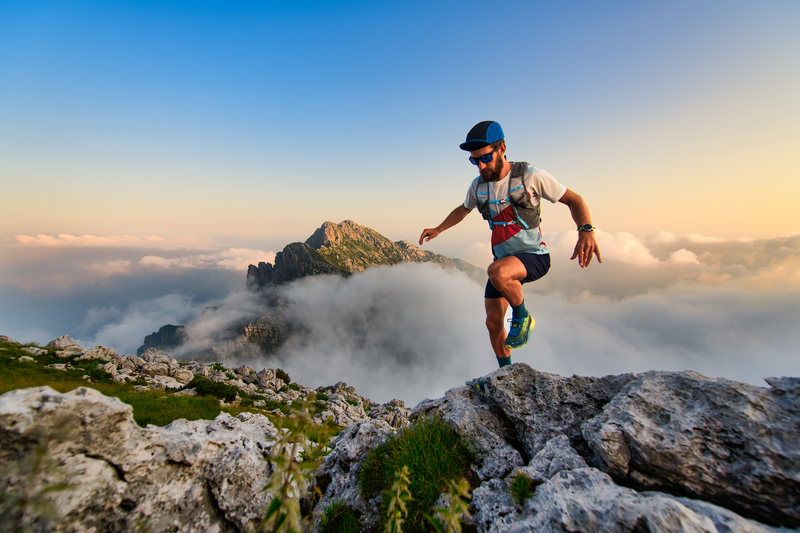
95% of researchers rate our articles as excellent or good
Learn more about the work of our research integrity team to safeguard the quality of each article we publish.
Find out more
ORIGINAL RESEARCH article
Front. Educ. , 27 November 2024
Sec. Higher Education
Volume 9 - 2024 | https://doi.org/10.3389/feduc.2024.1416033
This article is part of the Research Topic Future Places of Learning and Higher Education: a Humanistic Perspective. View all 4 articles
Introduction: Building upon previous research, this study aims to provide answers to the questions of how the presence of a humanoid artificial pedagogical agent as teacher and instructor and visual self-other representation of the learners through avatars influence the immediate cognitive performance and learning experience in online learning among adult learners.
Methods: Several outcome measures were investigated to evaluate if effects are the same or different for the different experimental conditions and if learning with the pedagogical agent and visual self-other representation is modulated by the learner’s previous experiences with and preferences for online learning. Teacher presence and self-other presence of the learners were experimentally manipulated. A humanoid artificial agent, visible on all of the slides of the online course material and instructing the material represented the teacher. The avatars of the learners (self-avatar and peer avatars) were kept of minimal functionality but self-avatars were preselected or could be self-selected by the learners. The learner’s cognitive learning performance, the learner’s attention to the pedagogical agent, their sense of teacher presence and of self- and other-presence, their satisfaction with the course as well as the learner’s previous learning experiences were measured by cognitive testing, self-report, and linguistic analysis as major performance indicators and a positive learning experience. The analysis comprised 133 university students and results were additionally compared for two subsamples.
Results: Learning performance, learning satisfaction, and the attention paid to the teacher were positively related. In addition, positive evaluations of the cognitive presence elicited by the teacher were found. Self- or other-presence of avatars did not significantly influence the learner’s performance beyond teacher presence but the learner’s perception of it and their motivation to study online.
Discussion: The study and its results extend the previous literature that focused on the effects of pedagogical agents in online teaching or on virtual representations of the learner’s self and classmates in online learning. Despite limitations, the results of this study provide insights into combining teaching with artificial pedagogical agents and visual avatars for self-other representation during online teaching and the observations can serve as catalyst for future research.
Teaching has moved to the digital world. Advanced technical solutions have been developed for digital applications in education and teaching (Haleem et al., 2022). These include fully immersive learning environments to simulate classrooms, for example through techniques such as virtual reality (e.g., Hu et al., 2023; Jovanović and Milosavljević, 2022; Soliman and Guetl, 2013). The rapid advances in technologies allow teachers and learners not only to interact digitally but also to represent themselves physically, cognitively, or socially in the online context by virtual representations of themselves.
However, the technical demands of fully immersive educational systems are still high. Therefore, one might ask how immersive and technologically sophisticated the virtual learning environments need to be to elicit a positive learning experience and learning performance of the learner. In other words, what level of functionality, interactivity, or visual presence might ensure that learners have a positive online learning experience and perform as intended when using an artificial pedagogical agent as the teacher and visual avatars for self-other representation?
This study aims to provide answers to the questions raised above by examining how both, an artificially generated pedagogical agent as an instructor and visual self-other representation through avatars influence the immediate learning performance and learning experience of adult learners including the learners’ perception of teacher presence, self- and other-presence, and their motivation and satisfaction with online learning. As suggested by previous research discussed below, these human factors could be important predictors and indicators of academic achievement and success in online learning in the context of higher education.
Numerous previous studies examined the effectiveness of online teaching compared to face-to-face teaching in higher education (for an overview, e.g., Martin et al., 2020; Martin et al., 2023; Stevens et al., 2021). The results of this research suggest that a number of variables can have a significant impact on the learning performance, learning satisfaction and learning experience of adult learners. While it has often been reported that learning performance among adult learners does not differ between online and face-to-face learning, recent scoping reviews incorporating previous meta-analyses and review studies suggest that online learning can potentially outperform face-to-face learning with respect to learning performance at multiple levels (e.g., Stevens et al., 2021).
At least three fundamental human factors seem to contribute to this superiority by influencing performance and experience during online learning significantly. First, the presence of a teacher as an instructor, second, the relationship between teachers and students with the learning content, and third, the presence of peers or the possibility of interactions between students (for an overview, e.g., Kusmaryono et al., 2021; Martin et al., 2020; Martin et al., 2023; Nortvig et al., 2018). Teacher presence has been shown to relate to a number of outcome measures including measures of the learner’s perceived learning performance and learning satisfaction (e.g., Caskurlu et al., 2020). Theoretically, teacher presence includes a number of aspects related to the design or organization of teaching, as well as the extent to which the teacher facilitates cognitive presence (i.e., processes that promote meaning construction and higher-order thinking) or elicits social presence to achieve positive learning outcomes. For the theoretical concept of teacher presence including cognitive and social presence, see, e.g., Anderson et al. (2001), or McKerlich et al. (2011) or Kozan and Richardson (2014). Regarding social presence, the possibility of interactions between the learners and teacher but also studying together with other classmates versus studying alone have been highlighted as important social factors that can improve the learner’s motivation and the learner’s satisfaction with online learning (Miao and Ma, 2022; Muzammıl et al., 2020; Turk et al., 2022). Theoretically and empirically, the role of the co- or other-presence (i.e., studying together with classmates as peers) has been suggested to be of high importance in the context of online learning [for recent overviews, see, e.g., Kreijns et al. (2022) and Whiteside et al. (2023)].
Taken together, these human factors have been highlighted in previous studies as significant factors influencing online learning. Nevertheless, they might modulate performance measures in different ways, consistent with findings that during online learning different outcome measures such as learning performance or learning satisfaction may not necessarily be positively related (Ebner and Gegenfurtner, 2019).
Several studies have examined the usefulness of pedagogical agent systems in online learning. Recent meta-analytic and review studies provide a summary of the results (e.g., Apoki et al., 2022; Castro-Alonso et al., 2021; Dai and Ke, 2022; Dai et al., 2022; Dai et al., 2024; Lane and Schroeder, 2022). The results of the studies included in the reviews and meta-analytical studies suggest a significant positive effect of learning online with pedagogical agents compared to online learning without pedagogical agents. The effect sizes achieved in some of the reviews and meta-analytic studies, however, were small regarding the hypothesis that pedagogical agents increase learning performance better compared to learning without pedagogical agents (e.g., Castro-Alonso et al., 2021; Schroeder et al., 2013). Furthermore, some of the studies summarized in the meta-analyses found no significant differences in learning outcome measures when teaching online with pedagogical agents was compared to teaching performed by real human tutors.
Recent meta-analytic studies that examined the influence of several core characteristics of pedagogical agents on performance measures, particularly regarding the dimensions of functionality, appearance, and interactivity also report no significantly different effects. For example in the meta-analysis by Castro-Alonso et al. (2021), no difference could be found regarding the effectiveness of pedagogical agents that have more or less visual complexity or functionality in appearance and interaction (e.g., including non-verbal behavior of the virtual agent such as actions, movements and gestures). Moreover, visual representation in 2D or 3D format compared to the visual, auditory or symbolic representation of the agent was examined. Across the reviewed studies, the results suggest that 2D agents might improve learning performance even better than 3D animations, depending on the context, the gender of the agent or the learner e.g., (see Castro-Alonso et al., 2021; Dai and Ke, 2022; Dai et al., 2022; Martha et al., 2019).
Very recent reviews (Dai and Ke, 2022; Dai et al., 2024), in which the authors specifically evaluated the use of technologically-advanced pedagogical agents whose appearance, behavior and expressiveness were generated by artificial intelligence (AI) found that AI-powered agents were associated with higher learning outcomes of the learners in the context of computer-based learning compared to non-AI-powered pedagogical agent systems. The observed effect sizes for artificially generated agents were higher than those reported in the previous reviews above.
In their reviews, the authors aimed to identify several key factors that could account for the effect sizes and effectivity of the agents (Dai and Ke, 2022; Dai et al., 2024). Among the critical ones were the intervention length of the course material. Interventions of shorter durations were associated with higher effect sizes. In addition, the type of appearance, i.e., the significance levels were higher for humanlike than virtual artificial agents. The results remained inconclusive with respect to the modality of agentic representation, i.e., the use of module-based AI versus generative AI technology [based on large language models or neural networks; for a broader discussion specifically on the use of generative AI tools such as ChatGPT in education, see Yilmaz and Yilmaz (2023) or Fink et al. (2024)]. Regarding the physical representation of the artificially generated agents, the meta-analyses compared text, audio, visual or audio-visual, the latter achieving slight advantages over the other types of representation (Dai and Ke, 2022; Dai et al., 2024).
As for example pointed out by Dai et al. (2024), the results about the role of core features of virtual agents in online learning could imply that less could be sometimes more (for discussions see also Apoki et al., 2022; Tao et al., 2022; Yusuf and Noor, 2023). Theoretically, the “less is more” principle is supported by neuropsychological and cognitive theories of learning and education such as the Cognitive Load Theory (CLT; e.g., Sweller, 2011). Theories of cognitive load such as the CLT have received broad attention in the context of multimedia learning (e.g., Mayer, 2014). According to cognitive load theories, a learner’s mental capacity in terms of attention, working memory, or information storage is limited in time and space. Therefore, attention, encoding, and recall are limited to only a certain amount of congruent information. In this view, any information not related to or only loosely related to the learning content can distract the learner and impair the learning process.
Moreover, there have been conjectures that a high level of humanness of the virtual agent could reduce user acceptance, facilitating the so-called uncanny valley (Mori, 1970). The uncanny valley describes a paradox in the user’s perception of virtual agents. In particular, the uncanny valley suggests that a decline in the acceptance of humanoid agents occurs the more realistic and human the agents behave and physically appear. Recent studies found no consistent proof of the uncanny valley, as outlined for example in Mishra et al. (2022) or pointed out in Tao et al. (2022). Accordingly, current evidence seems to suggest that the uncanny valley appears particularly in scenarios that promote the sharing of tasks between the user and the agent (e.g., Mishra et al., 2022).
Correspondingly, there seems no clear indication from the literature that pedagogical agents increase the learner’s cognitive demands (for overviews, e.g., Beege et al., 2023; Siegle et al., 2023; Tao et al., 2022; Yusuf and Noor, 2023). In a meta-analysis, Wang et al. (2017) found that adding pedagogical agents to onscreen lessons improves the learning performance (see Li et al., 2022 for improvements and validation on a neural level). Beckoned upon recent recommendations (Peng and Wang, 2022), the authors (Wang et al., 2017) speculated that a pedagogical agent with a humanlike voice and appearance, animated by gestures, facial expression, eye contact or movement can improve learning when visually presented as an instructor on the slides. This according to the authors holds true even when no direct interaction between learner and instructor takes place during online learning.
Similar to the use of pedagogical agents, the use of avatars for virtual self-other representation has received increased attention in previous research (e.g., Oh et al., 2018; Rahill and Sebrechts, 2021; Zimmermann et al., 2023). This broader literature discusses the role of self-other representation in the virtual world in general. Compared to this broader literature, fewer studies have explored how virtual representations of the learner’s self and those of other learners influence learning performance or the learner’s perception and satisfaction with online teaching (for a historical overview, e.g., Ledger et al., 2024).
The existing studies that were conducted in the context of higher education, however, suggest promising results of avatar use. The results of these studies suggest that presenting avatars during online teaching can foster a collaborative learning experience (Jovanović and Milosavljević, 2022; Yuan and Gao, 2024). In addition, several studies suggest that students who repeatedly participate in classes that use avatars for self-other representation voluntarily report an increase in engagement and feelings of immersiveness and improvement in their learning performance (Gregory and Bannister-Tyrrell, 2017). Interaction with the avatar, familiarity with the avatar, motivation of avatar use by the learner but also aesthetic preferences of the user seem important elements that ensure emotional attachment and learner satisfaction in online teaching (Cunningham, 2015; Falloon, 2009; for recent overviews see, e.g., Whiteside et al., 2023).
Despite this evidence, there is still debate to what degree mere self-other representation (without interaction) can facilitate the learning performance and the satisfaction of the learner (Baylor and Kim, 2009; Ratan et al., 2022). On one hand, the visual presence of avatars might enhance the self-efficacy and motivation of the learner (Baylor and Kim, 2009; Martha et al., 2019). Some evidence suggests that self-presence combined with other-presence might additionally increase the sense of belonging of the learner (Nortvig et al., 2018). On the other hand, self-presence and other-presence could facilitate fatigue or increase the cognitive and mental load of the learner, for example when the own self or those of other classmates are continuously displayed in the online classroom. Consequently, avatar-based self-other representations could distract attention away from the learning content, as has been argued for pedagogical agents as well (for an overview, e.g., Tao et al., 2022), especially when combined with in-class interaction (Yuan and Gao, 2024).
Despite the extensive evidence from previous studies, reviews and meta-analytic research briefly reviewed above, the research on artificial pedagogical agents and self-other presence through avatars in online learning still leave a number of questions open that need clarification in more detail. As outlined above, most research focused on pedagogical agents as teachers or on visual avatars as visual representations of the learners. Therefore, the effects of both, artificial pedagogical agents and self-other representation of the learners by avatars could be explored together in the same context and across different learning outcome measures in the same study design. In such a design, it could be determined if effects of teaching with an artificial pedagogical agent are the same or different depending on whether participants study online alone or with their virtual self or those of peers or with both (self and peers) represented by avatars in class. Furthermore, it could be determined if self- and other-presence modulate the learning experience differently and influence learning performance beyond that achieved by use of a pedagogical agent. Learning outcome measures could therefore examine the learner’s actual learning performance and the learner’s perception of it in combination with the learner’s perceived self, other- and teacher presence. This was, for example, explored recently by investigating cognitive learning performance in an online class in which learners were represented by visual avatars (Herbert and Dołżycka, 2022) and a humanoid pedagogical agent was the teacher. Initial results of this exploration suggested that representing learners through avatars did not affect nor improve immediate learning performance, even if the participants themselves selected the avatars for self-representation. However, the initial results suggested that learning performance might be better when participants had paid attention to the pedagogical agent that acted as instructor in line with the expectations proposed by Wang et al. (2017) and discussed in recent studies (Peng and Wang, 2022). Thus, how artificially generated pedagogical agents modulate learning performance in the context of self-other presence and whether virtual self-other representation modulates cognitive performance beyond teacher presence in online learning requires further investigations.
Consequently, the present study follows up on and extends the previous studies and findings summarized above with respect to these recommendations and research gaps. In summary, the following research questions will be further examined.
Research Question 1: Examine if a humanoid artificially generated pedagogical agent and self-other representation through avatars affect the immediate learning performance of adult learners during online learning immediately after one online session.
Research Question 2: Examine how a humanoid artificially generated pedagogical agent and self-other representation through avatars affect the learner’s experience including different outcome measures.
Research Question 3: Explore if effects of teaching with a pedagogical agent are the same or different across the experimental conditions of self-other representation for the different performance and outcome measures.
Research Questions 4: Control if effects are modulated by the learner’s previous experiences with and preferences for online learning.
In total, N = 133 participants (N = 73 women, N = 59 men, N = 1 diverse) took part in the online study. Participants were recruited via academic platforms using the local university mailing lists, SurveyCircle (https://www.surveycircle.com/de/) and Prolific (https://www.prolific.co/). Thus, local and global recruitment of the entire sample of participants included (1) data from local recruitment, (2) data from global recruitment [N = 65, see Herbert and Dołżycka (2022), PAAMS conference proceedings], and (3) data from the global recruitment that contained additional questions about teacher presence and self-other presence. For an overview of the data recruitment, see Figure 1.
Figure 1. Overview of the study design including the six experimental conditions and assignment of the participants to the six different experimental conditions as well as distribution of the survey content across the different recruiting periods, see the Method section for details. Examples of the visual avatars representing self-avatars or peer avatars are shown. As described in detail in the Method section, all participants including the group who studied without avatars (experimental group 6) were asked to pick a nickname for entering the course. For further illustrations of parts of the study design see also Herbert and Dołżycka (2022).
All participants were invited to take part in an introductory online course on neuroimaging, for details see Section 2.2. The inclusion criteria of participation specifically asked for beginners and learners to account for prior knowledge of the participants with the topic. The previous knowledge of the participants was controlled by asking participants about their academic background and experience with neuroimaging techniques. In addition, the participants were asked about their age and gender, their previous experience with and preferences for online learning and their primary and secondary education to check the inclusion criteria.
Ethics: The participants received detailed written information about the study, the voluntariness of the study participation, anonymity, data protection policy, and inclusion criteria for participation (age 18 and older, fluent English). All participants received the information that study participation is anonymous, with no personal information such as real name, or IP addresses stored that would allow their identification. They could opt out at any time during their participation without giving reasons or consequences and all participants gave written informed consent before taking part in the online study. Participation was granted only after written informed consent was provided online and when inclusion criteria were reached (age: 18 years and older).
The participants could register online for taking part in the online introductory course. In that course, basic principles of neurophysiological methods were explained. The topic of the course is relevant to several areas and disciplines of science, technology, engineering and mathematics (STEM) and its content fosters the understanding and comprehension of the underlying neurophysiology, physics, signal processing, computation and application of these methods in application domains such as medicine, psychology or biology. This allows the recruitment of participants from different disciplines, avoiding the selection of too homogeneous groups of participants.
The online study was designed using LimeSurvey software (https://www.limesurvey.org/de/). A study design with a pedagogical agent as teacher and with six different experimental conditions that varied in the degree of self-other representation was chosen. The experimental design has already been reported in Herbert and Dołżycka (2022, PAAMS conference proceedings). An overview of the study design and a summary can be found in Figure 1 and as follows. At the beginning of the experiment, the participants could choose a nickname for entering the course. Next, the participants were randomly assigned to one of the six experimental conditions. As illustrated in Figure 1, two experimental groups (group 1 and group 2 in Figure 1) studied with a self-avatar but without peer avatars. Two experimental groups studied with self-avatars and peer avatars (group 3 and group 4 in Figure 1). One experimental group studied with symbolic peer avatars but no self-avatar (group 5 in Figure 1), and one experimental group studied - like all other groups - with the pedagogical agent but without any self- or peer avatars for self-other representation (group 6 in Figure 1).
In the experimental groups with self-avatar (the group 1 and group 3 in Figure 1), the participants could choose an avatar from a list of possible characters (self-selected self-avatar). In the other groups with self-avatar (group 2 and group 4), the avatar was preselected by the experimental condition (preselected self-avatar, see Figure 1). The participants who were assigned to the experimental groups with self-selected avatars made their choice before entering the classroom. The participants who were assigned to the experimental groups 3 and group 4 (see Figure 1) studied additionally with avatars that represented peers.
The participants with self-selected or preselected self-avatars were instructed that they would take part in an introductory course with their avatars as the representation of their selves. They were told that their avatars would be shown during the course to the class. The participants who could study with the avatars representing peers received the instruction that they would study together with their peers whose avatars would be presented during the course. Participants in the condition without self- or peer avatars received no information about the presence of themselves or others and there were no avatars presented. They were asked to enter a nickname (as all other participants) that would associate the course with them. For an overview, see Figure 1.
During the neuroimaging course, all participants received the same instruction. They were instructed that they would take part in an online introductory course about neuroimaging techniques that will last about 9 min in duration. The content comprised basic principles about neuroimaging methods, such as electroencephalography (EEG) or functional magnetic resonance imaging (fMRI), advantages, disadvantages, and their respective practical applications in research. The online material was conceptualized according to protocols of previous face-to-face hands-on courses and seminars (see for an illustration Figure 2).
Figure 2. Example of the course material and the artificially generated humanoid pedagogical agent used as teacher and instructor of the course content. The pedagogical agent appeared on each slide, for details see the Method section. For further illustrations of the course material, see also Herbert and Dołżycka (2022). The humanoid virtual agent was designed with synthesia software, https://www.synthesia.io/de, for details see Methods.
The design of the course material followed general principles, rules, and recommendations for web-based, instructional, and user-centered designs as proposed for example by Nielsen (2016, 2020) or Vlasenko et al. (2023) that include recommendations for effectively designing online courses concerning layout, illustrations, visual hierarchy, color scheme, typesetting, readability, or adaptability. The participants were told that a teacher whom they would be introduced to at the beginning of the course would teach the course. No information was provided that the teacher is a humanoid artificially generated pedagogical agent. The participants were instructed that after the course they would be engaged in a quiz to test their acquired knowledge and learning performance, and answer questions about their learning experience.
A humanoid artificially generated agent was the instructor and teacher. A professional customized software solution was used (https://www.synthesia.io/tools/video-production-software). The software provides humanoid, artificially generated agents with different animated visual humanoid characters and voices varying in gender and culture. For the present study, an artificially generated avatar with female gender, with natural body movements and an expressive voice with American English prosody was chosen. The pedagogical agent was presented online on all sides of the course (for an illustration, see Figure 2). Its behavior was controlled by a computer script (text input). The script allowed for the alignment of the agent’s expressive behavior to the course material, for example, to take a pause when necessary and to stress or highlight the importance of some passages in the course that were relevant to the learning outcome assessed by the quiz at the end of the course. In addition, the agent’s eye-gaze behavior was selected by the software to simulate contact with the learner while the agent introduced the content presented on the slides. For illustration, see Figure 2 or the conference proceedings of Herbert and Dołżycka (2022).
The avatars for self- and other-representation were constructed using the Python Multiavatar library (https://github.com/multiavatar/multiavatar-python). The library contains static avatars with face, hair, and shoulders. Selection can be based on a number of character features such as skin, color, outfit, eyes, hairs, hair color, or moods, see Figure 1 for examples of the avatars chosen in the present study. Selection of avatars followed the following rules: avatars from the library who had features such as hair coverage (e.g., hats) or dark glasses covering eyes were excluded as were unrealistic characters with untypical facial hair, eye patches, heart eyes, etc. Facial expressions of the avatars provided by the software include the six basic emotions happiness, sadness, fear, anger, surprise, or disgust. In addition, a range of complex social emotions could be randomly generated with the software by combinations of mouth and eyebrow styles. The characters of the avatar and their physical appearance (N = 20 in total, mimicking a full class) were shown from the front. The avatars were shown in sitting positions simulating a classroom setting (sitting behind desks), see Figure 1 for an illustration. Peer avatars representing classmates and the avatars representing self-avatars were taken from the same library, created according to the same principles and all avatars were visible to the learner during the class from a third-person perspective at a size of 200 × 200 pixels, see Figure 1 for an illustration. For self-selection of the avatars, a list of 170 different avatar characters was provided and the participants in the groups with self-selected avatars could pick one to best represent their identity including physical appearance or affective or cognitive character traits. For details and further illustrations, see also the conference proceedings in Herbert and Dołżycka (2022).
The learning performance was tested in a quiz. The quiz comprised multiple-choice questions. These contained questions that tested the learner’s performance on the content taught by the teacher. The quiz followed the online course immediately after its completion. In addition, the participants filled in quiz items that asked the participant to recall characteristics of the teacher. These items were used to determine the degree of attention paid to the teacher during the course.
The experience of the learner during online learning was assessed by self-report methods. These comprised standardized questionnaires and self-construed survey items, for an overview see Table 1. In addition, preferences of the participants about online teaching were assessed following the items recommended in previous studies (e.g., Muthuprasad et al., 2021). The questions asked for the previous preferences of the participants to study online in a virtual classroom or attend classes in presence. The questions were as follows: (1) Online classes help me comprehend the course material better compared to classroom learning, (2) I prefer to study online within a virtual classroom, (3) I feel I can focus better when I attend classes in person, (4) My test performance has increased since I am attending classes virtually, and (5) Online classes help me comprehend the course materials better compared to classroom learning. Answers could be given on a Likert scale [1 (“not at all”) to 5 (“at all”)].
Table 1. Overview of the research tools (software, survey items, and standardized questionnaires) used in the present study.
The learner’s experience with the pedagogical agent was assessed by a series of nine questions that could be answered on 11-point Likert scales ranging from 0 (“not at all/strongly disagree”) to 10 (“at all/fully/strongly agree”). The questions asked for the perceived degree of teacher presence. Teacher presence included aspects of cognitive presence and social presence and additionally asked for the degree of humanness or artificiality. Cognitive presence included questions asking for (1) the attention paid to the teacher, (2) the distraction of attention caused by the teacher, (3) improved comprehension of the course material elicited by the teacher’s presence, (4) the perceived interaction with the teacher, (5) willingness to interact with the teacher and (6) willingness to ask the teacher a question. Social presence was represented by a survey question asking for the degree of (7) attachment felt to the teacher and a free writing task (see below). The questions are consistent with previous survey-based measures that assessed teacher presence, cognitive and social presence with a series of 34 items (Arbaugh et al., 2008). In the present survey, the focus was on questions that specifically asked for the teacher. Therefore, the items from Arbaugh et al. (2008) were adapted and modified for the present purpose. For example, the survey items of cognitive presence elicited by the teacher was shortened from “the instructor provided useful information from a variety of sources that helped me to learn” [see Arbaugh et al. (2008) to “the teacher helped me to focus on the present course material,” see Figure 3 and Table 1]. Feelings of humanness included questions about (8) the artificiality of the teacher, and (9) the realism/humanness of the teacher (for an overview see Table 1 and Figure 3). Besides these questions, the participants could express and evaluate their feelings about the teacher and the course in a few words in a free writing task. In addition, they could evaluate their overall satisfaction with the course by giving grades on a scale from 1 to 5 for course evaluation (see Figure 1 for an overview of the full study design).
Figure 3. Overview of the teacher questions concerning cognitive presence (elicited by the teacher) and social presence (elicited by the teacher) and their evaluation by the learners as obtained from the extended examination, for details see the Method section or the Results section. Numbers (x-aches) correspond to the item numbers. Y-aches represents the evaluation score (11-point Likert scales ranging from 0 to 10). Of note: Item three ranges from zero (“not distracted”) to 10 (“fully distracted”). Numbers in the bars represent mean scores of the participants.
The learner’s experience with self-avatars and peer avatars was assessed with a series of questions covering the following topics: (A) Representation of self by an avatar. (B) Representation of classmates by peer avatars. Questions from (A) were given to those participants who studied with a self-avatar. Questions from (B) were given to those participants who studied with peer avatars.
The questions in A asked for the sense of self-presence experienced by being represented by an avatar (see Table 1 and Figure 4). To this end, the standardized questionnaire by Gonzalez-Franco and Peck (2018) was used. Specifically, the items assessing agentic engagement were included to estimate the degree of felt self-presence (see Figure 4). Additionally, the participants were asked to evaluate the degree the self-avatar resembled their real physical appearance. The participants who could choose their avatar could additionally answer to which degree they enjoyed choosing their avatar (see Table 1 and Figure 4). Answers could be provided on 5-point Likert scales ranging from 1 (“strongly disagree”) to 5 (“strongly agree”). The questions in B asked for (1) the degree of other-presence, (2) the degree of attention paid to the peer avatars, (3) the degree of distraction elicited by the presence of others, and (4) the degree of motivation to learn harder with classmates compared to studying alone (see Figure 5). Moreover, the participants of the control group who were studying without any avatars (i.e., the experimental group 6 in Figure 1) were asked about their preferences as well, (e.g., 1) how they felt during studying alone, (2) how motivated they were to study and (3) if they would have preferred to study in class with classmates present (see Figure 5). The answers to these questions and those referring to (A) and (B) above were given on 11-point Likert scales (anchors: e.g., “strongly disagree/not at all” vs. “strongly agree/at all”).
Figure 4. Evaluation of the self-avatar by the participants studying with self-selected or preselected avatars for self-representation. Numbers (y-aches) represent the evaluation score (5-point Likert scales ranging from “strongly disagree” to “strongly agree”). Numbers (x-aches) correspond to the item numbers. Items 3 and 5 (highlighted with yellow lines) were given only to the participants studying with self-selected avatars. The star “*” illustrates significant differences, for details see the Results section.
Figure 5. Evaluation of other-presence and of its relevance for studying online. Comparison between experimental groups studying with and without peer avatars representing classmates. The star “*” illustrates significant differences. Numbers (x-aches) correspond to the item numbers. Of note: Item seven ranges from zero (“not distracted”) to 10 (“fully distracted”). The numbers in the bars represent mean values of the groups of participants.
Table 1 presents a full overview of the research tools and the survey of the online study as described in the Section 2.3.
Data analysis included descriptive and quantitative data analyses. Descriptive analysis included analysis of the distribution of the participants’ age, gender, familiarity with the topic, and previous preferences regarding studying online or face-to-face. Quantitative data analysis comprised parametric testing (repeated or univariate measures of variance, ANOVA) or non-parametric alternatives, e.g., for testing differences between experimental groups. Regarding parametric testing, p-values are reported corrected in case sphericity was not met using Greenhouse–Geisser, where appropriate. In addition, correlation analyses were performed (parametric: Pearson, two-sided, or non-parametric: Spearman-Rho or Kendal-Tau-b, two-sided, where appropriate) for examining the relationships between the outcome measures. Additionally, the analyses included calculations of Cronbach’s alpha for the survey questions asking about teacher presence and self-other presence to evaluate the internal consistency of these questions. Quantitative linguistic analysis was applied using Linguistic Inquiry and Word Count software (LIWC) (Pennebaker, 2001) for analysis of the free writing tasks in which the participants were asked to write about their course satisfaction and teacher evaluation. LIWC is a text analysis software that calculates from text a variety of linguistic markers based on the categorization of words according to semantic, emotional/affective, social, and other linguistic categories.
The statistical analyses were performed for the entire sample of participants (N = 133) (see Section 2.1.). Additional statistical analyses included comparisons between the study samples from the local and global recruitments that included the data (N = 68) from local and extended global recruitment (see Figure 1, and Section 2.1) and the data (N = 65) from initial analysis (Herbert and Dołżycka, 2022, PAAMS conference proceedings). The analyses of the full sample (N = 133) as well as the comparisons between the two datasets (N = 68 vs. N = 65) will help scrutinize the results from the initial analysis (Herbert and Dołżycka, 2022, PAAMS conference proceedings), verify results observed in the entire sample and aid replication. Statistical analysis was performed with the software package SPSS (https://www.ibm.com/de-de/spss). p-values are reported uncorrected to avoid too conservative correction of the results with only a few post hoc test comparisons, however, where appropriate corrected p-values are reported.
In line with the research questions outlined in the Section 1.5., the following hypotheses were tested with the experimental design described above.
Research Question 1 (RQ1): Artificial pedagogical agent, avatar self-other representation and learning performance.
H1a: Significant correlations between the quiz performance asking for the content taught by the teacher and the questions determining the degree of attention paid to the teacher (see Table 1) would be expected if learning performance is modulated by the presence of the artificial pedagogical agent instructing the course material.
H1b: If the learning performance is modulated additionally by avatar-based self-other representation, significant differences between the experimental groups and the quiz performance would be expected.
Research Question 2 (RQ2): Artificial pedagogical agent, avatar self-other representation and learning experience.
H2a: Positive evaluations of the teacher for the questions asking for teacher presence (see Table 1) and significant positive correlations with the learner’s satisfaction with the course would be expected, if the artificial pedagogical agent has positive effects on the learning experience.
H2b: Significant differences between the participants in the different experimental groups who studied with or without self- or peer avatars would be expected, if self-other representation through avatars influences the learning satisfaction differently. Moreover, positive evaluations of the avatars for the questions asking for self-other presence (see Table 1) and significant positive correlations with the satisfaction with the course and the learner’s motivation to study online would be expected if the visual avatars representing the self and peers have positive effects on the learning experience.
Research Question 3 (RQ3): Relationship between learning performance and learning experience.
H3a: Significant relationships between the different outcome measures would be expected, if the artificial pedagogical agent facilitates both, the cognitive performance and the learning experience of the learner.
H3b: Significant relationships between the different outcome measures could be expected, if avatar-based self-other representation modulates both, the learning performance and the learning experience of the learner.
Research Question 4 (RQ4): Role of the learner’s previous experiences with and preferences for online learning.
H4: Significant correlations between the participants’ self-reported previous learning preferences with the learning performance or the learner’s satisfaction with the course could be expected if learning with the artificial pedagogical agent and self-other representation is related to previous teaching and learning experiences of the learners.
The full participant sample (N = 133 participants) had a mean age of 25.59 years (SD = 9.23). In line with the inclusion criteria (see Section 2.1.), all participants were learners of neuroimaging. The majority of the participants (69%) reported little knowledge of neuroimaging (Mean: 1.53, SD = 0.68; Likert scale ranging from 1 to 5 with 1: no experience/learner vs. 5: advanced). Only 9% of the participant sample reported to have some neuroimaging knowledge. All participants were fluent in English.
As far as the previous learning experiences of the participants, as assessed with the standardized questions from Muthuprasad et al. (2021) are concerned, 28% of the participants (N = 133) reported preferring to study online (5-point Likert scale, answers 4–5). 8.3% of the participants (N = 133) denied this preference (5-point Likert scale, answer 1), whereas 64% of the participants (N = 133) had no particular preference (5-point Likert scale, answers 2–3). More than half of the participant sample (51.2% of N = 133, 5-point Likert scale: answers 1–2) replied that they did not comprehend the course material of their study better when attending previous classes online compared to traditional classroom learning. However, 66.1% of the participant sample (N = 133) replied that they feel they could focus better when attending previous classes in presence compared to attending previous classes online (5-point Likert scale: answers 4–5). In addition, 43% of the participant sample (N = 133) were uncertain about whether their learning performance has increased due to joining classes online previously (5-point Likert scale: answer 3). 6.8% of the participants (N = 133) fully disagreed with this question (5-point Likert scale: answer 1), whereas 10.5% of the participants (N = 133) fully agreed with this question (5-point Likert scale: answer 5).
The familiarity with the topic (being a learner) as well as the self-reported learning preferences regarding previous experiences with online learning of the entire participant sample (N = 133) did not differ as a function of the self-reported gender (p > 0.05) nor correlate with the age of the participants (Pearson, p > 0.05). Moreover, did the previous experience with and preferences for online learning not differ between the six experimental groups supporting that assignment of the participants to the experimental groups was unbiased with respect to the previous experiences or preferences for online learning among the participants. A repeated measures ANOVA with “preferences” as within-subject factor and “experimental group” as between subject factor did not yield a significant “group” effect, F(5,127) = 2.12, p = 0.07, partial-eta squared = 0.078, or a significant “preferences” by group interaction effect, F(5,127) = 0.834, p = 0.53, partial-eta squared = 0.032. In addition, familiarity with the topic did not differ significantly across the participants of the six experimental groups. A one-way-ANOVA with the factor “familiarity” and the group factor “experimental group” did not yield significant group effects, F(5,127) = 1.749, p = 0.128, partial eta squared = 0.064.
In addition, the comparison between the data of the two subsamples of participants recruited during the different recruiting periods (N = 65 vs. N = 68) did not show significant differences in gender, F(1,132) = 0.182, p = 0.670, partial-eta squared = 0.001. Additionally, the comparison of the data of these two samples showed no difference in terms of the previous learning preferences and experiences of the participants. A repeated measures ANOVA with “preferences” as within-subject factor and a between-subject and group factor did not show a significant “preferences” by group interaction effect, F(5,127) = 0.834, p = 0.526, partial-eta squared = 0.032.
The participants achieved a high learning performance in the quiz (mean = 5.25, SD = 1.17, range: 0–6). The analysis of the items examining the learner’s attention to the teacher showed an overall good recall performance of the teacher characteristics among the participants (N = 133, mean = 1.86, SD = 0.429, range: 0–2). In line with Hypothesis H1a, the recall of teacher characteristics and hence, the degree of attention paid to the teacher during online learning correlated significantly with the performance in the quiz (N = 133, Kendall-Tau-b = 0.200, p = 0.014, two-sided).
The cognitive performance in the quiz did not significantly differ as a function of the experimental groups. As illustrated in Figure 6, the group with self-selected avatars and peer avatars (experimental group 3 in Figure 1) showed the lowest quiz performance. In the one-way ANOVA containing the factor “quiz performance” as the main factor and “experimental group” as between-subject and group factor, the factor “experimental group” was not significant, F(5,127) = 2.032, p = 0.078, partial-eta squared = 0.074. In addition, pairwise comparisons comparing the performance among experimental groups showed no significant differences between the groups when corrected for multiple comparisons (Bonferroni). Also, non-parametric testing yielded no significant differences between experimental groups [Kruskal-Wallis, H(5) = 7.611, p = 0.179]. Recall of teacher characteristics and hence, the degree of attention paid to the teacher was lower in the group with self-selected avatars and peer avatars (experimental group 3 in Figure 1). Again, a one-way ANOVA, F(5,127) = 1.898, p = 0.099, partial-eta squared = 0.070 showed no significant effects of the factor “experimental group.” Moreover, individual comparisons between the experimental groups showed that recall scores of the six experimental groups were not significantly different from each other when corrected for multiple comparisons (Bonferroni). Likewise non-parametric testing yielded no significant differences in recall of teacher characteristics and hence, the degree of attention paid to the teacher between the experimental groups (Kruskal-Wallis, H(5) = 9.215, p = 0.101).
Figure 6. Learning performance (left column) and attention paid to the teacher (right column) as obtained from the quiz and the recall items of teacher characteristics.
The learning performance assessed by the quiz did not differ between the datasets of the two subsamples, F(1,131) = 2.275, p = 0.134, partial-eta squared = 0.017 (non-parametric: Mann-Withney-U (133) = 2060, p = 0.448). Recall of teacher characteristics and hence, the degree of attention paid to the teacher was lower in the dataset (N = 68) compared to the dataset (N = 65), but the difference was not statistically significant F(1,131) = 0.083, p = 0.774, partial-eta squared = 0.001 (non-parametric: Mann-Withney-U (133) = 2.281, p = 0.563). In addition, the analysis of each of the datasets separately did not yield different results. In both datasets, quiz performance was positively correlated with the degree of attention paid to the teacher [recall scores, N = 68: Kendal-Tau = 0.263, p = 0.024; N = 65, see Herbert and Dołżycka (2022)]. Furthermore, quiz performance and attention paid to the teacher (recall scores) did not significantly differ as a function of the experimental groups in both datasets [N = 68: quiz performance: Kruskal-Wallis H(5) = 1.252, p = 0.940; attention to the teacher as assessed by recall: H(5) = 4.835, p = 0.436; N = 65, see Herbert and Dołżycka (2022)]. Thus, the comparisons of the two datasets support the results observed in the entire sample (N = 133). Taken together, the analyses support hypothesis H1a, and yield no evidence that self-other representations through avatars significantly modulated learning performance differently or beyond that of studying with the artificial pedagogical agent (H1b).
The degree of attention paid to the teacher, as measured by recall scores in the entire sample (N = 133) correlated positively with the satisfaction of the participants with the course (r = 0.233, p = 0.015). In addition, Figure 3 illustrates the evaluations of the teacher by the participants (N = 37) for the additional questions included in the extended assessment that asked for teacher presence (see Table 1). The analysis of the internal consistency of the questions (teacher presence) revealed good internal consistency for the six questions asking for cognitive presence elicited by the teacher (Cronbach’s alpha =0.680). Internal consistency improved after removal of one question (question: distraction, Cronbach’s alpha =0.745). Comparisons of the questions related to the cognitive presence elicited by the teacher showed that attention paid to the teacher and the degree to which the teacher was perceived as a help to focus on the course material were evaluated significantly higher than the additional questions about teacher-learner interaction (repeated measures ANOVA, F(5,36) = 22.56, p = 0.001, partial-eta squared = 0.385).
Overall, this supports the hypothesis H2a, that teaching with the artificial pedagogical agents was positively related to the learner’s learning experience, especially by eliciting cognitive presence and improving learning satisfaction.
The social presence elicited by the teacher, i.e., the degree of attachment felt towards the teacher was on average evaluated significantly lower than the mean (Mean = 5.5, t-test for one sample t(36) = −5.216, p < 0.001; Cohen’s d = 1.970), see Figure 3. The effect size showed a weak effect. Additionally, the participants evaluated the artificiality of the pedagogical agent higher than its humanness, F(1,36) = 4.126, p = 0.050, partial-eta squared = 103. According to correlation analyses (Spearman-Rho), the participants’ evaluation of the cognitive presence and social presence (attachment to the teacher) were unrelated to their evaluations of whether the teacher was a human teacher or a pedagogical agent (p > 0.05). The observations obtained from the full participant sample in the free writing task in which the participants could report what they liked or disliked about the course including the teacher showed that only 27 subjects of the N = 133 participants explicitly stated that they did not like the teacher (e.g., the voice or other characteristics of the teacher). Linguistic analysis of the teacher characteristics revealed that participants (N = 133) used more positive (M = 3.82, SD = 6.959) than negative words (M = 1.00, SD = 3.045) for the description of the teacher, F(1,132) = 17.293, p < 0.05, partial-eta squared = 0.116.
Regarding self- and other-presence in the entire sample of participants (N = 133), the overall satisfaction with the course did not significantly differ between the experimental groups, irrespective of whether the participants studied with self-avatars, with peer avatars, with both or with no avatar [Kruskal-Wallis: H(5) = 3.66, p = 0.59]. Analysis of the standardized questionnaire items assessing the degree of agentic engagement (Gonzalez-Franco and Peck, 2018) elicited by avatar-based self-representation showed that the more or the less the avatar representing the learner was perceived as a mirror of the self, the more or the less it was perceived as a means of self-expression (Spearman-RHO, r = 0.431, p < 0.001). In addition, the more or the less the avatar representing the learner was perceived as a mirror of the self, the more or less it was perceived as having realistic features (Spearman-RHO, r = 0.295, p = 0.007) and as a physical resemblance of the self (Spearman-RHO, r = 0.735, p < 0.001). As shown in Figure 4, the participants who could self-select their avatar differed significantly in their evaluation of agentic engagement from the groups of participants with preselected self-avatars. In a repeated measures ANOVA with the factor “agentic engagement” as within-subject factor and the between-subject group factor “avatar choice” (self-selected vs. preselected), the group factor was significant, F(1,81) = 8.820, p = 004; partial-eta squared = 0.098. As shown in Figure 4, compared to the groups of participants who studied with preselected self-avatars, the groups of participants who could self-select their avatar evaluated their avatar significantly higher as a mirror of themselves, [t(81) = 2.771, p = 0.003, Cohen’s d = 0.611]. They agreed more that the avatar was a great means of self-expression [t(81) = 2.353, p < 0.001, Cohen’s d = 0.513], and they would use such an avatar again in other online teaching environments [t(81) = 2.809, p = 0.003, Cohen’s d = 0.619]. Additionally, they reported that self-selecting the avatar was fun (see Figure 4). However, both groups did not differ in their evaluation of the questions whether the avatar they studied with had realistic features, p > 0.05. Also, the evaluation of the question that their avatar resembled the own real physical appearance [t(81) = 2.265, p = 0.013, Cohen’s d = 0.499] was not significantly different between the two groups after Bonferroni correction (p = 0.65). Internal consistency of the questionnaire items filled in by all groups who studied with self-avatars showed a Cronbach’s alpha of 0.813.
Among the participants who studied with peer avatars, self-reported feelings of other-presence were positively correlated with the self-reported degree of attention paid to the peer avatars (Spearman-RHO, r = 0.789, p < 0.001) and negatively correlated with the degree to which the avatars distracted the attention away from the course (Spearman-RHO, r = −0.637, p = 0.003). In addition, the degree of felt other-presence was positively correlated with the self-reported motivation to attend the class (Spearman-RHO, r = 0.500, p = 0.029). The Cronbach’s alpha for these four items was high, (Cronbach’s alpha = 0.919) and did not improve or worsen after removal of any of the items, supporting high internal consistency of the questions asking for avatar-based other-presence.
Moreover, the participants who studied with peer avatars showed significant differences in the questions asking for preferences and the motivation to study with peers when compared to participants who did not have peer avatars. As shown in Figure 5, participants who had no peer avatar had a stronger preference to feel other-presence compared to the participants who studied with avatars representing peers (Kruskal-Wallis, H(1) = 12.519, p < 0.001). In addition, they reported that studying with peers would have made them significantly more attentive to the course compared to the evaluations of this questions by the participants who studied with avatars representing peers [Kruskal-Wallis, H(1) = 7.184, p = 0.007]. They also reported that studying with peers would have made them significantly more motivated compared to the evaluations of this questions by the participants who studied with avatars representing peers [Kruskal-Wallis, H(1) = 4.041, p = 0.044]. However, the latter difference showed no significance after Bonferroni correction. In line with these evaluations, among the participants who studied with peer avatars, the felt presence of others correlated negatively with the learner’s self-reported attention to the course (Spearman-RHO, r = −0.595, p = 0.007). Moreover and in line with these observations, felt other-presence was positively evaluated to increase the motivation to study online, however, it was not evaluated as helpful for focusing one’s attention to the course material during the online course.
Taken together, self-other representation through avatars did not significantly influence the learning satisfaction in line with hypothesis H2b, and had both, positive and negative relationships with measures of the learners’ perception of their learning performance and experience.
Learning performance in the quiz and the satisfaction of the participants with the course were correlated, i.e., the better the learning performance the higher the satisfaction (N = 133, Pearson, r = 0.185, p < 0.045). Moreover, quiz performance and satisfaction with the course correlated with the recall of teacher characteristics, and hence, the attention paid to the teacher during the course (see 3.2.1 and 3.3.1). Quiz performance or satisfaction of the participants with the course showed correlations with the answers to the questions asking for teacher presence. Correlation analyses showed that the learner’s satisfaction with the online course correlated positively with the learner’s willingness to interact with the teacher (Spearman-Rho: r = 0.645, p < 0.001) and to ask the teacher questions (Spearman-Rho: r = 0.449, p < 0.001). Quiz performance was positively correlated with the degree of artificiality of the teacher: the more the learners believed the teacher was an artificial agent, the better the quiz performance (Spearman-Rho: r = 0.389, p < 0.017).
Thus, regarding hypothesis H3a, the results suggest that teaching with pedagogical agents contributes to the factual learning performance and the satisfaction with online learning of the learner via the learner’s attention paid to teacher and additionally by the learner’s evaluation of cognitive presence as an aspect of teacher presence.
Regarding self-other presence, quiz performance and learning satisfaction were uncorrelated with the degree of felt self-presence assessed with the questions asking for agentic engagement (3.3.2), all p > 0.05. Felt other-presence correlated negatively with the factually achieved quiz performance (Spearman-RHO, r = −0.540, p = 0.017).
The results suggest that the perceived self-presence elicited through avatar presentation is unrelated with the learning performance or learning satisfaction of the learners. Increased feelings of other-presence might facilitate the motivation to study online but impact learning performance negatively (see Hypothesis H3b).
Self-reported previous experiences and preferences for studying online or in presence (see 3.2.1) were unrelated with the quiz performance (N = 133, Pearson or Spearman-RHO, p > 0.05). Regarding the hypothesis H4, previous experience did not differ significantly between the experimental groups or datasets (see 3.1.1.).
Previous studies have examined the effectiveness of pedagogical agents or the role of virtual self-other representation in online learning (see Introduction). The present study and its results support and extend a number of the findings reported in the literature.
The results suggest that learning performance and the learning experience of the learner are related to the presence of the artificial pedagogical agent as teacher. Learning outcome as measured by quiz performance was positively related to the visual attention paid to the teacher (as measured by test items and correct recall of visual characteristics of the pedagogical agent). This was observed in the full sample of participants (N = 133) regardless of which experimental conditions (studying with the pedagogical agent with or without self-avatars or peer avatars) the participants were assigned. In previous studies, the effects of pedagogical agents were often compared to a control condition of studying online without agents or with a real teacher (see Section 1.3. in the Introduction). The present findings extend these previous observations to comparisons of effects elicited by a humanoid artificially generated pedagogical agent in the context of teacher- and self-other presence. The pedagogical agent was presented in 2D, was present on each slide of the course material and acted as an instructor. The artificial agent’s voice was experimentally supported by gestures, facial expressions, and eye contact to facilitate the simulation of direct communication, although there was no direct interaction between learner and instructor during online learning.
It has been discussed if pedagogical agents due to their humanness in appearance or their perceptual or cognitive complexity and functionality could impose cognitive demands on the learner (e.g., Dai et al., 2024; Schroeder, 2011; Schroeder et al., 2013; Tao et al., 2022; Yusuf and Noor, 2023). However, recent research suggested that 2D pedagogical agents, especially when added onscreen to the teaching material can improve learning performance (Wang et al., 2017) and in terms of their non-verbal communicative characteristics have a positive effect on learning performance. This should hold when the agent is visually presented as an instructor on the slides, although no direct verbal interaction between learner and instructor takes place during online learning (e.g., Wang et al., 2017; Peng and Wang, 2022). The present study that refrained from allowing verbal interactions between the pedagogical agents as the teacher and the learner provides further empirical support for these observations and assumptions.
Moreover, the present results suggest that the humanness of the pedagogical agent may not affect the learning performance or the experience and satisfaction of the learner with online learning negatively. Theoretically, it has been proposed that a high degree of humanness could lead to confusion (see the so-called uncanny valley hypothesis, Mori, 1970) or that with respect to cognitive load “less could be sometimes more” in terms of core features of the virtual agents (for a discussion see also, e.g., Dai et al., 2024). However, as proposed and observed recently, confusion by humanness appears particularly when tasks are shared between humans and virtual agents (e.g., Mishra et al., 2022) which was not the case in the present study. Moreover, the participants evaluated the artificiality of the pedagogical agent higher than its humanness. As was proposed theoretically (Sweller, 2011; Mayer, 2014), during learning, cognitive load is induced especially by information not related to the content because it can distract attention and interfere with the learning process (for a discussion see Dai et al., 2024). In the present study, the quiz performance that tested the correct recall of the content of the course and the recall of teacher characteristics that tested the degree of attention to the teacher were positively related. Moreover, in the questions about teacher presence, there was no indication that participants felt distracted by the teacher. The relationship between measures of the learning performance (recall in the quiz) and attention paid to the teacher (recall of teacher characteristics) was found for the entire participant sample (N = 133) as well as when datasets were split into two subsamples that did not differ in terms of the assignment of participants to experimental groups. Furthermore, when asked about teacher presence, the participants evaluated these questions positively as was revealed specifically for the questions about the degree of cognitive presence elicited by the teacher during the course. Questions that asked for cognitive presence of the teacher achieved good internal consistency. The answers showed that participants self-reported to have paid attention to the teacher and the teacher was a help for the comprehension of the course material. Moreover, the learner’s feelings of interaction with the teacher were positively correlated with their course satisfaction and satisfaction of the learners with the course positively correlated with their learning performance. Positive teacher evaluations were also found in the sentiment analysis of the free writing task. Linguistic inquiry of word count as performed with the LIWC software (Pennebaker, 2001) suggested that the participants had an affectively positive evaluation of the pedagogical agent in the role of a teacher and instructor although overall the degree of attachment felt towards the teacher was evaluated as moderate and below the mean. As mentioned, there was no real interaction between the agent and the learners. Therefore, it could be expected that with regard to the different dimensions of teacher presence assessed in the present study including cognitive presence, social presence and humanness, cognitive presence evoked by the teacher should be more pronounced than social presence such as the learner’s feelings of attachment to the teacher. Theoretically, cognitive presence is related to any aspect in a learning environment that empowers students to construct meaning and understanding. It has been highlighted as an important aspect for promoting educational experience and learning success in virtual learning environments (Garrison and Anderson, 2003; and for a discussion, e.g., Sadaf et al., 2021). The present observations additionally align with findings from online teaching that compared the relevance of teacher presence for social presence and cognitive presence by determining the learners’ perceptions thereof (for an overview and discussion see, e.g., Annand, 2011). As discussed in Annand (2011), the results from these studies suggest that teacher presence and its ability to facilitate the learner’s cognitive presence might be considered more relevant than the teacher’s ability to elicit social presence. Furthermore, the present results suggest that teaching with a humanoid pedagogical agent can modulate the learner’s learning performance and learning experience independently of the self-other presence simulated by the avatars of the learner or those of the peers.
Self-other representation of the learners through avatars did not modulate the learning performance or the learner’s attention to the teacher. Performance and attention were not significantly different between the experimental groups although the experimental groups differed in the degree of self-other representation and comprised conditions in which the learner could study with self-avatars and peer avatars or without any virtual and symbolic self-other representation (see Figure 1). Only the participants who studied with self-selected avatars and peer avatars showed a lower quiz performance and lower attention to the teacher as opposed to the other experimental groups (see Figure 6), but effects and between group comparisons were not significant. Recent studies suggest that viewing oneself may contribute to reduced memory for the content during online learning (Tien et al., 2023). However, other studies report better learning performance in online learning when the learners can represent themselves by avatars (Ratan et al., 2022), especially when the avatars mimic the learner’s actual self as opposed to the learner’s ideal self or future self (Ratan et al., 2022). The present study does not support the hypothesis that the mere presentation of self-avatars decreases or increases the learning performance or influences the learner’s attention to the teacher differently. If this were the case, the experimental groups in which participants were able to select the avatar for self-representation or learned with preselected self-avatars would significantly differ from the groups of learners where peer avatars were also present or no avatars were present. The participants who could study with a self-avatar reported that studying with the avatar was a great means of self-expression. The degree of self-expression was evaluated higher in those participants with self-selected avatars than in the participants with preselected avatars (see Figure 4). The evaluations of the participants with self-selected avatars were higher compared to the groups who studied with a preselected self-avatar. None of the evaluations among these participants were, however, significantly related to their learning satisfaction or their factual quiz performance.
However, in contrast to the evaluations of studying with self-avatars, the evaluations of felt other-presence correlated negatively with the participants’ self-reported attention to the course suggesting that peer avatars can distract attention away from the course. This evaluation of the learners was found albeit studying with peer avatars was positively related to the self-reported motivation of the participants to attend the class.
The evaluations about other-presence are also interesting in comparison to the evaluations of the participants who were studying without avatars. Participants who studied without avatars reported that they would have preferred to study with classmates and that presence of peers might help focus on the course. Compared to these participants, participants who studied with peer avatars evaluated the relevance of peers as significantly lower for focusing one’s attention during the online course (see Figure 5). This discrepancy between the preferences for other-presence of learners who studied without peers and the evaluation of other-presence in those learners who studied with peer avatars might point to a bias in the perception of the learner. These biases suggest that learners might overestimate the relevance of peers for studying online. Observations of college and university students’ behavior in online teaching support this overestimation bias. Many students prefer to study anonymously during online classes (Reilly et al., 2012), e.g., preferring black screens with initials as the norm for self-other representation. However, the representation of self and classmates by avatars might become especially relevant for learning performance in interactive teaching during which the course material stimulates students to interact with each other and work jointly together. This would support previous findings that found a significant influence of peer interaction during video-based learning (Yuan and Gao, 2024) irrespective of the type of representation (real versus comic) avatars.
In summary, the results of self-other representation through avatars do not support the hypothesis (see hypothesis H2b) that virtual self-other presence would affect learning performance or learning satisfaction differently when at the same time an artificially generated agent is present as teacher and acting as an instructor. However, the results are well in line with a relationship between self-other presence and learning experience (see hypothesis H3b) that suggests that self-other representations play an important role for achieving a positive online learning experience, facilitating the learner’s emotions (fun) via sense of self-presence and their motivation to study online via sense of other-presence. A growing number of studies suggest that the learner’s previous preferences, experiences and expectancies towards online learning including the learner’s readiness for online learning can affect the learner’s expected learning performance and satisfaction (e.g., Joosten and Cusatis, 2020; Landrum et al., 2021; Wei and Chou, 2020). Control of the learner’s previous experience with online teaching as well as the learner’s preferences for attending classes in person or online suggests that in the present study, these factors did not influence the learner’s learning performance or the learner’s evaluation of or attention to the pedagogical agent or the avatars.
Although the study is one of the few studies to date to address the effectiveness of artificially generated humanoid pedagogical agents and self-other representation through avatars in the same study design and with multiple learning outcome measures in adult learners, the study has limitations. Therefore and as already explained above, the findings may apply to only specific conditions of online learning and should not be overgeneralized. However, as indicated in the following, the findings and their limitations can serve as a catalyst for future research attempting to study the effects of artificially generated pedagogical agents on learning in the context of self-other presence and vice versa. In summary, the discussion of the limitations of the study can be divided into four main categories. These are related to learner characteristics, the format and content of the online course, the study design including agent- and avatar representation as well as the type of performance measures.
Concerning learner characteristics as well as the format and content of the online course, the participant sample comprised beginners with little knowledge about the topic. In line with this, the topic was an introductory course. Furthermore, the introductory course on neuroimaging was a topic of STEM (areas of science, technology, engineering and mathematics), in line with most previous research (see Introduction). Neuroimaging is a topic for online learning because, as a topic from STEM, it involves different types of knowledge that require from the learner the understanding, comprehension, and application of basic principles of neurophysiology, physics, signal processing, and computation. These topics are relevant to a range of academic disciplines and allow the recruitment of a wide range of learners from different disciplines. Consequently, the selection of too homogeneous groups of learners could be avoided in the present study. In line with this, there were no restrictions of inclusion criteria for study participation with respect to country, subject, discipline or academic institution unless being a learner and little familiar with the topic and speaking English, English being the academic language worldwide. Although selection of less homogeneous groups of learners and a broad recruiting strategy can be considered a strength of the present study, the participant sample was not equally matched for gender. In total, more women than men took part in the study. Comparisons between the participants’ self-reported gender did not reveal any significant effects in the present study. However, gender effects have been reported in previous studies on online learning with respect to, for example, preferences for different learning styles or different teaching formats, access to STEM online courses or the learner’s preparedness or readiness for online learning (e.g., Idrizi et al., 2023; Scherer et al., 2023). Therefore, gender- and additionally cultural differences between learners could be explored further in future studies using similar broad recruiting strategies as in the present study and gender- and culturally diverse samples of learners.
In addition, differences in the learner’s attitudes toward online teaching have been reported to influence outcome variables of online learning in previous studies (e.g., Cuadrado-García et al., 2010; Dunn and Guadagno, 2012). Control of the learner’s previous experience with online teaching showed that all the participants were beginners not familiar with the topic taught by the pedagogical agent, and reported a preference to study in presence as opposed to studying online. This is suggestive of low self-efficacy expectations about online learning among the participants of the participant sample. Previous studies suggested that artificially generated humanoid pedagogical agents that are equipped with a “lifelike” or “persona” character can foster the learning process (for an overview and discussion see, e.g., Tao et al., 2022) and in the present study, this could be particularly relevant for students with low self-efficacy expectations about online learning.
The online material followed the principles pointed out by instructional design methods (Mayer, 2021). Moreover, the course duration and the content taught by the pedagogical agent followed the recommended format structure and length of online teaching blocks to reduce unnecessary cognitive processing that would impose additional cognitive demands on the learner (see Dai et al., 2024). Therefore, the learner’s perception of the artificially generated humanoid pedagogical agent and its impact on the learning performance might change, if the learner repeatedly takes part in similar courses with the same pedagogical agent or if the course duration will be increased. This could be tested in future studies, as only a few longitudinal studies exist.
In addition, the embedding of the pedagogical agent into the course material as well as its behavior as teacher interacting with the material was designed to be in accord with basic instructional design principles and recommendations from recent meta- and review studies (e.g., Dai et al., 2024; Tao et al., 2022: Wang et al., 2017). In line with these, the agent was a humanoid pedagogical agent. It had a human and realistic character presented in 2D and behaved as an instructor promoting cognitive processing, comprehension, and understanding of the learner by modulating the voice, intonation, and facial expression in temporal and spatial contiguity with the material (centering the avatar on each slide in line with the text). Therefore, the present results may hold only for humanoid artificial agents that are designed with considerations of these design aspects mentioned before.
Regarding the gender of the pedagogical agent, a female gender was chosen. Previous studies report a gender-teacher bias based on general expectations, beliefs, or stereotypes that a particular gender would perform better as a teacher or learner on a particular topic (for an overview see Tao et al., 2022). However, as concluded by Tao et al. (2022), current observations across studies do not provide conclusive confirmation that an agent’s gender modulates learning outcomes in a particular direction. This might in part be due to design characteristics of previous studies. The embedding of humanoid pedagogical agents acting as instructors is becoming increasingly popular in many areas of education, including teaching with multimedia designs (Lane and Schroeder, 2022). Therefore, in extension to previous studies and the present study, future studies could more systematically compare the effects of the agent’s gender in conjunction with other human factors such as the age, particular personality traits or the cultural background of the agent and the learner.
Regarding the study design, all participants studied the course material with the pedagogical agent. Comparisons of results of two subsamples (N = 68 vs. N = 65) did not yield different observations in the two subsamples and analysis of each subsample confirmed the results of the pedagogical agent and self-other representation on the learning performance and learning satisfaction observed in the full study sample (N = 133). Nevertheless, in future research, it would be interesting to compare the results with a control condition in which participants would learn without a pedagogical agent, similar to the manipulation of the experimental conditions for self-other presence. Consistent with this advancement, future research could additionally investigate the role of very abstract representations in more detail. Studies for example have shown that the mere representation of one’s identity by the subject’s own name can elicit feelings of ownership because of the salience and self-relevance of one’s name automatically eliciting a capture of attention (Alexopoulos et al., 2012). In the present study design, not only the experimental groups with avatars but also the participants assigned to the control condition (studying with the pedagogical agent only) could choose a nickname for self-representation that personalized the course to them. It could be examined in future studies whether even very abstract representations of the self, similar to the abstract representation of others, already elicit self-presence and contribute to social belonging. Although this would increase the need for larger sample sizes, this could be further explored by adding additional experimental conditions with and without nickname choice to the present study design.
Finally, previous studies suggest that the learner’s emotions and attachment to the learning environment can influence the learning process (e.g., Segaran et al., 2021). Achievement emotions such as enjoyment, hope, pride, relief, anger, anxiety, shame, hopelessness, and boredom during class play an important role in academic online learning (Hoferichter et al., 2022; Wu and Yu, 2022) because they can significantly modulate the learner’s motivation and learning performance (Schutz and Pekrun, 2007). Achievement emotions such as enjoyment are elicited when the learning context is experienced as controllable and valuable and hence, when the learner feels competent to master the learning material. In the present study, especially choosing the own avatar elicited joy. Analysis of the emotionality towards the teacher revealed that positive emotions were expressed more often than negative emotions in the free writing task. The participants also evaluated attachment to the teacher. Estimation of effect sizes regarding this social dimension of teacher presence yielded only a small effect size compared to the effects obtained for the measures of cognitive presence elicited by the teacher. The emotionality towards the peer avatars was not directly measured. Moreover, as reported above, the analysis of the learner’s experience included only a subsample of the participant sample. Furthermore, the pedagogical agent and avatars were not specifically designed to experimentally manipulate their emotionality, nor were achievement emotions or the effects of the agent and avatars on them directly examined in the present study. Nevertheless, the learner’s achievement emotions (Pekrun et al., 2009, 2011) could affect the learner’s perceptions and feelings towards the teacher and classmates in online learning and modulate the learner’s effort and performance (for a discussion, e.g., Chien et al., 2022).
Several outcome measures targeting the learner’s experience were already included in the present study. Nevertheless, in future studies, it could be interesting to include additional affective outcome measures to further investigate affective and emotional aspects of the agents and avatars and their importance as predictors of online learning (Beege et al., 2023). This could help achieve a better understanding of the relationship between affective and cognitive human factors in the design of agents and the learner’s perception of it. Given the increasing interest in this topic (for reviews, e.g., see Wong and Adesope, 2021), this could be especially further explored in the context of teaching with educational chatbots (Yin et al., 2024) or affective pedagogical agents (Wang et al., 2024). These agents could be not only artificially generated as in the present study but powered by generative AI or include interactive conversational agent systems (for an overview of generative AI in education, e.g., Bozkurt, 2023).
Methodologically, multi-method approaches that include standardized questions and text analysis methods in addition to standardized questionnaires could be very efficient additional research tools for decoding the learner’s emotions, implicit beliefs, and preferences of artificially generated pedagogical agents, and their influence on learning performance and learning experience in online lessons. The assessment of the learner’s AI literacy and competence could provide further insights into interindividual learner characteristic that could modulate effects (e.g., Çelebi et al., 2023; for an overview, e.g., Laupichler et al., 2022).
Despite a wealth of research on pedagogical agents, as pointed out by Dai et al. (2024) or Fink et al. (2024) there are only a number of studies that used artificially generated pedagogical agents (e.g., for an overview and discussion, see the recent meta-analysis of Dai et al., 2024). Therefore, the present study is still one of the few studies that aimed to combine research on artificially generated pedagogical agents with research on self-other representation of the learners (Yuan and Gao, 2024). Therefore, further investigations are needed into the specific conditions under which artificially generated humanoid pedagogical agents can promote the learning process when combined with self-other representation and vice versa.
The raw data supporting the conclusions of this article will be made available by the corresponding author, without undue reservation.
The participants received detailed written information about the study, the voluntariness of the study participation, anonymity, data protection policy, and inclusion criteria for participation (age 18 and older, fluent English). All participants received the information that study participation is anonymous, with no personal information such as real name, or IP addresses stored that would allow their identification. They could opt out at any time during their participation without giving reasons or consequences and all participants gave written informed consent before taking part in the online study. Participation was granted only after written informed consent was provided online and when inclusion criteria were reached (age: 18 years and older). The study was conducted in line with ethical guidelines. The participants provided their written informed consent online to participate in the study.
CH: Conceptualization, Data curation, Formal analysis, Funding acquisition, Investigation, Methodology, Project administration, Resources, Software, Supervision, Validation, Visualization, Writing – original draft, Writing – review & editing. JD: Investigation, Supervision, Writing – review & editing.
The author(s) declare that financial support was received for the research, authorship, and/or publication of this article. This study was funded by the budgetary resources of the Department of Applied Emotion and Motivation Psychology, Ulm University, Germany.
Ref-n-Write’s paraphrasing tool was used as a grammar checker to detect potential grammatical errors during writing. https://www.ref-n-write.com/paraphrasing-tool/.
The authors declare that the research was conducted in the absence of any commercial or financial relationships that could be construed as a potential conflict of interest.
All claims expressed in this article are solely those of the authors and do not necessarily represent those of their affiliated organizations, or those of the publisher, the editors and the reviewers. Any product that may be evaluated in this article, or claim that may be made by its manufacturer, is not guaranteed or endorsed by the publisher.
Alexopoulos, T., Muller, D., Ric, F., and Marendaz, C. (2012). I, me, mine: automatic attentional capture by self-related stimuli. Euro. J. Soci. Psych. 42, 770–779. doi: 10.1002/ejsp.1882
Anderson, T., Rourke, L., Garrison, D.R., and Archer, W. (2001). Assessing teaching presence in a computer conferencing context. Available at: https://olj.onlinelearningconsortium.org/index.php/olj/article/view/1875
Annand, D. (2011). Social presence within the community of inquiry framework. Int. Rev. Res. Open Distrib. Learn. 12, 40–56. doi: 10.19173/irrodl.v12i5.924
Apoki, U. C., Hussein, A. M. A., Al-Chalabi, H. K. M., Badica, C., and Mocanu, M. L. (2022). The role of pedagogical agents in personalised adaptive learning: a review. Sustain. For. 14:6442. doi: 10.3390/su14116442
Arbaugh, J. B., Cleveland-Innes, M., Diaz, S. R., Garrison, D. R., Ice, P., Richardson, J. C., et al. (2008). Developing a community of inquiry instrument: testing a measure of the community of inquiry framework using a multi-institutional sample. Internet High. Educ. 11, 133–136. doi: 10.1016/j.iheduc.2008.06.003
Baylor, A. L., and Kim, S. (2009). Designing nonverbal communication for pedagogical agents: when less is more. Comput. Hum. Behav. 25, 450–457. doi: 10.1016/j.chb.2008.10.008
Beege, M., Schroeder, N. L., Heidig, S., Rey, G. D., and Schneider, S. (2023). The instructor presence effect and its moderators in instructional video: a series of meta-analyses. Educ. Res. Rev. 41:100564. doi: 10.1016/j.edurev.2023.100564
Bozkurt, A. (2023). Unleashing the potential of generative AI, conversational agents and chatbots in educational praxis: a systematic review and bibliometric analysis of GenAI in education. Open Praxis 15, 261–270. doi: 10.55982/openpraxis.15.4.609
Caskurlu, S., Maeda, Y., Richardson, J. C., and Lv, J. (2020). A meta-analysis addressing the relationship between teaching presence and students’ satisfaction and learning. Comput. Educ. 157:103966. doi: 10.1016/j.compedu.2020.103966
Castro-Alonso, J. C., Wong, R. M., Adesope, O. O., and Paas, F. (2021). Effectiveness of multimedia pedagogical agents predicted by diverse theories: a Meta-analysis. Educ. Psychol. Rev. 33, 989–1015. doi: 10.1007/s10648-020-09587-1
Çelebi, C., Yılmaz, F., Demir, U., and Karakuş, F. (2023). Artificial intelligence literacy: an adaptation study. Instruct. Technol. Lifelong Learn. 4, 291–306.
Chien, H. Y., Yeh, Y. C., and Kwok, O. M. (2022). How online learning readiness can predict online learning emotional states and expected academic outcomes: testing a theoretically based mediation model. Online Learn. 26, 193–208. doi: 10.24059/olj.v26i4.3483
Cuadrado-García, M., Ruiz-Molina, M.-E., and Montoro-Pons, J. D. (2010). Are there gender differences in e-learning use and assessment? Evidence from an interuniversity online project in Europe. Procedia. Soc. Behav. Sci. 2, 367–371. doi: 10.1016/j.sbspro.2010.03.027
Cunningham, J. M. (2015). Mechanizing people and pedagogy: establishing social presence in the online classroom. Online Learn. 19, 34–47. doi: 10.24059/olj.v19i3.667
Dai, L., Jung, M. M., Postma, M., and Louwerse, M. M. (2022). A systematic review of pedagogical agent research: similarities, differences and unexplored aspects. Comput. Educ. 190:104607. doi: 10.1016/j.compedu.2022.104607
Dai, C. P., and Ke, F. (2022). Educational applications of artificial intelligence in simulation-based learning: a systematic mapping review. Comp. Educ. 3:100087. doi: 10.1016/j.caeai.2022.100087
Dai, C. P., Ke, F., Pan, Y., Moon, J., and Liu, Z. (2024). Effects of artificial intelligence-powered virtual agents on learning outcomes in computer-based simulations: a meta-analysis. Educ. Psychol. Rev. 36:31. doi: 10.1007/s10648-024-09855-4
Dunn, R. A., and Guadagno, R. E. (2012). My avatar and me – gender and personality predictors of avatar-self discrepancy. Comput. Hum. Behav. 28, 97–106. doi: 10.1016/j.chb.2011.08.015
Ebner, C., and Gegenfurtner, A. (2019). Learning and satisfaction in webinar, online, and face-to-face instruction: A meta-analysis. Front. Educ. 4:92. doi: 10.3389/feduc.2019.00092
Falloon, G. (2009). Using avatars and virtual environments in learning: what do they have to offer? Brit. J. Educ. Tech. 41, 108–122. doi: 10.1111/j.1467-8535.2009.00991.x
Fink, M. C., Robinson, S. A., and Ertl, B. (2024). AI-based avatars are changing the way we learn and teach: benefits and challenges. Front. Educ. 9:1416307. doi: 10.3389/feduc.2024.1416307
Garrison, D. R., and Anderson, T. (2003). E-learning in the 21st century: A framework for research and practice. London, New York: RoutledgeFalmer.
Gonzalez-Franco, M., and Peck, T. C. (2018). Avatar embodiment. Towards a standardized questionnaire. Front. Robot. AI 5:74. doi: 10.3389/frobt.2018.00074
Gregory, S., and Bannister-Tyrrell, M. (2017). Digital learner presence and online teaching tools: higher cognitive requirements of online learners for effective learning. RPTEL 12, 18–17. doi: 10.1186/s41039-017-0059-3
Haleem, A., Javaid, M., Qadri, M. A., and Suman, R. (2022). Understanding the role of digital technologies in education: a review. Sustain. Operat. Comp. 3, 275–285. doi: 10.1016/j.susoc.2022.05.004
Herbert, C., and Dołżycka, J. D. (2022). “Personalized avatars without agentic interaction: do they promote learning performance and sense of self in a teaching context? A pilot study” in International conference on practical applications of agents and multi-agent systems (Cham: Springer International Publishing), 169–180.
Hoferichter, F., Kulakow, S., and Raufelder, D. (2022). How teacher and classmate support relate to students’ stress and academic achievement. Front. Psychol. 13, 1–13. doi: 10.3389/fpsyg.2022.992497
Hu, Y.-H., Yu, H.-Y., Tzeng, J.-W., and Zhong, K.-C. (2023). Using an avatar-based digital collaboration platform to foster ethical education for university students. Comput. Educ. 196:104728. doi: 10.1016/j.compedu.2023.104728
Idrizi, E., Filiposka, S., and Trajkovikj, V. (2023). Gender impact on STEM online learning-a correlational study of gender, personality traits and learning styles in relation to different online teaching modalities. Multimed. Tools Appl. 82, 30201–30219. doi: 10.1007/s11042-023-14908-x
Jovanović, A., and Milosavljević, A. (2022). VoRtex Metaverse platform for gamified collaborative learning. Electronics 11:317. doi: 10.3390/electronics11030317
Kozan, K., and Richardson, J. C. (2014). Interrelationships between and among social, teaching, and cognitive presence. Internet High. Educ. 21, 68–73. doi: 10.1016/j.iheduc.2013.10.007
Kreijns, K., Xu, K., and Weidlich, J. (2022). Social presence: conceptualization and measurement. Educ. Psychol. Rev. 34, 139–170. doi: 10.1007/s10648-021-09623-8
Kusmaryono, I., Jupriyanto, J., and Kusumaningsih, W. (2021). A systematic literature review on the effectiveness of distance learning: problems, opportunities, challenges, and predictions. Int. J. Educ. 14, 62–69. doi: 10.17509/ije.v14i1.29191
Landrum, B., Bannister, J., Garza, G., and Rhame, S. (2021). A class of one: students’ satisfaction with online learning. J. Educ. Bus. 96, 82–88. doi: 10.1080/08832323.2020.1757592
Lane, H. C., and Schroeder, N. L. (2022). “Pedagogical agents” in The handbook on socially interactive agents: 20 years of research on embodied conversational agents, intelligent virtual agents, and social robotics volume 2: Interactivity, platforms, application, 307–330.
Laupichler, M. C., Aster, A., Schirch, J., and Raupach, T. (2022). Artificial intelligence literacy in higher and adult education: a scoping literature review. Comp. Educ. 3:100101 doi: 10.1016/j.caeai.2022.100101
Ledger, S., Mailizar, M., Gregory, S., Tanti, M., Gibson, D., and Kruse, S. (2024). Learning to teach with simulation: historical insights. J. Comput. Educ. 1–28. doi: 10.1007/s40692-024-00313-2
Li, W., Wang, F., Mayer, R. E., and Liu, T. (2022). Animated pedagogical agents enhance learning outcomes and brain activity during learning. Comp. Assist. Learn. 38, 621–637. doi: 10.1111/jcal.12634
Martha, A. S. D., Santoso, H. B., Junus, K., and Suhartanto, H. (2019). “A scaffolding design for pedagogical agents within the higher-education context” in Proceedings of the 11th international conference on education technology and computers, 139–143.
Martin, F., Dennen, V. P., and Bonk, C. J. (2023). Systematic reviews of research on online learning: an introductory look and review. Online Learn. J. 27, 1–15. doi: 10.24059/olj.v27i1.3827
Martin, F., Sun, T., and Westine, C. D. (2020). A systematic review of research on online teaching and learning from 2009 to 2018. Comput. Educ. 159:104009. doi: 10.1016/j.compedu.2020.104009
Mayer, R. E. (2014). Incorporating motivation into multimedia learning. Learn. Instr. 29, 171–173. doi: 10.1016/j.learninstruc.2013.04.003
Mayer, R. E. (2021). Evidence-based principles for how to design effective instructional videos. J. Appl. Res. Mem. Cogn. 10, 229–240. doi: 10.1016/j.jarmac.2021.03.007
McKerlich, R., Riis, M., Anderson, T., and Eastman, B. (2011). Student perceptions of teaching presence, social presence, and cognitive presence in a virtual world.
Miao, J., and Ma, L. (2022). Students’ online interaction, self-regulation, and learning engagement in higher education: the importance of social presence to online learning. Front. Psychol. 13:815220. doi: 10.3389/fpsyg.2022.815220
Mishra, N., Ramanathan, M., Tulsulkar, G., and Thalmann, N. M. (2022). Uncanny valley for interactive social agents: an experimental study. Virt. Real. 4, 393–405. doi: 10.1016/j.vrih.2022.08.003
Muthuprasad, T., Aiswarya, S., Aditya, K. S., and Jha, G. K. (2021). Students' perception and preference for online education in India during COVID-19 pandemic. Soci. Sci. 3:100101. doi: 10.1016/j.ssaho.2020.100101
Muzammıl, M., Sutawıjaya, A., and Harsası, M. (2020). Investigating student satisfaction in online learning: the role of student interaction and engagement in distance learning university. Turk. Online J. Dist. Educ. 21, 88–96. doi: 10.17718/tojde.770928
Nielsen, J. (2016). F-Shaped Pattern For Reading Web Content (original study). Nielsen Norman Group. Retrieved March 1, 2022, from https://www.nngroup.com/articles/f-shaped-pattern-reading-web-content-discovered/
Nielsen, J. (2020). 10 usability heuristics for user interface design. Nielsen Norman Group. Retrieved March 1, 2022, from https://www.nngroup.com/articles/ten-usability-heuristics/
Nortvig, A. M., Petersen, A. K., and Balle, S. H. (2018). A literature review of the factors influencing E-learning and blended learning in relation to learning outcome, student satisfaction and engagement. EJEL 16, 46–55.
Oh, C. S., Bailenson, J. N., and Welch, G. F. (2018). A systematic review of social presence: definition, antecedents, and implications. Front. Robot. AI 5:409295. doi: 10.3389/frobt.2018.00114
Pekrun, R., Elliot, A. J., and Maier, M. A. (2009). Achievement goals and achievement emotions: testing a model of their joint relations with academic performance. J. Educ. Psychol. 101, 115–135. doi: 10.1037/a0013383
Pekrun, R., Goetz, T., Frenzel, A. C., Barchfeld, P., and Perry, R. P. (2011). Measuring emotions in students’ learning and performance: the achievement emotions questionnaire (AEQ). Contemp. Educ. Psychol. 36, 36–48. doi: 10.1016/j.cedpsych.2010.10.002
Peng, T. H., and Wang, T. H. (2022). Developing an analysis framework for studies on pedagogical agent in an e-learning environment. J. Educ. Comput. Res. 60, 547–578. doi: 10.1177/07356331211041701
Rahill, K. M., and Sebrechts, M. M. (2021). Effects of avatar player-similarity and player-construction on gaming performance. Comp. Hum. Behav. Rep. 4:100131. doi: 10.1016/j.chbr.2021.100131
Ratan, R., Klein, M. S., Ucha, C. R., and Cherchiglia, L. L. (2022). Avatar customization orientation and undergraduate-course outcomes: actual-self avatars are better than ideal-self and future-self avatars. Comput. Educ. 191:104643. doi: 10.1016/j.compedu.2022.104643
Reilly, J. R., Gallagher-Lepak, S., and Killion, C. (2012). "Me and my computer": emotional factors in online learning. Nurs. Educ. Perspect. 33, 100–105. doi: 10.5480/1536-5026-33.2.100
Sadaf, A., Wu, T., and Martin, F. (2021). Cognitive presence in online learning: a systematic review of empirical research from 2000 to 2019. Comp. Educ. Open 2:100050. doi: 10.1016/j.caeo.2021.100050
Scherer, R., Siddiq, F., Howard, S. K., and Tondeur, J. (2023). Gender divides in teachers' readiness for online teaching and learning in higher education: do women and men consider themselves equally prepared? Comput. Educ. 199:104774. doi: 10.1016/j.compedu.2023.104774
Schroeder, R. (2011). Being there together: Social interaction in virtual environments. New York: Oxford University Press.
Schroeder, N. L., Adesope, O. O., and Gilbert, R. B. (2013). How effective are pedagogical agents for learning? A Meta-analytic review. J. Educ. Comput. Res. 49, 1–39. doi: 10.2190/EC.49.1.a
Schutz, P. A., and Pekrun, R. (eds.). (2007). Emotion in education. Elsevier Academic Press. Available at: https://psycnet.apa.org/record/2007-04736-000
Segaran, K., Mohamad Ali, A. Z., and Hoe, T. W. (2021). Does avatar design in educational games promote a positive emotional experience among learners? E-learning Digital Media 18, 422–440. doi: 10.1177/2042753021994337
Siegle, R. F., Schroeder, N. L., Lane, H. C., and Craig, S. D. (2023). Twenty-five years of learning with pedagogical agents: history, barriers, and opportunities. TechTrends 67, 851–864. doi: 10.1007/s11528-023-00869-3
Soliman, M., and Guetl, C. (2013). Simulating interactive learning scenarios with intelligent pedagogical agents in a virtual world through BDI-based agents. Int. J. Eng. Ped. 3:41. doi: 10.3991/ijep.v3i2.2456
Stevens, G. J., Bienz, T., Wali, N., Condie, J., and Schismenos, S. (2021). Online university education is the new normal: but is face-to-face better? Interact. Technol. Smart Educ. 18, 278–297. doi: 10.1108/ITSE-08-2020-0181
Sweller, J. (2011). “Cognitive load theory” in Cognition in education. ed. J. Mestre (Amsterdam [u.a.]: Elsevier), 37–76.
Tao, Y., Zhang, G., Zhang, D., Wang, F., Zhou, Y., and Xu, T. (2022). Exploring persona characteristics in learning: a review study of pedagogical agents. Procedia Comp. Sci. 201, 87–94. doi: 10.1016/j.procs.2022.03.014
Tien, I. S., Imundo, M. N., and Bjork, E. L. (2023). Viewing oneself during synchronous online learning increases appearance anxiety and decreases memory for lecture content. Appl. Cogn. Psychol. 37, 443–451. doi: 10.1002/acp.4048
Turk, M., Heddy, B. C., and Danielson, R. W. (2022). Teaching and social presences supporting basic needs satisfaction in online learning environments: how can presences and basic needs happily meet online? Comput. Educ. 180:104432. doi: 10.1016/j.compedu.2022.104432
Vlasenko, K. V., Volkov, S. V., Lovianova, I. V., Sitak, I. V., Chumak, O. O., and Bohdanova, N. H. (2023). Exploring usability principles for educational online courses: a case study on an open platform for online education. Educ. Technol. Q. 2023, 173–187. doi: 10.55056/etq.602
Wang, F., Li, W., Xie, H., and Liu, H. (2017). Is pedagogical agent in multimedia learning good for learning? A meta-analysis. Adv. Psychol. Sci. 25:12. doi: 10.3724/SP.J.1042.2017.00012
Wang, H., Tang, S., and Lei, CU. (2024). AI Conversational Agent Design for Supporting Learning and Well-Being of University Students. Available at: https://osf.io/preprints/edarxiv/w4rtf
Wei, H. C., and Chou, C. (2020). Online learning performance and satisfaction: do perceptions and readiness matter? Distance Educ. 41, 48–69.
Whiteside, A. L., Dikkers, A. G., and Swan, K. (2023). “Social presence in online learning” in Multiple perspectives on practice and research (Taylor & Francis). Available at: https://www.routledge.com/Social-Presence-in-Online-Learning-Multiple-Perspectives-on-Practice-and-Research/Whiteside-GarrettDikkers-Swan/p/book/9781620365090?srsltid=AfmBOooqHi9PJ_EjkOnxS_soGsjEe8pTz8yJ3TGJUmdYwTWv8_SQIoWO
Wong, R. M., and Adesope, O. O. (2021). Meta-analysis of emotional designs in multimedia learning: a replication and extension study. Educ. Psychol. Rev. 33, 357–385. doi: 10.1007/s10648-020-09545-x
Wu, R., and Yu, Z. (2022). Exploring the effects of achievement emotions on online learning outcomes: a systematic review. Front. Psychol. 13:977931. doi: 10.3389/fpsyg.2022.977931
Yilmaz, R., and Yilmaz, F. G. K. (2023). The effect of generative artificial intelligence (AI)-based tool use on students' computational thinking skills, programming self-efficacy and motivation. Comp. Educ. 4:100147. doi: 10.1016/j.caeai.2023.100147
Yin, J., Goh, T. T., and Hu, Y. (2024). Interactions with educational chatbots: the impact of induced emotions and students’ learning motivation. Int. J. Educ. Technol. High. Educ. 21:47. doi: 10.1186/s41239-024-00480-3
Yuan, Q., and Gao, Q. (2024). Being there, and being together: avatar appearance and peer interaction in VR classrooms for video-based learning. Int. J. Hum. Comp. Interact. 40, 3313–3333. doi: 10.1080/10447318.2023.2189818
Yusuf, A., and Noor, N. M. (2023). Research trends on learning computer programming with program animation: a systematic mapping study. Comput. Appl. Eng. Educ. 31, 1552–1582. doi: 10.1002/cae.22659
Keywords: pedagogical agents, avatars, teacher presence, self-other presence, learning performance, learning experience, online learning, artificial intelligence
Citation: Herbert C and Dołżycka JD (2024) Teaching online with an artificial pedagogical agent as a teacher and visual avatars for self-other representation of the learners. Effects on the learning performance and the perception and satisfaction of the learners with online learning: previous and new findings. Front. Educ. 9:1416033. doi: 10.3389/feduc.2024.1416033
Received: 11 April 2024; Accepted: 26 September 2024;
Published: 27 November 2024.
Edited by:
Halvdan Haugsbakken, Østfold University College, NorwayReviewed by:
Ramazan Yilmaz, Bartin University, TürkiyeCopyright © 2024 Herbert and Dołżycka. This is an open-access article distributed under the terms of the Creative Commons Attribution License (CC BY). The use, distribution or reproduction in other forums is permitted, provided the original author(s) and the copyright owner(s) are credited and that the original publication in this journal is cited, in accordance with accepted academic practice. No use, distribution or reproduction is permitted which does not comply with these terms.
*Correspondence: Cornelia Herbert, Y29ybmVsaWEuaGVyYmVydEB1bmktdWxtLmRl
Disclaimer: All claims expressed in this article are solely those of the authors and do not necessarily represent those of their affiliated organizations, or those of the publisher, the editors and the reviewers. Any product that may be evaluated in this article or claim that may be made by its manufacturer is not guaranteed or endorsed by the publisher.
Research integrity at Frontiers
Learn more about the work of our research integrity team to safeguard the quality of each article we publish.