- 1PhD program in Teaching and Learning, Faculty of Humanities Educational Sciences, Nablus, Palestine
- 2Faculty of Humanities and Educational Sciences, An Najah National University, Nablus, Palestine
This study aims to identify the level of attitudes of faculty members in Palestinian universities regarding the opportunities and challenges of employing artificial intelligence applications in higher education. The researchers used a descriptive approach, and the study’s sample consisted of (130) faculty members at An-Najah National University. Data was collected using two specific questionnaires, one focused on opportunities and the other on challenges. Data analysis was conducted using statistical tests, specifically calculating means and standard deviations, Independent Samples Test, Mann–Whitney test, One Way ANOVA, and Kruskal-Walli’s test. The study’s results indicated that the average level of attitudes among faculty members regarding the opportunities and challenges of employing artificial intelligence applications in higher education was high. Furthermore, the results revealed no statistically significant differences in all areas of opportunities and challenges related to gender, except in “supporting learning and teaching processes,” which favored males. The results indicated no statistically significant differences in all areas of opportunities and challenges related to the educational qualifications, except for the “Benefits of AI applications in teaching and education,” in favor of an associate professor. The results also indicated no statistically significant differences in the opportunities and challenges of employing artificial intelligence applications attributed to variables of years of experience and the college. Based on this, the study recommends the necessity of implementing intensive training programs for university faculty members to enhance their skills in using artificial intelligence applications in higher education, as well as addressing the concerns and risks that hinder the adoption of these applications. Additionally, conducting experimental research to explore the integration of artificial intelligence applications in education and evaluate their effectiveness is essential.
Highlights
• Teachers have positive attitudes to use Gen AI in teaching.
• Trust and continuance upgrading as well as rapid development were the main challenges of using Gen AI.
1 Introduction
Higher education is undergoing rapid and sustained development, marked by a transformative shift in knowledge transfer, educational delivery, and skill acquisition (Garad and Al-Ansi, 2023). This swift progress is largely due to technological advancements and their broad adoption, which ties the future of higher education closely to the evolution of new technologies and the computing capabilities of advanced smart machines (Al-Ansi et al., 2021; Khlaif and Farid, 2018). Within this framework, advancements in artificial intelligence present new opportunities and challenges for higher education, with the potential to significantly alter the management and internal structures of higher education institutions.
Artificial intelligence (AI) technology has garnered significant attention in higher education. Ghalayini and Smith (2023) emphasizes the importance of AI in enhancing learning outcomes, providing comprehensive education for all students, and supporting personalized and adaptive learning, grading, and feedback. AI also offers valuable insights into student behavior and engagement, aiding in sound decision-making. In contemporary society, AI applications facilitate numerous aspects of higher education for both teachers and students by providing virtual classrooms and fostering the creation of more flexible, efficient educational solutions (Al-Ansi et al., 2021). In some cases, AI can even act as a learning companion, assisting students in their educational journey (Avery et al., 2023; Jain and Jain, 2019).
AI applications also address challenges related to access to higher education for large student populations and provide systems for monitoring student behavior, such as the Smart Classroom Behavior Management System and Smart Campus, displaying this information on a teacher’s dashboard (Zawacki-Richter et al., 2019). According to UNESCO, AI has the potential to tackle major educational challenges, innovate teaching and learning practices, and accelerate progress toward the fourth goal of the Sustainable Development Goals, as well as all other Sustainable Development Goals (Pedró, 2020).
Numerous studies have underscored the significance of employing artificial intelligence (AI) applications in higher education, as well as the challenges associated with their use. Chatterjee and Bhattacharjee (2020) investigated how policymakers in Indian universities can adopt AI in higher education. Their study highlighted the importance of AI applications amid higher education inflation and identified the perceived risks of using AI technology. Zawacki-Richter et al. (2019) conducted a literature review outlining the wide range of possibilities AI offers in higher education, benefiting students, faculty, and administrators, while also pointing out potential risks associated with AI implementation.
Huang et al. (2021) examined the positive impact of AI applications, such as adaptive learning, teaching assessment, and virtual classrooms, on enhancing teaching quality for faculty and student learning outcomes. They also discussed future challenges that AI in education might face. Kayyali (2024) focused on employing AI applications in higher education by reviewing AI concepts, characteristics, benefits, and the major challenges of implementing AI in this field.
Iqbal et al. (2022) conducted a study examining faculty attitudes toward the adoption of ChatGPT at a private university in Pakistan. The findings reveal a prevalent negative disposition among university faculty toward ChatGPT usage, primarily attributed to apprehensions regarding academic integrity issues such as cheating and plagiarism. Despite these concerns, the study identifies potential benefits including enhanced ease in lesson planning and assessment processes. In contrast, Rahiman and Kodikal (2024) investigated faculty members’ awareness of the potential application and adoption of artificial intelligence (AI). Their study aimed to explore how AI enhances the learning experience and influences faculty engagement in higher education. Moreover, the current study aims to assess the attitudes of faculty members in Palestinian universities toward AI applications in higher education, utilizing factor analysis to examine both opportunities and challenges associated with AI integration.
1.1 Study problem
Artificial intelligence is one of the most prominent topics that most researchers are currently researching globally; to contribute to the development of this field, and to provide good and useful contributions. Higher education, in light of the development of artificial intelligence applications, is an important subject that requires serious thought and improvements, as universities deal with a digitally immersed generation, and therefore need to keep up with rapid technological changes and find ways to adapt and stay connected to the digital age.
The significance of this study lies in its contribution to advancing higher education. The integration of smart applications and artificial intelligence (AI) in higher education is essential to keeping pace with ongoing changes and enhancing educational processes. By examining faculty members’ beliefs about AI applications, this study identifies ways to improve educational quality and effectiveness. Additionally, it highlights the challenges and obstacles faculty may encounter when adopting new technologies and offers recommendations to address these issues.
Moreover, the findings can inform policies and strategies in Palestinian higher education, optimizing the benefits of AI technology. This research also provides valuable insights for guiding scientific inquiry into the use of smart applications and AI in education, fostering the development of knowledge and technologies in this area. In addition, it serves as a crucial tool for researchers to understand faculty attitudes toward AI applications in higher education. The importance of this study is underscored by its focus on a critical and timely topic that is relevant to universities worldwide.
To achieve the purpose of this study, two research questions were guided it:
1. What is the level of beliefs of faculty members at An-Najah National University regarding the employment of artificial intelligence applications in higher education?
2. What is the level of beliefs of faculty members at An-Najah National University regarding the obstacles to employing artificial intelligence applications in higher education?
2 Theoretical framework
Artificial Intelligence (AI) comprises a set of technologies and tools that enable computer systems to perform tasks intelligently, akin to human thinking. This is achieved by the system learning from its input data and proactively modifying itself. This means that the computer system is capable of learning how to make smart decisions through processing and analyzing the input information. It identifies preferred and acceptable patterns for this information and data, using this learning to modify its behavior and make appropriate decisions automatically, without direct human intervention. This leads to simplification and acceleration of processes and enhances their effectiveness (Cioffi et al., 2020).
2.1 Applications of artificial intelligence in higher education
Artificial intelligence (AI) is utilized in various areas of higher education, including language learning, engineering, mathematics, and medical education (Garad et al., 2022). It plays a significant role in providing educational materials and solutions to students, as well as offering personalized education (Garad et al., 2022). According to a review of AI in higher education from 2016 to 2022 (Crompton and Burke, 2023; Chaudhry and Kazim, 2022; Bates et al., 2020; Huang et al., 2023), there are five main applications of AI in this sector. Pisica et al. (2023) highlight that AI applications offer various educational resources, such as translation tools, augmented and virtual reality in education, customized lesson programs, learning games, and voice assistants that respond to questions on various educational topics and lessons. These tools can also teach correct pronunciation and facilitate foreign language conversations, improving educational performance and enhancing students’ abilities and skills. These resources can be tailored to meet individual students’ needs and abilities, providing personalized learning experiences (Khlaif et al., 2023).
Kuleto et al. (2021) notes that faculty members often face a significant workload with time-consuming tasks like assessing tests, providing feedback, and assigning collaborative groups for projects, which leaves them with little time for activities that contribute to knowledge acquisition and skills development. AI tools, such as Feedback Fruits Automated Feedback, offer immediate feedback on grammar, spelling, citation, and content structure, encouraging students to make necessary improvements. This allows teachers to focus on more critical aspects of writing, such as thinking and reasoning.
AI can also assist in curriculum development by creating smart study content, examples, lesson plans, presentations, assignments, assessment models, and more. For instance, you can ask ChatGPT to develop a lesson plan based on specific learning objectives or provide numerous examples to help explain study concepts to students. Additionally, many AI tools can aid in building or editing multimedia study content, including images, videos, and documents (Bajahzar, 2024).
2.2 Applications of AI and administrative efficiency
AI applications can increase administrative efficiency. Pisica et al. (2023) believe they can be used to analyze data, identify key trends and patterns in public administration, and generate important suggestions that can be used in making crucial decisions. For instance, AI applications can assist in conducting personal interviews for university admissions and selecting suitable students. They can be used to provide accurate predictions related to future student needs and guide financial investments more efficiently. The use of AI in public administration can lead to increased efficiency, improved productivity, and reduced labor costs. Crompton and Burke (2023) see that AI can adjust education programs according to ongoing changes in educational conditions, such as local and international circumstances, or individual and collective educational needs of students and communities. Administrative efficiency can be improved through content customization and developing e-learning platforms using AI technologies (Chatterjee and Bhattacharjee, 2020).
2.3 Challenges of employing artificial intelligence applications in higher education
The lack of sufficient, appropriate educational data for training machine learning models significantly hampers the system’s ability to effectively guide learning. Additionally, the absence of suitable data structures or proper organization further exacerbates this issue, as data must be well-organized to be effectively utilized. Presenting educational content in a way that is easy for students to understand and absorb also presents challenges (Felder and Brent, 2024). Other issues include the incompatibility of traditional systems and technologies with machine learning applications, necessitating changes in the technological infrastructure of education and potentially reducing human control in traditional processes. Integrating machine learning into educational institutions requires changes in procedures and teaching methods to ensure better integration (Cioffi et al., 2020).
Moreover, organizational shortcomings within institutions hinder the adoption of artificial intelligence technology due to a lack of sufficient technical expertise. Training staff requires adequate training and support to develop their skills in using AI applications. Financial constraints, such as difficulties in securing sufficient funding for the adoption and maintenance of AI technology, also pose significant challenges. Effective data management and integration are crucial but often difficult, preventing the full realization of AI’s benefits. Additionally, there is insufficient support from institutional leaders. Concerns about student privacy, algorithmic bias, or discrimination toward specific groups, and the overall impact on the quality of education are also prevalent. Overreliance on technology, including AI, could lead to job losses, particularly in teaching positions. A survey by EDUCAUS revealed that colleges and universities remain unconvinced that the benefits of AI outweigh these concerns (Owoc et al., 2019; George and Wooden, 2023).
Another significant challenge is that AI-based systems may not be accessible to all students, potentially increasing existing inequalities. Persuading all stakeholders in higher education, including faculty members and administrative staff, to adopt these applications is another hurdle (Chatterjee and Bhattacharjee, 2020). Academics also fear that students might use AI to cheat, as AI-generated essays can be nearly indistinguishable from human-written ones. There is also anxiety about AI altering the teacher-student relationship and fundamentally changing the educational landscape. However, this potential change represents an opportunity for growth and necessary redirection in the teaching profession (Hashmi and Bal, 2024).
3 Methodology
The research relies on the descriptive analytical method, which studies a particular phenomenon by relying on an accurate description of the phenomenon as it is in reality. Then analyzing and interpreting this phenomenon with the aim of understanding it more deeply and deducing the factors and details influencing it. This aims to reach truths that help in improving and developing existing conditions and enhancing them.
3.1 Study population and sample
The population of this study consists of faculty members at An-Najah National University. The researchers selected a sample consisting of (130) members, using random sampling. The study tool was distributed to the study sample through an electronic link via Google Drive, where we received 110 responses through the Google Drive platform, and (20) paper responses by distributing them to faculty members in various colleges and departments. Their data came in Table 1.
3.2 Study tool
To achieve the desired objectives of the study, the researchers adopted a questionnaire as a tool for collecting information. After reviewing the previous theoretical literature, they constructed two questionnaires: one on the opportunities offered by artificial intelligence applications in higher education, consisting of fifteen items in its final form, and another on the challenges faced in employing artificial intelligence applications in higher education, consisting of eleven items according to the five-point Likert scale.
3.3 Discriminant validity
The questionnaire construction procedures were carried out after reviewing theoretical literatures and scientific researches related to the foundations of building the Likert scale. The procedures were as follows:
Formulating a large set of items, ranging in number (93, of which 36 items measure the challenges of employing artificial intelligence applications in higher education, and 57 items measure the opportunities offered by artificial intelligence applications in higher education). The items were randomly distributed on the arbitration questionnaire model1 before distribution.
The preliminary version was distributed to (20) faculty members at An-Najah National University by purposive sampling. They were individually asked to classify each item in terms of its preference intensity or non-preference for the characteristic to be measured, using a standard consisting of 5 equal categories, where category 1 indicates the highest degree of non-preference, and category 5 indicates the highest degree of preference, while category 3 represents the midpoint and indicates neutrality.
Pearson correlation coefficient was calculated for each item in relation to the overall characteristic, which was calculated using SPSS 26 program, selecting items that had a correlation coefficient greater than (0.5) with the overall characteristic (see footnote 1). Through this, items were filtered to become (37 items: 21 opportunities, 16 challenges).
After filtering the items in the previous step, another filtering was performed by extracting the values of the first and third quartiles, and classifying the responses into two groups. The first indicates responses equal to or less than the first quartile (Q1) value, and the other indicates responses equal to or more than the third quartile (Q3) value. Then, a t-test for two independent samples was used with each item separately. The results were as in the Table 2.
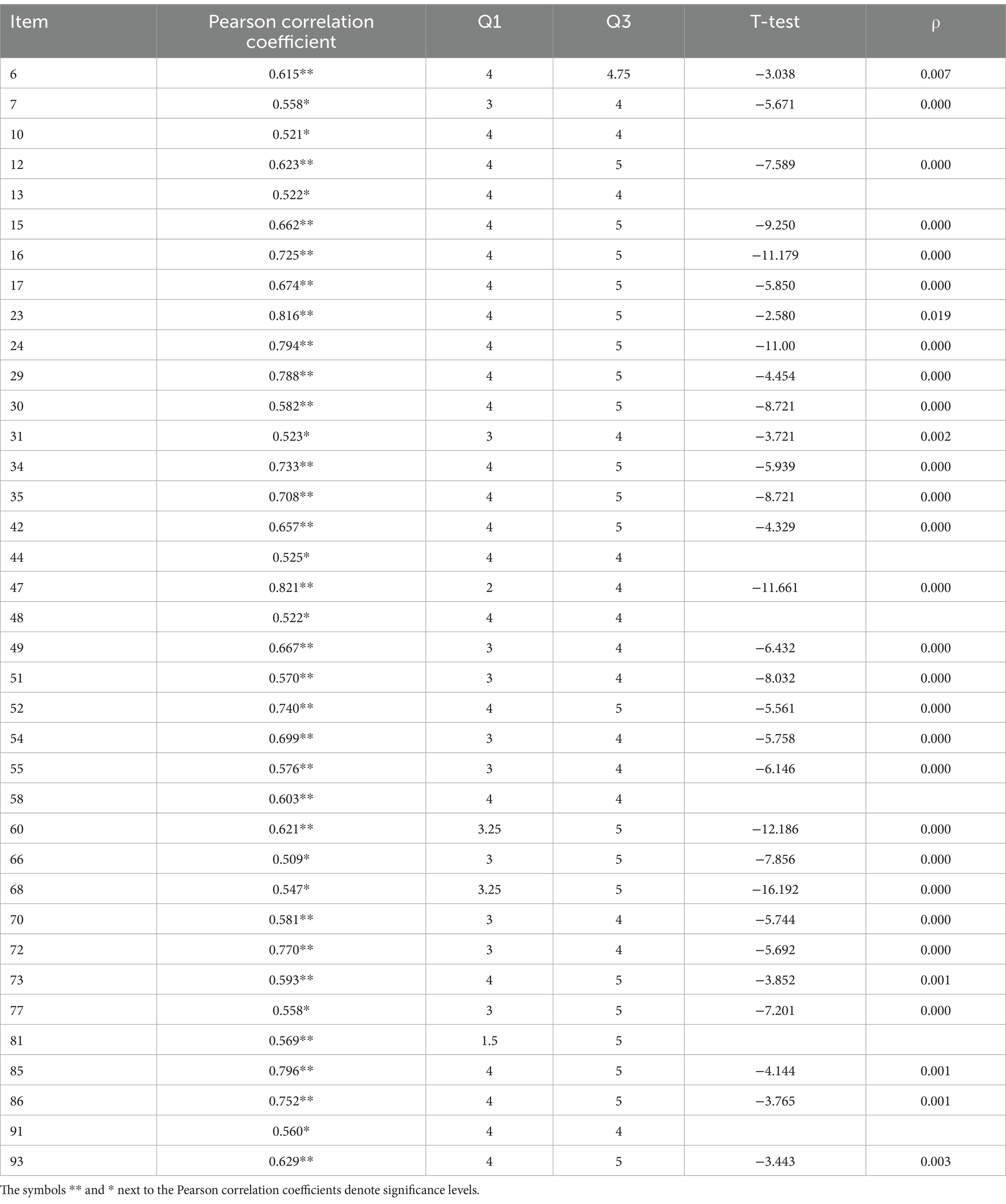
Table 2. Correlation degree of items in the scale and the values of the first and third quartiles, T-test, and ρ.
From the previous table, it is clear from the previous table that the items (10, 13, 44, 48, 58, 91) had values where (Q3 = Q1), which means that the responses are concentrated at a specific point, and therefore they cannot be distinguished between. As a result, these items were deleted. As for item (81), the standard deviation for both groups was zero, therefore making it impossible to conduct a T-test, and it was excluded. The value of ρ for the remaining items was less than (0.05), indicating statistically significant differences between the two groups, and thus accepting these items. Consequently, the number of items ready for the questionnaire became (30) items.
3.4 Exploratory factor analysis
Factor analysis relies on the idea of summarizing the number of measured variables and reducing them to a few latent variables that represent the complete information related to the causal relationships between the measured variables (Yong and Pearce, 2013).
After identifying the 30 items selected in the previous steps, measuring the opportunities and challenges of artificial intelligence applications, these items were randomly distributed in the questionnaire model to a group of faculty members at An-Najah National University, where their number was (130 members). They were asked to answer each item on a five-point Likert scale (1–5), where the value 1 means: strongly disagree, 2: disagree, 3: neutral, 4: agree, 5: strongly agree. After applying exploratory factor analysis to the questionnaire items using the SPSS var. 26 program and ensuring compliance with the conditions of factor analysis, which are: Determinant value reached (0.005) for the opportunity questionnaire and (0.076) for the challenge questionnaire, where its value must not be less than (0.0001). Sample adequacy was measured through the KMO test, which should not be less than (0.50), and its value for the opportunity questionnaire was (0.794) and for the challenge questionnaire (0.723), indicating sufficient sample size and increasing the reliability of the factors obtained. The sample’s compatibility in terms of size was measured by the statistical significance of the Chi-square value for the Bartlett test, where it reached (653.04) with a significance level of (0.000) for the opportunity questionnaire, and (320.83) with a significance level of (0.000) for the challenge questionnaire, indicating statistically significant inter-correlations between the items, making factor analysis feasible and executable. The compatibility of each variable was measured on its own with the sample variables, obtained from the MSA values, found in the diagonal of the Anti-Image Matrices correlation coefficients, which must be greater than (0.5). In the questionnaires for this study, all values were greater than (0.5).
3.5 Firstly, questionnaire on the opportunities of employing artificial intelligence applications
After verifying the conditions for exploratory factor analysis, it was conducted using the principal component method and Varimax orthogonal rotation, as shown in the Table 3.
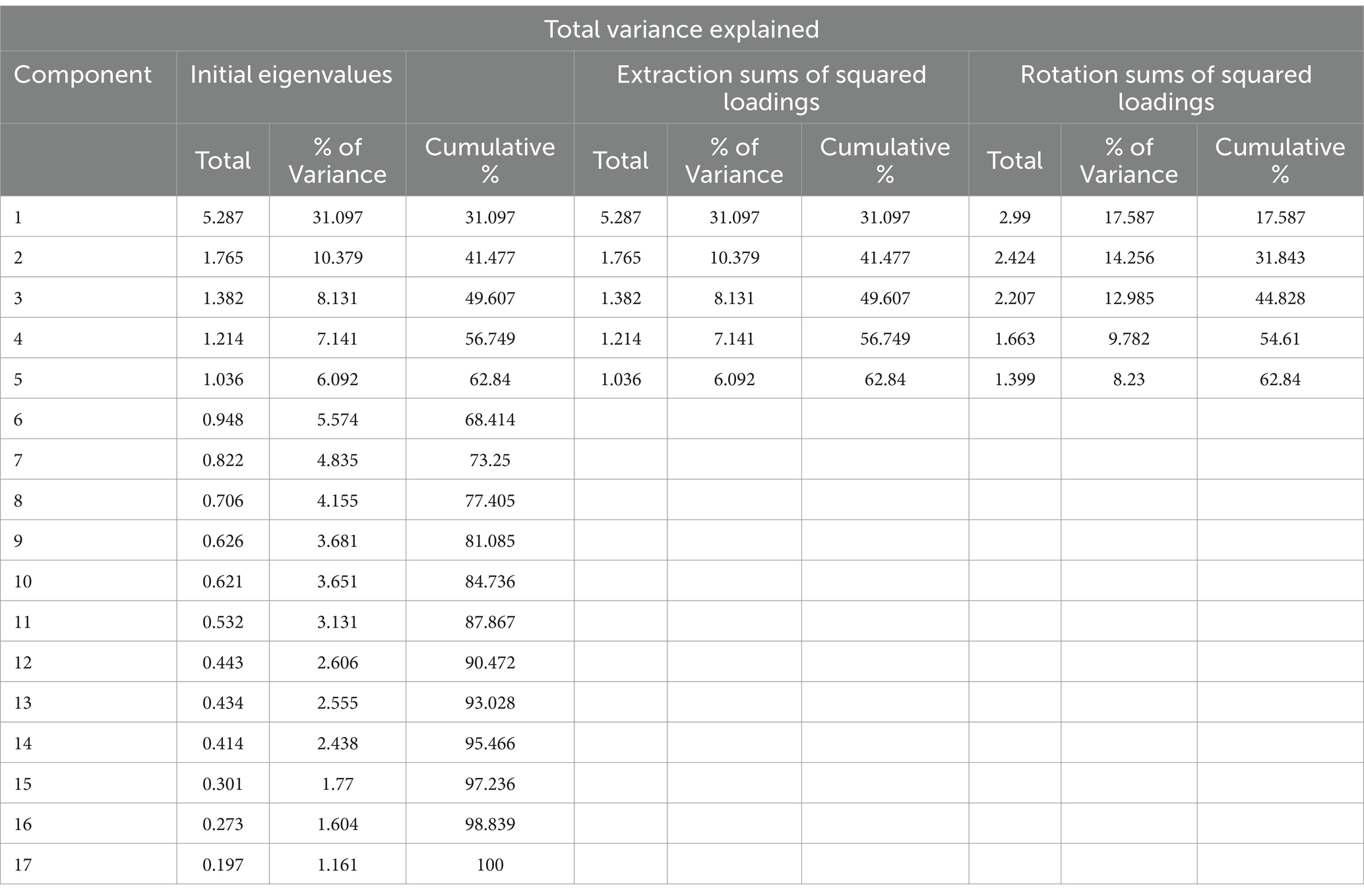
Table 3. Explained variance data for the questionnaire on opportunities of employing artificial intelligence applications in higher education.
Table 2 presents the data of the explained variance. The analysis yielded five factors whose eigenvalues exceeded one. The variance explained by the first factor was 17.587%, and the second factor accounted for 14.256% of the variance. The third factor explained 12.985% of the variance, while the fourth factor accounted for 9.782%. The fifth factor explained 8.23% of the variance. Each item’s saturation on the factor it belonged to is as shown in Table 2. Together, these factors explained 62.84% of the total variance, which is a high percentage, indicating a clear variance. The higher the variance percentage, the greater the factor detection. According to Gorsuch (1983) and Howard (2016), the emergence of a factor is confirmed by the saturation of at least three variables on it. If this is not achieved, the factor is considered insignificant and should be deleted along with the items belonging to it. Hence, items (9, 17) were deleted. This leaves us with four main factors, as shown in the Figure 1.
The eigenvalues in Figure 1 confirms the reason for choosing 4 factors, each of which was higher than one. Consequently, orthogonal rotation using the Varimax method was conducted to extract the matrix of saturations on the items. Table 4 illustrates this.
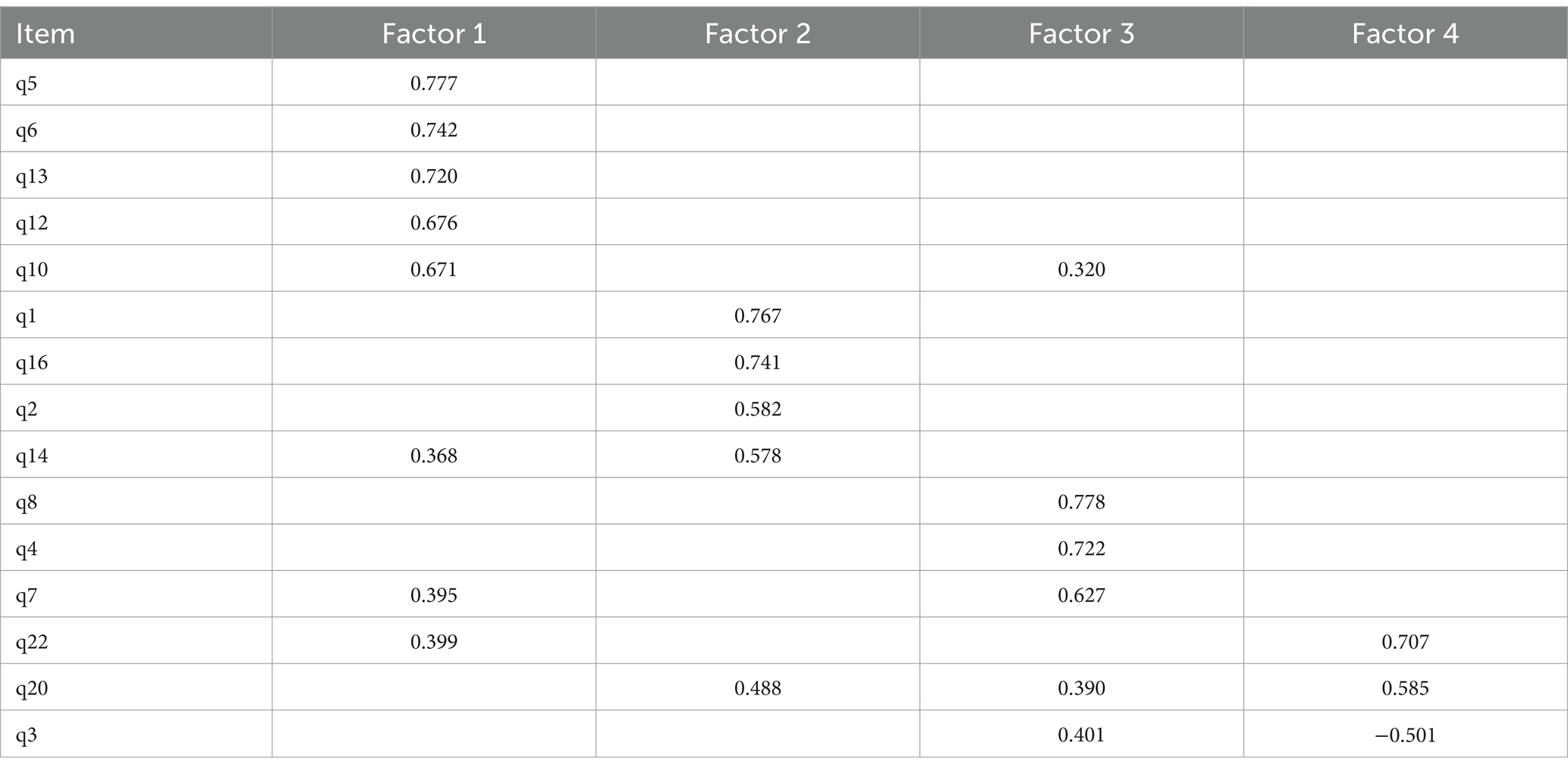
Table 4. Displays the statements of the scale for opportunities of employing artificial intelligence applications in higher education after conducting the orthogonal rotation (Varimax).
The previous table clearly shows the four underlying factors and the saturations of the items on these factors, where all of them were above (0.3). The first dimension was saturated by five items: (13, 5, 12, 6, 10). The second dimension was saturated by four items: (16, 1, 2, 14). The third dimension was saturated by three items: (8, 4, 7). As for the fourth and final dimension, it was also saturated by three items: (20, 22, 3). Thus, the questionnaire in its final form is as shown in Table 5.
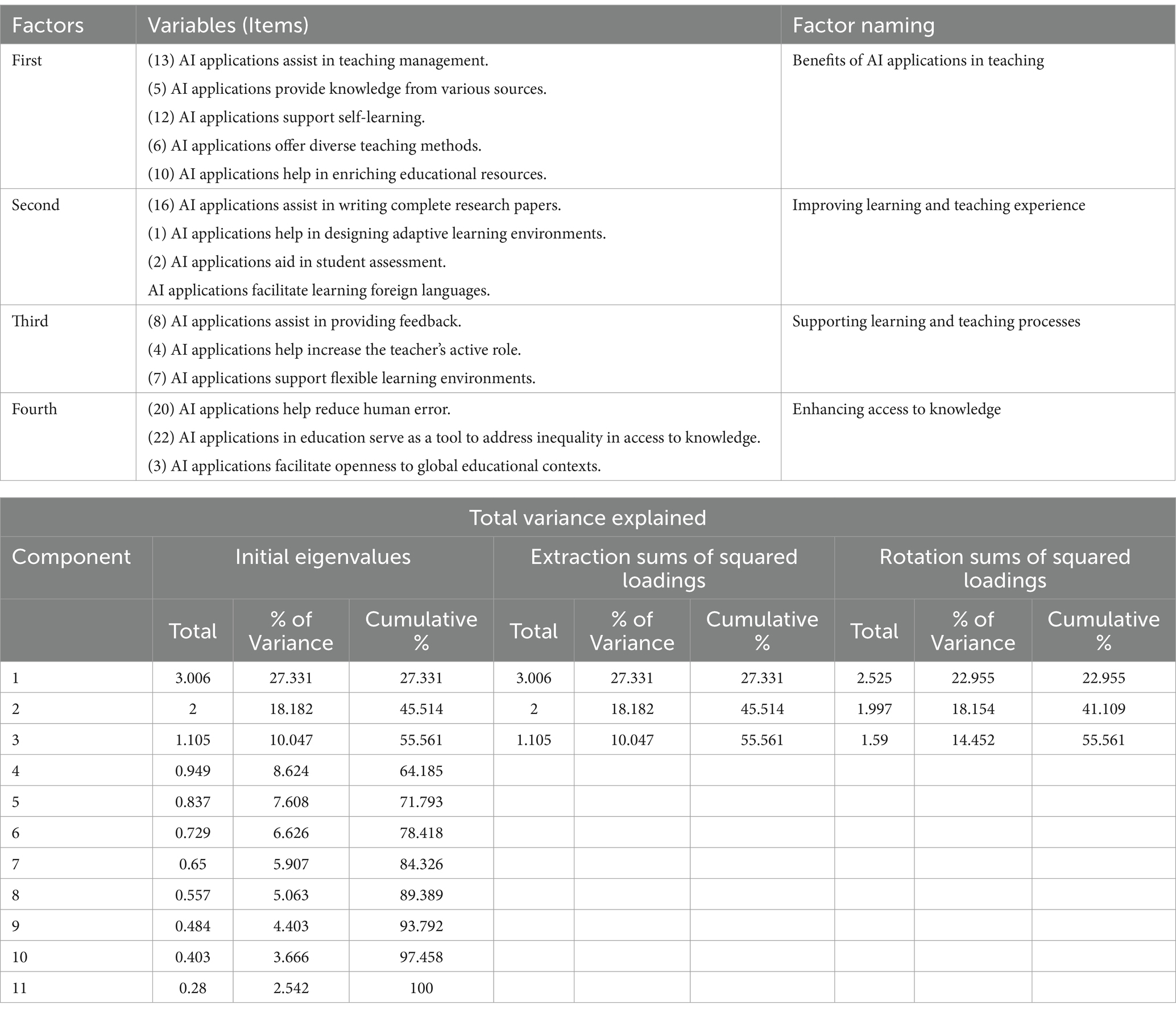
Table 5. (A) The final factors and their naming for the questionnaire on opportunities of employing artificial intelligence applications in higher education; (B) Explained variance data for the questionnaire on challenges of employing artificial intelligence applications in higher education.
3.6 Challenges questionnaire of integrating artificial intelligence applications in higher education
During the verification of the conditions for exploratory factor analysis on the questionnaire regarding the challenges of employing artificial intelligence applications in higher education, items (29, 15) were deleted due to not meeting the conditions for factor analysis. After conducting the factor analysis again using the principal component method and Varimax orthogonal rotation, the Table 5 illustrates the results.
Table 5 shows the explained variance data, and the analysis yielded three factors with eigenvalues exceeding one. The variance explained by the first factor was 22.955%, the second factor accounted for 18.154% of the variance, and the third factor explained 14.452% of the variance. The saturation of each item on its corresponding factor, as shown in Table 4, collectively explained 55.561% of the total variance. This high percentage indicates a clear variance, meaning that the higher the variance percentage, the greater the factor detection efficiency (Figure 2).
The diagram of eigenvalues confirms the reason for selecting 3 factors, each of which was higher than one. Consequently, orthogonal rotation using the Varimax method was conducted to extract the matrix of saturations on the items. Table 5 illustrates this (Table 6).
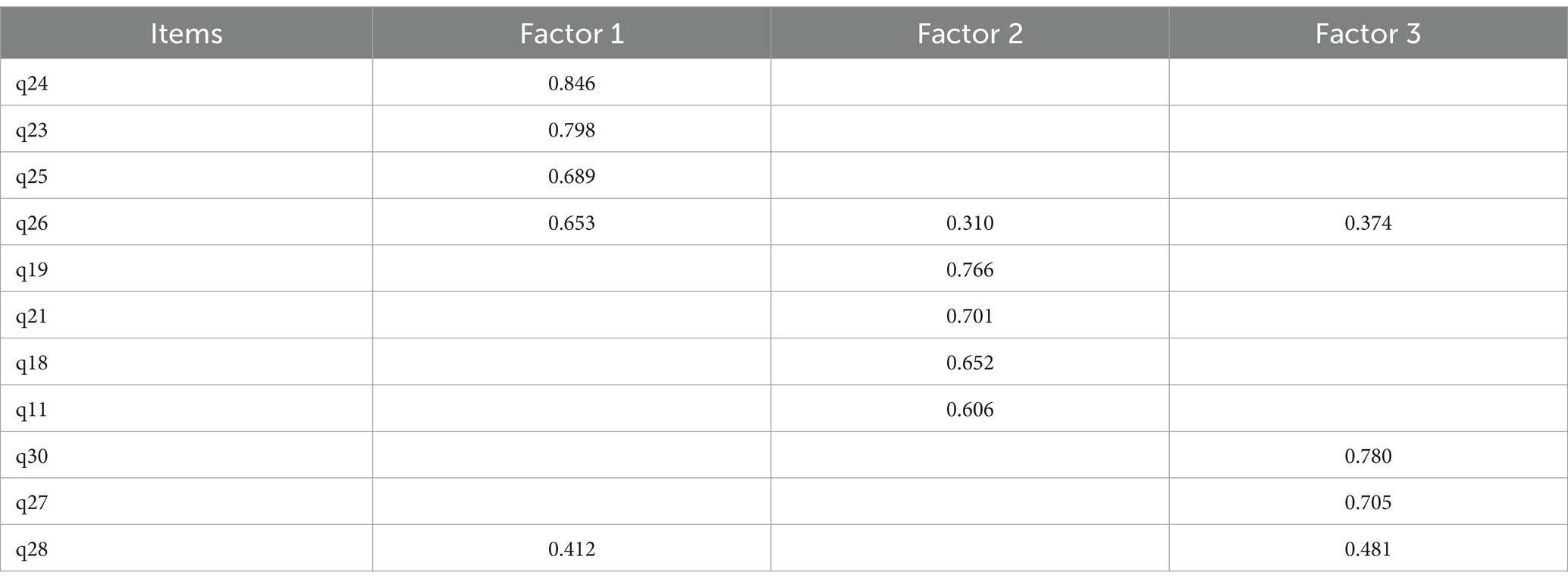
Table 6. Displays the Statements of the Scale for challenges of integration artificial intelligence applications in higher education after conducting the orthogonal rotation (Varimax).
This table shows how each questionnaire item (q24, q23, q25, etc.) is associated with one of the three factors identified in the study of challenges of employing AI applications in higher education. Each cell indicates the degree of association of each item with the corresponding factor.
Table 7 lists the items of each factor along with a descriptive name for each factor, highlighting different aspects of the challenges of employing AI applications in higher education.
3.7 Reliability and validity of the research instrument
The reliability of the study tools was calculated using Cronbach’s Alpha coefficient through SPSS software to verify the internal consistency reliability of the questionnaire items as shown in the Table 8.
From the previous table, it’s evident that the reliability of the Opportunities Questionnaire was 0.840, a value indicating good stability and reliability upon which results can be generalized (Taber, 2018). The reliability of the Challenges Questionnaire was 0.687, which is considered acceptable, allowing for reliable generalization of the results (Streiner, 2003).
3.8 Statistical assumptions
The assumption of normal distribution is satisfied, as the sample size is larger than (30), according to the Central Limit Theorem (CLT). Deviation from the assumption of normal distribution does not affect the results of parametric tests (Kaur and Kumar, 2015). To ensure data homogeneity, Levene’s test was used for variables (gender, degree, faculty, age, and experience) across the questionnaire domains (Opportunities and Challenges). It was found that the domain of Supporting Learning and Teaching Processes (Factor 3) in the Opportunities Questionnaire is not homogeneous with respect to gender, degree, age, and experience. The domain of Enhancing Access to Knowledge (Factor 4) is not homogeneous with respect to age. In the Challenges Questionnaire, all three domains meet the homogeneity condition for variables (gender, experience, and age), but there is no homogeneity for the degree variable across all domains. For the faculty variable, the domain of Challenges in Learning and University Administration (Factor 1) is not homogeneous, necessitating the use of appropriate non-parametric tests for these domains. Outliers were identified and confirmed using boxplots, which showed some upper and lower extreme values, but they were not excessively extreme.
3.8.1 Statistical procedures
SPSS V 26 software was used to perform statistical tests. To understand the beliefs of faculty members about employing AI applications in higher education, we calculated the level of beliefs using the following formula: Category length = (Highest value - Lowest value) ÷ Number of tool options = (5–1) ÷ 5 = 0.80. In this way, we obtained intervals for the level of beliefs about employing AI applications, as shown in Table 9.

Table 9. Classification of beliefs toward integrating AI applications in terms of using the grading system.
Additionally, the Independent Samples Test and Mann–Whitney Test were used to determine if there are differences in the beliefs of the sample members about employing artificial intelligence applications attributed to the gender variable and the faculty variable. The One-Way ANOVA and Kruskal-Wallis Test were used to find out the differences in the responses of the sample members in their beliefs about employing artificial intelligence applications in higher education attributed to the academic degree variable and years of experience.
4 Results
The results related to answering the first question, which is:
What is the level of beliefs of faculty members at An-Najah National University regarding the opportunities of employing artificial intelligence applications in higher education?
To answer this question, the arithmetic means and standard deviations were extracted. The averages of the domains of the Opportunities Questionnaire for employing artificial intelligence applications and the total average of the tool were calculated. Table 10 illustrates the results:

Table 10. Level of beliefs of faculty members regarding the opportunities of employing artificial intelligence applications in higher education.
This table displays the mean and standard deviation for each domain of the questionnaire assessing faculty members’ beliefs about the opportunities of employing artificial intelligence in higher education. The belief levels across all domains are categorized as high, indicating a generally positive perception among faculty members regarding the potential benefits and opportunities of AI applications in this field.
The previous table indicates that the level of beliefs among faculty members at An-Najah National University regarding the opportunities of employing artificial intelligence applications in higher education is high. The overall average is 3.99, with a standard deviation of 0.51. The domain of ‘Benefits of AI Applications in Teaching and Learning’ recorded the highest level with an average of 4.15 and a standard deviation of 0.65, while the domain of ‘Enhancing Access to Knowledge’ scored the lowest with an average of 3.86 and a standard deviation of 0.63. All domains were rated high, suggesting that faculty members strongly believe that AI applications can offer promising opportunities to improve the quality of higher education.
To answer the second question, arithmetic means and standard deviations were extracted. The averages of the domains of the Challenges Questionnaire for employing artificial intelligence applications and the total average of the tool were calculated. Table 11 illustrates the results:

Table 11. Level of beliefs of faculty members regarding the challenges of employing artificial intelligence applications in higher education.
The previous table indicates that the level of beliefs among faculty members at An-Najah National University regarding the challenges of employing artificial intelligence applications in higher education is high. The overall average was 3.90, with a standard deviation of 0.47. All domains were rated high, with the domain of ‘Challenges in Teaching and University Administration’ achieving the highest level with an average of 3.94 and a standard deviation of 0.67. The domain of ‘Technical and Security Challenges in Employing AI Applications’ scored the lowest with an average of 3.67 and a standard deviation of 0.73. This suggests that faculty members strongly believe that there are significant problems and obstacles to employing AI applications in higher education.
To determine if there were statistically significant differences in faculty members’ beliefs about employing artificial intelligence applications in higher education attributed to years of experience, the One Way ANOVA test was used for all fields except for the field of (Supporting Teaching and Learning Processes), which was calculated using the non-parametric Kruskal-Wallis test. The results are shown in Tables 12, 13.

Table 13. Results of the non-parametric Kruskal-Wallis Test according to the variable of years of experience.
The table indicates that there are no statistically significant differences in the beliefs of faculty members regarding the employment of artificial intelligence applications in higher education attributed to the variable of years of experience. The values of the significance level for all domains were greater than 0.05. This suggests that the variable of years of experience does not have an impact on the beliefs of faculty members in employing artificial intelligence applications in higher education.
The table shows no significant statistical differences in the beliefs of faculty members about the use of artificial intelligence applications in higher education in the field of support for learning and teaching processes, attributed to the variable of years of experience. The significance level value came out to be 0.590, which is higher than the threshold of 0.05.
To determine if there were statistically significant differences in the beliefs of faculty members regarding the deployment of artificial intelligence applications in higher education attributed to the variable of college, the Independent Samples Test was used for all areas except for the area of “Challenges in the Field of Education and University Administration,” for which the Mann–Whitney test was applied. The results are presented in Tables 13, 14.
The previous table indicates that there are no statistically significant differences at the significance level (0.05) between the responses of the sample members regarding the beliefs of faculty members about employing artificial intelligence applications in higher education across all the fields mentioned in the table, based on the college variable. The p-values in all cases were greater than (0.05). This means that the type of college (scientific or humanities) does not have an effect on these fields (Table 15).

Table 15. Mann–Whitney Test results for the field of challenges in University Education and Management according to the college variable.
The table shows no statistically significant differences at the 0.05 level between the responses of the sample in faculty members’ beliefs about the employment of artificial intelligence applications in higher education in the field of “Challenges in University Education and Management” according to the college variable. This is attributed to the fact that colleges adopt common directives, strategies, goals, and institutional culture toward technology, which caused a consensus in members’ beliefs across different colleges in this regard. In addition, all faculty members at the university are familiar with artificial intelligence applications and capable of dealing with technology, especially after the COVID-19 pandemic and the continuation of remote education during it in all colleges. The continuation of remote education did not end with the pandemic in Palestinian universities due to the ongoing Israeli aggression on Palestinian governorates. Due to the continuous Israeli aggression on Palestinian governorates, which often includes closing roads and crossings to students, remote education strategies have been developed in Palestinian universities. Consequently, faculty members had to continue providing remote education and giving students access to education. This experience has increased their ability to use technology and positively influenced their beliefs about artificial intelligence technology and its applications.
5 Discussion
The current study reveals a significant insight into the attitudes of faculty members in Palestinian universities toward the integration of artificial intelligence (AI) applications in higher education. Faculty members show a high level of awareness and readiness to employ AI in their teaching methodologies and administrative tasks. This aligns with global trends indicating a growing reliance on AI to enhance educational outcomes and administrative efficiency (Crompton and Burke, 2023).
A substantial body of research underscores the transformative potential of AI in education. AI applications have been shown to support personalized learning, streamline administrative processes, and offer innovative solutions to pedagogical challenges (Huang et al., 2021). For instance, AI-driven tools can analyze student data to provide personalized learning experiences, predict academic performance, and identify at-risk students (Jain and Jain, 2019). This capability to tailor educational experiences to individual needs is particularly valuable in addressing diverse student populations and enhancing overall educational quality (Zawacki-Richter et al., 2019).
5.1 Challenges and concerns
Despite the enthusiasm for AI, the study also highlights significant challenges and concerns associated with its implementation. Faculty members express apprehensions regarding over-reliance on AI, potential job displacement, and ethical issues surrounding data privacy and algorithmic bias (Chatterjee and Bhattacharjee, 2020). These concerns are not unfounded, as the rapid adoption of AI technologies can lead to unintended consequences, such as diminishing human interaction in the learning process and exacerbating existing inequalities if access to technology is not evenly distributed (Arıkan et al., 2013; George and Wooden, 2023).
The technical and security challenges associated with AI adoption are also prominent. Effective AI integration requires robust data infrastructure and stringent cybersecurity measures to protect sensitive educational data (Cioffi et al., 2020). Additionally, the lack of adequate training and technical support for faculty members can hinder the effective use of AI tools, limiting their potential benefits (Gaber et al., 2023).
5.2 Gender and academic degree differences
The study found no statistically significant differences in attitudes toward AI based on gender, except in the area of supporting learning and teaching processes, where males showed more positive attitudes. This finding suggests that, while there may be some gender-specific differences in certain aspects of AI adoption, overall attitudes toward AI are broadly consistent across genders (Franken and Mauritz, 2021). Similarly, differences based on academic degree were minimal, except for a more favorable attitude toward the benefits of AI in teaching and learning among associate professors. This might reflect varying levels of exposure to and experience with AI technologies among different academic ranks (Ahmed et al., 2022).
5.3 Implications for practice and policy
The high level of positive attitudes toward AI among faculty members indicates a readiness to embrace these technologies, provided that adequate support and training are available. Universities should invest in comprehensive training programs to equip faculty with the necessary skills to effectively use AI tools (Sharawy, 2023; Garad et al., 2022). Additionally, addressing the ethical and technical challenges associated with AI can foster a more supportive environment for its integration into higher education (Pedró, 2020).
Future research should focus on evaluating the effectiveness of AI applications in educational settings through experimental studies and exploring the long-term impacts of AI on teaching and learning processes. This will provide valuable insights into best practices and inform policy decisions to maximize the benefits of AI in higher education (Pisica et al., 2023).
5.4 Limitations
This study has several limitations that must be acknowledged. First, the sample size, though comprising 130 faculty members from An-Najah National University, may not be sufficiently large to generalize the findings across all Palestinian universities or other higher education institutions in different regions. The reliance on a single university’s faculty members might not capture the broader spectrum of attitudes and experiences related to AI applications in higher education across diverse academic settings. Additionally, the data collection method, which utilized both electronic and paper-based questionnaires, could have introduced inconsistencies in responses due to different levels of access to and familiarity with digital tools among the respondents.
Second, the study’s focus on attitudes toward AI applications does not encompass the actual implementation and usage of these technologies in educational practices. While the research provides valuable insights into faculty members’ beliefs and perceived challenges, it does not explore the practical aspects of integrating AI into teaching and administrative processes. Moreover, the study does not account for the rapidly evolving nature of AI technologies and the continuous changes in higher education environments. Future research should consider longitudinal studies to track changes in attitudes and the real-world impact of AI over time, as well as expanding the scope to include a more diverse and representative sample of institutions and respondents.
5.5 Theoretical implications
5.5.1 Exploration of AI integration
The study underscores the critical need for experimental and quasi-experimental research to explore the integration of AI applications in education. Such research is essential to evaluate the efficiency and effectiveness of AI in enhancing learning outcomes and administrative processes. By investigating various AI technologies, including deep learning, machine learning, augmented learning, natural language processing, and robotics, researchers can significantly contribute to the theoretical understanding and knowledge base in these domains. This exploration will provide a robust foundation for future AI applications in education, ensuring they are grounded in empirical evidence and best practices.
5.5.2 Ethical and justice issues
There is an urgent need for comprehensive studies focusing on the ethical and justice issues associated with AI applications in higher education. Understanding the ethical implications, data privacy concerns, and potential biases in AI algorithms is crucial for developing fair and equitable AI systems. Such research will offer valuable insights into addressing these challenges and promoting ethical AI use in educational settings. This will help create a theoretical framework that guides the responsible and just implementation of AI technologies in education, ensuring they benefit all stakeholders.
5.5.3 Sustainability in higher education
Research focusing on AI applications to enhance sustainability in higher education can offer significant insights. By exploring sustainable practices and the long-term impacts of AI integration, researchers can develop theoretical frameworks that guide the sustainable implementation of AI technologies. This research will ensure that AI applications contribute positively to the educational environment without causing unintended harm. It will also provide a basis for developing strategies that promote the long-term viability and effectiveness of AI in higher education.
5.6 Practical implications
5.6.1 Training programs for faculty
Implementing intensive training programs for faculty members is essential to enhance their skills in using AI applications in higher education. These programs should be designed to be accessible and suitable for all faculty members, ensuring they have the necessary resources and support. By improving faculty proficiency in AI, the efficiency and quality of the educational process in universities will be significantly enhanced. Training programs will equip educators with the knowledge and skills needed to effectively integrate AI into their teaching practices, ultimately benefiting students and the broader educational community.
5.6.2 Resource allocation
Decision makers should prioritize providing all necessary requirements for the effective use of AI applications in higher education. Addressing concerns and risks that hinder AI adoption is crucial. This includes investing in the necessary infrastructure, technical support, and continuous professional development to ensure the successful implementation and integration of AI technologies in educational institutions. Proper resource allocation will enable institutions to fully leverage the benefits of AI, enhancing both administrative efficiency and educational outcomes.
5.6.3 Guidance for policy and strategy development
The findings of this study offer valuable guidance for policymakers and educational leaders in developing strategies and policies for AI integration. By considering the theoretical and practical implications outlined above, decision makers can create informed and comprehensive plans that support the ethical, effective, and sustainable use of AI in higher education. These policies and strategies will provide a roadmap for institutions to follow, ensuring that AI is implemented in a way that maximizes its benefits while mitigating potential risks.
5.6.4 Enhanced educational practices
For educators, the practical implications of this study highlight the importance of being proactive in adopting and integrating AI technologies. Educators should be encouraged to participate in training programs, stay informed about the latest AI developments, and actively contribute to the research on AI applications in education. This proactive approach will ensure that educators are well-equipped to leverage AI to enhance teaching and learning experiences effectively. By embracing AI, educators can improve student engagement, personalize learning experiences, and better prepare students for the future.
6 Conclusion
The study revealed that faculty members in Palestinian universities have a positive attitude toward the opportunities and challenges associated with employing artificial intelligence (AI) applications in higher education. The attitudes toward both opportunities and challenges were rated high. Interestingly, no statistically significant differences were found in areas such as “Benefits of AI Applications in Teaching and Learning,” “Improving the Learning and Teaching Experience,” “Enhancing Access to Knowledge,” “Challenges in University Education and Management,” “Concerns of Over-reliance on AI Applications,” and “Technical and Security Challenges in Employing AI Applications” based on gender. However, in the field of “Supporting Learning and Teaching Processes,” males showed a significantly more positive response.
Additionally, no significant differences were observed between faculty members’ responses across various academic degrees, except in the domain of “Benefits of AI Applications in Teaching and Learning,” where associate professors demonstrated more favorable attitudes. Years of experience did not influence the beliefs about employing AI applications in higher education, and similarly, no significant differences were attributed to the college variable.
The study highlights a positive outlook among faculty members toward the adoption of AI in higher education, recognizing its potential to enhance educational outcomes and administrative efficiency. However, it also underscores the need to address significant challenges related to technical, ethical, and training aspects. By investing in training programs, addressing ethical concerns, and ensuring robust data infrastructure, universities can effectively harness the potential of AI to transform higher education for the better. These steps will ensure that AI is used ethically and equitably, benefiting a broad spectrum of students and educators alike.
Data availability statement
The raw data supporting the conclusions of this article will be made available by the authors, without undue reservation.
Ethics statement
The studies involving human/animal participants were reviewed and approved by the Institutional Review Board (IRB) at An Najah National University under No. Int. Nov./78. The studies were conducted in accordance with the local legislation and institutional requirements. The participants provided their written informed consent to participate in this study.
Author contributions
AO: Conceptualization, Data curation, Formal analysis, Investigation, Project administration, Resources, Validation, Writing – original draft. AS: Methodology, Writing – original draft, Conceptualization, Data curation, Investigation, Resources, Supervision. ZK: Methodology, Writing – original draft, Software, Validation, Visualization, Writing – review & editing.
Funding
The author(s) declare that no financial support was received for the research, authorship, and/or publication of this article.
Acknowledgments
We used ChatGPT 4o for proofreading and we recheck the accuracy.
Conflict of interest
The authors declare that the research was conducted in the absence of any commercial or financial relationships that could be construed as a potential conflict of interest.
Publisher’s note
All claims expressed in this article are solely those of the authors and do not necessarily represent those of their affiliated organizations, or those of the publisher, the editors and the reviewers. Any product that may be evaluated in this article, or claim that may be made by its manufacturer, is not guaranteed or endorsed by the publisher.
References
Ahmed, S., Khalil, M. I., Chowdhury, B., Haque, R., Senathirajah, B. S., and Bin Omar Din, F. M. (2022). Motivators and barriers of artificial intelligent (AI) based teaching. Eurasian J. Educ. Res. 100, 74–89.
Al-Ansi, A. M., Garad, A., and Al-Ansi, A. (2021). ICT-based learning during Covid-19 outbreak: advantages, opportunities and challenges. Gagasan Pendidikan Indonesia 2, 10–26. doi: 10.30870/gpi.v2i1.10176
Arıkan, A., Saraç, H. S., Çelik, S., Caner, M., Sert, O., and Zorba, M. G. (2013). What is effective in forming our beliefs: Experience or education? Amsterdam: Elsevier.
Avery, M., Leibbrandt, A., and Vecci, J. (2023). Does artificial intelligence help or hurt gender diversity? Evidence from two field experiments on recruitment in tech. Evidence from Two Field Experiments on Recruitment in Tech (February 14, 2023).
Bajahzar, A. (2024). Multimedia educational system and its improvement using AI model for a higher education platform. SN Comput. Sci. 5, 1–12.
Bates, T., Cobo, C., Mariño, O., and Wheeler, S. (2020). Can artificial intelligence transform higher education? Int. J. Educ. Technol. High. Educ. 17, 1–12.
Chatterjee, S., and Bhattacharjee, K. K. (2020). Adoption of artificial intelligence in higher education: a quantitative analysis using structural equation modelling. Educ. Inf. Technol. 25, 3443–3463. doi: 10.1007/s10639-020-10159-7
Chaudhry, M. A., and Kazim, E. (2022). Artificial intelligence in education (AIEd): a high-level academic and industry note 2021. AI and Ethics 2, 157–165. doi: 10.1007/s43681-021-00074-z
Cioffi, R., Travaglioni, M., Piscitelli, G., Petrillo, A., and De Felice, F. (2020). Artificial intelligence and machine learning applications in smart production: Progress, trends, and directions. Sustain. For. 12:492. doi: 10.3390/su12020492
Crompton, H., and Burke, D. (2023). Artificial intelligence in higher education: the state of the field. Int. J. Educ. Technol. High. Educ. 20, 1–22. doi: 10.1186/s41239-023-00392-8
Felder, R. M., and Brent, R. (2024). Teaching and learning STEM: A practical guide. Hoboken, NJ: John Wiley & Sons.
Franken, S., and Mauritz, N. (2021). Gender and artificial intelligence–differences regarding the perception, competence self-assessment and trust. 23RD General Online Research Conference.
Gaber, S. A., Shahat, H. A., Alkhateeb, I. A., Al Hasan, S. A., Alqatam, M. A., Almughyirah, S. M., et al. (2023). Faculty members’ awareness of artificial intelligence and its relationship to technology acceptance and digital competencies at King Faisal University. Int. J. Learn. Teach. Educ. Res. 22, 473–496. doi: 10.26803/ijlter.22.7.25
Garad, A., and Al-Ansi, A. M. (2023). Transforming higher education into integrated productive institutions: a conceptualization review. European J. Contemp. Educ. E-Learn. 1, 3–18. doi: 10.59324/ejceel.2023.1(3).01
Garad, A., Haryono, S., Yaya, R., Pratolo, S., and Rahmawati, A. (2022). The relationship between transformational leadership, improving employee’s performance and the raising efficiency of organizations. Manag. Product. Eng. Rev., 15–30. doi: 10.24425/mper.2022.142052
George, B., and Wooden, O. (2023). Managing the strategic transformation of higher education through artificial intelligence. Adm. Sci. 13:196. doi: 10.3390/admsci13090196
Ghalayini, M., and Smith, N. (2023). Unlocking the potential of AI in education: what higher education needs to know, 80.
Hashmi, N., and Bal, A. S. (2024). Generative AI in higher education and beyond. Bus. Horiz. doi: 10.1016/j.bushor.2024.05.005
Howard, M. C. (2016). A review of exploratory factor analysis decisions and overview of current practices: what we are doing and how can we improve? Int. J. Human Comput. Interact. 32, 51–62.
Huang, J., Saleh, S., and Liu, Y. (2021). A review on artificial intelligence in education. AJIS 10.
Huang, X., Zou, D., Cheng, G., Chen, X., and Xie, H. (2023). Trends, research issues and applications of artificial intelligence in language education. Educ. Technol. Soc. 26, 112–131.
Iqbal, N., Ahmed, H., and Azhar, K. A. (2022). Exploring teachers’ attitudes towards using ChatGPT. Global J. Manag. Adm. Sci. 3, 97–111. doi: 10.46568/gjmas.v3i4.163
Jain, S., and Jain, R. (2019). Role of artificial intelligence in higher education—an empirical investigation. Int. J. Res. Anal. Rev. 6, 144z–150z.
Kaur, A., and Kumar, R. (2015). Comparative analysis of parametric and non-parametric tests. J. Comput. Math. Sci. 6, 336–342.
Kayyali, M. (2024). “Future possibilities and challenges of AI in education” in Transforming education with generative AI: Prompt engineering and synthetic content creation (Pennsylvania: IGI Global), 118–137.
Khlaif, Z. N., and Farid, S. (2018). Transforming learning for the smart learning paradigm: lessons learned from the Palestinian initiative. Smart Learn. Environ. 5, 1–21.
Khlaif, Z. N., Mousa, A., Hattab, M. K., Itmazi, J., Hassan, A. A., Sanmugam, M., et al. (2023). The potential and concerns of using AI in scientific research: ChatGPT performance evaluation. JMIR Med. Educ. 9:e47049. doi: 10.2196/47049
Kuleto, V., Ilić, M., Dumangiu, M., Ranković, M., Martins, O. M., Păun, D., et al. (2021). Exploring opportunities and challenges of artificial intelligence and machine learning in higher education institutions. Sustain. For. 13:10424. doi: 10.3390/su131810424
Owoc, M. L., Sawicka, A., and Weichbroth, P. (2019). “Artificial intelligence technologies in education: benefits, challenges and strategies of implementation” in IFIP international workshop on artificial intelligence for knowledge management (Cham: Springer International Publishing), 37–58.
Pedró, F. (2020). Applications of artificial intelligence to higher education: possibilities, evidence, and challenges. IUL Research 1, 61–76. doi: 10.57568/iulres.v1i1.43
Pisica, A. I., Edu, T., Zaharia, R. M., and Zaharia, R. (2023). Implementing artificial intelligence in higher education: pros and cons from the perspectives of academics. Societies 13:118. doi: 10.3390/soc13050118
Rahiman, H. U., and Kodikal, R. (2024). Revolutionizing education: artificial intelligence empowered learning in higher education. Cogent Educ. 11:2293431.
Sharawy, F. S. (2023). The use of artificial intelligence in higher education: A study on faculty perspectives in universities in Egypt [Master's thesis, the American University in Cairo]. New Cairo: AUC Knowledge Fountain.
Streiner, D. L. (2003). Starting at the beginning: an introduction to coefficient alpha and internal consistency. J. Pers. Assess. 80, 99–103. doi: 10.1207/S15327752JPA8001_18
Taber, K. S. (2018). The use of Cronbach’s alpha when developing and reporting research instruments in science education. Res. Sci. Educ. 48, 1273–1296. doi: 10.1007/s11165-016-9602-2
Yong, A. G., and Pearce, S. (2013). A beginner’s guide to factor analysis: focusing on exploratory factor analysis. Tutorials Quant. Methods Psychol. 9, 79–94. doi: 10.20982/tqmp.09.2.p079
Keywords: generative AI, higher education, attitudes, artificial intelligence, Palestine
Citation: Omar A, Shaqour AZ and Khlaif ZN (2024) Attitudes of faculty members in Palestinian universities toward employing artificial intelligence applications in higher education: opportunities and challenges. Front. Educ. 9:1414606. doi: 10.3389/feduc.2024.1414606
Edited by:
Abdullah M. Al-Ansi, Thamar University, YemenReviewed by:
Askar Garad, Muhammadiyah University of Yogyakarta, IndonesiaTahani Bsharat, International Islamic University Malaysia, Malaysia
Copyright © 2024 Omar, Shaqour and Khlaif. This is an open-access article distributed under the terms of the Creative Commons Attribution License (CC BY). The use, distribution or reproduction in other forums is permitted, provided the original author(s) and the copyright owner(s) are credited and that the original publication in this journal is cited, in accordance with accepted academic practice. No use, distribution or reproduction is permitted which does not comply with these terms.
*Correspondence: Zuheir N. Khlaif, emtobGFpZkBuYWphaC5lZHU=
†ORCID: Zuheir N. Khlaif, https://orcid.org/0000-0002-7354-7512