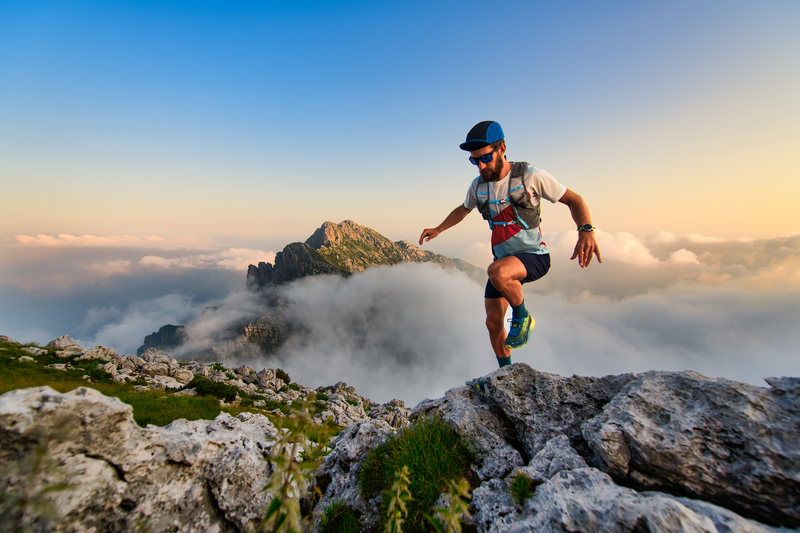
95% of researchers rate our articles as excellent or good
Learn more about the work of our research integrity team to safeguard the quality of each article we publish.
Find out more
ORIGINAL RESEARCH article
Front. Educ. , 29 August 2024
Sec. Leadership in Education
Volume 9 - 2024 | https://doi.org/10.3389/feduc.2024.1415755
This article is part of the Research Topic Continuing Engineering Education for a Sustainable Future View all 19 articles
In contemporary higher education within STEM fields, fostering and assessing sustainability competencies is essential for promoting lifelong learning with a comprehensive understanding of the relationships between innovation and environmental, social, and economic factors. However, training and grading processes for these competencies face significant challenges due to the intricate, adaptable, and multi-modal nature of current academic models. Better understandings and approaches to educating higher education STEM students in sustainability are paramount. Therefore, we have conducted a data-driven analysis on 159,482 records from 22 STEM programs at Tecnologico de Monterrey between 2019 and 2022, employing data science methodologies. These competencies align with the four dimensions of the UNESCO program “Educating for a Sustainable Future”: social, environmental, economic, and political. The study aims to identify the primary challenges faced by students in developing sustainability competencies within this flexible and multi-modal academic environment. Notably, the analysis revealed a widespread distribution of courses with sustainability competencies across all semesters and programs. By the end of the first semester, 93.5% of students had been assessed in at least one sustainability competency, increasing to 96.7% and 97.2% by the end of the second and third semesters, respectively. Furthermore, findings indicate that sustainability competencies are assessed 21 times on average by the end of the sixth semester, with varying levels of development. Interestingly, no significant differences were observed in competency development based on gender, age, or nationality. However, certain competencies such as Commitment to sustainability, Ethical and citizen commitment, and Social Intelligence posed notable challenges across programs and semesters.
Fostering and assessing sustainability competencies among Science Technology Engineering and Math (STEM) students in contemporary Higher Education (HE) is imperative. Integrating sustainability competencies into STEM HE cultivates a holistic understanding of the interconnections between innovation and environmental, societal, and economic dimensions for lifelong learning (Žalėnienė and Pereira, 2021; Håkansson Lindqvist et al., 2024). STEM students play an active role in enhancing sustainable development through current and future innovations. Hence, their sustainability competencies are critical not only for their academic success but also for their contributions to solving environmental, economic, and societal sustainability problems.
In recent years, universities and STEM programs have increasingly focused on transitioning toward a green economy and fostering sustainable innovation and research (Caeiro et al., 2020; Cihan Ozalevli, 2023). Developing value-based competencies alongside academic knowledge and technical skills is crucial for cultivating a sustainability culture among university students (Žalėnienė and Pereira, 2021). Implementing such a combination can be achieved through STEM programs designed with social responsibility, ethical leadership, integrity, critical thinking, and empathy. Many Higher Education Institution (HEI) leaders recognize the importance of their role in training and assessing sustainability competencies in both undergraduate students and lifelong learners (Redman et al., 2021).
Tecnologico de Monterrey, a Mexican private institution, is at the forefront of this educational evolution with a strong focus on social impact and sustainability. In 2019, it launched the Tec21 pedagogical model based on competencies (Olivares et al., 2021). All the academic programs have competencies by training units defined and revised by pedagogical architects and experienced professors as described by Olivares et al. (2021). This model orchestrates the training and evaluation of competencies using challenge-based learning and offers high flexibility. However, the complexities of implementing such flexible, adaptable, and multi-modal academic models present critical challenges for competency assessment and their future analysis.
HEIs that implement Competency-Based Education (CBE) typically use rubrics to evaluate students (Malhotra et al., 2023). These rubrics rely on data, documents, or objects to support students' compliance with pre-established competency criteria. The combination of these data with the evaluations assigned by professors and other anonymized sociodemographic and academic data provides a valuable source of information for identifying positive trends and opportunities for improving CBE systems. Such data contain valuable insights into the theoretical training of STEM students in sustainability competencies.
The information obtained by applying data science techniques to sustainability competency assessment data helps to bridge the gap between theoretical frameworks and practical applications in sustainability competency assessment. This approach enables educators to tailor their teaching methods and evaluation practices based on empirical data, thus enhancing the effectiveness of sustainability education (Gao et al., 2020; Burk-Rafel et al., 2023). Despite the relevance of data-driven analyses of competency assessment for improving competencies' impact on professional careers (Burk-Rafel et al., 2023; Rhoney et al., 2023; Segui and Galiana, 2023), there are insufficient studies supported by large datasets and extended periods that describe the relationships between sociodemographic, academic, and sustainability-competency evaluation variables in HE STEM students. To address this gap, we analyzed a large dataset (around 1.6 million records) with competency-based training and assessment data collected from HE STEM students between 2019 and 2022 at Tecnologico de Monterrey.
By applying descriptive and correlational analysis to the collected data, we aim to shed light on the relevance of each feature for sustainability competency assessment and the behavior of such assessments across academic periods, programs, and years. Our work seeks to answer two research questions: (1) How do sustainability competency assessments perform across semesters, years, and academic programs in the data collected? (2) What is the relationship between sociodemographic, academic, and competency variables with the evaluation of sustainability competencies in the data collected? Our findings will provide empirical insights into sustainability competencies training and evaluation in HE STEM students and highlight some risks associated with assessing sustainability competencies in any teaching and learning scenario. In summary, the main contributions of this work are as follows:
1. A characterization of the sustainability competencies training and assessment in the STEM programs at Tecnologico de Monterrey under a highly flexible pedagogical model and its relationship with sociodemographic and academic variables.
2. A discussion about some risks of sustainability competency assessment in HE STEM students based on a data science approach analyzing a large dataset collected between 2019 and 2022.
Our paper is organized as follows: In Section 2, we discuss previous work on sustainability competencies, providing a foundation for the research reported in this work. Section 3 describes the data characteristics, the tidiness process, and the methods used for analyzing competency evaluations. Section 4 presents our findings and their rationale. Finally, Section 5 concludes with findings about sustainability competencies in STEM programs, their impact on other HEIs and employers, and future work directions derived from this work.
UNESCO identifies key sustainability competencies as -system-thinking, future-thinking, value-thinking, strategic-thinking, and interpersonal competencies- (Rieckmann, 2017). Some authors have investigated the assessment methods for these competencies in HE and their alignment with UNESCO's key competencies. For example, Redman et al. (2021) conducted a systematic literature review on current practices in assessing students' sustainability competencies, proposing a typology of eight assessment tools classified into Self-perceiving, Observation, and Test-based approaches. Their review underscores the importance of pre and post-assessment during the teaching process, though it does not include empirical data analysis on large-scale datasets, limiting its findings to existing literature.
Annelin and Boström (2022) examined self-assessment tools for essential sustainability competencies, revealing confusion around scales and criteria. They proposed enhancements to current methods and emphasized the need to understand students' existing competencies. However, their study did not address professors' evaluations of these competencies or leverage extensive data to support their findings.
Lafuente-Lechuga et al. (2024) reviewed sustainability teaching in HE, particularly in the mathematical discipline in Spain, highlighting the importance of cross-disciplinary approaches and practical activities for integrating sustainability competencies into curricula. They also stressed the need for curricular changes in HE programs to include sustainability competency assessments.
Other works advocate using data mining and data science to evaluate the effectiveness of Competency-Based Education (CBE). For instance, Rhoney et al. (2023) recommended developing an active approach to collecting implementation data and investing in technology platforms for student performance data repositories to support knowledge management and data analytics in pharmacy education. Gao et al. (2020) discussed the complexity of assessing learning outcomes in STEM education and suggested using coding frameworks to analyze different assessment methods. They highlighted the importance of data science in handling large datasets and extracting insights from interdisciplinary educational approaches. Li et al. (2020) reviewed projects employing data-intensive methods to evaluate educational outcomes in STEM, underscoring the need for advanced data analytics to understand teaching methods' effectiveness and their impact on student assessments. However, these studies do not specifically address sustainability competency-based assessment in STEM programs.
While previous research has emphasized the importance of sustainability competency assessment in undergraduate STEM programs and the potential of large-scale data for insights, there is a notable gap in studies providing empirical findings from extensive datasets. This gap is particularly evident in the lack of research involving a substantial number of students, programs, and periods. Therefore, research utilizing data science techniques to analyze extensive datasets, such as the 160,000 records of HE STEM students' evaluations used in this work, is crucial. This approach can provide a deeper understanding of sustainability competencies assessment and its impact on employers and lifelong learning.
The Institute for the Future of Education of Tecnologico de Monterrey has made available anonymized data about the competency assessment of all students between 2019 and 2022. The raw data encompasses approximately 5 million records about competency assessments of 16,500 STEM students for 22 STEM programs in 100 competencies. Each record contains information about the evaluation of a competency, for a student, in a complexity level (A, B, and C), a training unit, a semester, and an academic program. The professor assigns the competency evaluation as ‘Observed' or ‘Not Observed' using the rubric defined for the competency in the training unit. Since the raw data were collected during three academic years, it has some challenges for its analysis, some variables contain string values that are difficult to analyze, many null values, and some variables have different labels for the same value, so they need to be merged. Additionally, the same student has various records for different academic programs, training units, semesters, competencies, and complexity levels. Figure 1 illustrates the data structure in the database.
Figure 1. Structure of the data in the competency assessment database. The database encompasses other sociodemographic and academic data related to the student. We have omitted them for visualization since they are the same for each student, training unit, and semester.
Subsection 3.2 describes the transformation steps to reach a tidy and reliable dataset. Such a transformation made the dataset reliable for data analysis. As a result of the transformation, we have ended with 159,482 records of the assessment of 17 sustainability competencies in 16,061 undergraduate students of 22 STEM programs between 2019 and 2022.
We have assumed two criteria to select sustainability competencies among all the competencies in the database. First, we have considered the United Nations Sustainable Development Goal SDG Target 4.7 “ensure that all learners acquire the knowledge and skills needed to promote sustainable development, including, among others, through education for sustainable development and sustainable lifestyles, human rights, gender equality, promotion of a culture of peace and non-violence, global citizenship and appreciation of cultural diversity and of culture's contribution to sustainable development” (United, 2023). United Nations SDG Target 4.7 establishes as its second indicator that “education for sustainable development be mainstreamed in national education policies, curricula, teacher education, and student assessment (UNESCO, 2020). Second, we have considered the consensus about five key sustainability competencies (system-thinking, future-thinking, value-thinking, strategic-thinking, and interpersonal competencies) (Rieckmann, 2017; Redman et al., 2021). Starting from these criteria we have filtered the competency assessment records with a semiautomatic process using keywords obtained from the UN SDG Target 4.7 and the competencies descriptions of the academic programs as shown in Figure 2. After filtering, we have manually checked the pertinence of each selected competency to the sustainability framework defined by UNESCO and the five key sustainability competencies assumed by the research community (Rieckmann, 2017; Redman et al., 2021).
Figure 2. Semi-automatic filtering process for selecting sustainability competencies according to the United Nations Sustainability Development Goal 4 Target 4.7.
With the pedagogical model Tec21, all students are evaluated at each complexity level as competency ‘Observed' or ‘Not observed' in different training units, depending on the pedagogical design of the program and training units (Olivares et al., 2021). Besides, Tec21 offers entrance academic programs for students undecided about the specific program they want to study. Such entrance programs are grouped by knowledge area and offer common core training units. Students can enroll in an entrance or specific program depending on whether they are decided about their career (Olivares et al., 2021). Figure 3 illustrates the relationship between training units and sustainability competencies for the students during the first semester of the program Innovation and Transformation (IIT), which is an entrance program for several Engineering programs.
Figure 3. Bipartite graph illustrating the relationship between training units and sustainability competencies for the students in the first semester of the program Innovation and Transformation.
A link between columns indicates that at least one student enrolled in the IIT program has completed the training unit on the left in the first semester. Consequently, the student has been trained and assessed in the corresponding sustainability competencies, linked in the right column. These training units evaluate other competencies not directly related to sustainability and therefore were excluded from this work. Nonetheless, the graph illustrates the complexity of the competency training and assessment with many training units evaluating the same sustainability competency even two sustainability competencies in the same program and semester.
The graph encompasses data from cohorts 2019, 2020, and 2021. It shows the flexibility of the pedagogical model Tec21 with 32 training units taken by students of different cohorts during their first semester of the IIT program. Simultaneously, the graph depicts multiple training units where students are assessed through how far they have one or more sustainability competencies. As a result, all students are graded on the same sustainability competency multiple times within various knowledge areas fostering sustainability for their lifelong learning in any scenario.
We have developed a six-step procedure based on the CRISP-DM methodology for data analysis (Wirth and Hipp, 2000). Step 1, dedicated to management and ethics, has been carried out throughout our research work. In step 2, we conducted a thorough analysis toward the understanding of sustainability competencies and the criteria used at Tec de Monterrey for their assessment. Having done so, we proceeded with data comprehension in step 3. Following this, we modified the database to obtain a tidy dataset through step 4. Next, in step 5, we applied feature selection algorithms, and feature engineering to identify worthy features dropping the unnecessary ones. In step 6, we have analyzed the relationships between academic, sociodemographic, and competency data for sustainability competencies.
The database published by Tec de Monterrey has 45 features. It is delivered as a collection of text files separated by academic programs due to their large size. We grouped the database features into three categories: sociodemographic, academics, and competencies. Table A1 in the Appendix gives an overview of those features, according to their category. In the initial phase of data processing, the focus lies on loading datasets and transforming feature types to ensure consistency and usability. The following steps are undertaken:
1. All databases are consolidated into a single dataframe to facilitate unified analysis. Certain columns (see Table A1 columns 36, 37, 38, 41, 42, and 43 in the Appendix) presented problematic data types due to the combination of records with strings, numeric, or missing values in different text files. Thus, we converted them into appropriate types, whether string or numeric. Also, we parsed Datetime data, originally formatted as ‘%d-%m-%Y' to the ‘%d/%m/%Y' format for standardization.
2. Next, we analyzed and fixed some string columns. We corrected some erroneous competency names according to their specific competency names. We used a semiautomatic approach, for some competency names we employed the pattern [A-Z]3[0-9]4[A-Z]_, but for others like ‘Scientific thought' and ‘Pensamiento científico' we manually unified them as ‘Scientific thinking'. We removed leading and trailing white spaces in competency descriptions. Besides, we renamed the column 'Pais de nacimiento' as ‘student.nationality'.
3. Once all the data were in a dataset and string data formatted, we analyzed the missing values and the feature unique values. We dropped those rows with missing values in the features “competence.desc”, and “competency.level_assigned” because we do not have sufficient information to impute them and they are key for the competency analysis. Rows with unpublished activity status were also removed from the dataset because students never observed them. We dropped various schedule-related features because they had many missing values. The column “group.isAcademicSupport” is removed due to containing only 0 values.
Feature engineering involves transforming, generating, extracting, evaluating, analyzing, and selecting features (Dong and Liu, 2018; De Armas Jacomino et al., 2021). In our data analysis problem, we employed some of these tasks to delve into the data relationships and possible competency assessment explanations. Hence, we modified some features and added others based on the existing information in the dataset.
First, we transformed binary features into boolean features for computational efficiency. For example, the feature ‘student.gender_desc' was transformed into a boolean feature “student.isWoman”. Similarly, Features “student_originSchool.isITESM”, “student.isForeign”, “group.isEnglishLanguage”, “group.hasEvaluationInst”, and ‘student.isConditioned' were converted to boolean features. We transformed the features indicating the number of evidence and activities into two new features counting the number of evidence and activities. However, a posterior analysis showed that these variables were equal to zero for all academic periods except ‘2022FJ', hence, we removed these columns. Moreover, we transformed to boolean the “competency.level_assigned”, originally denoting “Observed” or “Not observed” competency assessment as strings.
We added a feature indicating the competency code, including the “competency.level_required” (A, B, and C). This added feature facilitates identifying equivalent competencies. With such a new feature, we standardized equivalent competencies across different programs. We included new features denoting enrollment period, periods since enrollment, the number of training units enrolled, and a feature distinguishing between entrance or specific programs. Also, we incorporated two features representing the count of “Observed” and not “Not observed” assessments of the competency in previous semesters. Other four features were added for counting the number of competencies and competencies evaluated in the current training unit (“competencies_evaluated.count”, “competencies_evaluated.count”), and the number of training units that evaluate the current competency and competency (“training unit_evaluating.count”, “training unit_evaluating_competency.count”). Such new features facilitate further analysis of the sustainability competency assessment.
We modified the competency categories from Transversal or Disciplinary to General education, Area, or Disciplinary to include the subdivision in the Disciplinary category as a new category. We modified the feature “group.id” to ‘group.size' for a comprehensive analysis.
To reach a tidy dataset, we removed duplicated rows based on the uniqueness of the following features:
student.id term_period.id
training unit.longName training unit.longName
training unit.longName competency.level_required
competency.level_assigned
This gives us a unique competency assessment for every student and training unit in a semester, a competency, at a required level.
As a result, we obtained a dataset with 159, 482 records detailing the assessments of 17 sustainability competencies in 16, 061 undergraduate students of 22 STEM programs between 2019 and 2022. The final feature set is described in Table 1.
The academic programs at Tecnologico de Monterrey are flexible and multi-modal. Thus, students can take different training units in different semesters and modes: face-to-face, remote, or hybrid. We have performed descriptive and correlational analyses of the sustainability competencies among all the School of Engineering and Science academic programs. Our results are described below.
Every academic program has at least one training unit in each academic term where students are evaluated in terms of the level of development of sustainability competency. This distribution supports the continued preparation of the students in a growing sense of belonging to sustainability. Table 2 lists the competency and training unit count by the academic program. It illustrates similar counts among all programs.
Figure 4 graphs the student count by the number of sustainability competencies assessed and semester. Unsurprisingly, the highest numbers of students with one sustainability competency evaluated are reported during the first and second semesters. Nevertheless, the graphs show that more than 3, 000 students have been graded in only one sustainability competency even for the later semesters. The pedagogical model flexibility causes such differences. Simultaneously, such flexibility facilitates some students to evaluate four sustainability competencies in the first and second semesters. This is the maximum number of competencies reported for semesters 1 and 2, while five is the maximum for semesters 3 and 4 and seven for semesters 5 and 6.
From our analysis of student data by semester and the number of competencies assessed, we found that 93.5% of students were evaluated in at least one sustainability competency by the end of their first semester, 96.7% by the end of their second semester, and 97.2% by the end of their third semester. By the sixth semester, students had been trained and graded an average of 21 times in various sustainability competencies, covering all levels. These findings highlight the feasibility of implementing competency-based programs that incorporate multiple sustainability competencies early in the academic journey of STEM students. Furthermore, this data is valuable for the corporate sector, providing insight into the sustainability skills and capabilities of graduates, which are essential for driving sustainable transformation within their organizations.
The pedagogical model Tec21 guarantees that most STEM students are trained and evaluated in multiple sustainability competencies in a growing and diverse way using three levels. However, we have found room for improvement regarding the competency assessment. Various students previously evaluated as “Not observed” in a sustainability competency are assessed as “Not observed” when they re-evaluate the same competency. Consequently, those students continue with deficient competency development, possibly affecting their role in the labor scenario. Table 3 summarizes the number of students and the sustainability competencies being re-evaluated as “Not observed” by semester.
The more common sustainability competencies among those re-evaluated as “Not observed” are -Commitment to sustainability, Ethical and citizen commitment, and Application of international standards-. Special attention should be given to these sustainability competencies during training and assessment. “Ethical and citizen commitment” was trained by 80 training units in all semesters, across all 22 academic programs, and evaluated by 11,381 students (70.8% of the total students). Similarly, “Commitment to sustainability” was trained by 43 training units in all semesters, across all 22 academic programs, and evaluated by 15,837 students (96%). “Application of international standards” was trained by 9 training units, during all semesters, but in 14 academic programs (63.6%), and evaluated by 3,392 students (20.55%). These sustainability competencies represent challenges for the STEM students and the corporate sector receiving these students. Two key points to be considered before ending the academic programs or during the lifelong learning are sharing previous results of the students with the new professors or pre-evaluating the competencies at the beginning of the training unit to create the necessary strategies with the students previously assessed as ‘Not observed'. Besides, the corporate sector could consider applying training strategies for these challenging sustainability competencies.
We have evaluated the correlation of some socio-demographic and academic features with the target feature, which is the competency development level assigned by the instructor. We aim to determine if the competency assessment is determined by some other features. We have split our experiments according to the feature domains in categorical and boolean, and numeric and boolean.
We have noticed no differences regarding gender, region, or nationality in developing sustainability competencies. Figures 5, 6, 7 depict the Cramér's V and p-val for the correlation between each nominal variable and the competency evaluation variable, which takes dichotomous values -“Observed” or “Not observed”-. All Cramér's V values indicate very low correlation between the independent variable and the competency evaluation.
Violin plots in Figure 8 demonstrate no differences between male and female students in terms of sustainability competency evaluations and the numerical grades reported in the training unit. Both categories show similar distribution shapes, means, and interquartile ranges across genders, indicating that there is no disparity between male and female students in these assessments. This finding is significant for employers, as it suggests that there should be no gender bias in hiring decisions based on sustainability competency training and evaluation results because both male and female students exhibit comparable performance levels.
Figure 8. Violin plots by student's gender representing the boxplots and the distribution shape of the numerical grades in the training units and separated by competency evaluations “Observed” and “Not observed”.
The heatmap in Figure 9 depicts Pearson's correlation coefficients between the numerical features. Three attributes, namely “student_grades.final_numeric_afterAdjustment”, “student.lastTerm_gpa”, “student.term_gpa_program”, show a moderate positive correlation to the target feature. It means that the numerical qualifications impact the sustainability competency assessment and the competency assessments also affect the student's grades. Besides, the feature “competence.notobserved_count” has a moderate inverse correlation with the target feature. Such a result corroborates the previous observation about assigning the same evaluation of “Not observed” when re-evaluating a competency.
Our analysis reveals that students in the School of Engineering and Science at Tecnologico de Monterrey receive extensive training and assessment in sustainability competencies from the onset of their academic programs. By the end of their sixth semester, students are evaluated an average of 21 times on these competencies. Our findings indicate that the assessment of these competencies is unbiased concerning gender, age, or nationality, promoting social justice within the student body.
We observed a moderate correlation between competency assessments and both training unit grades and overall grade point average (GPA). This suggests that faculty members consider a range of factors beyond numerical scores when evaluating sustainability competencies. To further enhance competency development, it is recommended that professors employ targeted strategies to assist students in improving competencies initially marked as ‘Not observed'. Given the tendency for such initial evaluations to persist, providing additional resources and tailored guidance can effectively support students' progress in sustainability competencies.
These findings are relevant for other higher education institutions aiming to integrate sustainability competencies into their curricula. The results illustrate that a structured and frequent assessment of sustainability competencies in STEM can be implemented without depending on the sociodemographic characteristics of the students, fostering an equitable educational environment. They also illustrate a risk of failing the competency training in competencies previously evaluated as failed, with particular emphasis on “The application of international standards”, “Commitment to sustainability”, and “Ethical and citizen commitment”.
Findings are also worthy for the corporate sector because graduates with robust training in sustainability competencies are increasingly valuable as companies pivot toward more sustainable practices. The ability to assess and ensure these competencies in graduates means that businesses can rely on new hires to contribute meaningfully to sustainability goals from day one.
Future work will involve the use of additional instruments with the employers to evaluate the results of the sustainability competency training and assessment on the STEM students after going to the corporate sector. Also, we will increase the number of academic variables collected, such as evaluation activities, platforms, and rubrics to dig into the impact of such new variables in the sustainability competency assessment.
The data that support the findings of this study are available from the Institute for the Future of Education (IFE)'s Educational Innovation collection of the Tecnológico de Monterrey's Research Data Hub but restrictions apply to the availability of this data, which was used under a signed Terms of Use document for the current study, and so are not publicly available. Data are however available from the IFE Data Hub upon reasonable request at: https://doi.org/10.57687/FK2/R9LOXP.
DV-R: Conceptualization, Data curation, Formal analysis, Software, Visualization, Writing – original draft, Writing – review & editing. LA: Investigation, Validation, Writing – original draft, Writing – review & editing. RM: Conceptualization, Formal analysis, Methodology, Writing – original draft, Writing – review & editing. GZ: Formal analysis, Funding acquisition, Writing – original draft, Writing – review & editing.
The author(s) declare financial support was received for the research, authorship, and/or publication of this article. We have received funding for the APC from the Writing Lab of the Institute for the Future of Education at Tecnologico de Monterrey. Writing Lab is an initiative within the Institute for the Future of Education that is dedicated to the development of the culture of research in educational innovation and the improvement of the academic production of the teaching community at the Tecnológico de Monterrey.
The authors would like to thank the Datahub department at the Institute for the Future of Education of Tecnologico de Monterrey for providing the data. Also, we thank the Writing Lab at the Institute for the Future of Education of Tecnologico de Monterrey for their valuable contribution to this work.
The authors declare that the research was conducted in the absence of any commercial or financial relationships that could be construed as a potential conflict of interest.
All claims expressed in this article are solely those of the authors and do not necessarily represent those of their affiliated organizations, or those of the publisher, the editors and the reviewers. Any product that may be evaluated in this article, or claim that may be made by its manufacturer, is not guaranteed or endorsed by the publisher.
Annelin, A., and Boström, G.-O. (2022). An assessment of key sustainability competencies: a review of scales and propositions for validation. Int. J. Sustain. Higher Educ. 24, 53–69. doi: 10.1108/IJSHE-05-2022-0166
Burk-Rafel, J., Reinstein, I., and Park, Y. S. (2023). Identifying meaningful patterns of internal medicine clerkship grading distributions: application of data science techniques across 135 U.S. medical schools. Acad. Med. 98, 337–341. doi: 10.1097/ACM.0000000000005044
Caeiro, S., Sandoval Hamón, L. A., Martins, R., and Bayas Aldaz, C. E. (2020). Sustainability assessment and benchmarking in higher education institutions—a critical reflection. Sustainability 12:543. doi: 10.3390/su12020543
Cihan Ozalevli, C. (2023). Why Sustainability Must Become an Integral Part of STEM Education? Cologny: World Economic Forum.
De Armas Jacomino, L., Medina-Pérez, M. A., Monroy, R., Valdes-Ramirez, D., Morell-Pérez, C., and Bello, R. (2021). Dwell time estimation of import containers as an ordinal regression problem. Appl. Sci. 11:9380. doi: 10.3390/app11209380
Dong, G., and Liu, H. (2018). Feature Engineering for Machine Learning and Data Analytics. Boca Raton, FL: CRC Press.
Gao, X., Li, P., Shen, J., and Sun, H. (2020). Reviewing assessment of student learning in interdisciplinary stem education. Int. J. STEM Educ. 7:24. doi: 10.1186/s40594-020-00225-4
Håkansson Lindqvist, M., Mozelius, P., Jaldemark, J., and Cleveland Innes, M. (2024). Higher education transformation towards lifelong learning in a digital era - a scoping literature review. Int. J. Lifelong Educ. 43, 24–38. doi: 10.1080/02601370.2023.2279047
Lafuente-Lechuga, M., Cifuentes-Faura, J., and Faura-Martínez, Ú. (2024). Teaching sustainability in higher education by integrating mathematical concepts. Int. J. Sustain. Higher Educ. 25, 62–77. doi: 10.1108/IJSHE-07-2022-0221
Li, Y., Wang, K., Xiao, Y., Froyd, J. E., and Nite, S. B. (2020). Research and trends in stem education: a systematic analysis of publicly funded projects. Int. J. STEM Educ. 7:17. doi: 10.1186/s40594-020-00213-8
Malhotra, R., Massoudi, M., and Jindal, R. (2023). Shifting from traditional engineering education towards competency-based approach: The most recommended approach-review. Educ Inf Technol. 28, 9081–9111. doi: 10.1007/s10639-022-11568-6
Olivares, S. L. O., Islas, J. R. L., Garín, M. J. P., Chapa, J. A. R., Hernández, C. H. A., and Ortega, L. O. P. (2021). Tec21 Educational Model: Challenges for a Transformative Experience. Monterrey: Editorial Digital del Tecnológico de Monterrey.
Redman, A., Wiek, A., and Barth, M. (2021). Current practice of assessing students' sustainability competencies: a review of tools. Sustain. Sci. 16, 117–135. doi: 10.1007/s11625-020-00855-1
Rhoney, D. H., Chen, A. M., Churchwell, M. D., Daugherty, K. K., Jarrett, J. B., Kleppinger, E. L., et al. (2023). Recommendations and next steps for competency-based pharmacy education. Am. J. Pharm. Educ. 87:100549. doi: 10.1016/j.ajpe.2023.100549
Rieckmann, M. (2017). Education for Sustainable Development Goals: Learning Objectives. Paris: UNESCO Publishing.
Segui, L., and Galiana, M. (2023). The challenge of developing and assessing transversal competences in higher education engineering courses. Int. J. Eng. Educ. 39, 1–12. Available at: https://www.ijee.ie/1atestissues/Vol39-1/02_ijee4287.pdf
United Nation (2023). Sustainable Development Goal 4: Ensure Inclusive and Equitable Quality Education and Promote Lifelong Learning Opportunities for All. Technical Report. Department of Economic and Social Affairs, New York, NY, United States.
Wirth, R., and Hipp, J. (2000). “Crisp-dm: towards a standard process model for data mining,” in Proceedings of the 4th International Conference on the Practical Applications of Knowledge Discovery and Data Mining (Manchester: AAAI Press), 29–39.
Žalėnienė, I., and Pereira, P. (2021). Higher education for sustainability: a global perspective. Geogr. Sustain. 2, 99–106. doi: 10.1016/j.geosus.2021.05.001
Keywords: sustainability competencies, data science competency analysis, higher education, competency assessment analysis, competencies for lifelong learning
Citation: Valdes-Ramirez D, de Armas Jacomino L, Monroy R and Zavala G (2024) Assessing sustainability competencies in contemporary STEM higher education: a data-driven analysis at Tecnologico de Monterrey. Front. Educ. 9:1415755. doi: 10.3389/feduc.2024.1415755
Received: 11 April 2024; Accepted: 05 August 2024;
Published: 29 August 2024.
Edited by:
Kiriaki M. Keramitsoglou, Democritus University of Thrace, GreeceReviewed by:
Laura-Diana Radu, Alexandru Ioan Cuza University, RomaniaCopyright © 2024 Valdes-Ramirez, de Armas Jacomino, Monroy and Zavala. This is an open-access article distributed under the terms of the Creative Commons Attribution License (CC BY). The use, distribution or reproduction in other forums is permitted, provided the original author(s) and the copyright owner(s) are credited and that the original publication in this journal is cited, in accordance with accepted academic practice. No use, distribution or reproduction is permitted which does not comply with these terms.
*Correspondence: Genaro Zavala, Z2VuYXJvLnphdmFsYUB0ZWMubXg=
Disclaimer: All claims expressed in this article are solely those of the authors and do not necessarily represent those of their affiliated organizations, or those of the publisher, the editors and the reviewers. Any product that may be evaluated in this article or claim that may be made by its manufacturer is not guaranteed or endorsed by the publisher.
Research integrity at Frontiers
Learn more about the work of our research integrity team to safeguard the quality of each article we publish.