- 1College of Education, Arts, and Sciences at Danao Campus, Cebu Technological University, Danao, Philippines
- 2Graduate School at Danao Campus, Cebu Technological University, Danao, Philippines
- 3Educational Research and Resource Center, Cebu Technological University, Danao, Philippines
In the ever-evolving landscape of educational technology, it is vital to understand the empirical relationships of teachers' Technological Pedagogical and Content Knowledge (TPACK) and its interplay with explanatory factors such as cyber wellness, school climate, and digital nativity. This study employs Covariance-Based Structural Equation Modeling (CB-SEM) to analyze self-reported data from 311 basic education teachers in Central Visayas, Philippines. The model demonstrates robust validity and reliability, showing diverse direct impacts on the antecedent variables to the seven constructs of TPACK. The findings reveal 16 significant hypothesized paths, with digital nativity has a significant effect on all three core knowledge domains of TPACK while school climate and cyber wellness have a significant effect on two knowledge domains. Another significant finding shows that content knowledge emerged with the highest significant effect on technological content knowledge (β = 0.621). This connection highlights the fundamental role of content knowledge in successfully integrating technological competencies among teachers, especially from a developing economy. The study provides policy insights tailored to post-pandemic educational management.
1 Introduction
The educational landscape has undergone radical changes from the onslaught of the global pandemic, adapting to the new normal in teaching and learning. The changes are brought about by the technological advances that require digitizing teaching materials, affecting how teachers teach and learners learn (Núñez-Canal et al., 2022). Thus, educators play critical roles in the new teaching model, confirming the necessity of revisiting their knowledge of technology and updating pedagogical skills to digitize teaching materials successfully. The new normal challenges include a steadfast balancing of technology and pedagogy with the precise realignment of the content that supports learning opportunities that were not possible before but can be effectively delivered in present situations (Rapanta et al., 2021). The crisis that the pandemic has instigated urged educators, especially in teacher education programs, to rethink their roles in integrating technology into design processes and learning outcomes. Particular attention must be paid to developing technological knowledge for basic education teachers, especially since they are dealing with learners in the next generation mainly affected by rapid technological changes.
While the education sector struggles to prepare their basic education teachers to integrate appropriate technology, literature has reported successful appraisal of teachers' technological skills, reflecting their ability to include digital materials in teaching. For example, Schmid et al. (2021) outlined self-reported technological, pedagogical, and content knowledge (TPACK) with coded technological inclusions in teachers' lesson planning. According to Nelson and Voithofer (2022), coursework could effectively predict teachers' behavior toward digitalizing materials via the TPACK framework. The TPACK, introduced by Mishra and Koehler (2006), is a framework of knowledge theorized by making connections among teachers' technological knowledge (TK), pedagogical knowledge (PK), and content knowledge (CK). From the three core knowledge bases, four constructs are formed in the intersections; these are the technological pedagogical knowledge (TPK), pedagogical content knowledge (PCK), technological content knowledge (TCK), and the innermost intersection TPACK. These are discussed in detail in the literature review and methods sections. The TPACK framework's components have been explored in emerging literature (e.g., Zhang and Chen, 2022; Celik, 2023) using structural equation modeling (SEM).
Despite the framework's overwhelming success, the mere acquisition of technological, pedagogical, and content knowledge does not always lead to technology integration (Polly et al., 2010). A closer look at possible antecedent variables that may add to the discussions of teachers' TPACK is critically argued in this study. For example, school climate (SC) and technology integration in a social and educational environment in the school are important factors that may create a positive setting for learning, academic achievement, and student growth amidst rapid technological changes (Yildiz, 2017; Raygan and Moradkhani, 2022). These include the adequacy of technological resources and administrative support, online facilities, technical and pedagogical support sufficiency, and the convenience of using technological devices. Schools aim to provide learners with classrooms equipped with technology, and teachers who can use the tools must also have aligned skills to implement the program successfully. SC plays a vital role in the technology integration process. In its most general sense, educators have reached a consensus that the appropriateness of technology and management support, online resources and platforms, the sufficiency of pedagogical provision, and the ease of use of technological devices are critical concerns affecting the technology integration (Raygan and Moradkhani, 2022).
Another antecedent latent factor explored in this paper is digital nativity (DN). Digital nativity refers to individuals who have grown up with and are highly proficient in digital technology and can navigate various digital tools and platforms quickly and fluently (Prensky, 2001). It is argued that digital natives, which include most of these day basic education teachers, do not automatically convert their DN characteristics into something useful in the classroom (Kabakci Yurdakul, 2018). Although in a general context, digital natives' innate familiarity with technology enhances their technological knowledge and extends to their pedagogical understanding since they can control their intuitive grasp of digital systems to effectively integrate technology into teaching practices (Bender, 2023). Thus, although the hypothetical path from DN to the latent factors of TPACK is not yet explored in the current literature, these examples provide a scholarly basis for adding DN as an antecedent variable that could potentially affect teachers' TPACK.
On the other hand, as the new normal is more concerned about responsible technology users, cyber-wellness (CW) constructively argued in this paper to have a plausible relationship with teachers' TPACK. CW promotes wellbeing and safety in the digital world and elevates these concepts to a more balanced level (Searson et al., 2015). It covers practices and behaviors that ensure mental, emotional, and physical health while engaging with digital technologies, including the Internet and social media platforms. Most digital natives only have a medium level of awareness concerning safe and responsible Internet use and values toward protection in the cyber world (Mihci Türker and Kiliç Çakmak, 2019). These attributes are subsumed under one heading of CW. The definition of CW can also be applied to the growing importance of ICT in modern society and its associated threats, especially to young children. There is a need to balance the use of technology (e.g., touch screen applications) and traditional concrete teaching tools (e.g., hand manipulatives) to lessen young learners' digital media exposure (Gonzales, 2022). These threats include inappropriate disclosure of important and private information, potentially dangerous information, cyberbullying, sex solicitation, online email scams, and Internet fraud (Meena et al., 2021). While educators see the benefits of incorporating ICT into their classrooms, the potential cyber hazards and a lack of CW knowledge training may affect the teachers' TPACK.
Thus, the primary intention of this work is to connect the gaps in the empirical evidence in the current literature explaining the teachers' TPACK with the inclusion of the identified variables using covariance-based structural equation modeling (CB-SEM). In particular, we extend the Mishra and Koehler (2006) TPACK framework and offer a more inclusive path model with SC, DN, and CW as antecedent constructs to induce critical discussions on how to deal with the challenges of the new normal. We find these latent constructs necessary in the debates about digitalization in education, especially in developing economies where technological infrastructure challenges are prevalent.
The rest of the paper is organized as follows: Section 2 presents the literature review, Section 3 presents the hypothesis development, and Section 4 describes methodological procedures. Section 5 reports the result of the SEM analysis with the model specification through confirmatory factor analysis and the evaluation of the structural model. Section 6 offers the discussion, while Section 7 provides the conclusion.
2 Literature review
This section provides the foundational literature necessary to substantiate the arguments presented in the proposed model. The discussion begins with a detailed review of the TPACK framework. Subsequently, it focuses on identifying and establishing the fundamental factors that underpin the gap that we wish to convey regarding the delineation of the path model.
2.1 TPACK framework
Teacher expertise as a distinct form of knowledge was first introduced by Shulman (1986), who termed it “pedagogical content knowledge”. It is argued that teachers' pedagogical and content knowledge are closely connected and should not be considered separately. Mishra and Koehler (2006) expanded on this idea by incorporating technology and exploring its impact on classrooms. They introduced the term TPACK to describe teachers' unique expertise in integrating technology, illustrating how they use technology thoughtfully in teaching (Koehler et al., 2007). TPACK was initially called TPCK, but later, it was changed for more straightforward pronunciation and to emphasize the integrated use of technology, pedagogy, and content knowledge for effective technology integration (Thompson and Mishra, 2007).
Mishra and Koehler (2006) developed a visual representation of their TPACK framework, illustrating the interplay between technological knowledge, pedagogical knowledge, and content knowledge (refer to Figure 1). These three knowledge domains—technological, pedagogical, and content knowledge—are depicted as three distinct circles in their visual representation. The points where they converge form four overlapping areas, illustrating the dynamic interplay between these crucial facets of teaching expertise. This depiction is an influential visual symbol for the complex fusion of knowledge required for effective teaching in the digital age. For detailed definitions of the seven TPACK components, refer to Table 1.
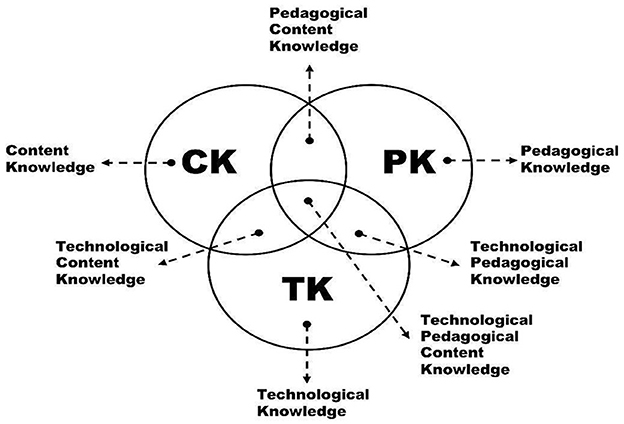
Figure 1. TPACK schema (Harris et al., 2009).
2.2 Antecedent variables for the TPACK framework
The argument highlighting the dearth of literature addressing specific and overarching variations within the TPACK framework is outlined in the subsequent sub-sections. Each latent construct is methodically extracted from established and emerging research, offering an articulate comprehension of the knowledge gap this paper aims to address.
2.2.1 School climate
School climate encompasses educators' shared perceptions of their working environment, including its distinguishing features and impact on staff behavior (Hoy, 1990). A positive school climate encourages collaboration, support, and enthusiasm for technology integration, facilitating educators in enhancing their TPACK competencies (Zakariya, 2020). Existing research suggests that when teachers perceive a supportive climate for technology integration, they are more likely to employ effective pedagogical practices that leverage technology tools (Raygan and Moradkhani, 2022). This aligns with the core principles of the TPACK framework, emphasizing integrating technology, pedagogy, and content knowledge for optimal teaching and learning outcomes.
2.2.2 Digital nativity
Digital nativity, a term coined by Prensky (2001), refers to individuals who have grown up with and are highly proficient in digital technology. It is recognized as a vital factor within the TPACK framework, as emphasized by Kabakci Yurdakul (2018). This concept aligns closely with TPACK's emphasis on integrating technology, pedagogy, and content knowledge. As digital natives, this generation possesses unique skills and experiences that significantly influence their understanding and utilization of technology in educational settings. Their advanced information and communication technology (ICT) skills enable them to navigate and control digital tools effortlessly (Helsper and Eynon, 2010). This familiarity with technology empowers digital natives to explore innovative teaching practices, employ digital resources, and adapt to emerging technological advancements, all of which are essential components of the TPACK framework (Orlando and Attard, 2016).
2.2.3 Cyber wellness
Cyber wellness encompasses the knowledge, skills, and values essential for safeguarding Internet users and promoting responsible technology use (Mihci Türker and Kiliç Çakmak, 2019). Additionally, it involves attitudes and wellbeing related to Internet usage and self-protection in the digital sphere (Shaikh et al., 2021). As the demand for technology, technology users, and technology integration in education continues to escalate, recent research has brought to light the growing concerns and challenges teachers face concerning cyber wellness issues (Lewin et al., 2021). Within the TPACK framework, understanding and addressing cyber wellness become paramount for teachers in effectively integrating technology into their pedagogy and content knowledge, ensuring safe and responsible technology use for themselves and their students.
3 Hypothesis development
The theoretical foundation of the study model aimed to introduce and verify various hypothetical paths rooted in Mishra and Koehler's (2006) TPACK framework. The main objective is to establish teachers' perceived TPACK trajectories as explained by the identified antecedent factors such as cyber wellness, school climate, and digital nativity. The proposed model is presented in Figure 2.
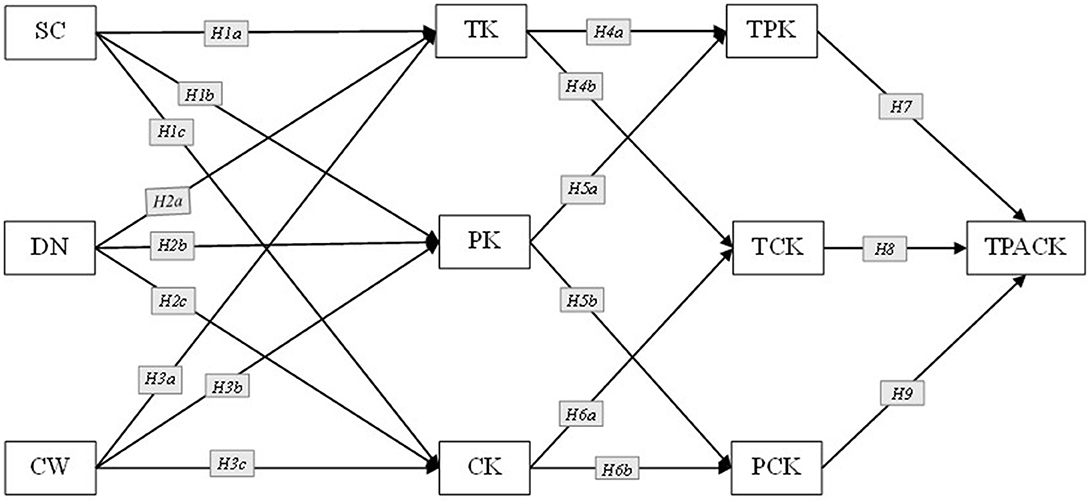
Figure 2. The proposed model. SC, school climate; DN, digital nativity; CW, cyber wellness; TK, technology knowledge; PK, pedagogical knowledge; CK, content knowledge; TPK, technological pedagogical knowledge; TCK, technological content knowledge; TPACK, technological pedagogical and content knowledge.
3.1 The hypothetical paths with the SC, DN, and CW
The importance of school climate in facilitating effective technology integration has been highlighted in recent literature. Various aspects of school climate, including technical support (Chen, 2008; Naima, 2017), technical infrastructure and resources (Chen, 2008), instructional assistance, and environmental support (Chen, 2008; Alghasab et al., 2020), have been identified as significant contributors. A study by Raygan and Moradkhani (2022) further emphasizes the role of school climate in explaining classroom technology integration. Their findings suggest that when teachers experience an encouraging and supportive school climate, they are more likely to develop favorable attitudes toward digital technologies. This, in turn, increases their comfort level in accepting and integrating technology into their classrooms. Based on these insights, the following hypotheses are proposed: H1a: SC will significantly and positively impact TK. H1b: SC will significantly and positively impact PK. H1c: SC will significantly and positively impact CK.
Digital competencies correlate positively to TPACK competencies (Çebi et al., 2022). Consequently, the roles of digital competencies should be considered for successful technology integration. Kabakci Yurdakul (2018) indicates that digital nativity significantly predicts TPACK competence. This suggests that increased digital nativity will almost certainly increase perceived TPACK competency. Such an investigation leads to a broader understanding of digital natives, particularly on the following hypotheses: H2a: DN will significantly and positively impact TK. H2b: DN will significantly and positively impact PK. H2c: DN will significantly and positively impact CK.
Cyber wellness predicts teachers' TPACK when designing learning competencies using online platforms (Chai et al., 2012). Access to and effective use of digital age ICT are becoming more crucial to the educational agenda in formal and informal school settings, indicating that cyber wellness is a more holistic and balanced approach (Searson et al., 2015). Thus, we identify cyber wellness knowledge as an explanatory factor for TPACK. Premised on these arguments, we propose the following hypotheses: H3a: CW will significantly and positively impact TK. H3b: CW will significantly and positively impact PK. H3c: CW will significantly and positively impact CK.
3.2 The hypothetical paths with the TK, PK, and CK
Mishra and Koehler (2006) illustrate that TK refers to the teachers' knowledge of various technologies ranging from low technology (i.e., textbook lectures) to high technology (online resources, digital platforms, learning management systems, etc.). This knowledge also included the ability to apply technologies in teaching and learning processes (Mishra and Koehler, 2006). Wang (2019) showed that TK could work with TPK and TCK to integrate better technology programs to support curriculum improvements related to teacher knowledge and teaching methods. This technology adoption and integration into teaching and learning environments enable teachers and students to succeed in a globalized digital age (Lawrence and Tar, 2018). On the other hand, TK was found to have a positive and significant impact on TPK and TCK mathematics teachers regarding their acceptance of online professional development (Mailizar et al., 2021). Based on these findings, we propose the following hypotheses: H4a: TK will significantly and positively impact TPK. H4b: TK will significantly and positively impact TCK.
PK refers to the teachers' specialized skills and knowledge in effective teaching-learning experiences in the intended course content, which is associated with teaching strategies and methodologies implemented in the classroom (Chai et al., 2010). Accordingly, PK contains the ability to know and use methods or techniques to help students master the content of the curriculum in the classroom (Wang, 2019). Chai et al. (2012) reported that PK significantly influences preservice teachers' TPK. Likewise, in a study conducted by Pamuk et al. (2015), PK was also significant to TPK. Dong et al. (2015) asserted that both TPK and PCK were significantly affected by PK. In a recent study by Mailizar et al. (2021), PK was found to have a positive and significant impact on the TPK and PCK of mathematics teachers regarding their acceptance of online professional development. Thus, we propose the following hypotheses: H5a: PK will significantly and positively impact TPK. H5b: PK will significantly and positively impact PCK.
CK is the knowledge of the subject matter that can be considered and presented in teaching or learning (Mishra and Koehler, 2006). Content knowledge is important for teachers because it gives basic concepts, principles, and teaching methods. CK varies in various areas; however, teaching the course requires a deep understanding of the knowledge needed in a particular field (Wang, 2019). In this study, CK is important in predicting the two secondary knowledge bases, TCK and PCK (Dong et al., 2015; Pamuk et al., 2015). Dong et al. (2015) showed that CK significantly affected TCK. Meanwhile, TCK and PCK have been confirmed to influence CK significantly (Pamuk et al., 2015). CK was found to have a positive and significant impact on TCK and TPK mathematics teachers regarding their acceptance of online professional development (Mailizar et al., 2021). Thus, we propose the following hypotheses: H6a: CK will significantly and positively impact TCK. H6b: CK will significantly and positively impact PCK.
3.3 The hypothetical paths with the TPK, TCK, PCK
TPK encompasses the application of technology in teaching, resulting in transformative pedagogical methods that enhance learning effectiveness (Mishra and Koehler, 2006). When combined with pedagogical and technological knowledge, curriculum development becomes more specialized and effective in addressing students' needs (Gonzales et al., 2020). As revealed in previous studies, TPK was significantly correlated with TPACK (e.g., Pamuk et al., 2015). Conversely, TCK pertains to the adept use of technology to convey specific content domains (Schmidt et al., 2009). The TPK is associated with TPACK in several contexts. For example, in the field of hospitality, tourism, and leisure, students must use the technology provided in the classroom to gain sufficient knowledge of the curriculum they are studying (Wang, 2019). The affected domain of teachers in the case of TPK on using mobile Internet in teaching is significantly associated with the teachers' TPACK (Nikolopoulou et al., 2021). Santos and Castro (2021) examined how preservice teachers implemented TPACK in public schools with available EdTech, revealing significant associations between TCK and TPK with TPACK. In a recent study by Mailizar et al. (2021), TPK, TCK, PCK were found to have a positive and significant impact on TPACK of mathematics teachers regarding their acceptance of online professional development. Thus, we hypothesize the following. H7: TPK will significantly and positively impact TPACK. H8: TCK will significantly and positively impact TPACK. H9: PCK will significantly and positively impact TPACK.
4 Methodology
The paper utilized a quantitative survey research approach, utilizing multivariate data analysis of self-reported responses. The methodology is outlined in participants, instrument development, and data analysis.
4.1 Participants
The participants in this study comprised elementary and secondary school teachers from public and private educational institutions in the Central Visayas region of the Philippines. This region in the Philippines is composed of four island provinces. Online invitations, including the e-questionnaire URL, were sent to participants using snowball sampling. Three hundred fifty basic education teachers completed the questionnaires, and 311 usable questionnaires were retained and used for further analysis. Thirty-nine were removed based on duplication, failure to hold the sincerity tests (non-permissible homogeneity of the response, i.e., SD<0.5), and disqualification to the inclusion-exclusion criteria (e.g., regional assignment, non-teaching staff) of the study.
Table 2 provides an overview of the participant demographics (N = 311). This table presents the distribution of participants based on their gender, teaching grade levels, digital nativity, and highest educational qualifications. The data indicates that 19.94% of participants were male, while 79.74% were female. Concerning teaching grade levels, 36.01% were elementary school teachers, 46.20% taught at the junior high school level, and 14.79% were senior high school teachers. The majority of participants (74.77%) belonged to the digital native category (age ≤ 40), while 25.72% were classified as non-digital natives (age > 41). Regarding the highest educational attainment, 68.49% of participants held a bachelor's degree, 15.43% had completed master's units, 13.83% held a master's degree, and a smaller percentage (1.29%) had taken doctorate units, with an even smaller percentage (0.96%) holding a doctorate.
4.2 Development of survey instrument
We developed the instrument based on the indicators used by existing studies, which were contextualized to make it suitable to the nature of the respondents' demography (see Supplementary Appendix A). The TPACK indicators were adapted from the work of Schmid et al. (2020), whose scale was technically named TPACK.xs. It consists of 28 items containing seven sections, each representing one of the knowledge domains in the TPACK framework. The indicators can evaluate the extent to which teachers possess the multifaceted knowledge required for effective technology integration in education (Schmid et al., 2020). This assessment helps identify areas of strength and areas for improvement, guiding professional development efforts and informing policy decisions aimed at enhancing technology-enhanced teaching and learning practices. Current research often applies the TPACK indicators to explore various aspects of technology integration in education, such as teachers' technostess (Özgür, 2020), computer self-efficacy and school support (Dong et al., 2020), and digital lesson planning (Schmid et al., 2021).
The indicators of the antecedent factors of the school climate construct were adopted from Papanastasiou and Angeli (2008) and Raygan and Moradkhani (2022). The school climate criterion includes eight items that assess the school climate and support the use of technology. The work of Chai et al. (2012) comprised the development of cyber wellness indicators. The digital nativity questionnaire was adopted from the papers of Kabakci Yurdakul (2018) and Teo (2013), which they operationally call the digital nativity assessment scale (DNAS).
The indicators of the SC provide insights into the overall atmosphere and support for technology integration within educational institutions, providing a conducive environment for utilizing technology in teaching and learning practices. CW indicators assess the wellbeing and safety in the digitalization of teaching, capturing the healthy digital behaviors among the teacher respondents. Furthermore, the DN questionnaire evaluates the teachers' familiarity and proficiency with digital technologies, identifying their readiness to navigate digital tools effectively.
4.2.1 Technological knowledge
TK was assessed using the well-established five-item scale by Schmidt et al. (2009). Sample items include “I keep up with important new technologies,” “I often experiment with technology,” and “I possess the necessary technical skills for technology use.” Respondents rated these statements on a five-point scale ranging from “strongly agree” (5) to “strongly disagree” (1). The scale's internal consistency, evaluated using Cronbach's alpha, was 0.745.
4.2.2 Pedagogical knowledge
The following are examples of the items measuring the PK (Schmidt et al., 2009; Schmid et al., 2020): “I can adapt my teaching based upon what students currently understand or do not understand,” and “I can change my teaching style to different learners.” The items are answered along a five-point scale ranging from “strongly agree” (5) to “strongly disagree” (1). The Cronbach's alpha for the scale was 0.860.
4.2.3 Content knowledge
The following are examples of the items to measure the CK (Schmidt et al., 2009; Chai et al., 2011): “I have enough knowledge about my teaching subject.” “I know the basic principles and concepts of my teaching subject.” “I know the history and development of important principles in my teaching subject.” Respondents were given a five-point scale from “strongly agree” (5) to “strongly disagree” (1). The Cronbach's alpha for the current study was 0.857.
4.2.4 Technological pedagogical knowledge
The following items measure the TPK (Schmidt et al., 2009; Schmid et al., 2020): “I can choose technologies that improve the teaching approaches for a lesson.” ‘I can choose technologies that enhance students” learning in the lesson.” “I can adapt the use of the technologies I am learning about to different teaching activities.” “I am thinking critically about how to use technology in my classroom.” Responses were given along a five-point scale ranging from “strongly agree” (5) to “strongly disagree” (1). Cronbach's alpha for the scale was 0.846.
4.2.5 Technological content knowledge
The following are examples of the items measuring the TCK (Schmid et al., 2020). “I know how technological advances in my field have changed my subject.” “I know which new technologies are currently being developed in the field of my subject.” “I know how to use technologies to participate in scientific discourse in my field.” The five-point scale for this set of indicators ranges from “strongly agree” (5) to “strongly disagree” (1). Cronbach's alpha for the scale was 0.806.
4.2.6 Pedagogical content knowledge
PCK was measured by the following sample items (Schmidt et al., 2009; Chai et al., 2011; Schmid et al., 2020). “I know how to choose effective teaching approaches to guide student thinking and learning in my teaching subject.” “I know how to develop exercises with which students can consolidate their knowledge of my teaching subject.” “I know how to evaluate student performance in my teaching subject.” The respondents were given a five-point scale ranging from “strongly agree” (5) to “strongly disagree” (1). Cronbach's alpha for the scale was 0.885.
4.2.7 Technological pedagogical content knowledge
The following are sample items to measure the TPCK (Schmidt et al., 2009; Schmid et al., 2020). “I can use strategies that combine content, technologies, and teaching approaches I have learned about in my class.” “I can teach lessons that appropriately combine my teaching subject, technologies, and teaching approaches.” The responses are given on a five-point scale from “strongly agree” (5) to “strongly disagree” (1). Cronbach's alpha for the scale was 0.901.
4.2.8 School climate
Sample items to measure the SC (Papanastasiou and Angeli, 2008; Raygan and Moradkhani, 2022) are: “There are other teachers in my school who integrate computers in the teaching and learning process.” “I often exchange ideas about technology integration with other teachers,” “Teachers at my school understand the value of computers as a part of the teaching and learning process,” and “In school meetings, we often discuss the importance of integrating computers into the school curriculum.” The items are measured along a five-point scale from “strongly agree” (5) to “strongly disagree” (1). Cronbach's alpha for the scale was 0.886.
4.2.9 Cyber wellness
CW indicators are adapted from previously tested work (Chai et al., 2012). Some items are “I model and teach IT legal and ethical use.” “I can teach students to use the Internet safely.” “I implement classroom procedures to help students resolve legal and ethical IT issues.” “I can teach students about cyber wellness issues.” The items were answered using a five-point scale from “strongly agree” (5) to “strongly disagree” (1). Cronbach's alpha for the scale was 0.826.
4.2.10 Digital nativity
The following are examples of the items to measure the DN (Teo, 2013; Kabakci Yurdakul, 2018). “I use computers for many things in my daily life.” “I can easily browse on the internet and perform another activity comfortably.” “I contact my friends through the computer every day.” The items are measured along a five-point Likert scale ranging from “strongly agree” (5) to “strongly disagree” (1). Cronbach's alpha for the scale was 0.851.
4.3 Data analysis
The data analysis in this study is done in two phases: (i) the confirmatory factor analysis (CFA) to evaluate the construct convergent and discriminant validity, and (ii) the structural equation modeling (SEM) to test the hypothesized path model. Hurley et al. (1997) proposed that scales with sufficient theoretical and empirical evidence could be used directly in CFA without first performing exploratory factor analysis (EFA). Six items were removed during the CFA to improve the measurement model's fit. Generally, the remaining items range from 3 to 8, indicating a good specification of the final measurement model. In the SEM analysis, the researchers re-computed Cronbach's alpha to establish the reliability of the remaining indicators (Hair, 2009). The internal reliability of each factor was good, as all had Cronbach alpha indices of at least 0.70 (Table 4).
The factor and path analysis of the final measurement model were performed with AMOS software (Version 26) with maximum likelihood as the discrepancy estimation. Maximum likelihood estimation was used to achieve the best model fit. According to Hox and Bechger (1998), a reasonable sample size for reaching maximum likelihood estimation in SEM is around 200, which was met in this study. The model validation was evaluated using regression weights (standardized) or factor loadings, average variance extracted (AVE), and composite reliability (CR) (Fornell and Larcker, 1981). The model fit indices were established using the Chi-square test, comparative fit index (CFI), Tucker-Lewis index (TLI), the standardized root mean square residual (SRMR), and the root-mean-square error of approximation (RMSEA) (Hair, 2009). However, since the Chi-square test will naturally become significant on large sample sizes, the researchers alternatively use the minimum discrepancy index of the Chi-square (CMIN/df) (Wheaton et al., 1977).
5 Results
The results are presented in sequence: (1) Preliminary analysis establishes bivariate relationships and identifies potential multicollinearity among the model's constructs. (2) The measurement model is established through confirmatory factor analysis, followed by (3) testing the hypothesized path model.
5.1 Preliminary analysis
The descriptive measures (i.e., mean, standard deviation) and correlations of the latent constructs were analyzed to confirm the normality of the distributions and to detect multicollinearity among factors (see Table 3). Zero-order correlations between TPACK variables indicate that the highest correlation exists between TPACK and PCK (r = 0.762, p < 0.01), and the lowest correlation exists between DN and PCK (r = 0.351, p < 0.01). The results showed no existing multicollinearity among the constructs, as all correlation indices of the study variables are <0.90 (Lischetzke, 2014). All correlation coefficients are significant at the alpha level of 0.01 (**).
5.2 Confirmatory factor analysis
A total of 311 responses were included in the confirmatory factor analysis (CFA). Using covariance-based SEM (CB-SEM) methodology, we use the following cutoff scores to come up with a good-fitting model: (i) RMSEA must be ≤ 0.070, (ii) SRMR must be ≤ 0.080, (iii) TLI must be ≥0.900, and (iv) CFI must be ≥0.900 (Hu and Bentler, 1999). The main objective of co-variating the factors is to eliminate any effects among sub-groups of respondents and achieve unbiased results (Hair, 2009). The factor loading must be ≥0.5 to improve the goodness of fit indices (Hair et al., 2014; Awang, 2015). Therefore, items in a construct with factor loading <0.5 were deleted one at a time (starting with the lowest value) until parsimonious unidimensionality was achieved. Based on the threshold values, the items TK1, SC1, DN4, DN5, DN6, and DN7 were removed to improve the model fit measures.
Table 4 presents the factor loadings of each indicator, the constructs' composite reliability (CR), average variance extracted (AVE), and Cronbach's alpha of the final model. All indicators have acceptable factor loadings ranging from 0.507 to 0.878 (Fornell and Larcker, 1981). Composite reliability measures the internal consistency of the constructs (Kline, 2016). All constructs presented high interconnectedness with a 0.70 threshold (Hair, 2009). Regarding the reliability data of the model, Cronbach's alpha reliability coefficient ranged from 0.716 to 0.901. The measurement model indicates an excellent fit with computed values SRMR (0.0608), TLI (0.910), CFI (0.919), and RMSEA (0.049).
5.3 Testing the relationships among latent variables
Table 5 revealed that the factors DN and PCK have moderate and significant correlation (r = 0.337, p < 0.01). A strong and significant correlation was observed between PCK and TPACK (r = 0.762, p < 0.01). However, among the construct of TPACK, the correlation between TK and TPACK (r = 0.438, p < 0.01) is moderate since it is below 0.60 (Hair, 2009), whereas the other six constructs were large. Concerning the TPACK constructs, CW has a moderate and significant correlation with the range of 0.367 to 0.506. SC has a moderate and significant correlation with the range of 0.355 to 0.465. DN has a moderate and significant correlation ranging from 0.337 to 0.441.
5.4 Structural model
To validate the hypothesis in Figure 2, the results of the path analysis of the structural model are shown in Table 6. The model of this study yielded the indices of a satisfactory model fit (χ2/df = 1.726, TLI = 0.922, and CFI = 0.929), and the RMSEA = 0.067 indicates an acceptable fit (Steiger, 2007).
As shown in Table 6, 10 paths are significant at p < 0.001, two paths are significant at p < 0.01, four paths are significant at p < 0.05, and two paths are not significant. The result revealed that SC is positively significant to TK (β = 0.175; p < 0.05) and CK (β = 0.168; p < 0.01). However, the relationship between SC and PK was found to be not significant. DN was found to be positively significant to the three core bases of knowledge (PK, CK, and TK). Specifically, DN has a significant positive influence on TK (β = 0.432; p < 0.001), PK (β = 0.508; p < 0.001), and CK (β = 0.359; p < 0.001). In addition, there is a positive and significant influence between CW to PK (β = 0.219; p < 0.01) and CK (β = 0.124; p < 0.05). However, there is no significant relationship between CW to TK.
Moreover, the interconnections between core knowledge bases (PK, CK, and TK) significantly and positively influenced the second-level bases of knowledge (TPK, TCK, and PCK). Thus, H4, H5, and H6 are supported. In detail, TK is reported to have significant effects on TPK (β = 0.123; p < 0.05) and TCK (β = 0.376; p < 0.001). In addition, PK significantly predicts TPK (β = 0.385; p < 0.001) and PCK (β = 0.442; p < 0.001). The last core base, CK, is informed to be significant in determining TCK (β = 0.621; p < 0.001) and PCK (β = 0.387; p < 0.001). There is a strong link between PK and PCK for these three core bases of knowledge. These results showed that the fundamental components of CK, PK, and TK could serve as good connections toward TPK and TCK. These findings were consistent with the study of Habibi et al. (2020).
In affecting TPACK, the second-level bases of knowledge (TPK, TCK, and PCK) are considered significant. Thus, the three hypotheses (H8, H9, and H10) are statistically confirmed. TPK is positive and significantly influences TPACK (β = 0.441; p < 0.001), which is found to have the strongest correlation of all proposed hypotheses in this study. TCK is the weakest predictor influencing TPACK (β = 0.128; p < 0.05). In addition, TPACK is most strongly predicted by PCK (β = 0.604; p < 0.001). The final model with coefficients and significance is presented in Figure 3.
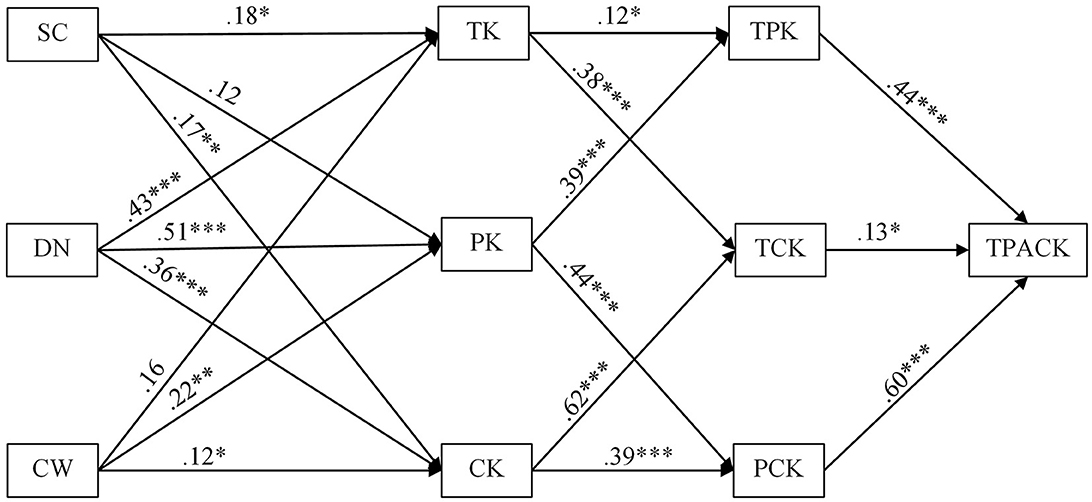
Figure 3. The final path model with coefficients and significant. ***p < 0.001, **p < 0.01, *p < 0.05.
6 Discussions
This study investigated the statistically significant paths to teachers' TPACK with the antecedent variables of cyber wellness, social climate, and digital nativity. There are three prominent findings in this study: (1) the CK is a strong predictor of TCK (β = 0.621, p < 0.001), (2) the PCK is a strong predictor of TPACK (β = 0.604, p < 0.001), and (3) DN is a strong predictor of PK (β = 0.508, p < 0.001). The first two prominent findings confirm several works in the literature. For instance, the alignment of CK in enhancing PK through technology, such as the use of interactive whiteboards (IWB), underscores the importance of ensuring that the content aligns with the digital materials educators intend to utilize (Gonzales and Gonzales, 2021). This ensures that technology integration enhances rather than detracts from the educational objectives. Conversely, Valle (2024) presented that teachers who face challenges in enhancing their mathematical expertise will likely encounter obstacles in utilizing and merging technology tools into the teaching-learning process. These findings affirm the study of Dong et al. (2015) and Mailizar et al. (2021) that teachers equipped with a deep understanding of their subject matter are better positioned to convey complex concepts effectively. This foundational expertise not only aids in crafting comprehensive lesson plans but also fosters insightful discussions. Teachers' delivery of the subject matter is enhanced with the use of technological tools (Pamuk et al., 2015; Wang, 2019; Nikolopoulou et al., 2021). In a sense, a mixture of CK and PK could be attributable to the effects of TCK and TPACK. However, it should be noted that there is a need to carefully monitor the pedagogical skills (i.e., formulation of lesson objectives) so that this aspect will not be left due to the excitement of implementing the technology during classes (Gonzales and Gonzales, 2021). Moreover, this implies that efficient use of technology that can enhance the efficiency of the teaching-learning process requires teachers' knowledge of content and pedagogy. Notably, teachers with a profound knowledge of their subject matter are likelier to incorporate technology into their teaching. Similarly, teachers with a nuanced understanding of how to teach concepts are better equipped to leverage technology to support their instructional objectives.
To explain the association of DN to PK, the paper identified the teacher respondents as digital natives or not. Based on Prensky's (2001) classification, 255 or 72.98% are digital natives, while only 95 or 27.14% are non-digital natives. The strong association could be attributed to the fact that digital natives have traits that are helpful in the new pedagogical skills intended for 21st-century learners. These traits include but are not limited to, quick access to online resources (Thompson, 2013), multitasking skills, and the ability to teach effectively when online. Although the definition of DN does not directly refer to these traits, these associations, including DN to TK (β = 0.432) and DN to CK (β = 0.359), are evidence that part and overall variations of TPACK are attributed to the majority of the digital native teacher respondents. This means that the model was able to explain the amount of variance along the paths of DN to related constructs of technology skills and pedagogical skills of the respondents. These characteristics significantly affect their knowledge of technology, pedagogy, and content necessary to transition from traditional to online classes. For this reason, non-digital native teachers must up-skill and embrace digital technologies, or they won't be able to guide their students in creating new knowledge. Teachers must be equipped with the necessary technological and pedagogical skills to contribute effectively and productively to the information-based, knowledge-driven, and digital society.
The implications of this study are valuable for educational managers, especially in the post-pandemic new normal. Key areas for strategic focus include enhancing teachers' wellness using digital materials and developing policies to improve school technological support. Professional development programs should emphasize integrating pedagogy with technology to improve instructional delivery. Supporting innovative teaching practices with strong content and pedagogical knowledge can enhance educational performance, especially in developing economies like the Philippines. Educational leaders and policymakers may prioritize initiatives that develop content and pedagogical expertise and digital fluency to optimize technology's benefits in education. Acknowledging the traits of digital natives, such as quick access to online resources and multitasking abilities, can inform targeted interventions to support the modern-day intended learning outcomes. The paper also capitalizes on the importance of balancing technological enthusiasm with pedagogical rigor to ensure technology integration does not overshadow essential teaching skills.
7 Conclusion
Recent studies have investigated TPACK, intending to explain variations with different behavioral constructs in the context of teaching. However, none explicitly explored these variables, along with the interconnectedness of the TPACK pathways to relevant latent constructs affecting variations of teachers' perceptions. This paper investigates the antecedent factors of teachers' TPACK with cyber-wellness, school climate, and digital nativity. The model was tested using covariance-based structural equation modeling (CB-SEM). The survey data is validated in a cross-sectional study of 311 basic education teacher participants, confirming 16 significant paths on the proposed model, three of which have higher explanatory powers.
Based on the significance of the paths from antecedent variables to the TPACK framework, we explicitly put forward three important contributions based on the salient findings of this paper. First, the high explanatory power of DN to PK indicates an advantage for a digital native in translating digital materials to pedagogical competencies in delivering the lessons. Thus, it is imperative that the crafting of policy guidelines for the digitalization of materials must focus on intervening factors such as digital nativity and cyber-wellness. For example, educational leaders may invest more in capacity building for basic education teachers to enhance their confidence in creating digital materials, especially among non-digital natives. Secondly, the high explanatory power of PCK to TPACK affirms the results of emerging literature contextualized in this paper from a developing economy amidst the intricacies of the post-pandemic new normal. This finding is a critical contribution, especially since the basic education of the developing economies was the most affected by the disruptions brought about by the pandemic. Thus, there is a statistical reason to believe that the PCK of teachers was translated to cater to the technological integration during the abrupt transition. Lastly, the high explanatory power of CK to TCK is also a point of discussion because even in economically challenged countries like the Philippines, the teachers are resilient enough to convert their expertise to a more technology-enhanced recital of classes.
8 Limitations of the study
Like any survey research, this paper has limitations in interpreting the findings. Firstly, voluntary participation may introduce biases because the sample consists of individuals who chose to participate, potentially limiting the findings to a more specific group. Secondly, self-reported responses may be biased. Lastly, the gender imbalance, with females comprising 79.74% of participants, may limit the diversity of perspectives, especially those of male participants. However, these limitations do not affect the overall generalizability of the results, and the findings still offer valuable insights into the relationships between the hypothesized antecedent factors and the TPACK framework.
Data availability statement
The raw data supporting the conclusions of this article will be made available by the authors, without undue reservation.
Ethics statement
The studies involving humans were approved by Dr. Christeodoflor Ramos, Chairman, Local Ethics Review Committee Cebu Technological University. The studies were conducted in accordance with the local legislation and institutional requirements. The participants provided their written informed consent to participate in this study.
Author contributions
LV: Conceptualization, Data curation, Formal analysis, Funding acquisition, Investigation, Methodology, Project administration, Supervision, Validation, Visualization, Writing – original draft, Writing – review & editing. RG: Writing – original draft, Writing – review & editing. RA: Writing – original draft, Writing – review & editing. GB: Conceptualization, Data curation, Formal analysis, Investigation, Methodology, Validation, Visualization, Writing – original draft, Writing – review & editing. GG: Conceptualization, Data curation, Formal analysis, Funding acquisition, Investigation, Methodology, Project administration, Supervision, Validation, Visualization, Writing – original draft, Writing – review & editing.
Funding
The author(s) declare that financial support was received for the research, authorship, and/or publication of this article. This paper was funded under the General Appropriations Act (GAA) of the Cebu Technological University, Cebu City, Philippines.
Conflict of interest
The authors declare that the research was conducted in the absence of any commercial or financial relationships that could be construed as a potential conflict of interest.
Publisher's note
All claims expressed in this article are solely those of the authors and do not necessarily represent those of their affiliated organizations, or those of the publisher, the editors and the reviewers. Any product that may be evaluated in this article, or claim that may be made by its manufacturer, is not guaranteed or endorsed by the publisher.
Supplementary material
The Supplementary Material for this article can be found online at: https://www.frontiersin.org/articles/10.3389/feduc.2024.1397888/full#supplementary-material
References
Alghasab, M. B., Alfadley, A., and Aladwani, A. M. (2020). Factors affecting technology integration in EFL classrooms: the case of Kuwaiti Government Primary Schools. J. Educ. Learn. 9, 10–27. doi: 10.5539/jel.v9n4p10
Awang Z. (2015). “Validating the measurement model: CFA,” in A Handbook on SEM. 2nd Edn. (Kuala Lumpur: Universiti Sultan Zainal Abidin), 54–73.
Bender, T. (2023). Discussion-based Online Teaching to Enhance Student Learning: Theory, Practice and Assessment. Boca Raton, FL: Taylor and Francis.
Çebi, A., Özdemir, T. B., Reisoglu, I., and Çolak, C. (2022). From digital competences to technology integration: re-formation of preservice teachers' knowledge and understanding. Int. J. Educ. Res. 113:101965. doi: 10.1016/j.ijer.2022.101965
Celik, I. (2023). Towards Intelligent-TPACK: An empirical study on teachers' professional knowledge to ethically integrate artificial intelligence (AI)-based tools into education. Comput. Hum. Behav. 138:107468. doi: 10.1016/j.chb.2022.107468
Chai, C. S., Koh, J. H. L., Ho, H. N. J., and Tsai, C. C. (2012). Examining preservice teachers' perceived knowledge of TPACK and cyberwellness through structural equation modeling. Australas. J. Educ. Technol. 28, 1000–1019. doi: 10.14742/ajet.807
Chai, C. S., Koh, J. H. L., and Tsai, C. C. (2010). Facilitating preservice teachers' development of technological, pedagogical, and content knowledge (TPACK). J. Educ. Technol. Soc. 13, 63–73. Available online at: http://www.jstor.org/stable/jeductechsoci.13.4.63
Chai, C. S., Koh, J. H. L., Tsai, C. C., and Tan, L. L. W. (2011). Modeling primary school preservice teachers' Technological Pedagogical Content Knowledge (TPACK) for meaningful learning with information and communication technology (ICT). Comput. Educ. 57, 1184–1193. doi: 10.1016/j.compedu.2011.01.007
Chen, Y. L. (2008). A mixed-method study of EFL teachers' Internet use in language instruction. Teach. Teach. Educ. 24, 1015–1028. doi: 10.1016/j.tate.2007.07.002
Dong, Y., Chai, C. S., Sang, G. Y., Koh, J. H. L., and Tsai, C. C. (2015). Exploring the profiles and interplays of preservice and in-service teachers' technological pedagogical content knowledge (TPACK) in China. J. Educ. Technol. Soc. 18, 158–169. Available online at: http://www.jstor.org/stable/jeductechsoci.18.1.158
Dong, Y., Xu, C., Chai, C. S., and Zhai, X. (2020). Exploring the structural relationship among teachers' technostress, technological pedagogical content knowledge (TPACK), computer self-efficacy and school support. Asia-Pac. Educ. Res. 29, 147–157. doi: 10.1007/s40299-019-00461-5
Fornell, C., and Larcker, D. F. (1981). Evaluating structural equation models with unobservable variables and measurement error. J. Market. Res. 18:39. doi: 10.1177/002224378101800313
Gonzales, G. (2022). Mapping pupil's learning progression using hand manipulatives and touch screen applications: implications to post-COVID-19 new normal. Educ. Res. Int. 2022, 1–10. doi: 10.1155/2022/9976083
Gonzales, G., Gonzales, R., Costan, F., and Himang, C. (2020). Dimensions of motivation in teaching: relations with social support climate, teacher efficacy, emotional exhaustion, and job satisfaction. Educ. Res. Int. 2020, 1–10. doi: 10.1155/2020/8820259
Gonzales, G. G., and Gonzales, R. R. (2021). Introducing IWB to preservice mathematics teachers: an evaluation using the TPACK framework. Cypriot J. Educ. Sci. 16, 436–450. doi: 10.18844/cjes.v16i2.5619
Habibi, A., Yusop, F. D., and Razak, R. A. (2020). The role of TPACK in affecting preservice language teachers' ICT integration during teaching practices: Indonesian context. Educ. Inform. Technol. 25, 1929–1949. doi: 10.1007/s10639-019-10040-2
Hair, J. F., Black, W. C., Babin, B. J., Anderson, R. E., and Tatham, R. L. (2014). Pearson New International Edition. Multivariate Data Analysis. London: Pearson.
Harris, J., Mishra, P., and Koehler, M. (2009). Teachers' technological pedagogical content knowledge and learning activity types: curriculum-based technology integration reframed. J. Res. Technol. Educ. 41, 393–416. doi: 10.1080/15391523.2009.10782536
Helsper, E. J., and Eynon, R. (2010). Digital natives: where is the evidence? Br. Educ. Res. J. 36, 503–520. doi: 10.1080/01411920902989227
Hox, J. J., and Bechger, T. M. (1998). An introduction to structural equation modeling. Fam. Sci. Rev. 11, 354–373.
Hoy, W. K. (1990). Organizational climate and culture: a conceptual analysis of the school workplace. J. Educ. Psychol. Consult. 1, 149–168. doi: 10.1207/s1532768xjepc0102_4
Hu, L. T., and Bentler, P. M. (1999). Cutoff criteria for fit indexes in covariance structure analysis: conventional criteria versus new alternatives. Struct. Equ. Model. 6, 1–55. doi: 10.1080/10705519909540118
Hurley, A. E., Scandura, T. A., Schriesheim, C. A., Brannick, M. T., Seers, A., Vandenberg, R. J., et al. (1997). Exploratory and confirmatory factor analysis: guidelines, issues, and alternatives. J. Organ. Behav. 18, 667–683. doi: 10.1002/(SICI)1099-1379(199711)18:6<667::AID-JOB874>3.0.CO;2-T
Kabakci Yurdakul, I. (2018). Modeling the relationship between preservice teachers' TPACK and digital nativity. Educ. Technol. Res. Dev. 66, 267–281. doi: 10.1007/s11423-017-9546-x
Kline, R. B. (2016). Principles and Practice of Structural Equation Modeling (Fourth; TD Little, Ed.). New York, NY: The Guilford Press.
Koehler, M. J., Mishra, P., and Yahya, K. (2007). Tracing the development of teacher knowledge in a design seminar: integrating content, pedagogy and technology. Comput. Educ. 49, 740–762. doi: 10.1016/j.compedu.2005.11.012
Lawrence, J. E., and Tar, U. A. (2018). Factors that influence teachers' adoption and integration of ICT in teaching/learning process. Educ. Media Int. 55, 79–105. doi: 10.1080/09523987.2018.1439712
Lewin, C., Niederhauser, D., Johnson, Q., Saito, T., Sakamoto, A., Sherman, R., et al. (2021). Safe and responsible internet use in a connected world: promoting cyber-wellness. Can. J. Learn. Technol. 47:n4. doi: 10.21432/cjlt28069
Lischetzke, T. (2014). “Daily diary methodology,” in Encyclopedia of Quality of Life and Well-Being Research, ed. A. C. Michalos (Dordrecht: Springer), 1413–1419. doi: 10.1007/978-94-007-0753-5_657
Mailizar, M., Hidayat, M., and Al-Manthari, A. (2021). Examining the impact of mathematics teachers' TPACK on their acceptance of online professional development. J. Digit. Learn. Teach. Educ. 37, 196–212. doi: 10.1080/21532974.2021.1934613
Meena, Y., Sankhla, M. S., Sonone, S. S., Parashar, A., Parihar, K., Saini, K., et al. (2021). “Cyber exploitation through cybercrimes and challenges, in 2021 3rd International Conference on Advances in Computing, Communication Control and Networking (ICAC3N) (Greater Noida: IEEE), 1467–1472. doi: 10.1109/ICAC3N53548.2021.9725493
Mihci Türker, P., and Kiliç Çakmak, E. (2019). An investigation of cyber wellness awareness: Turkey secondary school students, teachers, and parents. Comput. Sch. 36, 293–318. doi: 10.1080/07380569.2019.1677433
Mishra, P., and Koehler, M. J. (2006). Technological pedagogical content knowledge: a framework for teacher knowledge. Teach. Coll. Rec. 108, 1017–1054. doi: 10.1111/j.1467-9620.2006.00684.x
Naima, L. (2017). Exploring the status and teachers' perceptions of technology integration in EFL classrooms at Chadli Bendjedid University, Algeria. Arab World Engl. J. 8, 160–170. doi: 10.24093/awej/vol8no2.11
Nelson, M. J., and Voithofer, R. (2022). Coursework, field experiences, and the technology beliefs and practices of preservice teachers. Comput. Educ. 186:104547. doi: 10.1016/j.compedu.2022.104547
Nikolopoulou, K., Gialamas, V., and Lavidas, K. (2021). Habit, hedonic motivation, performance expectancy and technological pedagogical knowledge affect teachers' intention to use mobile Internet. Comput. Educ. Open 2:100041. doi: 10.1016/j.caeo.2021.100041
Núñez-Canal, M., de Obesso, M. D. L. M., and Pérez-Rivero, C. A. (2022). New challenges in higher education: a study of the digital competence of educators in COVID times. Technol. Forecast. Soc. Change 174:121270. doi: 10.1016/j.techfore.2021.121270
Orlando, J., and Attard, C. (2016). Digital natives come of age: the reality of today's early career teachers using mobile devices to teach mathematics. Math. Educ. Res. J. 28, 107–121. doi: 10.1007/s13394-015-0159-6
Özgür, H. (2020). Relationships between teachers' technostress, technological pedagogical content knowledge (TPACK), school support and demographic variables: a structural equation modeling. Comput. Hum. Behav. 112:106468. doi: 10.1016/j.chb.2020.106468
Pamuk, S., Ergun, M., Cakir, R., Yilmaz, H. B., and Ayas, C. (2015). Exploring relationships among TPACK components and development of the TPACK instrument. Edu. Inform. Technol. 20, 241–263. doi: 10.1007/s10639-013-9278-4
Papanastasiou, E. C., and Angeli, C. (2008). Evaluating the use of ICT in education: psychometric properties of the survey of factors affecting teachers teaching with technology (SFA-T3). J. Educ. Technol. Soc. 11, 69–86. Available online at: http://www.jstor.org/stable/jeductechsoci.11.1.69
Polly, D., Mims, C., Shepherd, C. E., and Inan, F. (2010). Evidence of impact: transforming teacher education with preparing tomorrow's teachers to teach with technology (PT3) grants. Teach. Teach. Educ. 26, 863–870. doi: 10.1016/j.tate.2009.10.024
Prensky, M. (2001). Digital natives, digital immigrants part 2: Do they really think differently? On the Horizon 9, 1–6. doi: 10.1108/10748120110424843
Rapanta, C., Botturi, L., Goodyear, P., Guàrdia, L., and Koole, M. (2021). Balancing technology, pedagogy and the new normal: post-pandemic challenges for higher education. Postdigital Sci. Educ. 3, 715–742. doi: 10.1007/s42438-021-00249-1
Raygan, A., and Moradkhani, S. (2022). Factors influencing technology integration in an EFL context: investigating EFL teachers' attitudes, TPACK level, and educational climate. Comput. Assist. Lang. Learn. 35, 1789–1810. doi: 10.1080/09588221.2020.1839106
Santos, J. M., and Castro, R. D. (2021). Technological Pedagogical content knowledge (TPACK) in action: application of learning in the classroom by preservice teachers (PST). Soc. Sci. Humanit. Open 3:100110. doi: 10.1016/j.ssaho.2021.100110
Schmid, M., Brianza, E., and Petko, D. (2020). Developing a short assessment instrument for Technological Pedagogical Content Knowledge (TPACK. xs) and comparing the factor structure of an integrative and a transformative model. Comput. Educ. 157:103967. doi: 10.1016/j.compedu.2020.103967
Schmid, M., Brianza, E., and Petko, D. (2021). Self-reported technological pedagogical content knowledge (TPACK) of preservice teachers in relation to digital technology use in lesson plans. Comput. Hum. Behav. 115:106586. doi: 10.1016/j.chb.2020.106586
Schmidt, D. A., Baran, E., Thompson, A. D., Mishra, P., Koehler, M. J., Shin, T. S., et al. (2009). Technological pedagogical content knowledge (TPACK) the development and validation of an assessment instrument for preservice teachers. J. Res. Technol. Educ. 42, 123–149. doi: 10.1080/15391523.2009.10782544
Searson, M., Hancock, M., Soheil, N., and Shepherd, G. (2015). Digital citizenship within global contexts. Educ. Inform. Technol. 20, 729–741. doi: 10.1007/s10639-015-9426-0
Shaikh, F. B., Rehman, M., Amin, A., and Hashmani, M. A. (2021). “Cyber Wellness and youth: a systematic literature review,” in 2021 IEEE International Conference on Computing (ICOCO) (Kuala Lumpur: IEEE), 7–12. doi: 10.1109/ICOCO53166.2021.9673586
Shulman, L. S. (1986). Those who understand: knowledge growth in teaching. Educ. Res. 15, 4–14. doi: 10.2307/1175860
Steiger, J. H. (2007). Understanding the limitations of global fit assessment in structural equation modeling. Pers. Individ. Diff. 42, 893–898. doi: 10.1016/j.paid.2006.09.017
Teo, T. (2013). An initial development and validation of a Digital Natives Assessment Scale (DNAS). Comput. Educ. 67, 51–57. doi: 10.1016/j.compedu.2013.02.012
Thompson, A. D., and Mishra, P. (2007). Editors' remarks: Breaking news: TPCK becomes TPACK! J. Comput. Teach. Educ. 24, 38–64. doi: 10.1080/10402454.2007.10784583
Thompson, P. (2013). The digital natives as learners: technology use patterns and approaches to learning. Comput. Educ. 65, 12–33. doi: 10.1016/j.compedu.2012.12.022
Valle, L. (2024). Reimagining mathematics education: Identifying training needs and challenges among public elementary school teacher's post-pandemic. Int. J. Educ. Pract. 12, 527–539. doi: 10.18488/61.v12i3.3723
Wang, C. J. (2019). Facilitating the emotional intelligence development of students: use of technological pedagogical content knowledge (TPACK). J. Hosp. Leis. Sport Tour. Educ. 25:100198. doi: 10.1016/j.jhlste.2019.100198
Wheaton, B., Muthen, B., Alwin, D. F., and Summers, G. F. (1977). Assessing reliability and stability in panel models. Sociol. Methodol. 8, 84–136. doi: 10.2307/270754
Yildiz, M. A. (2017). Emotion regulation strategies as predictors of internet addiction and smartphone addiction in adolescents. J. Educ. Sci. Psychol. 7, 66–78.
Zakariya, Y. F. (2020). Effects of school climate and teacher self-efficacy on job satisfaction of mostly STEM teachers: a structural multigroup invariance approach. Int. J. STEM Educ. 7, 1–12. doi: 10.1186/s40594-020-00209-4
Keywords: TPACK, digital nativity, school climate, post-pandemic new normal, cyber-wellness, SEM
Citation: Valle LC, Gonzales RR, Almacen RML, Batucan G and Gonzales GG (2024) Modeling the relationship of the TPACK framework with cyber wellness, school climate, and digital nativity of basic education teachers. Front. Educ. 9:1397888. doi: 10.3389/feduc.2024.1397888
Received: 08 March 2024; Accepted: 24 June 2024;
Published: 12 July 2024.
Edited by:
Yi-Fen Yeh, National Taiwan Normal University, TaiwanReviewed by:
Ella Fitriani, Jakarta State University, IndonesiaTzu-Chiang Lin, National Kaohsiung University of Science and Technology, Taiwan
Aodah Diamah, Jakarta State University, Indonesia
Wanda Nugroho Yanuarto, Muhammadiyah University of Purwokerto, Indonesia
Copyright © 2024 Valle, Gonzales, Almacen, Batucan and Gonzales. This is an open-access article distributed under the terms of the Creative Commons Attribution License (CC BY). The use, distribution or reproduction in other forums is permitted, provided the original author(s) and the copyright owner(s) are credited and that the original publication in this journal is cited, in accordance with accepted academic practice. No use, distribution or reproduction is permitted which does not comply with these terms.
*Correspondence: Lislee C. Valle, bGlzbGVlLnZhbGxlJiN4MDAwNDA7Y3R1LmVkdS5waA==