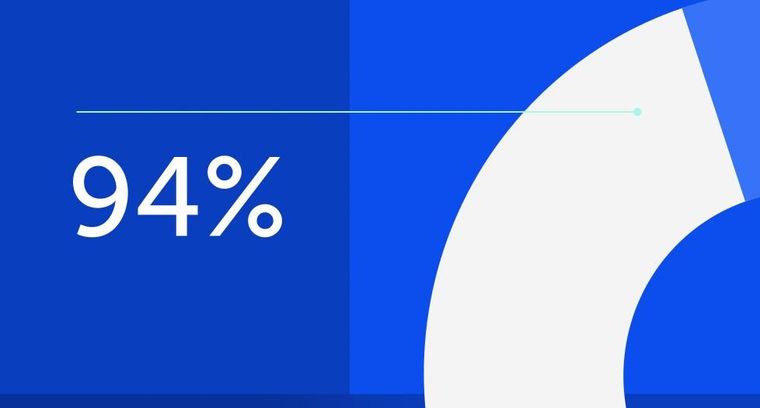
94% of researchers rate our articles as excellent or good
Learn more about the work of our research integrity team to safeguard the quality of each article we publish.
Find out more
ORIGINAL RESEARCH article
Front. Educ., 29 May 2024
Sec. Assessment, Testing and Applied Measurement
Volume 9 - 2024 | https://doi.org/10.3389/feduc.2024.1409099
The Study Demands and Resources Scale (SDRS) has shown promise as a valid and reliable measure for measuring students’ specific study demands and -resources. However, there is no evidence as to its psychometric properties outside of the original context in which it was developed. This study aimed to assess the psychometric properties of the SDRS in a cross-national student population through examining its longitudinal factorial validity, internal consistency, and temporal invariance as well as criterion validity through its association with study engagement and task performance over time. Results showed that a Bifactor Exploratory Structural Equation Model (ESEM) with one general factor (overall study characteristics) and five specific factors (workload, growth opportunities, lecturer support, peer support, information availability) fitted the data, showed strong measurement invariance over time, and was reliable at different time points. The study further established criterion validity for the overall study characteristics factor through its concurrent and predictive associations with study engagement and task performance. However, the specific factors’ concurrent and predictive capacity could only partially be established when controlling for the general study characteristics factor. These findings suggest that study characteristics should be measured as a dynamic interaction between study demands and resources, rather than a hierarchical model.
University students are considered an extremely vulnerable group that experiences higher levels of psychological distress and mental health problems than non-student age-matched groups (Stallman and Kavanagh, 2020). These high rates of psychological disorders stem from numerous stressors students face, such as demanding coursework, time pressure, and poor interpersonal relationships with peers and/or lecturers (Basson and Rothmann, 2019), which has negative consequences for both the student (e.g., poor academic performance) and the academic institution (e.g., student retention rates, academic throughput, and overall study satisfaction). It is, therefore, not surprising that universities have taken a strong interest in developing and implementing interventions to support students in their mental health and academic success (Harrer et al., 2018).
One promising approach on which interventions can be designed and evaluated is the Study Demands and Resources Framework (SDR: Mokgele and Rothmann, 2014; Lesener et al., 2020). The SDR is a framework for understanding the role that the characteristics of students’ educational experiences play in predicting the factors influencing students’ wellbeing and performance. Drawing from the conservation of resources theory and the job-demands-resources model (Demerouti et al., 2000), the SDR posits that student wellbeing and academic performance is a function of a dynamic interaction between study characteristics (study demands and resources) and its (de) energizing and motivational consequences (Lesener et al., 2020). Specifically, it argues that studying is a goal-oriented activity which involves a balance between study demands and resources. The model suggests that study demands such as workload, task complexity, and work pressure can negatively impact students’ engagement and performance, whereas study resources such as peer- and lecturer support, growth opportunities and information availability can positively impact these outcomes.
According to Van Zyl et al. (2021a), study demands refer to a study program’s physical, psychological, social and institutional aspects that require sustained physical, emotional or mental effort over time. When these study demands exceed a student’s capabilities or personal limits, it induces stress and anxiety, which may lead to burnout, poor engagement with study material and lower academic performance (Lesener et al., 2020). In contrast, study resources refer to aspects of an educational program that are functional in achieving academic goals, reducing study demands, and facilitating wellbeing. When students have access to the necessary study resources, they are more likely to reduce the harmful or de-energizing effects external demands have on their engagement and wellbeing (Kember, 2004; Krifa et al., 2022). Recent research has shown the importance of specific study resources (i.e., peer support, lecturer support, growth opportunities, and information availability) for important individual (e.g., motivation, engagement, mental health) and university outcomes (e.g., academic/task performance; academic throughput) (Mokgele and Rothmann, 2014; Lesener et al., 2020; Van Zyl, 2021; Van Zyl et al., 2024a).
The dynamic interaction between study demands and resources can activate: (a) a health impairment process/de-energizing effect or (b) a motivational process. According to Lesener et al. (2020) the health impairment process occurs when there is an imbalance in study characteristics (high study demands, low study resources) and the students’ personal resources or ability to cope. This imbalance leads to mental exhaustion and physical/psychological fatigue, which negatively affects engagement with and performance in academic tasks (Schaufeli and Bakker, 2004). In contrast, Lesener et al. (2020) argue that the motivational process is triggered when there is an abundance of study resources, as it not only acts as a buffer against the effects of high study demands but promotes motivation and engagement. In essence, this implies that when study demands are low and resources are high, students are more motivated to perform (Schaufeli and Bakker, 2004; Lesener et al., 2020).1 Designing interventions to help facilitate an optimal balance between these study characteristics is therefore essential for student success.
To develop, implement, and evaluate the effectiveness of interventions around the SDR framework, it is essential to utilise measures that can model the data captured around study characteristics (study demands and -resources) in a valid and reliable manner (Van Zyl et al., 2023). These instruments could aid in identifying the specific study related factors which students believe affect their mental health, wellbeing, and academic performance in different contexts. These could also help evaluate or monitor the effects of interventions aimed at helping students find a balance between their demands and resources over time. However, there is currently no consensus on operationalizing study characteristics comprehensively and consistently (Van Zyl, 2021). Moreover, there is a need to investigate the psychometric properties of existing measures in order to ensure that study characteristics are measured in a valid and reliable manner.
One measure which has shown promise in evaluating study characteristics is Mokgele and Rothmann’s (2014) Study Demands and Resources Scale (SDRS). The SDRS is a 23-item self-report measure, rated on a five-point Likert-type scale. It aims to assess the extent to which students perceive their academic environments as demanding and resourceful. The instrument aims to measure students’ perception of the dynamic interaction between various study characteristics (Van Zyl, 2021). Specifically, it measures students’ perceptions related to one study demand (workload) and four study resources (growth opportunities, peer support, lecturer support, and information availability), which have all shown to be important across educational sectors (Mokgele and Rothmann, 2014). These perceptions as to the availability of these demands and resources are measured as subjective experiences, which may differ from the actual availability of such.
Mokgele and Rothmann’s (2014) instrument measures and defines study characteristics as the design attributes (study demands/resources) of an academic program that may impact students’ attitudes and performance-related behaviors. From this perspective, workload (as a study demand) reflects the amount of physical/emotional/psychological effort students are expected to exert within a specific timeframe relating to the completion of academic tasks (Van Zyl et al., 2021a). In contrast, the scale considers growth opportunities, peer support, lecturer support, and information availability as study resources. Here, growth opportunities refer to the features of a study program that offer students opportunities for personal and professional development (Van Zyl et al., 2021a). As social resources, peer support pertains to the emotional, informational, and practical support or assistance students receive from their classmates, whereas lecturer support involves the guidance, feedback, and encouragement lecturers provide (Mokgele and Rothmann, 2014; Van Zyl, 2021). Finally, information availability relates to the extent to which students can access timely and relevant information to efficiently perform their study-related tasks (Mokgele and Rothmann, 2014).
Although the scale has been used in several papers, no studies other than the original paper specifically evaluated the instrument’s psychometric properties. Empirical evidence of the SDRSs factorial validity, internal consistency, temporal stability, and criterion validity is lacking in the literature. However, some support can be drawn from applied research where the instrument was used in different university contexts.
In their original study, Mokgele and Rothmann (2014) explored the factorial validity of the SDRS by estimating and comparing several competing independent cluster confirmatory factor analytical models. First, a two-factor first-order factorial model comprising two unidimensional models of study demands and overall study resources was estimated. Second, a five-first-order factorial model was estimated, which consisted of study demands (workload), growth opportunities, peer support, lecturer support, and information availability. Finally, a second-order factorial model was estimated with study resources as a higher-order factor consisting of growth opportunities, peer support, lecturer support, information availability, and a single first-order factor for study demands. Both the first and second factorial models showed poor data-model fit with model fit statistics falling outside of the suggested and ranges (CFI & TLI >0.90; RMSEA <0.08; SRMR <0.08; Non-Significant Chi-square). Only Model 3, with the higher-order factorial model for study resources (comprising four first-order factors) and the first-order factor for study demands, showed good data-model fit and was retained for further analysis. Although no other study explicitly explored the factorial validity of the instrument, various applied research studies have shown support for its factorial validity. In a study aimed at tracking study characteristics and mental health, Van Zyl et al. (2021a) found support for a unidimensional study demands and a unidimensional study resources factor which showed good reliability over 10 weeks. Similarly, Van Zyl (2021) used the peer- and lecturer support subscales of the SDRS, which showed support for a single first-order factorial model for overall social study resources (comprising lecturer support and peer support) across a 3 months period. In a cross-sectional study on a South African student population, Van der Ross et al. (2022) found that both a five-factor first-order factorial model (workload, growth opportunities, lecturer support, peer support, and information availability), as well as a unidimensional model for overall study demands and study resources fitted the data well.
It is, however, important to note that all four of these studies only employed independent clustering confirmatory factory analytical (ICM-CFA) approaches to estimate the factorial validity of the instrument. ICM-CFA models are based on an a priori specification of items onto target latent factors, whereby cross-loadings on other factors are not permitted (Marsh et al., 2009). The aim is to determine if a theoretical factorial model “fits” the collected data (Van Zyl and Ten Klooster, 2022). This approach poses several empirical and conceptual problems as to how factors are conceptualized and how these are eventually measured (cf. Van Zyl and Ten Klooster, 2022 for a full explanation). Specifically, the ICM-CFA approach (a) undermines the multidimensional view and measurement of constructs, (b) ignores the conceptual interaction between factors and items when modelling data, (c) negatively affects the discriminant- and predictive validity of instruments due to high levels of multicollinearity, and (d) undermines the practical, diagnostic utility of a measure (Marsh et al., 2009, 2014; Morin et al., 2016; Van Zyl and Ten Klooster, 2022).
The ICM-CFA approach also brings about challenges between how factors are theoretically conceptualised and how they are modelled (Van Zyl and Ten Klooster, 2022; Van Zyl et al., 2023, 2024a). Mokgele and Rothmann (2014) theoretically positioned study demands and -resources as factors that dynamically interacts but measured and modelled such via the ICM-CFA approach as orthogonal (i.e., statistically independent). This implies that various study demands, and study resources are measured as independent factors however, in both the practical and theoretical sense, these factors are related and interacts. For example, workload and time pressure are seen as independent factors, however when students experience high levels of workload, its usually driven by extreme time pressure (Lesener et al., 2020). Similarly, lecturer support and information availability as study resources are measured as independent factors, yet lecturers are primarily responsible for providing information about study processes and practices, which affects how students perceive the support they get from their lectures (Lesener et al., 2020). There is thus a difference between how study characteristics are theoretically conceptualised and practically assessed/modelled. The ICM-CFA approach, which forces non-target factor loadings to be zero, is therefore not able to accurately model nor capture these interactions.
To address the conceptual orthogonality of study demands and study resources while acknowledging their empirical interdependencies, we utilized exploratory structural equation modelling (ESEM) and target rotation within ESEM to model the theoretical conceptualisation of the instrument and address the empirical limitations of the ICM-CFA approach more accurately (Marsh et al., 2014). ESEM is also a confirmatory factor analytical technique but expressed in an exploratory manner. Specifically, items target their a priori latent factor but cross-loadings between non-target loadings are permitted and constrained to be close to zero (Asparouhov and Muthén, 2009). This less restrictive approach allows for an interaction between items when modelling latent factors (Marsh et al., 2014). Given that study characteristics are conceptualized as a dynamic interaction between study demands and resources, and that there are conceptual overlaps between certain resources (e.g., lecturer support and growth opportunities/information availability as well as lecturer support and peer support), the ESEM approach seems more appropriate to model these factors. No study has been found that modelled study characteristics through the less restrictive ESEM framework, however it is presumed that these models will fit data on the SDRS better than more traditional ICM-CFA approaches.
The reliability and internal consistency of the SDRS has also been explored. In the original study, the SDRS measured these factors reliably with point estimate reliability estimates ranging from 0.70 to 0.79 (Mokgele and Rothmann, 2014). In Van Zyl et al. (2021a) the instrument assessed the two unidimensional models for study demands and study resources reliably in students over a 10 week period with Cronbach’s alpha ranging from 0.84 to 0.96 across time. In respect of the social study resources, Van Zyl (2021) reported that the instrument assessed such reliability with acceptable levels of internal consistency across eight-time points with both composite reliability and Cronbach’s alpha ranging from 0.74 to 0.86. Similarly, Van der Ross et al. (2022) showed that the study demands (workload) and study resources sub-scales produce McDonald’s Omegas (upper-bound estimates of reliability) ranging from 0.68 to 0.84. There is thus sufficient support for the ability of the SDRS to measure study characteristics reliably. It is therefore hypothesized that the SDRS will produce acceptable levels of reliability over time.
Another matter to consider when evaluating the appropriateness of a psychometric instrument is its temporal stability. Meaningful comparisons of study demands and resources can only be made if there is evidence that the factors are measured and understood the same at different time points. Given that study demands and resources fluctuate significantly throughout the duration of a study period, it’s imperative to ensure that these factors are measured accurately, concisely and similarly over time, using for example, longitudinal confirmatory factor analysis (LFA) and longitudinal measurement invariance (LMI) to estimate scale factorial equivalence over time (Wang and Wang, 2020). LFA and LMI are used to assess: (a) if an instrument’s factorial models are stable over time with similar patterns of item loadings, (b) if items load similarly, and consistently onto their respective target factors over time, and (c) if intercepts of items are equivalent over time (Wang and Wang, 2020). Although there is some anecdotal evidence as to the SDRS factorial equivalence (at least from a factorial validity perspective; cf. Van Zyl, 2021; Van Zyl et al., 2021a) over time, no study has attempted to specifically assess its temporal stability. Thus, there are no reference points for such from the current literature. However, given that the reliability estimates of the different factorial models were relatively stable in two longitudinal studies (cf. Van Zyl, 2021; Van Zyl et al., 2021a), it is expected that the SDRS will be relatively stable over time.
To further explore the validity of the SDRS, criterion validity (concurrent and predictive validity) should be established through its relationship with different constructs, such as study engagement and task performance at different timepoints. Study engagement is a positive motivational state characterized by vigour, dedication, and absorption in studying (Ouweneel et al., 2011). Task performance pertains to “the proficiency of students to make the right choices and take the initiative to perform the most important or core/substantive tasks central to their studies” (van Zyl et al., 2022, p. 11). These tasks include reading course materials, taking notes during lectures and reading, practicing problems or exercises, writing essays or topics with opportunities for peer review, taking exams and engaging with tasks that provide feedback from peers and lecturers. Drawing from the SDR framework, Lesener et al. (2020) argued that high study demands (such as a heavy workload or severe time pressure) increase stress and decrease motivation to engage in academic content. When study demands are high, students must exert increased physical, psychological, and emotional effort to meet academic expectations and complete tasks (Schaufeli and Bakker, 2004). This mental exhaustion in turn leads to lower levels of study engagement and performance in academic-related tasks (Mokgele and Rothmann, 2014; Lesener et al., 2020).
In contrast, study resources such as growth opportunities, lecturer support, peer support, and information availability, promote self-efficacy, academic mastery, and intrinsic motivation, which ultimately leads to higher engagement and task performance (Van Zyl, 2021; Van Zyl et al., 2024b). When students have access to appropriate study-related resources, they are more likely to cope with academic demands and are more motivated to perform (Lesener et al., 2020). For example, research suggests that when academic environments provide students with growth opportunities (e.g., challenging assignments), it fosters a sense of personal mastery and enhances their competence in future tasks, which leads to higher motivation and study engagement (Lesener et al., 2020). Similarly, when students have access to appropriate social support systems, such as getting guidance/feedback from lecturers or emotional support from their peers, it enhances their self-confidence and reduces academic uncertainty, leading to improved engagement and task performance (Schaufeli and Bakker, 2004; Lesener et al., 2020).
Taken together, the SDR framework posits that students require an optimal balance between their study demands and resources in order for them to feel motivated to engage in their studies and to perform in academic tasks. Therefore, to establish concurrent validity, it’s essential to consider the relationship between study characteristics, study engagement and task performance at different time points. Similarly, to find support for the scale’s predictive validity, the relationship between study characteristics at the beginning of a course/semester and study engagement and task performance at the end of the course/semester should be considered. As such, it’s hypothesized that statistically significant relationships between these factors, at different timepoints, will be present.
Given the importance of study characteristics in both the design of educational programs and the wellbeing of students, it’s imperative to ensure that such is measured in a reliable and valid manner. The SDRS could be a viable tool to assess study characteristics and to track the impact of study demands/resources over time. Despite initial promise and anecdotal evidence in applied research, the psychometric properties of the SDRS have not been extensively investigated.
As such, this study aimed to investigate the psychometric properties, longitudinal invariance, and criterion validity of the SDRS within a cross-national student population. Specifically, it aimed to determine the (a) longitudinal factorial validity and the internal consistency of the instrument, (b) its temporal invariance, and (c) its criterion validity through its relationship with study engagement and task performance within and between different time points.
An availability-based sampling strategy was employed to draw 1,410 participants at Time 1 and 1,265 participants at Time 2 from five universities located in Belgian, Netherlands, India, Hong Kong, and the United States. Questionnaires were distributed electronically at the beginning and end of a given course. Data collection took place over a period of three years. The demographic characteristics of the sample at Time 1 and Time 2 are summarised in Table 1. The majority of the participants were Dutch-speaking (Time 1: 36.81%, Time 2: 41.03%) males (Time 1: 56.95%, Time 2: 61.74%) of European descent (Time 1: 70.59%, Time 2: 78.70%) between the ages of 18 and 23 years (Time 1: 81.42%, Time 2: 78.70%).
The Study Demands and Resources Scale (SDRS: Mokgele and Rothmann, 2014) was used to measure study characteristics (study demands, study resources). The 23-item self-report scale measured overall study demands with items such as “Do you have too much work to do?,” and four study resources: (a) peer support (“When necessary, can you ask fellow students for help?”), (b) lecturer support (“Can you discuss study problems with your lecturers?”), (c) growth opportunities (“Do your studies offer opportunities for personal growth/development?”), and (d) information availability (“Are you kept adequately up-to-date about issues within the course?”). The scale employed a 5-point Likert scale ranging from 1 (“Never”) to 5 (“Always”). The SDRS has been shown to be a reliable instrument in other studies with McDonald’s Omega and Cronbach’s alpha ranging from 0.68 to 0.96 (Mokgele and Rothmann, 2014; Van Zyl, 2021; Van Zyl et al., 2021a; van der Ross et al., 2022).
The student version of the Utrecht Work Engagement Scale (UWES-S: Schaufeli et al., 2006) was used to measure study engagement. The nine-item self-report scale is rated on a seven-point Likert-type scale ranging from 0 (“Never”) to 6 (“Always”). The instrument measures three components of study engagement: (a) vigour (“When I am doing my work as a student, I feel bursting with energy”), (b) dedication (“I am proud of my studies”), and (c) absorption (“I get carried away when I am studying”). The UWES-S has shown to be a reliable measure with Cronbach Alpha’s ranging from 0.72 to 0.93 in various studies (Schaufeli et al., 2009).
The student version of the Task Performance Scale (TPS: Koopmans et al., 2012) was used to measure students’ overall task performance. The seven-item self-report instrument is measured on a six-point Likert type scale ranging from 1 (“Never”) to 6 (“Always”) with items such as “I knew how to set the right priorities.” The TPS has also shown to be a reliable measure with McDonald’s Omegas and Cronbach Alpha’s ranging from 0.72 to 0.93 in various studies (Magada and Govender, 2017; van Zyl et al., 2022; Van Zyl et al., 2024a).
Data were analysed with both JASP 0.15 (JASP, 2021) and Mplus 8.8 (Muthén and Muthén, 2023).
First, to explore the factorial structure of the SDRS, all items at each time stamp were entered into an exploratory factor analysis with a varimax rotation. Here, the structural equation modelling (SEM) approach was employed with the robust maximum likelihood estimation method (MLR). Competing EFA models were specified, estimated and compared based on conventional data-model fit statistics (cf. Table 2). Factors with eigenvalues larger than one were extracted, and items were required to load statistically significantly on each extracted factor (factor loading >0.35; p < 0.01). Further, items needed to explain at least 50% of the overall variance.
Second, the factorial validity of the SDRS was explored by employing a competing confirmatory factor analytical measurement modelling strategy with the MLR estimation method. To determine the best fitting model for the SDRS, both independent cluster modelling confirmatory factor analytical approaches (CFA) and modern exploratory structural equation modelling (ESEM) approaches were used to estimate and compare different competing measurement models at both time points separately (Van Zyl and Ten Klooster, 2022; Muthén and Muthén, 2023). For the traditional CFAs, items were only permitted to load onto their a priori factorial models, and cross-loadings were constrained to zero. A target rotation was used for the bifactor CFA models, and factors were specified as orthogonal. Here a general study characteristics factor (G-Factor) and different permutations of specific study characteristics (S-Factors) which corresponded to the theoretical dimensions of the SDRS were extracted. The ESEM models were estimated and specified in accordance with the best practice guidelines of Van Zyl and Ten Klooster (2022). The De Beer and Van Zyl (2019) ESEM syntax generator were used to generate the Mplus code for the ESEM Models. Within this framework, ESEM factors were specified similar to the CFA models; however, cross-loadings between items and non-target factors were permitted. These were constrained to be close to zero and a target rotation was used (Van Zyl and Ten Klooster, 2022). Bifactor ESEM models similar to their CFA counterparts were also specified. Here cross-loadings between S-factors were permitted and constrained to be as close to zero as possible (Van Zyl and Ten Klooster, 2022). Set-ESEM models were also estimated where cross-loadings were only permitted (but constrained to be close to zero) for theoretically related factors. The best-fitting measurement model for the data was determined by evaluating and comparing models based on Hu and Bentler’s (1999) data-model fit criteria (cf. Table 2) and indicators of measurement quality. Measurement quality was evaluated through standardized factor loadings (e.g., λ > 0.35), item uniqueness (e.g., >0.10, but <0.90), and levels of tolerance for cross-loadings (Kline, 2010). For the bifactor models, measurement quality was indicated through a well-defined general factor of overall study characteristics and relatively well-defined specific factors (that allows for lower factor loadings on target factors). Models that met both measurement quality and data-model fit criteria were retained for further analysis (McNeish et al., 2018).
Third, a longitudinal factor analytic (LFA) strategy was employed to determine the factorial stability of the SDRS between Time 1 and Time 2. Here, the best-fitting CFA / ESEM measurement models from Time 1 were regressed on their corresponding counterparts in Time 2 (Von Eye, 1990; Van Zyl et al., 2021a). In addition to meeting the data-model fit and measurement quality criteria, the regression paths between factors were required to be significant and large (Standardized β > 0.50; p < 0.05) and the standard errors low (Von Eye, 1990). LFA models that met all four criteria were retained for further analysis.
Fourth, the temporal equivalence or “longitudinal measurement invariance” (LMI) of the best-fitting LFA model of the SDRS was estimated to determine whether study characteristics were measured similarly between Time 1 and Time 2. LMI was evaluated by estimating and comparing a series of increasingly restrictive models: (a) configural invariance- (similar factor structures over time), (b) metric invariance (similar factor loadings over time), and (c) scalar invariance (similar intercepts over time). Models were compared based on Chen’s (2007) criteria: changes in RMSEA (Δ < 0.015; p > 0.01), SRMR (Δ < 0.02 for configural versus metric/scalar; Δ < 0.01 for metric versus scalar), CFI (Δ < 0.01), and TLI (Δ < 0.01) (Chen, 2007; Wang and Wang, 2020). Chi-square and chi-square differences test were estimated and reported for transparency, but due to current debates and challenges associated with the statistic, the results were not used as evaluation criteria (cf. Morrin et al., 2020; Wang and Wang, 2020). Latent mean comparisons were also estimated for the model, which showed to be invariant over time. Mean scores at Time 1 were used as the reference group and constrained to zero, and mean scores at Time 2 were freely estimated (Wang and Wang, 2020). Latent mean score differences should differ significantly from zero (p < 0.05).
Fifth, standardized factor loadings and internal consistency were reported for the model that showed to be invariant over time. Internal consistency was estimated through McDonald’s Omega (ω > 0.70; Hayes and Coutts, 2020).
Finally, to establish concurrent and predictive validity, separate structural models were estimated based on the best-fitting LFA and LMI model. Study characteristics were indicated as exogenous factors and study engagement and task performance as endogenous factors. For concurrent validity, study characteristics (both the general and specific factors) at Time 1 were regressed on Study Engagement and Task Performance at Time 1. Similarly, study characteristics at Time 2 were regressed on Study engagement and Task Performance at Time 2. For predictive validity, study characteristics at Time 1 were regressed on Study Engagement and Task Performance at Time 2. A significance level of p < 0.05 (95% confidence interval) for each regressive path was set.
The psychometric properties, longitudinal invariance, and criterion validity results are briefly described, tabulated, and reported in separate sections.
To explore the factorial structure of the SDRS, an EFA approach with a Varimax rotation was employed on the data at both Time 1 and Time 2. As an initial measure, one to six factorial models were specified to be extracted. Factorial models were specified based on the a priori factorial structure of the original SDRS. At both timepoints, four items (“Do your studies require creativity?”, “Do you have enough variety in your studies?” “Do you know exactly what your lecturers expect of you in your studies?”, “Do you know exactly what your lecturers think of your performance?”) needed to be removed due to poor factor loadings.
The results summarised in Table 3 showed that a five-factor model fitted the data best at both Time 1 (χ2(1410) = 291.017; df = 86; RMSEA = 0.04 [0.036, 0.046] p = 0.99; SRMR = 0.02; Cumulative R2 = 57.98) and Time 2 (χ2(1265) = 268.481; df = 86; RMSEA = 0.04 [0.035, 0.047] p = 0.99; SRMR = 0.02; Cumulative R2 = 60.31). The five-factor model showed a significantly better fit than any other factorial model. The five factors were labelled: Workload (5 items), Growth Opportunities (4 items), Peer Support (3 items), Lecturer Support (3 items), and Information Availability (4 items). The item loadings and declared variance for this model are presented in Table 3. All items loaded larger than 0.35 onto their respective factors (cf. Kline, 2010).
A competing measurement modelling strategy was employed to establish the factorial validity of the SDRS at each of the two-time points. Measured items were used as observed indicators, no items were removed, and error terms were freely estimated. In total, 27 separate models were tested and systematically compared at each time point (cf. Appendix A for a visual representation). A description of each model and the associated model fit statistics are summarised in Table 4.2
Table 4. Cross-sectional confirmatory factor analyses: measurement model fit statistics for Time 1 and Time 2.
The results showed that only two models fitted the data at both Time 1 and Time 2. At Time 1, Model 14, the Bifactor CFA Model with one General Factor (Overall Study Characteristics) and five specific factors (Workload, Growth Opportunities, Peer Support, Lecturer Support, and Information Availability: cf. Figure 1) (χ2(1410) = 387.85; df = 133; CFI = 0.96; TLI = 0.95; RMSEA = 0.04 [0.033, 0.041] p = 1.00; SRMR = 0.04; AIC = 64126.38; BIC = 64525.49) and the corresponding Bifactor ESEM Model 22 with one General Factor (Overall Study Characteristics) and five specific factors (Workload, Growth Opportunities, Peer Support, Lecturer Support, and Information Availability: cf. Figure 1) (χ2(1410) = 175.11; df = 72; CFI = 0.98; TLI = 0.96; RMSEA = 0.03 [0.026, 0.038] p = 1.00; SRMR = 0.01; AIC = 63993.94; BIC = 64713.37) fitted the data best.
A similar pattern was found at Time 2, where the Bifactor CFA Model 14 with one General and five Specific factors (χ2(1265) = 354,07; df = 133; CFI = 0.97; TLI = 0.96; RMSEA = 0.04 [0.032, 0.041] p = 1.00; SRMR = 0.03; AIC = 53801.79; BIC = 54192.64) and the corresponding Bifactor ESEM Model 22 with one General Factor and five specific (χ2(1265) = 161,84; df = 72; CFI = 0.99; TLI = 0.97; RMSEA = 0.03 [0.025, 0.038] p = 1.00; SRMR = 0.01; AIC = 53676.42; BIC = 54380.99) fitted the data best.
Both models at both time points met the measurement quality criteria producing acceptable standardized factor loadings (λ > 0.35; p < 0.01), standard errors, and item uniqueness (δ < 0.10 but >0.90; p < 0.01) (Asparouhov and Muthén, 2009; Kline, 2010), as well as similar patterns of item loadings (Morin et al., 2020).
In the next step, longitudinal factor analytical models were estimated and compared based on the best-fitting models from the previous phase. In each of the LFA models, the general and specific factors of the measurement model specified in Time 1 were regressed on their counterparts at Time 2 (cf. Appendix B for a visual representation). The following models were tested:
LFA Model 1: a Bifactor CFA model with one General Factor (Overall Study Characteristics) and five specific factors (Workload, Growth Opportunities, Peer Support, Lecturer Support, and Information Availability) at Time 1 was regressed onto their corresponding factorial counterparts at Time 2. Covariances between specific and general factors at each time point and between time points were not permitted.
LFA Model 2: a Bifactor ESEM model with one General Factor (Overall Study Characteristics) and five specific factors (Workload, Growth Opportunities, Peer Support, Lecturer Support, and Information Availability) at Time 1 was regressed onto their corresponding factorial counterparts at Time 2. Covariances between specific and general factors at each time point and between time points were not permitted. Cross-loadings between items at a given time point were permitted, but constrained to be as close to zero as possible.
The results summarised in Table 5 indicated that only LFA Model 2 (the Bifactor ESEM Model with one General and Five Specific factors) fitted the data (χ2(1265) = 1769.72; df = 530; CFI = 0.93; TLI = 0.90; RMSEA = 0.04 [0.039, 0.043] p = 1.00; SRMR = 0.05; AIC = 115258.82; BIC = 116566.41). The LFA Model 1 met all criteria except that of TLI (TLI <0.90). LFA Model 2 also fitted the data significantly better than LFA Model 1 (∆χ2 = −467.27; ∆df = −91; ∆CFI = 0.02; ∆TLI = 0.01; ∆RMSEA = 0.00; ∆SRMR = 0.00; ∆AIC: −347.81; ∆BIC: 130.06). Both longitudinal models showed acceptable levels of measurement quality with standardized factor loadings, standard errors, and item uniqueness meeting the specified thresholds (Asparouhov and Muthén, 2009; Kline, 2010).
Table 5. Longitudinal confirmatory factor analyses: measurement model fit statistics for Time 1 and Time 2.
To assess the final two assumptions for LFA, the regressive paths, as well as the variance explained by factorial models of Time 1 on Time 2, were estimated and summarised in Table 6. The results showed that all factors at Time 1 for both LFA models predicted their counterparts in Time 2. Both models, therefore, showed regression paths between factors to be significant and large (Standardized β > 0.50; p < 0.05) and the standard errors low (Von Eye, 1990). Both LFA models, therefore, met the relationship criteria. Although LFA Model 1 did not meet the TLI criteria, both models were retained for invariance testing.
After confirming the factorial structure over time, the next step was to evaluate the scale’s temporal equivalence through LMI. Separate LMI tests were estimated for the LFA Model 1 and LFA Model 2. The results, summarised in Tables 7, 8, showed that both the Bifactor CFA and the Bifactor ESEM models fitted the data. However, LMI could only be established for the Bifactor ESEM Model (LFA Model 2). No statistically significant differences in terms of RMSEA (Δ < 0.015), SRMR (Δ < 0.015), CFI (<0.010), and TLI (<0.010) between the configural, metric, and scalar invariance models were found (Wang and Wang, 2020). Therefore, only the Bifactor ESEM model with one general and five specific factors showed to be consistent over time, hence meaningful mean comparisons between Time 1 and Time 2 can be made.
Latent mean scores for the general and specific factors at Time 1 were constrained to zero, whereby their counterparts were permitted to be freely estimated at Time 2. The results showed that only overall Study Characteristics (Δx‐ = −0.15; SE = 0.03; p = 0.00), Lecturer Support (Δx‐ = 0.34; SE = 0.06; p = 0.00) and Information Availability (Δx‐ = 0.13; SE = 0.05; p = 0.04) at Time 2 differed meaningfully from Time 1.
Taken together, only the Bifactor ESEM model with One General and Five Specific factors was retained for further analysis. Next, the standardized factor loadings, item uniqueness and the level of internal consistency were estimated for this model. The results are summarised in Tables 9, 10.
The results show that both general and specific factors are measured reliably at both time points with McDonald’s Omegas (ω > 0.70) exceeding the suggested ranges. Further, at both time points the General factors are well defined and Specific factors are relatively well defined with significant factor loadings present on target factors. However, items SDR_2 and SDR_3 at Time 2 seem to be better defined by the specific factor. Taken together, the Bifactor ESEM Model with one General and five Specific factors showed good measurement quality and is retained for the final analysis.
To establish criterion validity, both concurrent and predictive validity was estimated through the SDRS’s relationship with study engagement and task performance at different time points. Here, the general and specific factors of the Bifactor ESEM model were specified as exogenous factors while study engagement (as a function of vigour, dedication, and absorption) and task performance were specified as endogenous factors. The results for both concurrent and predictive validity are summarised in Tables 11, 12 (cf. Appendices C & D for a visual representation).
For concurrent validity, SDRS’s general and specific factors at Time 1 were first regressed on Study Engagement at Time 1. The model showed adequate fit (χ2(1410) = 703.329; df = 276; CFI = 0.96; TLI = 0.94; RMSEA = 0.03 [0.030, 0.036] p = 1.00; SRMR = 0.03; AIC = 102140.04; BIC = 102969.75). Only Overall Study Characteristics (β = 0.51; S.E = 0.04; p < 0.05) and Growth Opportunities (β = 0.31; S.E = 0.06; p < 0.05) at Time 1 were associated with Study Engagement at Time 1.
At Time 2, Overall Study Characteristics (β = 0.55; S.E = 0.04; p < 0.05), Growth Opportunities (β = 0.43; S.E = 0.05; p < 0.05) and Workload at Time 2 (β = −0.09; S.E = 0.04; p < 0.05) were associated with Study Engagement at Time 2. Here, the overall SDRS explained 36% of the overall variance in Study Engagement at Time 1 and 50% at Time 2. This model also showed adequate fit (χ2(1265) = 638.30; df = 277; CFI = 0.96; TLI = 0.94; RMSEA = 0.03 [0.029, 0.035] p = 1.00; SRMR = 0.03; AIC = 85312.55; BIC = 86119.97).
Similarly, concurrent validity was explored through determining the relationship of SDRS’s general and specific factors at Time 1 with Task Performance at Time 1. This model showed good fit (χ2(1410) = 631.89; df = 227; CFI = 0.96; TLI = 0.94; RMSEA = 0.04 [0.032, 0.039] p = 1.00; SRMR = 0.03; AIC = 91120.36; BIC = 91908.060). The results showed that Overall Study Characteristics (β = 0.51; S.E = 0.04; p < 0.05), Growth Opportunities (β = 0.13; S.E = 0.06; p < 0.05), Peer Support (β = 0.14; S.E = 0.03; p < 0.05), and Lecturer Support (β = 0.18; S.E = 0.04; p < 0.05) were associated with Task Performance at Time 1.
At Time 2, Overall Study Characteristics (β = 0.54; S.E = 0.04; p < 0.05), Peer Support (β = 0.10; S.E = 0.03; p < 0.05), Lecturer Support (β = 0.22; S.E = 0.05; p < 0.05), and Workload at Time 2 (β = −0.13; S.E = 0.04; p < 0.05) were associated with Study Engagement at Time 2. Here, the overall SDRS explained 34% of the overall variance in Task Performance at Time 1 and 37% at Time 2. This model also showed adequate fit (χ2(1265) = 649.820; df = 228; CFI = 0.96; TLI = 0.95; RMSEA = 0.04 [0.035, 0.042] p = 1.00; SRMR = 0.03; AIC = 77367.84; BIC = 78134.12). Concurrent validity could therefore be established for overall study characteristics and partially for the specific factors.
For predictive validity, SDRS’s general and specific factors at Time 1 were first regressed on Study Engagement at Time 2. The model showed adequate fit (χ2(1265) = 600.68; df = 276; CFI = 0.97; TLI = 0.96; RMSEA = 0.03 [0.026, 0.032] p = 1.00; SRMR = 0.03; AIC = 95832.03; BIC = 96661.74). Again, only Overall Study Characteristics (β = 0.47; S.E = 0.04; p < 0.05) and Growth Opportunities (β = 0.35; S.E = 0.05; p < 0.05) at Time 1 were associated with Study Engagement at Time 2.
Predictive validity was further explored by determining the relationship between SDRS’s general and specific factors at Time 1 and Task Performance at Time 2. This model showed good fit (χ2(1263) = 622.20; df = 227; CFI = 0.96; TLI = 0.94; RMSEA = 0.04 [0.032, 0.038] p = 1.00; SRMR = 0.03; AIC = 87839.97; BIC = 88627.67). The results showed that Overall Study Characteristics (β = 0.39; S.E = 0.04; p < 0.05), Growth Opportunities (β = 0.13; S.E = 0.05; p < 0.05), Peer Support (β = 0.11; S.E = 0.04; p < 0.05), Lecturer Support (β = 0.10; S.E = 0.05; p < 0.05), and Workload at Time 1 (β = −0.11; S.E = 0.04; p < 0.05) were associated with Task Performance at Time 2. Here, the overall SDRS model at Time 1 explained 35% of the overall variance in Engagement at Time 2 and 21% of the variance in Task Performance at Time 2. Predictive validity could therefore be established for overall study characteristics and partially for the specific factors.
The study aimed to assess the psychometric properties of the SDRS in a cross-national student population. Specifically, it aimed to examine the longitudinal factorial validity, internal consistency, and temporal invariance of the SDRS and its criterion validity through its association with study engagement and task performance over time. The study estimated and compared various CFA and ESEM models to evaluate the factorial validity of the SDRS. A bifactor ESEM model with one general factor (overall study characteristics) and five specific factors (one study demand and four study resources) best fitted the data, showed strong measurement invariance over time and was reliable at different time points. Latent mean comparisons showed that experiences associated with overall study characteristics decreased over time, whereby perceptions of lecturer support and information availability increased. The study further established criterion validity for the overall study characteristics factor through its concurrent and predictive associations with study engagement and task performance. However, the specific factors’ concurrent and predictive capacity could only partially be established when controlling for the general study characteristics factor.
The results showed that none of the traditional first- or second-order CFA- and less restrictive ESEM models fitted the data adequately at both time points consecutively. There was initial support for both a bifactor CFA- and bifactor ESEM model with one general factor for overall study characteristics and five specific factors related to workload, growth opportunities, lecturer support, peer support, and information availability at each time point separately.
However, both longitudinal factor analysis and longitudinal measure invariance could only be established for the less restrictive bifactor ESEM model. Considering the longitudinal factorial validity, the less restrictive bifactor ESEM model with one general and five specific factors not only fitted the data better than its CFA counterpart, but also provided better indicators of measurement quality. The bifactor ESEM model ignores the hierarchical function of study characteristics through the expression of a general factor and specific factors with cross-loadings. From this perspective, there is some shared variance between factors with each specific factor having unique explanatory power, over and above that of the general study characteristics factor. This implies that study characteristics are both a function of, yet separate from, the dynamic interaction between workload, growth opportunities, information availability, peer- and lecturer support.
The results further imply that when allowing for cross-loadings between items that are constrained to be as close to zero as possible, it provides a more accurate representation of how study characteristics and its components are experienced in real-world terms by participants (Morin et al., 2020; van Zyl et al., 2022). Where traditional CFA approaches view each element or factor of a psychometric instrument in isolation from one another, ESEM allows for an interaction between items on different factors (Van Zyl and Ten Klooster, 2022). Therefore, the bifactor ESEM approach is more in line with how study demands and resources were initially conceptualized in the literature as a dynamic interaction between the design elements (demands/resources) of a given course/program. This dynamic interaction (where perceptions of one factor may influence that of another) cannot be accurately captured or modelled through traditional CFA approaches where factor loadings on non-target factors are constrained to be zero (van Zyl et al., 2022). Further, the ESEM approach compensates not only for the wording effects which may occur with certain items within cross-national studies, but also for the differences in the physical design of different courses at the different institutions (Morin et al., 2020; van Zyl et al., 2022).
Further, both the general and specific factors of the bifactor ESEM model showed acceptable levels of upper-bound reliably over time, with McDonald’s Omegas exceeding thresholds (ω > 0.70; Hayes and Coutts, 2020). This is in line with other findings that showed the SDRS to be a reliable measure in South Africa (Mokgele and Rothmann, 2014), and Netherlands (Van Zyl, 2021; van Zyl et al., 2022). Taken together, the results support the notion that the bifactor ESEM approach is a viable factor analytical strategy when measuring study characteristics in cross-national samples at the beginning and end of a course/semester.
When considering the temporal stability or the factorial equivalence of the bifactor ESEM model over time, the results provided support for configural, metric, and scalar invariance. This implies that both the general or “overall study characteristics” factor, as well as the five specific factors (with cross loadings permitted but constrained to be close to zero), are measured equally and consistently across time. The SDRS thus showed similar factor structures, factor loadings, intercepts and error variances at both time points. The data, therefore, shows support for the instrument’s temporal stability, which implies that latent mean differences indicate actual temporal changes in the factors over time and not changes in the meaning of constructs (Wang and Wang, 2020). Therefore, meaningful comparisons across time can be made with the instrument (Wang and Wang, 2020).
In this study, latent mean comparisons between the general and specific factors showed that only perceptions of overall study characteristics, lecturer support, and information availability changed over time. Students’ perceptions of the overall study characteristics (the balance between study demands and resources decreased) decreased throughout their course. It is important to note that perceptions of the balance between study demands and resources naturally fluctuate throughout a course/semester (Cheng, 2020). Study demands are deemed to be low, and perceptions of available resources are high at the onset of a course (Cheng, 2020). As the course progresses, study demands naturally increase, and the perceptive availability of study resources fluctuates significantly (Basson and Rothmann, 2019; Mtshweni, 2019). The decrease in study characteristics implies that students may have perceived a slight imbalance between their resources, high workloads, time pressures, and other study demands (Lesener et al., 2020). Further, the results showed that lecturer support and information availability increased over time. This implies that students perceived more guidance, feedback, encouragement, and support from their lecturers over the duration of the course and that they perceived to have access to the necessary information they required to complete study-related tasks.
The final objective of the study was to investigate the concurrent and predictive validity of the SDRS through its associations with study engagement and task performance at different time points. The results showed that concurrent and predictive validity could be established for overall study characteristics, but only partially for the specific factors.
In respect of concurrent validity, positive relationships between growth opportunities and study characteristics and study engagement were found at both Time 1 and Time 2. This is broadly in line with previous research (Mokgele and Rothmann, 2014; Lesener et al., 2020; Van Zyl, 2021; van Zyl et al., 2022). The results suggest that when students perceive that their studies provide them with opportunities to grow and develop, that they are more likely to engage in and with study-related content (Lesener et al., 2020). The finding also aligns with Self-Determination Theory which posits that when individuals perceive their need for growth and development to be met, they engage in (study-related) activities with greater enthusiasm and persistence (Ryan and Deci, 2000). Similarly, the positive relationship between overall study characteristics and study engagement can also be explained through the Cognitive Load Theory (Sweller, 2011). This theory suggests that when students perceive a balance between their demands and resources, and when their studies challenge them, they are more likely to engage with their study material, resulting in deeper learning and higher performance. Students who perceive an overall balance between their study demands/resources are more likely to be deeply engrossed in their studies (Lesener et al., 2020).
Similarly, study characteristics, peer support, and lecturer support were positively associated with task performance at both time points. The results imply that when students perceive a balance between their overall study demands/resources, and when they have access to supportive social resources such as peer and lecturer support, they may be more confident and motivated to complete study-related tasks successfully. Similar relationships have been found in previous research. For example, Wang and Eccles (2012) found that academic support (incl. Peer and lecturer support) increased the motivation and academic performance of students. Overall, this finding suggests that providing students with access to appropriate and supportive social resources, and designing courses that balance their overall demands and resources, can be beneficial for improving their performance in academic-related tasks.
Interestingly, workload was not associated with neither study engagement nor task performance at the onset of the course, but showed a statistically significant negative relationship between these factors at the end of the course. At the start of a course or semester, students may not yet have a complete understanding of the course content nor a realistic expectation of the workload, making it difficult to accurately assess the required workload (Van Zyl et al., 2021a,b). In other words, students may not perceive the workload of a given course as too heavy or too light at the onset; therefore, it may not significantly impact their current level of study engagement or performance in academic-related tasks (Lesener et al., 2020). However, as courses progress, students may begin to experience increased levels of study-related pressure or stress due to a higher workload, which could decrease their levels of study engagement and task performance towards the end of the semester (Schaufeli et al., 2006). This may explain why there is a statistically significant negative relationship between workload and these factors at the end of the course.
There were no statistically significant relationships between resources such as peer support, lecturer support, and information availability and study engagement at both time points. This is in contrast to expectations and previous research. One possibility is that students may not be aware of or perceive the availability of peer or lecturer support or information as important for their engagement at the beginning or end of a course. Other factors such as personal motivation, interest in the subject matter, required course, and perceived importance of the course in their overall study program may have a more substantial influence on their engagement (Christenson et al., 2012). It is also possible that the non-significant relationship was due to the presence of certain contextual factors or the nature of the sample. Given that this is a cross-national study, different student populations and educational settings may have different perceptions of and need for study support and resources. This difference may influence the strength and direction of the relationships (Christenson et al., 2012).
Further, no association between growth opportunities and information availability and task performance was found at both time points. At the beginning of a course or semester, students may not have had the opportunity to fully understand or engage with the learning outcomes or course material and may thus not know what information they may require to understand and perform (Christenson et al., 2012). They may also not be aware as to available growth opportunities which a course or programme may provide at its onset. Similarly, at the end of a course/semester, it is possible that students may have developed established study routines and thus adapted to the demands of the course. This implies that resources such as growth opportunities and information availability may have less of an impact on their performance over time.
In terms of predictive validity, the association between study characteristics at Time 1 and study engagement and task performance at Time 2 was estimated. The results showed a positive relationship between growth opportunities and study characteristics at the beginning of a course/semester, with study engagement at the end. This implies that if students perceive a balance between their study demands and resources, they are more likely to be engaged with their studies throughout the course. Further, when they perceive that a course will provide personal/professional development opportunities, they are more likely to immerse themselves emotionally, physically, and cognitively in the course content throughout the duration of the semester.
Further, at Time 1, growth opportunities, peer support, lecturer support, and overall study characteristics were positively and workload negatively associated with task performance at Time 2. This suggests that students who perceived a balance between their overall study demands/resources and who had specific growth opportunities, peer support, and lecturer support at the beginning of the course/semester were likelier to perform well in their academic-related tasks later in the semester. The results also showed that workload at the beginning of a course/semester was negatively associated with performance on academic tasks later in the semester. These findings corroborate those of Lesener et al. (2019) and Mokgele and Rothmann (2014). In contrast, information availability at the beginning of the course showed no relationship with task performance at the end. This may be because the relevance or importance of the information at the beginning of the course may not be well understood by students, and thus have little impact on their performance towards the end. In other words, students may not recognize the importance of certain topics or concepts until they encounter study-related tasks or assessments where this information is necessary or relevant. Students may also not have had the opportunity to process, integrate, or apply the study material with existing knowledge, thus making it difficult to recall or apply at a later stage (Van Zyl et al., 2021a). Furthermore, the role of information availability in predicting task performance may also be moderated by other individual- (e.g., motivation, self-efficacy, the perceived value of the tasks), university- (e.g., class structure, course content, first generation vs. non-first-generation students) or contextual factors (e.g., cultural differences) not measured as part of this study (Lesener et al., 2020). Thus, while information availability may be deemed a necessary condition for completing academic tasks, it may not be sufficient to predict task performance over time.
While this study provides valuable insights into the psychometric properties, temporal stability, and criterion validity of the SDRS, several limitations must be considered. These limitations provide opportunities for future research. First, although a relatively large cross-national sample was obtained, the majority of the participants were from the European education system which may have skewed results. The educational systems within the USA, Hong Kong, and India differ from those in Europe. At the European level, there is strategic alignment on educational units, learning outcomes, and educational strategies, making the students from European universities within this sample rather homogenous. Differences in educational design, tuition methods, available lecturers, and the like may significantly impact how study characteristics are perceived. A more representative sample is required for future research. Second, the majority of the participants were relatively young (ages 18–23), presumably still busy with their undergraduate training. Undergraduate and postgraduate students are faced with different demands, whereby the latter may already have developed various personal resources which may affect how study demands and resources are perceived (Van Zyl et al., 2021a). Future research should attempt to diversify the sample to include a balance between post- and undergraduate students and the differences between these two groups need to be investigated. Third, the SDRS may not have been sensitive enough to capture the nuances of specific study characteristics like peer support, lecturer support, and information availability at the start of a new course. It is recommended that the questions be formulated to measure the perceived importance of these elements rather than the current experience of such in the moment when the SDRS is used as a baseline measure. Fourth, while the overall study characteristics factor was a strong predictor of study engagement and task performance, the specific factors were found to have a limited predictive capacity. Future research should employ other exogenous factors such as stress or motivation as a means to explore the scale’s criterion validity. Finally, Fifth, although measurement invariance was demonstrated over time, the study only assessed reliability at two time points. This may not be sufficient to fully establish the scale’s temporal stability. Future research should aim to assess and compare reliability on a weekly basis throughout the duration of a course/semester. Finally, since the relationship between study characteristics, motivational factors and performance outcomes are moderated by personal demands (e.g., academic boredom) and personal resources (e.g., grit and resilience), we suggest that future studies test and control for such in order to have a more nuanced understanding of how study demands/resources translate into important study related outcomes.
Our results support the notion that overall study characteristics should be seen and measured as a dynamic interaction between study demands and resources rather than a hierarchical model where demands and resources are classified in isolation from one another. The findings further showed that the SDRS is a valid and reliable measure of overall study characteristics (demands/resources), and that it is associated with study engagement and task performance cross-sectionally, and longitudinally. However, the limited contribution of the specific factors of the scale to the prediction of study engagement and task performance should be taken into account when interpreting the results. The SDRS can thus be a useful tool for educators interested in understanding the factors associated with student success. Nevertheless, researchers should show further caution when modelling data obtained by the SDRS through traditional independent cluster modelling techniques, as constraining cross-loadings to zero may lead to biased results. This implies that the straightforward use of the SDRS as an instrument is limited, and that manual scoring may be problematic/complex. In contrast, ESEM modelling approaches may address the limitations of the independent cluster modelling approaches by controlling- and compensating for cross-national differences in the interpretation of the SDRS’s items and for differences in educational environments in cross-institutional studies. In conclusion, this contributes to research on the psychometric properties and criterion validity of the SDRS, but more research is needed to establish its practical usefulness and generalizability.
The data analyzed in this study is subject to the following licenses/restrictions: the data contains sensitive personal data which is not permitted to be published in open forums. Requests to access these datasets should be directed to LZ bGxld2VsbHluMTAxQGdtYWlsLmNvbQ==.
The studies involving humans were approved by Georgia Southern University & KU Leuven. The studies were conducted in accordance with the local legislation and institutional requirements. The participants provided their written informed consent to participate in this study. Written informed consent was obtained from the individual(s) for the publication of any potentially identifiable images or data included in this article.
LZ: Conceptualization, Data curation, Formal analysis, Funding acquisition, Investigation, Methodology, Project administration, Resources, Software, Supervision, Validation, Visualization, Writing – original draft, Writing – review & editing. RS: Data curation, Writing – review & editing. JK: Data curation, Writing – review & editing. NV: Data curation, Writing – review & editing. SR: Conceptualization, Data curation, Writing – review & editing. VC: Data curation, Writing – review & editing. KF: Data curation, Writing – review & editing. ES-T: Writing – review & editing. LR: Data curation, Writing – review & editing. AG: Data curation, Writing – review & editing. LM: Data curation, Writing – review & editing. DA: Data curation, Writing – review & editing. MC: Data curation, Writing – review & editing. JS: Data curation, Writing – review & editing. IH: Writing – review & editing. ZB: Writing – review & editing. LB: Supervision, Writing – review & editing.
The author(s) declare that no financial support was received for the research, authorship, and/or publication of this article.
The authors declare that the research was conducted in the absence of any commercial or financial relationships that could be construed as a potential conflict of interest.
The author(s) declared that they were an editorial board member of Frontiers, at the time of submission. This had no impact on the peer review process and the final decision.
All claims expressed in this article are solely those of the authors and do not necessarily represent those of their affiliated organizations, or those of the publisher, the editors and the reviewers. Any product that may be evaluated in this article, or claim that may be made by its manufacturer, is not guaranteed or endorsed by the publisher.
The Supplementary material for this article can be found online at: https://www.frontiersin.org/articles/10.3389/feduc.2024.1409099/full#supplementary-material
1. ^It is important to note that the relationship between study demands, study resources and engagement/performance is moderated by numerous factors like personal resources (e.g., grit and resilience) and personal demands (e.g., academic boredom).
2. ^To conserve in text space, only the models that fitted the data best at both Time 1 and Time 2 are discussed.
Asparouhov, T., and Muthén, B. (2009). Exploratory structural equation modelling. Struct. Equ. Model. Multidiscip. J. 16, 397–438. doi: 10.1080/10705510903008204
Bakker, A. B., and Demerouti, E. (2007). The job demands-resources model: state of the art. J. Manag. Psychol. 22, 309–328. doi: 10.1108/02683940710733115
Basson, M. J., and Rothmann, S. (2019). Pathways to flourishing among pharmacy students: the role of study demands and lecturer support. J. Psychol. Afr. 29, 338–345. doi: 10.1080/14330237.2019.1647953
Chen, F. F. (2007). Sensitivity of goodness of fit indexes to lack of measurement invariance. Struct. Equ. Model. Multidiscip. J. 14, 464–504. doi: 10.1080/10705510701301834
Cheng, C. (2020). Duration matters: peer effects on academic achievement with random assignment in the Chinese context. J. Chin. Sociol. 7, 1–20. doi: 10.1186/s40711-020-0114-0
Christenson, S., Reschly, A. L., and Wylie, C. (2012). Handbook of research on student engagement, vol. 840. New York: Springer.
Demerouti, E., Bakker, A. B., Nachreiner, F., and Schaufeli, W. B. (2000). A model of burnout and life satisfaction amongst nurses. J. Adv. Nurs. 32, 454–464. doi: 10.1046/j.1365-2648.2000.01496.x
Harrer, M., Adam, S. H., Baumeister, H., Cuijpers, P., Karyotaki, E., Auerbach, R. P., et al. (2019). Internet interventions for mental health in university students: a systematic review and meta-analysis. Int. J. Methods Psychiatr. Res. 28:e1759. doi: 10.1002/mpr.1759
Harrer, M., Adam, S. H., Fleischmann, R. J., Baumeister, H., Auerbach, R., Bruffaerts, R., et al. (2018). Effectiveness of an internet-and app-based intervention for college students with elevated stress: randomized controlled trial. J. Med. Internet Res. 20:9293. doi: 10.2196/jmir.9293
Hayes, A. F., and Coutts, J. J. (2020). Use omega rather than Cronbach’s alpha for estimating reliability. But…. Commun. Methods Meas. 14, 1–24. doi: 10.1080/19312458.2020.1718629
Hu, L. T., and Bentler, P. M. (1999). Cutoff criteria for fit indexes in covariance structure analysis: conventional criteria versus new alternatives. Struct. Equ. Model. Multidiscip. J. 6, 1–55. doi: 10.1080/10705519909540118
JASP. (2021). Jeffery’s Amazing Stats Program (v.0.15). Available at: https://jasp-stats.org
Kember, D. (2004). Interpreting student workload and the factors which shape students’ perceptions of their workload. Stud. High. Educ. 29, 165–184. doi: 10.1080/0307507042000190778
Kline, R. B. (2010). Principles and practices of structural equation modelling. 3rd Edn. London, UK: Gilford Press.
Koopmans, L., Bernaards, C., Hildebrandt, V., Van Buuren, S., Van der Beek, A. J., and De Vet, H. C. (2012). Development of an individual work performance questionnaire. International journal of productivity and performance management, 62, 6–28.
Krifa, I., van Zyl, L. E., Braham, A., Ben Nasr, S., and Shankland, R. (2022). Mental health during COVID-19 pandemic: the role of optimism and emotional regulation. Int. J. Environ. Res. Public Health 19:1413. doi: 10.3390/ijerph19031413
Lesener, T., Gusy, B., and Wolter, C. (2019). The job demands-resources model: a meta-analytic review of longitudinal studies. Work Stress 33, 76–103. doi: 10.1080/02678373.2018.1529065
Lesener, T., Pleiss, L. S., Gusy, B., and Wolter, C. (2020). The study demands-resources framework: An empirical introduction. Int. J. Environ. Res. Public Health 17:5183. doi: 10.3390/ijerph17145183
Magada, T., and Govender, K. K. (2017). Culture, leadership and individual performance: a south African public service organisation study. J. Econ. Manag. Theory 1, 1–2. doi: 10.22496/jemt20161104109
Marsh, H. W., Morin, A. J., Parker, P. D., and Kaur, G. (2014). Exploratory structural equation modelling: An integration of the best features of exploratory and confirmatory factor analysis. Annu. Rev. Clin. Psychol. 10, 85–110. doi: 10.1146/annurev-clinpsy-032813-153700
Marsh, H. W., Muthén, B., Asparouhov, T., Lüdtke, O., Robitzsch, A., Morin, A. J., et al. (2009). Exploratory structural equation modelling, integrating CFA and EFA: application to students’ evaluations of university teaching. Struct. Equ. Model. Multidiscip. J. 16, 439–476. doi: 10.1080/10705510903008220
McNeish, D., An, J., and Hancock, G. R. (2018). The thorny relation between measurement quality and fit index cutoffs in latent variable models. J. Pers. Assess. 100, 43–52. doi: 10.1080/00223891.2017.1281286
Mokgele, K. R., and Rothmann, S. (2014). A structural model of student wellbeing. S. Afr. J. Psychol. 44, 514–527. doi: 10.1177/0081246314541589
Morin, A. J., Arens, A. K., and Marsh, H. W. (2016). A bifactor exploratory structural equation modelling framework for the identification of distinct sources of construct-relevant psychometric multidimensionality. Struct. Equ. Model. Multidiscip. J. 23, 116–139. doi: 10.1080/10705511.2014.961800
Morin, A. J. S., Myers, N. D., and Lee, S. (2020). Modern factor analytic techniques: Bifactor models, exploratory exploratory structural equation modelling 19 structural equation modelling and bifactor-ESEM. G. Tenenbaum and R. C. Eklund (Eds.), Handbook of sport psychology, 4th edn, 2, 1044–1073. Wiley Publishers
Mtshweni, V. B. (2019). The effects of sense of belonging adjustment on undergraduate students’ intention to dropout of university [Unpuiblished doctoral dissertation]. Pretoria: University of South Africa.
Ouweneel, E., Le Blanc, P. M., and Schaufeli, W. B. (2011). Flourishing students: a longitudinal study on positive emotions, personal resources, and study engagement. J. Posit. Psychol. 6, 142–153. doi: 10.1080/17439760.2011.558847
Ryan, R. M., and Deci, E. L. (2000). Intrinsic and extrinsic motivations: classic definitions and new directions. Contemp. Educ. Psychol. 25, 54–67. doi: 10.1006/ceps.1999.1020
Schaufeli, W. B., and Bakker, A. B. (2004). Job demands, job resources, and their relationship with burnout and engagement: a multi-sample study. J. Organizational Behav. 25, 293–315. doi: 10.1002/job.248
Schaufeli, W. B., Bakker, A. B., and Salanova, M. (2006). The measurement of work engagement with a short questionnaire: a cross-national study. Educ. Psychol. Meas. 66, 701–716. doi: 10.1177/0013164405282471
Schaufeli, W. B., Bakker, A. B., and Van Rhenen, W. (2009). How changes in job demands and resources predict burnout, work engagement, and sickness absenteeism. Journal of Organizational Behavior: The International Journal of Industrial, Occupational and Organizational Psychology and Behavior, 30, 893–917.
Stallman, H. M., and Kavanagh, D. J. (2020). Development of an internet intervention to promote wellbeing in college students. Aust. Psychol. 53, 60–67. doi: 10.1111/ap.12246
Sweller, J. (2011). Cognitive load theory. J. P. Mestre and B. H. Ross (Eds.), Psychology of learning and motivation, 37–76. Academic Press
van der Ross, R. M., Olckers, C., and Schaap, P. (2022). Student engagement and learning approaches during COVID-19: the role of study resources, burnout risk, and student leader-member exchange as psychological conditions. Higher Learn. Res. Commun. 12, 77–109. doi: 10.18870/hlrc.v12i0.1330
Van Zyl, L. E. (2021). Social study resources and social wellbeing before and during the intelligent COVID-19 lockdown in the Netherlands. Soc. Indic. Res. 157, 393–415. doi: 10.1007/s11205-021-02654-2
Van Zyl, L. E., Arijs, D., Cole, M. L., Glinska, A., Roll, L. C., Rothmann, S., et al. (2021b). The strengths use scale: psychometric properties, longitudinal invariance and criterion validity. Front. Psychol. 12:676153. doi: 10.3389/fpsyg.2021.676153
Van Zyl, L. E., Dik, B. J., Donaldson, S. I., Klibert, J. J., Di Blasi, Z., Van Wingerden, J., et al. (2024a). Positive organisational psychology 2.0: embracing the technological revolution. J. Posit. Psychol., 1–13,
Van Zyl, L. E., Gaffaney, J., van der Vaart, L., Dik, B. J., and Donaldson, S. I. (2023). The critiques and criticisms of positive psychology: a systematic review. J. Posit. Psychol. 19, 206–235. doi: 10.1080/17439760.2023.2178956
van Zyl, L. E., Heijenk, B., Klibert, J., Shankland, R., Verger, N. B., Rothmann, S., et al. (2022). Grit across nations: an investigation into the cross-cultural equivalence of the grit-O scale. J. Happiness Stud. 23, 3179–3213. doi: 10.1007/s10902-022-00543-0
Van Zyl, L. E., Klibert, J., Shankland, R., Stavros, J. M., Cole, M., Verger, N. B., et al. (2024b). The academic task performance scale: psychometric properties, and measurement invariance across ages, genders and nations. Front. Educ. 9, 1–11. doi: 10.3389/feduc.2024.1281859
Van Zyl, L. E., Rothmann, S., and Zondervan-Zwijnenburg, M. A. J. (2021a). Longitudinal trajectories of study characteristics and mental health before and during the COVID-19 lockdown. Front. Psychol. 12, 1–13. doi: 10.3389/fpsyg.2021.633533
Van Zyl, L. E., and Ten Klooster, P. M. (2022). Exploratory structural equation modelling: practical guidelines and tutorial with a convenient online tool for mplus. Front. Psychiatry 12:795672, 1–13. doi: 10.3389/fpsyt.2021.795672
Von Eye, A. (1990). Statistical methods in longitudinal research: Principles and structuring change, vol. 1: Elsevier.
Wang, M.-T., and Eccles, J. S. (2012). Social support matters: longitudinal effects of social support on three dimensions of school engagement from middle to high school. Child Dev. 83, 877–895. doi: 10.1111/j.1467-8624.2012.01745.x
Keywords: psychometric properties, longitudinal invariance and criterion validity study demands, study resources, exploratory structural equation modelling, university students
Citation: van Zyl LE, Shankland R, Klibert J, Verger NB, Rothmann S, Cho V, Feng K, See-To EWK, Roll LC, Ghosh A, van der Meij L, Arijs D, Cole ML, Stavros JM, Hulshof IL, Di Blasi Z and De Beer LT (2024) The study demands and resources scale: psychometric properties, longitudinal invariance, and criterion validity. Front. Educ. 9:1409099. doi: 10.3389/feduc.2024.1409099
Received: 29 March 2024; Accepted: 13 May 2024;
Published: 29 May 2024.
Edited by:
Wanderson Silva, São Paulo State University, BrazilReviewed by:
Miriane Zucoloto, University of São Paulo, BrazilCopyright © 2024 van Zyl, Shankland, Klibert, Verger, Rothmann, Cho, Feng, See-To, Roll, Ghosh, van der Meij, Arijs, Cole, Stavros, Hulshof, Di Blasi and De Beer. This is an open-access article distributed under the terms of the Creative Commons Attribution License (CC BY). The use, distribution or reproduction in other forums is permitted, provided the original author(s) and the copyright owner(s) are credited and that the original publication in this journal is cited, in accordance with accepted academic practice. No use, distribution or reproduction is permitted which does not comply with these terms.
*Correspondence: Llewellyn E. van Zyl, TGxld2VsbHluMTAxQGdtYWlsLmNvbQ==
Disclaimer: All claims expressed in this article are solely those of the authors and do not necessarily represent those of their affiliated organizations, or those of the publisher, the editors and the reviewers. Any product that may be evaluated in this article or claim that may be made by its manufacturer is not guaranteed or endorsed by the publisher.
Research integrity at Frontiers
Learn more about the work of our research integrity team to safeguard the quality of each article we publish.