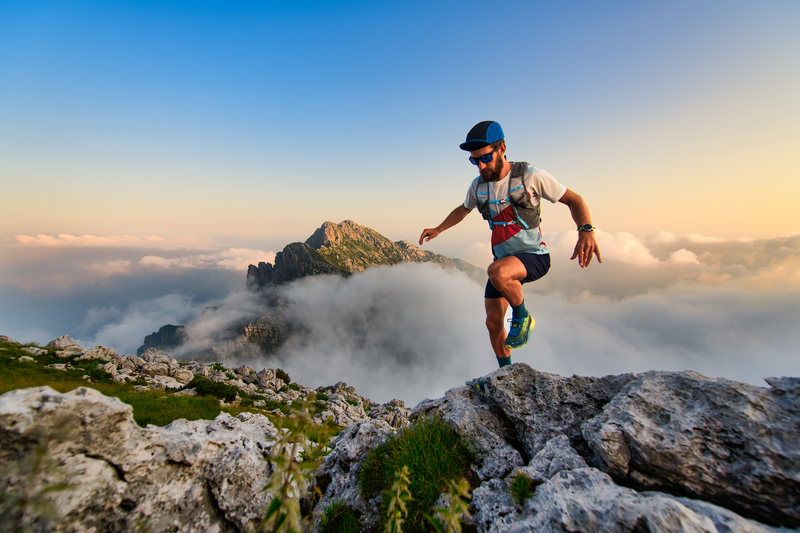
95% of researchers rate our articles as excellent or good
Learn more about the work of our research integrity team to safeguard the quality of each article we publish.
Find out more
ORIGINAL RESEARCH article
Front. Educ. , 01 November 2024
Sec. Higher Education
Volume 9 - 2024 | https://doi.org/10.3389/feduc.2024.1426183
This article is part of the Research Topic Educational Transformation: 21st century skills and challenges for higher education View all 22 articles
This study analyzed the relationship between academic performance and the development of transversal competencies of reasoning for complexity and digital transformation in higher education students. The Tec21 educational model of the Tecnológico de Monterrey was used as a framework for analysis. Data from 33,319 students were analyzed following a CRISP-DM methodology, first an exploratory analysis was performed on the data to recognize and understand the presence of competencies in the educational model. Machine Learning methodologies, including Random Forest and XGBoost, were then employed to identify the most influential curricular and co-curricular variables in the acquisition of studied competencies. The results revealed a significant correlation between academic performance and the acquisition of the competencies, highlighting that high grades are consistently associated with success in developing these competencies. This study extends the understanding of how competency-focused educational interventions can facilitate professional and personal development in a labor market that demands advanced skills and adaptability. The discussion focuses on the implications of these findings for formulating educational policies and pedagogical practices that prioritize both academic achievement and the comprehensive development of essential 21st-century competencies. From a broader perspective, our work is framed in the achievement of Sustainable Development Goal 4 Quality Education and is oriented to identify variables that boost the acquisition of 21st Century Skills in higher education contexts.
The effect of the pandemic in the organizational context has impacted the young population, both in terms of job stability and recruitment processes, making it difficult to choose competent candidates. It has been observed a 23% increase in post-pandemic unemployment rates among 18 to 24-year-olds, as well as a reduction in the earnings of those who remained employed, generating a discouraging perception among young people regarding their professional expectations, so that 40% face the future with uncertainty and up to 14% do so with fear (UN, 2021). On the other hand, three-quarters of American companies have expressed difficulties in recruiting the right people for their vacancies, identifying critical thinking, problem solving, innovation and creativity in the number one missing soft skills (37%); followed by the ability to deal with complexity and ambiguity (32%), and communication skills (31%) (WEF, 2019). This reality highlights the pressing need for organizations to adapt their recruitment strategies and foster an environment that not only attracts but also nurtures the requisite soft skills among the younger workforces.
Consequently, in the field of higher education, leaders of international organizations have proposed guidelines that promote the construction of competencies known as 21st century skills, through the collaborative work of different nations. Such skills are framed within the Sustainable Development Goals (SDGs) of the 2030 Agenda and of these, SDG 4 (UN, 2022a) stands out, which aims to ensure quality, inclusive and equitable education, promoting learning opportunities that last throughout life for all people (Oficina Regional de Educación para América Latina y el Caribe, 2017; UN, 2022b). The skills of the 21st century arise from the emerging issues of the century in question. The World Economic Forum (2016a) states that to face the challenges imposed by the present, students need to acquire abilities that transcend traditional learning systems, so that it is possible to develop skills linked to technology and social and emotional learning that will be useful in the labor scenario. In this context, with the arrival of the so-called fourth industrial revolution or Industry 4.0, conceptualized from the implementation of smart technology, big data, artificial intelligence (AI), nano and biotechnology, the internet of things (IoT), among others, workers of the present and future need to develop new skills to keep pace with what organizations demand from them (Industrial Global Union, 2016; World Economic Forum, 2016b; Xu et al., 2022). Acknowledging the rapid onset of Industry 4.0, it becomes imperative for educational institutions to restructure curricula to encompass not only technology-oriented skills but also the social and emotional competencies that are crucial for students to navigate the multifaceted challenges of the current labor landscape and beyond.
For the development of 21st century skills in employees, it is necessary to first have an impact on the educational systems where the concept of Education 4.0 has emerged (González-Pérez and Ramírez-Montoya, 2022; Kennedy and Sundberg, 2020; Patiño et al., 2023). Ramírez-Montoya et al. (2022) state that education 4.0 derives from the adaptation of new technology based on teaching-learning systems to the changes that have arisen in higher education environments and to the challenges and complexity imposed by the present; where the integration of competencies such as complex thinking, and digital transformation becomes necessary in higher education institutions as a support for all their instructive processes (Miranda et al., 2021). As strategies for the development of transversal competencies and the promotion of a critical perspective of reality are implemented, it will be possible to minimize the negative effects that COVID-19 pandemic has generated in young people and contribute to a better training of students for their optimal performance, both in the educational and organizational contexts (Walsh et al., 2021; Rof et al., 2022).
The 21st century skills are predictive of the challenges that may be imposed by the digital transformation of various social scenarios. Therefore, they prepare higher education spaces for the future needs of a constantly changing population and provide future professionals with an awareness of the reality that transcends the physical teaching-learning environments (Fadel, 2008). Despite the efforts of higher education institutions to foster this type of competencies, there is widespread ambiguity regarding the teaching criteria for assessing performance and what is expected to be achieved in everyone, as well as uncertainty (UN, 2021) as to the degree to which these skills impact on the training and academic performance of students. Thus, it becomes essential for higher education institutions to establish clear, actionable criteria for teaching and assessing 21st century skills, ensuring that students are not only equipped with these competencies but also understand their tangible impact on academic and professional success in a digitally transformed society.
There are some actions that respond to these needs that can be implemented from higher education settings. Among such actions we can find fostering skills, supporting teacher specialization, and eliminating connectivity barriers (UN, 2021). Specifically, in this work we will focus on exploring the effect of some of the most relevant competencies recognized by UNESCO with the objective of knowing to what extent curricular variables enhance the competencies of reasoning for complexity, and digital transformation, together with their sub-competencies. Likewise, there’s a need to know how the development of those skills itself may impact the academic performance of the students and, in turn, would impact in the near future over their performance in organizational contexts.
Complex thinking (also called reasoning for complexity) refers to the acquisition of multidimensional knowledge. This competency is composed of four sub competencies: critical thinking, systemic thinking, scientific thinking, and innovative thinking that allow higher education students to reach problem solving in diverse scenarios (Ramírez-Montoya et al., 2022). In general terms, critical thinking refers to the application, analysis, synthesis, and evaluation of information acquired or generated through processes of observation, experience, reflection, reasoning, or communication as guides for action. Systemic thinking is the reasoning necessary for university students to analyze and understand complex global phenomena. Innovative thinking refers to the creative capacity with a tendency to succeed, and scientific thinking is a higher order capacity that allows students to face the problems of today’s world, through logical, analytical, systemic, inductive, and deductive problem solving (Suárez-Brito et al., 2022a,b). Therefore, the promotion of complex thinking within higher education is crucial, as it arms students with a multifaceted toolkit of sub competencies for analyzing and navigating the complex landscape of contemporary global challenges.
On the other hand, digital transformation refers to the creation of new paradigms in the changing relationships between individuals, industry, and society, supported by the Internet of things and digital technology. It is a global change of socio-economic relations within a cyber-physical world (Mikheev et al., 2021; Garcez et al., 2022; George-Reyes et al., 2023; Vázquez-Parra et al., 2023). For the student community and faculty, digital transformation in higher education improves scientific activity, teaching-learning processes and their experience, as well as the flow of information due to the presence of distance courses and open access to educational resources (Bygstad et al., 2022; Mikheev et al., 2021). Digital transformation, therefore, catalyzes new interaction paradigms and socio-economic shifts in higher education, enhancing scientific activities, learning processes, and information accessibility through digital technologies among the most prominent are the Internet of Things (IoT), Cloud Computing, Virtual Reality, and 3D holograms.
The Tec21 Educational Model, implemented by Tecnologico de Monterrey, is a transformative initiative designed to align education with the demands of the 21st century. It is characterized by four main pillars: Challenge-based Learning, flexibility in learning modalities, inspirational faculty, and an engaging university experience (Swain-Oropeza and Renteria-Salcedo, 2019; Olivares Olivares et al., 2021; Villanueva, 2018). This model contrasts with traditional educational approaches by emphasizing real-world problem-solving, interdisciplinary collaboration, and the integration of emerging technologies, as seen in its application to engineering education to meet Industry 4.0 needs (López et al., 2021). The model has been well-received by students, with surveys indicating a positive perception compared to traditional models (Medina et al., 2021). It also satisfies the requirements of a changing world by offering greater real-world connection and flexibility (Pérez and Campos, 2021). Likewise, during the COVID-19 pandemic, the model demonstrated resilience, with Student Evaluation of Teaching (SET) scores remaining stable or even improving, suggesting that the model’s characteristics may have contributed to a successful transition to online learning (Campos et al., 2022). Thus, interdisciplinary learning as a main characteristic of this educational model, encourages the synthesis of ideas and techniques from various fields, leading to greater creativity and innovation; being especially important in industries that thrive on new ideas and cutting-edge solutions.
Tec21 model encompasses seven transversal competencies that aim to enhance the personal and professional development of students. These competencies are: (1) Ethical and Citizen Commitment, focusing on implementing projects that transform the environment and promote the common good with an ethical conscience and social responsibility; (2) Reasoning for Complexity, which integrates various types of reasoning in problem analysis, synthesis, and resolution, fostering continuous learning; (3) Communication, involving the effective use of different languages, resources, and communicative strategies according to the context; (4) Digital Transformation, encouraging the strategic use of digital tools to innovate and improve processes and products; (5) Self-Knowledge and Management, which involves building a personal and professional well-being project through lifelong reflection and resource integration; (6) Innovative Entrepreneurship, generating innovative, versatile solutions in changing environments that create value and positively impact society; (7) Social Intelligence, developing effective collaboration and negotiation environments in multicultural contexts, respecting and appreciating the diversity of people, knowledge, and cultures (Modelo Tec, n.d.; Villanueva, 2018). These competencies are designed to prepare students not only for professional success but also for personal growth and responsible citizenship.
Specifically, the competencies of Reasoning for Complexity and Digital Transformation within the Tec21 model distinguish themselves by focusing on critical and forward-thinking skills necessary for modern challenges. Reasoning for Complexity is characterized by its emphasis on integrating various forms of reasoning to tackle complex problems, promoting an interdisciplinary approach and continuous learning, essential for adapting to ever-evolving professional landscapes (Baena-Rojas et al., 2022, 2023; Ramírez-Montoya et al., 2022). This competency stands out by encouraging students to synthesize information from diverse fields, enabling them to develop comprehensive solutions that consider multiple dimensions of a problem. On the other hand, Digital Transformation is notable for its focus on the strategic adoption of digital technologies to innovate and enhance productivity and creativity in various domains. It emphasizes not only the use of digital tools but also the transformation of processes and methodologies to stay relevant in the digital age (Aditya et al., 2021; Teixeira et al., 2021; Ostmeier and Strobel, 2022). This competency is particularly distinct in its direct response to the digital economy’s demands, preparing students to be proactive in leveraging technology for sustainable and impactful advancements. In sum, the Tec21 Educational Model exemplifies Tecnológico de Monterrey’s commitment to a dynamic, responsive, and comprehensive approach to higher education, where students are equipped with critical, technical, and social skills that empower them to lead and innovate in a globally connected and digitally driven world.
The objective of the present study was to establish the relationships between the performance of transversal competencies of complex thinking and digital transformation and the variable of academic performance, measured as the average of the student’s grades for the academic program in the academic period in which these competencies are promoted. The following research questions guide this study:
1. How are transversal competencies in complex thinking and digital transformation integrated and contribute to educational outcomes in a competency-based educational model in higher education?
2. What impact do students’ academic achievements have on the development and performance of complex thinking and digital transformation skills in the context of higher education outcomes?
This work pretends to explore the potential of data science, following CRoss-Industry Standard Process for Data Mining (CRISP-DM) methodology (Abbasi et al., 2016), in order to analyze student performance by using the result of students’ assessment based on the defined attributes or learning outcomes, on the records described in previous section.
CRISP-DM is still the de facto standard for developing data mining and knowledge discovery projects. In particular, when data science projects become more exploratory the paths that the project can take become more varied, and a more flexible model is called for. Here, in the purpose of this project, we pretend to categorize, depending on the available data and the corresponding match within objectives we defined, the experiments can deal with goal-directed and exploratory cases.
This methodology facilitates business and data understanding, data preparation, modeling, evaluation and deployment, ensuring that each phase contributes significantly to aligning with business objectives and extracting value from data. The iterative nature of CRISP-DM is ideal for big data projects, where accuracy in handling and analyzing complex data is crucial (Abbasi et al., 2016).
In this work, we employed specific criteria to select and evaluate the data used. The primary indicators for academic performance included the final grades and GPA of students, while competencies were assessed through predefined metrics in the Tec21 educational model. These indicators were chosen based on their relevance and proven reliability in previous educational research (IFE Data Hub, 2023).
Ethical considerations were predominant throughout this research. We adhered to strict data privacy protocols to ensure the confidentiality of student information. Data were anonymized and stored securely, following ethical guidelines established by the Institute for the Future of Education (IFE)‘s Educational Innovation collection of Tecnologico de Monterrey. We ensure that our research practices upheld the highest standards of integrity and respect for participant rights.
The results section is organized according to the stages of the CRISP-DM methodology. For each stage, the methods applied to the information analysis and the consequent obtaining of results are detailed.
Within the framework of the proposal of the Tec21 Model, the aim is to complement educational resources with competencies through the subjects taught to promote comprehensive development of the student that facilitates their insertion into the market field. This approach covers technical requirements and various soft skills that allow the student to adapt to any situation that may arise in the present or near future, in an environment where technology and problem-solving acquire increasing relevance. Therefore, in Tec21, some courses are complemented with two relevant competencies that meet these objectives: Digital Transformation and Complex Thinking.
The analyzed dataset (IFE Data Hub, 2023) comes from the record of the first six academic periods of operation of the Tec21 Model from August 2021 to June 2023. The dataset records general information (e.g., age, gender, school) of students and their relationships with courses, competencies, and sub-competencies, generating repeated rows. Each student has a variable number of rows depending on the number of courses, the number of academic periods enrolled, and the number of competencies associated with each course. The “unique()” function algorithm for data frames from Pandas was used to find and return the unique student elements in the dataset, obtaining 33,319 higher education students, of which 56.46% are men and 43.54% are women. They have an average age of 18.7. They come from 52 different countries, the majority (93.2%) being Mexican, followed by students from the United States (2.98%), and the rest distributed mostly through Latin American countries in order: Ecuador, Honduras, Guatemala, Venezuela, among others. Students are enrolled in five different schools according to the discipline: Architecture and Design (EAAD), Social Sciences and Government (ECSG), Humanities & Education (EHE), Engineering & Sciences (EIC), and Business (EN).
The data understanding phase initially focuses on the detailed analysis of each of the columns of the data set to discern its potential relevance to the study. This process involved a thorough evaluation to identify the possible values of each column and thus determine its usefulness. Data dictionary was consulted to obtain a general description of the information contained in each column or variable. It was ensured that all columns in the data set were present as documented, with no missing items, and that the data matched their descriptions. In those cases where the descriptions did not match the data found, an effort was undertaken to understand the nature of the data available.
Through this exhaustive analysis, 45 columns were identified within the data set, of which 26 are categorical and 19 are numerical. Of the numeric columns, 14 have float values, and 5 have integer values, covering a total of 583,073 rows. A detailed analysis was carried out for each categorical column, identifying the possible values they could assume. We observed columns with a limited number of possible values, such as student gender, which only includes Male and Female, and other columns, such as dates, which present a wide range of possible values. The Python programming language (Python Software Foundation, 2024) and the Dask library (Dask Development Team, 2024) were used to analyze the numerical variables. A descriptive statistics analysis was obtained that included the count of non-null values, the mean, the standard deviation, the minimum, the maximum, and the quartiles. The use of the Dask library was preferred over the Pandas library (McKinney, 2010) due to the large volume of data. Dask improves memory efficiency by processing data in chunks unlike pandas, which require loading the entire data set into RAM memory. This allowed us to run the analysis using a computer with fewer hardware resources.
The data preparation stage constitutes a crucial step in the analysis process, where the data set is subjected to a series of transformations to optimize it for subsequent modeling and evaluation. Initially, the data set was divided into two different subsets, each corresponding to one of the focal competencies of the study: ‘Digital Transformation’ and ‘Reasoning for Complexity’, using the column that describes the competencies as a separation criterion. Within each subset, special attention was paid to the variable that shows the level assigned to the student in said competence, which indicates with the terms ‘Observed’ and ‘Not observed’ whether the corresponding competence was acquired or not, respectively. To facilitate subsequent analysis, these terms were coded numerically as 1 and 0.
Data preparation included a detailed assessment of the null values in each data set. During this analysis, we identified several columns with a high proportion of null values, the presence of which not only lacked relevance to our research objectives but also compromised the integrity of the remaining data set. Removing all rows containing null values would have resulted in a significant loss of valuable data in other columns. Since filling in these null values was not feasible due to their volume, and their relationship to our objective was nonexistent, we opted to discard such columns to preserve the quality of the other relevant variables. Subsequently, those columns with some unique identifiers such as ‘student.id’, columns with detailed descriptions such as ‘subject.longName’, and columns that represented uniqueness in all their values such as ‘group.isLIFE’ and ‘group.isAcademicSupport’ were removed. Finally, the acquired level column was segregated from the rest to prepare it for use in prediction.
In the modeling and evaluation stage, we created a data model corresponding to each of the research questions. Concerning research question 1, we identified the number of courses that promote the competency of complex thinking or digital transformation. We then grouped these courses according to the discipline where they are taught. Figure 1 shows the distribution of the number of courses where Complex Thinking or Digital Transformation competencies are involved according to academic schools’ different disciplines of knowledge. The results of this analysis revealed a greater incorporation of both competencies in the School of Engineering and Sciences (EIC). The rest of the schools present a similar number of courses where the two competencies of the present study are involved. This distribution reflects the emphasis and relevance that the Tec21 Model assigns to the competencies of Complex Thinking and Digital Transformation in the field of engineering, thus underlining its importance in the comprehensive training of students in this area.
Figure 1. Number of courses containing complex thinking or digital transformation competencies among academic schools.
Modeling and evaluation concerning research question 2 were performed through the application of machine learning models. Machine Learning methodologies were capable of providing insights into the relative importance of the different variables in the dataset. Special emphasis was placed on the identification of the most relevant characteristics (feature importance) that influence the obtaining by students of Complex Thinking and Digital Transformation competencies. Based on the data model, Random Forest RF (Schonlau and Zou, 2020), and XGBoost (Chen and Guestrin, 2016) techniques were applied to determine the variables that most influence the attainment of complex thinking and digital transformation competencies. Additionally, Logistic Regression (Bailly et al., 2022), and Support Vector Machine (SVM) (Cervantes et al., 2020) models were included to validate the predictive capacity of the analysis, comparing the relevance of the characteristics identified by the RF and XGBoost models. Data processing included the selection of potentially significant variables, such as student demographic information, course details, and previous grades, as well as the treatment of categorical variables and the normalization of numerical variables. The data set was divided into a ratio of 80% for training and 20% for testing, to evaluate the robustness of the models.
Results indicated that the student final_grade variable emerged as the most influential characteristic in obtaining proficiency, standing out considerably over the other variables in the Random Forest and XGBoost models. According to the data dictionary, final_grade corresponds to the student’s final grade for the subject. In these models, the final_grade variable received approximately twice the importance of any other characteristic, with other variables such as semester GPA showing less relevance. This finding was consistent in both models, which reinforces their validity. However, it is important to highlight that a significant imbalance was observed in the dataset, with more than 90% of the records indicating obtaining Complex Thinking or Digital Transformation competencies. This imbalance could induce a bias in the models, predisposing them to predict obtaining such competencies more frequently due to the underrepresentation of negative cases. Despite this limitation, the models showed high performance in the precision, recall and F1 score metrics, for both the positive and negative classes, with a precision value ranging from 0.78 to 0.97, suggesting a capacity robust predictive within the context of the present imbalance.
To obtain a more detailed understanding of the impact of the final grade on the acquisition of specific competencies, Figure 2, shows box plots describing the difference between obtaining and not obtaining Complex Thinking and Digital Transformation competencies, using the final grade as a comparison variable. In the analysis of these visualizations, it was observed that students who managed to acquire the competencies consistently presented higher final grades. In the boxplots corresponding to the acquisition of competencies, the median and quartiles were substantially above the threshold of 70, thus highlighting the positive correlation between high final grades and the achievement of competencies. On the other hand, students who did not acquire the competence showed a distribution of scores that, although more varied, focused on a lower median, particularly in the Complex Thinking competence, where outliers below a numerical grade of 35 were observed.
Figure 2. Boxplots distribution for final grade and acquisition of complex thinking and digital transformation competencies.
Boxplots of Figure 2, revealed not only reinforce the premise that the final grade is a significant predictor for obtaining competencies but also show a general trend of lower grades associated with not obtaining competencies. Despite the presence of outliers and the wide distribution of qualifications for not obtaining Digital Transformation, the relationship between high qualifications and the acquisition of competencies remains robust. This finding underscores the importance of the final grade as a critical variable in the educational model and suggests that, despite the full range of grades, there is a distinctive division in the attainment of competencies based on academic performance.
An analysis was performed to estimate the probability of acquiring the competencies of Complex Thinking and Digital Transformation based on the score obtained in the final_grade variable. Table 1 shows the score ranges of the final_grade variable for the probability of obtaining each of the two competencies under study. Table 1 shows a segmentation of the range of scores into four categories: from 0 to 69, from 70 to 79, from 80 to 89, and from 90 to 100. The findings revealed an incremental pattern: for students with scores in the range of 0 to 69, the probabilities of acquisition were 46.63% for Complex Thinking and 44.80% for Digital Transformation. This percentage almost doubled for the next range of scores, with 83.20% for Complex Thinking and 89.67% for Digital Transformation in the range of 70 to 79. The upward trend remained consistent for the higher ranges, culminating in those with scores of 90 to 100 had, on average, a 98.64% probability of acquiring Complex Thinking and a 98.88% for Digital Transformation. These data suggest that there is a relationship between the final grade (final_grade variable) and the probability of obtaining a competency, indicating that higher academic performance may be associated with the achievement of competencies.
Table 1. Association between student’s final grade ranges and the percentage of competency acquisition.
To substantiate our findings, we performed a comprehensive statistical analysis using Random Forest and XGBoost models. The final grades emerged as the most influential factor in acquiring competencies, as depicted in Table 1. This correlation aligns with our hypothesis that academic performance is a significant predictor of competency acquisition.
The box plots in Figure 2 further illustrate the distribution of final grades among students who acquired competencies versus those who did not. As shown, students with higher final grades were more likely to develop competencies, supporting our discussion on the impact of academic achievement on competency development.
These data points are critical in understanding the broader implications of our findings. They demonstrate a clear link between academic performance and the development of essential competencies, validating the criteria established for this study.
A competency-based educational model has an independent relationship between grades received and competencies acquired. Table 1 shows that the higher the grade, the higher the probability of acquiring the Complex Thinking and Digital Transformation competencies. This relationship also implies that not all of the students who obtain one of the two competencies that are being studied will pass the course. Likewise, not all students who perform well acquire these competencies.
The modeling and evaluation related to the research questions were carried out through the application of automatic learning models, providing an adequate analysis. As shown in Figure 1, the distribution of courses containing the competencies of analysis was established and the presence of them was differentiated among Academic Schools that make up the Institution; unlike previous studies (Swain-Oropeza and Renteria-Salcedo, 2019), where the competencies were analyzed transversally without distinguishing the area of specialization of the participants. Data processing included the selection of potentially significant variables, as well as the treatment of categorical variables and the normalization of numerical variables, in order to assess the robustness of the models for each Academic School.
The educational model (Tec21) from which the data was obtained for the study is based on competencies. In Tec 21 there are two evaluation factors: on the one hand, the final grade and, on the other hand, the obtaining of the competencies associated with each course. These evaluation factors are independent. Thus, a student can pass the course (when obtaining a final grade > =70/100) but not obtain the competencies. Similarly, a student might obtain the competencies but fail the course. Because competencies are not exclusive to a single course but are distributed in a group of courses, a student who did not achieve a competence will have the opportunity to achieve it in other classes. When a student accredits a level of competence through a course, this level is marked as achieved in the student’s record of competencies and can no longer be reversed. If the student subsequently fails a previously achieved competence, the student does not have to reaccredit that competency. An analysis corresponding to obtaining competencies with valid results was performed, even with the limitation of the specific data analyzed in this study as seen in section 3.4. A careful selection of the analyzed database was carried out, highlighting the values of interest according to the CRISP-DM methodology (Abbasi et al., 2016). Thus, the models developed by analyzing the database, showed high performance in their accuracy.
The relationship between high grades for students and the acquisition of competencies is demonstrated analytically. Figure 2 shows the above statement. In Salas Velasco (2014), a proposed model for the development of competencies in their graduates is described by assuring that evaluation positively improves competencies with strong econometric evidence. Then, the premise that the final grade is a significant predictor for the attainment of competencies and its opposite effect at par is reinforced. However, results should be taken carefully, as the observed correlation does not necessarily imply causation. Further investigation is needed to fully understand the underlying factors and to confirm these findings across different contexts and populations.
An analysis was performed to estimate the probability of acquiring the competencies analyzed related to a more precise score range by the students. Table 1 shows the score ranges of the final variable for the probability of obtaining each of the two competencies under study. There are not many references that converse between grades and competencies, although (Chernikova et al., 2020) comments that diagnostic competencies are adequate for less advanced students, or that self-regulation levels are more effective for advanced students, there is no consistent analysis between grades for this level of students. The data in this paper support the idea that students’ final grades are a significant indicator of their likelihood of achieving competencies, highlighting a direct correlation between high academic performance and the acquisition of competencies valued by the educational institution. This implies that students who achieve higher final grades are more likely to obtain the competencies that the educational institution deems important. It suggests that academic performance, as measured by final grades, is closely related to the attainment of key skills and knowledge that the institution aims to impart. Therefore, focusing on academic success could be a crucial pathway to ensuring students acquire these valuable competencies.
It’s worth mentioning that final grades may also be influenced by various external factors such as teaching quality, assessment methods, and individual student circumstances, which could affect the generalizability of the results. For example, variations in teaching quality across different instructors or courses can lead to differences in grading standards and student performance. Similarly, assessment methods that favor certain types of learners over others can skew final grades, making them less reliable as indicators of competency attainment. Individual student circumstances, such as socioeconomic status, health issues, or personal responsibilities, can also impact academic performance and, consequently, final grades. Given these complexities, a holistic approach that considers multiple factors is essential to draw more comprehensive conclusions.
This research has shown a significant relationship between academic performance and acquiring transversal competencies of complex thinking and digital transformation in higher education. These findings are consistent with previous studies highlighting the importance of outstanding academic performance as a predictor of a student’s ability to develop critical and advanced skills needed in the digital age (e.g., Salas Velasco, 2014; Chernikova et al., 2020). Thus, our study not only reaffirms the observed correlation between final grades and the acquisition of competencies but also broadens the understanding of how curricular and extracurricular variables influence this process. The ethical considerations adhered to throughout the study ensure that these conclusions are drawn from a robust and ethically sound dataset.
By demonstrating how competency-based educational models, such as Tecnológico de Monterrey’s Tec21 Educational Model, can positively impact students’ professional and personal development, the results significantly contribute to the field of educational innovation. Through its focus on specific skills that are critical to the contemporary labor market, this empirical study provides a solid foundation for future educational theories and models that are responsive to these dynamics.
From a practical perspective, the results underscore the importance of designing educational interventions that focus not only on academic performance but also on the comprehensive development of competencies crucial in the contemporary labor market. This approach is especially relevant within the framework of Tecnológico de Monterrey’s Tec21 educational model, which seeks to prepare students for academic success and outstanding performance in complex and changing professional contexts.
Limitations and future direction. A crucial aspect that emerges from this study is the variability in the influence of specific competencies on academic outcomes, considering additional variables and diverse educational contexts to further validate and expand upon these findings by keeping ethical aspects. Although a strong association was observed between high grades and the acquisition of competencies, it is necessary to recognize that not all high-achieving students achieve these competencies, suggesting the existence of other mediating or moderating factors in this process, such as personal motivation, teaching methods, and the learning environment. In addition, the prevalence of acquired competencies in our dataset could induce bias in the predictive models used, suggesting the need for future research to employ alternative methods to control these deviations and explore the underlying causes of these differences. Based on the limitations and findings of the study, it is recommended that future research explore the mediating and moderating factors that influence the relationship between academic performance and competency acquisition. In addition, it would be beneficial to investigate how different teaching methods and learning environments may affect the development of cross-cutting competencies.
The data analyzed in this study is subject to the following licenses/restrictions: The use of the data was restricted to academic and research purposes only. Requests to access these datasets should be directed to am9zZS5tb2xpbmFAdGVjLm14.
The studies involving humans were approved by Institute for the Future of Education, Tecnológico de Monterrey. The studies were conducted in accordance with the local legislation and institutional requirements. Written informed consent for participation was not required from the participants or the participants' legal guardians/next of kin in accordance with the national legislation and institutional requirements.
JM-E: Conceptualization, Data curation, Methodology, Supervision, Validation, Writing – original draft, Writing – review & editing. PS-B: Conceptualization, Project administration, Writing – review & editing. BG-P: Formal analysis, Visualization, Writing – original draft. EL-C: Resources, Validation, Writing – review & editing. MG-M: Supervision, Validation, Visualization, Writing – review & editing.
The author(s) declare that financial support was received for the research, authorship, and/or publication of this article. The authors would like to thank the financial support from Tecnologico de Monterrey through the "Challenge-Based Research Funding Program 2022". Project ID # I003 -IFE001 -C2-T3 -T.
The authors would like to acknowledge the Living Lab & Data Hub of the Institute for the Future of Education, Tecnologico de Monterrey, Mexico, for the data used in this work and provided through the Call “Fostering the Analysis of Competency-based Higher Education.” The authors acknowledge the financial and technical support of Writing Lab, Institute for the Future of Education, Tecnologico de Monterrey, Mexico, in the production of this work.
The authors declare that the research was conducted in the absence of any commercial or financial relationships that could be construed as a potential conflict of interest.
All claims expressed in this article are solely those of the authors and do not necessarily represent those of their affiliated organizations, or those of the publisher, the editors and the reviewers. Any product that may be evaluated in this article, or claim that may be made by its manufacturer, is not guaranteed or endorsed by the publisher.
Abbasi, A., Sarker, S., and Chiang, R. H. L. (2016). Big data research in information systems: toward an inclusive research agenda. J. Assoc. Inf. Syst. 17:423. doi: 10.17705/1jais.00423
Aditya, B. R., Ferdiana, R., and Kusumawardani, S. S. (2021). A barrier diagnostic framework in process of digital transformation in higher education institutions. J. Appl. Res. High. Educ. 14, 749–761. doi: 10.1108/jarhe-12-2020-0454
Baena-Rojas, J. J., Ramírez-Montoya, M. S., Mazo-Cuervo, D. M., and López-Caudana, E. O. (2022). Traits of complex thinking: a bibliometric review of a disruptive construct in education. J. Intellig. 10:37. doi: 10.3390/jintelligence10030037
Baena-Rojas, J. J., Suárez-Brito, P., and López-Caudana, E. (2023). Reflections about complex thought and complex thinking: why these theoretical constructs maters on higher education? Eur. J. Contemp. Educ. 12, 4–18. doi: 10.13187/ejced.2023.1.4
Bailly, A., Blanc, C., Francis, É., Guillotin, T., Jamal, F., Wakim, B., et al. (2022). Effects of dataset size and interactions on the prediction performance of logistic regression and deep learning models. Comput. Methods Programs Biomed. 213:106504. doi: 10.1016/j.cmpb.2021.106504
Bygstad, B., Øvrelid, E., Ludvigsen, S., and Dæhlen, M. (2022). From dual digitalization to digital learning space: exploring the digital transformation of higher education. Comput. Educ. 182, 104463–104411. doi: 10.1016/j.compedu.2022.104463
Campos, E., Daruich, S., Enriquezdela, O. J., Castaño, R., Escamilla, J., and Hosseini, S. (2022). Educational model transition: Student evaluation of teaching amid the COVID-19 pandemic. Front. Educ. 7:991654. doi: 10.3389/feduc.2022.991654
Cervantes, J., Garcia-Lamont, F., Rodríguez-Mazahua, L., and Lopez, A. (2020). A comprehensive survey on support vector machine classification: applications, challenges and trends. Neurocomputing 408, 189–215. doi: 10.1016/j.neucom.2019.10.118
Chen, T., and Guestrin, C. (2016). XGBoost: a scalable tree boosting system. In Proceedings of the 22nd ACM SIGKDD international conference on knowledge discovery and data mining (KDD '16) Association for Computing Machinery, New York, NY, 785–794.
Chernikova, O., Heitzmann, N., and Fink, M. C. (2020). Facilitating diagnostic competences in higher education—a Meta-analysis in medical and teacher education. Educ. Psychol. Rev. 32, 157–196. doi: 10.1007/s10648-019-09492-2
Dask Development Team. (2024). Dask documentation. Available at: https://docs.dask.org/en/stable/ (Accessed July 9, 2024).
Fadel, C. (2008). Multimodal Learning Through Media: What the Research Says. Available at: https://www.researchgate.net/publication/237593175_Multimodal_Learning_Through_Media_What_the_Research_Says (Accessed October 15, 2024).
Garcez, A., Silva, R., and Franco, M. (2022). Digital transformation shaping structural pillars for academic entrepreneurship: a framework proposal and research agenda. Educ. Inf. Technol. 27, 1159–1182. doi: 10.1007/s10639-021-10638-5
George-Reyes, C. E., Peláez Sánchez, I. C., Glasserman-Morales, L. D., and López-Caudana, E. O. (2023). The Metaverse and complex thinking: opportunities, experiences, and future lines of research. Front. Educ. 8:1166999. doi: 10.3389/feduc.2023.1166999
González-Pérez, L. I., and Ramírez-Montoya, M. S. (2022). Components of education 4.0 in 21st century skills frameworks: systematic review. Sustain. For. 14, 2–31. doi: 10.3390/su14031493
IFE Data Hub (2023). “Higher Education Competency Dataset based on the TEC21 Educational Model of Tecnologico de Monterrey”, Tecnológico de Monterrey, V2.
Industrial Global Union. (2016). What is industry 4.0? Available at: https://www.industriall-union.org/industry-40-the-industrial-revolution-happening-no (Accessed July 9, 2024).
Kennedy, T., and Sundberg, C. (2020). “Chapter 32. 21st century skills” in Science education in theory and practice. An introductory guide to learning theory. eds. B. Akpan and T. Kennedy (Cham: Springer), 479–496.
López, H. A., Ponce, P., Molina, A., Ramírez-Montoya, M. S., and Lopez-Caudana, E. (2021). Design Framework Based on TEC21 Educational Model and Education 4.0 Implemented in a Capstone Project: A Case Study of an Electric Vehicle Suspension System. Sustainability 13. doi: 10.3390/su13115768
McKinney, W. (2010). Data structures for statistical computing in Python. Proceedings of the 9th Python in Science Conference, 51–56.
Medina, R. B., Ordoñez, S. J., and Packza, M. E. U. (2021). The Tec21 educational model and its perception. An educational innovation for student-based learning. 2021 IEEE Global Engineering Education Conference (EDUCON), 861–866.
Mikheev, A., Serkina, Y., and Vasyaev, A. (2021). Current trends in the digital transformation of higher education institutions in Russia. Educ. Inf. Technol. 1–15. doi: 10.1007/s10639-021-10467-6
Miranda, J., Navarrete, C., Noguez, J., Molina-Espinosa, J.-M., Ramírez-Montoya, M.-S., Navarro-Tuch, S. A., et al. (2021). The core components of education 4.0 in higher education: three case studies in engineering education. Comput. Elect. Eng. 93:107278. doi: 10.1016/j.compeleceng.2021.107278
Modelo Tec. (n.d.). Tecnológico De Monterrey. Available at: https://tec.mx/es/modelo-tec (Accessed July 9, 2024).
Oficina Regional de Educación para América Latina y el Caribe. (2017). E2030: Educación y habilidades para el siglo XXI. Reunión Regional de Ministros de Educación de América Latina y el Caribe Buenos Aires, Argentina, 24 y 25 de enero de 2017. Organización de las Naciones Unidas para la Educación, la Ciencia y la Cultura. Available at: https://unesdoc.unesco.org/ark:/48223/pf0000250117 (Accessed July 9, 2024).
Olivares Olivares, S. L., Islas, L., Rafael, J., Garín, P., José, M., and Rodríguez Chapa, J. A.., Aguayo Hernández, C. H., and Peña Ortega, L. O.. (2021). Modelo Educativo Tec21: Retos para una vivencia que transforma. Monterrey, México: Editorial Digital Tecnológico de Monterrey. Available at: https://www.researchgate.net/publication/353889494_Modelo_Educativo_Tec21_retos_para_una_vivencia_que_transforma (Accessed April 22, 2024).
Ostmeier, E., and Strobel, M. (2022). Building skills in the context of digital transformation: how industry digital maturity drives proactive skill development. J. Bus. Res. 139, 718–730. doi: 10.1016/j.jbusres.2021.09.020
Patiño, A., Ramírez-Montoya, M. S., and Buenestado-Fernández, M. (2023). Active learning and education 4.0 for complex thinking training: analysis of two case studies in open education. Smart Learn. Environ. 10, 1–21. doi: 10.1186/s40561-023-00229-x
Pérez, J. A., and Campos, J.-M. (2021). Tec 21: First outcomes of a new integral university framework for long-life education through challenge-based learning. 2021 IEEE Global Engineering Education Conference (EDUCON), 316–321.
Python Software Foundation. (2024). Python language reference. Available at: https://www.python.org (Accessed July 9, 2024).
Ramírez-Montoya, M. S., Castillo-Martínez, I. M., Sanabria-Z, J., and Miranda, J. (2022). Complex thinking in the framework of education 4.0 and open innovation—a systematic literature review. J. Open Innov. Technol. Mark. Complex. 8:4. doi: 10.3390/joitmc8010004
Rof, A., Bikfalvi, A., and Marques, P. (2022). Pandemic-accelerated digital transformation of a born digital higher education institution: towards a customized multimode learning strategy. Educ. Technol. Soc. 25, 124–141.
Salas Velasco, M. (2014). Do higher education institutions make a difference in competence development? A model of competence production at university. High. Educ. 68, 503–523. doi: 10.1007/s10734-014-9725-1
Schonlau, M., and Zou, R. Y. (2020). The random forest algorithm for statistical learning. Stata J. 20, 3–29. doi: 10.1177/1536867X20909688
Suárez-Brito, P., Baena-Rojas, J. J., López-Caudana, E. O., and Glasserman-Morales, L. D. (2022a). Academic literacy as a component of complex thinking in higher education: a scoping review. Eur. J. Contemp. Educ. 11, 931–945. doi: 10.13187/ejced.2022.3.931
Suárez-Brito, P., López-Caudana, E. O., Baena-Rojas, J. J., and Ramírez-Montoya, M. S. (2022b). Eliciting complex thinking through open educational resource projects. J. Soc. Stud. Educ. Res. 13, 56–77.
Swain-Oropeza, R., and Renteria-Salcedo, J. A. (2019). Tec21 Can Be an Educational Model for a VUCA World. 2019 IEEE 11th International Conference on Engineering Education (ICEED), 147–152.
Teixeira, A. F., Gonçalves, M. J. A., and Taylor, M. D. L. M. (2021). How higher education institutions are driving to digital transformation: a case study. Educ.Sci. 11:636. doi: 10.3390/educsci11100636
UN (2021). COVID-19 and youth: COVID-19 and learning. Available at: https://www.un.org/en/academic-impact/covid-19-and-youth-learning-and-employment (Accessed July 9, 2024).
UN (2022a). Goal 4. Ensure inclusive and equitable quality education and promote lifelong learning opportunities for all. Available at: https://sdgs.un.org/goals/goal4 (Accessed July 9, 2024).
UN. (2022b). Do you know all 17 SDGs? Available at: https://sdgs.un.org/es/goals (Accessed July 9, 2024).
Vázquez-Parra, J. C., Alonso-Galicia, P. E., Cruz-Sandoval, M., Suárez-Brito, P., and Carlos-Arroyo, M. (2023). Social entrepreneurship, complex thinking, and entrepreneurial self-efficacy: correlational study in a sample of Mexican students. Admin. Sci. 13, 2–18. doi: 10.3390/admsci13040104
Villanueva, A. V. (2018). El Tec transforma su modelo educativo; será más flexible y vivencial. Tecnológico De Monterrey. Available at: https://conecta.tec.mx/es/noticias/nacional/educacion/el-tec-transforma-su-modelo-educativo-sera-mas-flexible-y-vivencial (Accessed April 22, 2024).
Walsh, L., Gleeson, J., Magyar, B., and Gallo Cordoba, B. (2021). Life, disrupted: young people, education and employment before and after COVID-19. Melbourne: Monash University.
WEF. (2019). Here’s how to improve critical thinking and why it’s important. Available at: https://www.weforum.org/agenda/2019/10/improve-critical-thinking-why-important/ (Accessed October 15, 2024).
World Economic Forum. (2016a). New vision for education: fostering social and emotional learning through technology. Available at: https://www3.weforum.org/docs/WEF_New_Vision_for_Education.pdf (Accessed July 9, 2024).
World Economic Forum. (2016b). The future of jobs employment, skills and workforce strategy for the fourth industrial revolution. Available at: https://www3.weforum.org/docs/WEF_Future_of_Jobs.pdf (Accessed July 9, 2024).
Keywords: reasoning for complexity, educational innovation, higher education, digital transformation, academic performance, students, competency-based education performance, Tec21
Citation: Molina-Espinosa JM, Suárez-Brito P, Gutiérrez-Padilla B, López-Caudana EO and González-Mendoza M (2024) Academic performance as a driver for the development of reasoning for complexity and digital transformation competencies. Front. Educ. 9:1426183. doi: 10.3389/feduc.2024.1426183
Received: 30 April 2024; Accepted: 07 October 2024;
Published: 01 November 2024.
Edited by:
Ana B. Bernardo, University of Oviedo, SpainReviewed by:
Mohamed Rafik Noor Mohamed Qureshi, King Khalid University, Saudi ArabiaCopyright © 2024 Molina-Espinosa, Suárez-Brito, Gutiérrez-Padilla, López-Caudana and González-Mendoza. This is an open-access article distributed under the terms of the Creative Commons Attribution License (CC BY). The use, distribution or reproduction in other forums is permitted, provided the original author(s) and the copyright owner(s) are credited and that the original publication in this journal is cited, in accordance with accepted academic practice. No use, distribution or reproduction is permitted which does not comply with these terms.
*Correspondence: José Martín Molina-Espinosa, am9zZS5tb2xpbmFAdGVjLm14
Disclaimer: All claims expressed in this article are solely those of the authors and do not necessarily represent those of their affiliated organizations, or those of the publisher, the editors and the reviewers. Any product that may be evaluated in this article or claim that may be made by its manufacturer is not guaranteed or endorsed by the publisher.
Research integrity at Frontiers
Learn more about the work of our research integrity team to safeguard the quality of each article we publish.