed May 8, 2024).
Edith Cowan University (2021). Integrative learning. ECU Intranet. Joondalup, WA: Edith Cowan University.
Executive Office of the President (2011). President’s council on advancement of science and technology (pcast). Report to congress on the state of STEM education. Washington, DC: White House, 25–37.
Fitzakerley, J. L., Michlin, M. L., Paton, J., and Dubinsky, J. M. (2013). Neuroscientists’ classroom visits positively impact student attitudes. PLoS One 8:e84035. doi: 10.1371/journal.pone.0084035
Google Colaboratory (2024). Google colaboratory. Available online at: https://colab.research.google.com/ (accessed August 20, 2024).
Harackiewicz, J. M., and Hulleman, C. S. (2010). The importance of interest: The role of achievement goals and task values in promoting the development of interest. Soc. Pers. Psychol. Compass 4, 42–52. doi: 10.3389/fpsyg.2024.1383118
Herro, D., Quigley, C., and Cian, H. (2019). The challenges of STEAM instruction: Lessons from the field. Act. Teach. Educ. 41, 172–190.
Hmelo-Silver, C., Chinn, C., Chan, C., and O’Donnell, A. (eds) (2013). The international handbook of collaborative learning, 1st Edn. Milton Park: Routledge, doi: 10.4324/9780203837290
Huang, B., Jong, M. S. Y., Tu, Y. F., Hwang, G. J., Chai, C. S., and Jiang, M. Y. C. (2022). Trends and exemplary practices of STEM teacher professional development programs in K-12 contexts: A systematic review of empirical studies. Comput. Educ. 189:104577.
James, C. A., Wheelock, K. M., and Woolliscroft, J. O. (2021). Machine learning: The next paradigm shift in medical education. Acad. Med. 96, 954–957. doi: 10.1097/ACM.0000000000003943
Jamshidian, M., and Mata, M. (2007). Advances in analysis of mean and covariance structure when data are incomplete. Handb. Comput. Stat. Appl. 2007, 21–44. doi: 10.1016/B978-044452044-9/50005-7
Johnson, D. W., Johnson, R. T., and Smith, K. A. (2007). The state of cooperative learning in postsecondary and professional settings. Educ. Psychol. Rev. 19, 15–29.
Khalaf, B. K., and Zin, Z. B. M. (2018). Traditional and inquiry-based learning pedagogy: A systematic critical review. Int. J. Instruct. 11, 545–564. doi: 10.12973/iji.2018.11434a
Knowlton, S., Fogleman, J., Reichsman, F., and de Oliveira, G. (2015). Higher education faculty collaboration with k-12 teachers as a professional development experience for faculty. J. Coll. Sci. Teach. 44, 46–53. doi: 10.1128/jmbe.v17i2.1074
Krajcik, J. S., and Czerniak, C. M. (2014). Teaching science in elementary and middle school: A project-based approach. Milton Park: Routledge.
Ladson-Billings, G. (1995). Toward a theory of culturally relevant pedagogy. Am. Educ. Res. J. 32, 465–491.
Lewis, L., Parsad, B., Carey, N., Bartfai, N., Farris, E., and Smerdon, B. (1999). Teacher quality: A report on the preparation and qualifications of public school teachers. Statistical analysis report. Educ. Stat. Q. 1, 7–14.
Maltese, A. V., Melki, C. S., and Wiebke, H. L. (2014). The nature of experiences responsible for the generation and maintenance of interest in STEM. Sci. Educ. 98, 937–962. doi: 10.1002/sce.21132
Maranan, S. (2018). Intrinsic motivation, academic engagement and academic achievement of senior high school students in a government funded university in Luzon SY 2017-2018. Quezon City: Archîum ATENEO.
Margot, K. C., and Kettler, T. (2019). Teachers’ perception of STEM integration and education: A systematic literature review. Int. J. STEM Educ. 6, 1–16.
Master, A., Cheryan, S., Moscatelli, A., and Meltzoff, A. N. (2017). Programming experience promotes higher stem motivation among first-grade girls. J. Exp. Child Psychol. 160, 92–106. doi: 10.1016/j.jecp.2017.03.013
National Skills Coalition (2023). Closing the digital skill divide. Washington, DC: National Skills Coalition.
Next Generation Science Standards (2022). HS-ETS1 engineering design. Washington, DC: Next Generation Science Standards.
Pucchio, A., Eisenhauer, E. A., and Moraes, F. Y. (2021). Medical students need artificial intelligence and machine learning training. Nat. Biotechnol. 39, 388–389.
Ragusa, G., and Leung, G. (2023). The impact of early robotics education on students’ understanding of coding, robotics design and interest in computing careers. Sensors 23:9335. doi: 10.3390/s23239335
Rajkomar, A., Dean, J., and Kohane, I. (2019). Machine learning in medicine. New Engl. J. Med. 380, 1347–1358.
Sen, C., Ay, Z. S., and Kiray, S. A. (2018). STEM skills in the 21st century education. Res. Highlights STEM Educ. 58, 81–101.
Shapiro, R. B., Fiebrink, R., and Norvig, P. (2018). How machine learning impacts the undergraduate computing curriculum. Commun. ACM 61, 27–29.
Srikant, S., and Aggarwal, V. (2017). “Introducing data science to school kids,” in Proceedings of the 2017 ACM SIGCSE technical symposium on computer science education, (New York, NY), 561–566.
Tai, R. H., Liu, C. Q., Maltese, A. V., and Fan, X. (2006). Planning early for careers in science. Science 312, 1143–1144.
The White House (2022). FACT SHEET: CHIPS and science act will lower costs, create jobs, strengthen supply chains, and counter China. Washington, DC: The White House.
Toledo, M. A., Koochak, N., Gupta, A., López, L. N., Nieri, T., and Currás-Collazo, M. C. (2020). Interactive student-centered neuroscience workshops for sixth graders enhance science knowledge and education attitudes. J. Undergrad. Neurosci. Educ. 18:A75.
UNESCO (2018). A global framework of reference on digital literacy skills for indicator. Montreal, QC: UNESCO Institute for Statistics.
Vartiainen, H., Toivonen, T., Jormanainen, I., Kahila, J., Tedre, M., and Valtonen, T. (2020). “Machine learning for middle-schoolers: Children as designers of machine-learning apps,” in Proceedings of the 2020 IEEE Frontiers in education conference (FiE), (Uppsala), 1–9.
von Glasersfeld, E. (1995). “A constructivist approach to teaching,” in Constructivism in education, eds L. P. Steffe and J. Gale (Hillsdale, MI: Erlbaum), 3–15.
Keywords: outreach, control education, machine learning, curriculum development, digital literacy
Citation: Bruckhaus AA, Bennett A, Brawer-Cohen M, Sinclair M, Ramirez-De La Cruz G, Ragusa G and Duncan D (2024) Evaluation of students’ digital literacy through an immersive university-high school collaboration. Front. Educ. 9:1429893. doi: 10.3389/feduc.2024.1429893
Received: 08 May 2024; Accepted: 30 September 2024;
Published: 09 October 2024.
Edited by:
Xiang Hu, Renmin University of China, ChinaReviewed by:
Chia Pin Kao, Southern Taiwan University of Science and Technology, TaiwanXiaoyan Lai, Guangdong Polytechnic Normal University, China
Copyright © 2024 Bruckhaus, Bennett, Brawer-Cohen, Sinclair, Ramirez-De La Cruz, Ragusa and Duncan. This is an open-access article distributed under the terms of the Creative Commons Attribution License (CC BY). The use, distribution or reproduction in other forums is permitted, provided the original author(s) and the copyright owner(s) are credited and that the original publication in this journal is cited, in accordance with accepted academic practice. No use, distribution or reproduction is permitted which does not comply with these terms.
*Correspondence: Alexander A. Bruckhaus, YnJ1Y2toYXVAdXNjLmVkdQ==