- Universidad Nacional del Altiplano, Puno, Peru
The research aimed to assess the urban-rural gap in mathematics achievement among secondary education students in Peru using the ECE 2018 evaluation and the Oaxaca-Blinder method. Employing a retrospective observational design, the study analyzed data from the ECE to understand this disparity. It revealed a significant gap favoring urban areas, even after adjusting for socioeconomic factors and school contributions, with a difference of 63.28 points. Decomposition analysis showed that 82.58% of this gap is explained by differences in observable attributes (endowments), primarily socioeconomic variables and school inputs. These findings underscore the importance of public policies targeting improvements in both school resources and the living standards of impoverished populations to address educational disparities effectively.
Introduction
Investigating the achievement gap in learning outcomes between urban and rural areas in Peru is crucial for understanding the multifaceted disparities and developing targeted interventions to address these challenges. The disparities in learning outcomes between urban and rural areas in Peru are influenced by a multitude of factors, including socioeconomic status, access to healthcare, educational infrastructure, and cultural differences. These factors contribute to the complex web of challenges that perpetuate the achievement gap. Investigating these disparities is essential for developing targeted interventions that address the root causes of the gap and promote equitable access to quality education and healthcare for all individuals, regardless of their geographical location.
Several studies provide valuable insights into the disparities between urban and rural areas, shedding light on the factors contributing to the achievement gap. For instance, highlight that the urban-rural differential is largest in Andean and central Latin America, including Peru, emphasizing the significance of understanding the specific challenges faced in these regions (Paciorek et al., 2013), further support this by demonstrating that rural areas generally have worse health status than urban areas, indicating a potential link between health and educational outcomes (Sun et al., 2011). Additionally, the role of income inequality between urban and rural households in explaining disparities in child malnutrition, suggesting a similar influence on educational outcomes (Sharaf and Rashad, 2016).
Moreover, the achievement gap requires capacity-building for principals and teachers working with rural pupils, highlighting the importance of educational infrastructure and support systems (Piyaman et al., 2017). Similarly, found that maternal education was a crucial predictor for narrowing urban-rural disparities in childhood stunting, emphasizing the role of education in addressing health and developmental disparities (Tadesse et al., 2023).
The hypothesis that the level of socioeconomic status in both the household and the surrounding environment contributes to the achievement gap between urban and rural students is supported by several studies. Reeves (2012) emphasizes the impact of family socioeconomic status on the rural math achievement gap in high school, indicating a correlation between socioeconomic status and academic achievement. Similarly, Ababneh and Kodippili (2020) found that students attending rural schools were not performing as well as students from urban schools, highlighting the association of variables with the mathematics achievement gap between rural and urban students.
Furthermore, Chatterjee and Burns (2021) discuss the inequity in attainment and achievement levels for students from different socioeconomic backgrounds, emphasizing the high dropout and academic failure rates for disadvantaged students. This suggests that socioeconomic factors play a significant role in academic achievement, particularly in rural areas. Additionally, Lee and Um (2021) suggest that more intensive socioeconomic and educational support is needed for rural areas, further supporting the hypothesis. Moreover, Sun and Du (2021) provide empirical evidence indicating that access to education is dependent on various factors, including socioeconomic endowment, which can contribute to the educational gap between urban and rural students. This aligns with the hypothesis that the level of socioeconomic status in the household and the surrounding environment influences the achievement gap between urban and rural students. In conclusion, the literature supports the hypothesis that a higher level of socioeconomic status in the household and the surrounding environment is associated with a wider achievement gap between urban and rural students. Factors such as family socioeconomic status, educational support, and access to resources play crucial roles in shaping the academic outcomes of students in different geographical settings.
To investigate the hypothesis that the quality of school inputs is correlated with the achievement gap between urban and rural students, it is essential to consider various factors that may contribute to this disparity. Several studies have explored the relationship between school inputs and student achievement, as well as the impact of urbanization, family involvement, teacher training, and educational resources on academic outcomes. Additionally, the influence of principal leadership and comprehensive school counseling programs on closing the achievement gap has been examined. Furthermore, the effects of environmental noise, distance from downtown, and creative thinking abilities on student achievement have also been studied. Rivkin et al. (2005) examined the relationship between teachers, schools, and academic achievement, emphasizing the importance of teacher quality and its impact on student achievemen.
Additionally, Ju et al. (2022) studied the nonlinear effect of urbanization on the gap between urban and rural education, indicating a strengthening of the narrowing effect with economic improvement. Furthermore, Sun and Du (2021) examined the role of teacher training in narrowing the educational gap between urban and rural students, providing empirical evidence to support their findings. In addition, Jacobs and Harvey (2010) explored the extent to which teacher attitudes and expectations predict academic achievement, emphasizing the influence of teacher perceptions on student outcomes.
The existing literature on the decomposition of educational achievement gaps at the regional level provides valuable insights into the factors contributing to these disparities. McEwan and Marshall (2004) on the indigenous-non-indigenous educational gap in Bolivia and Chile, finding that a significant portion of the educational gap could be attributed to differences in school attributes such as infrastructure and furniture. This research was extended to Guatemala, further emphasizing the role of school quality in explaining the achievement gap. Additionally, Ramos et al. (2016) analyzed the educational gap in Colombia and found that a substantial portion of the gap could be attributed to students’ family characteristics, particularly their socioeconomic status.
The socioeconomic conditions of households significantly impact the learning achievements of students in both urban and rural areas. Disparities in access to public services, income, and education levels are evident between rural and urban households, particularly those headed by females (Befort et al., 2012). These disparities extend to child nutrition, with rural–urban disparities influencing child nutrition in countries like Bangladesh and Nepal (Srinivasan et al., 2013). Furthermore, the significance of education in improving the socioeconomic conditions of individuals and communities has been highlighted (Monyai, 2018). This emphasizes the profound influence of socioeconomic conditions on educational outcomes, indicating the need for targeted interventions to address disparities and ensure equitable learning opportunities for all students.
The urban-rural achievement gap in Peru is influenced by various factors. Castro and Rolleston (2018) provide evidence of the significant role played by early childhood and schools in contributing to cognitive gaps. Additionally, Castro (2020) found that differences in school inputs play an important role and explain around 35% of the urban/rural gap in cognitive skill in Peru. These studies collectively underscore the multifaceted nature of the urban-rural achievement gap, influenced by socioeconomic factors, school conditions, and early childhood experiences.
To understand the influence of school inputs on the learning achievement gap between urban and rural environments, it is essential to consider various factors such as teacher-student ratio, premises condition, and student-computer ratio. Research has shown that observable school inputs occurring between ages 6 and 8 account for a significant share (around 35%) of the difference in cognitive skill (Castro, 2020). Additionally, the quality of teachers in urban schools compared to those in rural areas affects students’ academic attainment, leading to disparities in academic achievement (Owoeye and Yara, 2011). Moreover, the urban-rural difference in teacher effects contributes in large part to the observed urban-rural gap in student academic achievement (Zhang et al., 2018).
The article by Gimbert et al. (2007) examines how teacher preparation impacts student achievement in algebra in urban schools with staffing challenges. The study reveals that strong and specific preparation for teaching algebra can significantly enhance student academic performance in challenging contexts.
Furthermore, the condition of basic service provisions in peri-urban areas is unsatisfactory and far behind urban areas, indicating a disparity in infrastructure and resources (Mondal, 2021). Additionally, the study finds that the quality of rural and urban schooling differs significantly, with relatively higher quality schools found in suburban areas (Gadsden and Dixon-Román, 2016). This disparity in school quality can contribute to the learning achievement gap between urban and rural environments.
The hypotheses that guide the investigation are two: hypothesis 1: The learning achievement gap is due to observable characteristics at the student, home, and context levels. Hypothesis 2: The observable characteristics that have the greatest contributions to the mathematics learning achievement gap are related to socioeconomic characteristics and school inputs.
To understand the achievement gap in learning, it is essential to consider the influence of various observable factors at the student, household, and contextual levels. The socioeconomic status of students has been found to have a medium to strong relationship with academic achievement (Şirin, 2005). Additionally, context factors have been shown to impact student achievement, as evidenced by studies using data from the Trends in International Mathematics and Science Study (Caponera and Losito, 2016). Furthermore, the implementation of independent learning activities has been linked to improved learning achievement, indicating the potential impact of educational approaches on student outcomes (May, 2022).
Moreover, the influence of online learning on student characteristics has been explored, providing insights into the evolving nature of learning environments and their effects on students (Rudy et al., 2021). The analysis of the performance of students from different socioeconomic backgrounds on standardized assessments has revealed widening achievement gaps over time, underscoring the persistent challenges in addressing educational inequalities (Shala and Latifi, 2021). The impact of resource distribution and availability on pupil learning outcomes has been investigated, highlighting the importance of educational resources in shaping students’ learning experiences and achievements (Nyirenda, 2021). Moreover, the concept of the school as a learning organization has been reviewed, offering a broader perspective on the organizational factors influencing student learning and achievement (Stoll and Kools, 2017). Additionally, the most considered type of student characteristics by primary school teachers has been explored, emphasizing the role of educators in recognizing and addressing diverse student needs (Dwiwarna and Rahadian, 2018).
Various socio-economic factors and school inputs influence the urban-rural mathematics achievement gap. Here are the key findings from the relevant abstracts: socio-economic factors contributing to the urban-rural mathematics achievement gap: (i) research shows that mathematical proficiency gaps are related to students’ and schools’ indicators of poverty (González Canché, 2023), (ii) school socioeconomic status (SES) is strongly tied to participation and achievement in senior secondary school mathematics (Murphy, 2019), (iii) socioeconomic variation does not fully account for differences in achievement in rural locations, suggesting the role of rurality in mediating other effects on student achievement (Dean et al., 2023). Socio-economic status plays a significant role in educational development and achievement. Students from low socio-economic backgrounds often face challenges such as limited access to resources, inadequate healthcare, and unstable home environments, which can impact their academic performance. These disparities in economic well-being and family characteristics can impede educational development and readiness. The neighborhood in which a student resides can also influence their educational outcomes. Urban and rural areas often differ in terms of resources, opportunities, and community support. Urban areas may have more access to educational resources, extracurricular activities, and cultural institutions, which can positively impact student achievement. On the other hand, rural areas may face challenges such as limited access to quality schools, transportation barriers, and a lack of diverse learning opportunities.
School inputs affecting the urban-rural mathematics achievement gap: rural students take advanced math at a significantly lower rate than urban students, which contributes to the gap in educational attainment (Irvin et al., 2017). Nonmetropolitan schools are less likely to offer advanced mathematics subjects, and their students are less likely to choose those options (Murphy, 2019). Inadequate investment in rural education, such as funding and high-quality teaching resources, contributes to the gap (Xiang and Stillwell, 2023).
The neighborhood in which a student resides can also influence their educational outcomes. Urban and rural areas often differ in terms of resources, opportunities, and community support. Urban areas may have more access to educational resources, extracurricular activities, and cultural institutions, which can positively impact student achievement. On the other hand, rural areas may face challenges such as limited access to quality schools, transportation barriers, and a lack of diverse learning opportunities.
Transmission mechanisms through which socio-economic factors influence the urban-rural mathematics achievement gap: The presence of geography of mathematical (dis) advantage suggests that mathematical performance is spatially dependent across schools and neighborhoods (González Canché, 2023). Rurality mediates the effects of other factors on student achievement in a complex interplay of factors (Dean et al., 2023) Socio-economic conditions and the quantity of resources devoted to school systems trigger disparities in educational outcomes (Dean et al., 2023).
The urban-rural learning gap is mostly attributed to differences in school and family characteristics (Sumida and Kawata, 2021). This suggests that urban areas may have better school resources and more supportive family environments compared to rural areas. Inequality in access to resources: Another study in Peru found that inequalities in school environments, such as inadequate investment and lack of high-quality teaching resources, contribute to the cognitive gap between urban and rural children (Castro, 2020). This implies that urban areas may have better access to technological resources and educational facilities. Effects of government policies: The rural–urban education gap may also be influenced by government policies. One study suggests that inappropriate partitioning of the national territory and negative effects of school consolidation policies can hinder the educational achievements of rural areas (Xiang and Stillwell, 2023).
Various observable and non-observable factors influence the urban-rural gap in education performance. Observable factors include socioeconomic context, school resources, teaching quality, and infrastructure. Non-observable factors encompass discrimination, differences in opportunities, and school culture. Research has shown that urban schools generally have better access to quality education compared to rural schools (Nugba et al., 2022). Factors such as performance appraisal and teachers’ professional development have been found to influence value addition in secondary education (Odera et al., 2022). A proposed unified conceptual framework for quality education emphasizes the importance of collaboration within the education system for high-quality learning opportunities (Garira, 2020). Additionally, studies have reported that student performance is likely to improve in schools with higher shares of students from socioeconomically privileged families (Muñoz and Queupil, 2016). Academic supervision, school culture, and principal leadership have a positive influence on teacher performance (Wulandari et al., 2021). Settlement type has been linked to educational effectiveness, with rural schools exhibiting lower educational potential compared to urban schools (Murawska et al., 2019). The work environment and motivation significantly impact the performance of non-formal educators (Djibu and Duludu, 2020). Arts and cultural education play a crucial role in shaping pupils’ opinions and have a positive impact on education (Žnidaršič, 2020). Immigrant students’ educational success is influenced by factors such as attitudes towards achievement and strategic course selection (Marks, 2010). Character education based on local wisdom and meaningful encounters in schools contribute to educational success and social cohesion (Suastini and Sumada, 2022). Enhancing culture in education and community participation in Islamic elementary schools are also important for educational development (Backer et al., 2012; Sahlan, 2014; Budianur and Setiawan, 2021). Implementing professional development and creativity in artistic education are essential for improving educational outcomes (Steyn, 2005; Backer et al., 2012). Predictors of students’ academic success require a coherent analysis of educational policies and practices (Anghelache, 2017).
Main objective
The main objective of this research is to analyze the mathematics achievement gap between secondary school students in urban and rural areas in Peru during 2018, using the Oaxaca-Blinder decomposition to identify the factors contributing to this disparity.
Research hypotheses
Hypothesis 1: The mathematics achievement gap between urban and rural students is primarily due to observable characteristics at the student, household, and contextual levels.
Hypothesis 2: The observable characteristics that most contribute to the mathematics achievement gap between urban and rural students are related to socioeconomic factors and school inputs, such as household socioeconomic status and the quality of educational resources available.
Methods
The Oaxaca-Blinder decomposition
The Oaxaca-Blinder decomposition has been used to analyze urban-rural disparities in various contexts, such as healthcare utilization, income, and educational performance (Sakellariou, 2008; Fortin et al., 2011; Akita and Miyata, 2013; Vujicic and Nasseh, 2013; Jackson and VanderWeele, 2018; Luh and Wei, 2018; Berri et al., 2021; Fu et al., 2021; Nagi and Gummadi, 2022; Tadesse et al., 2023).
It has been instrumental in attributing a significant portion of the observed gaps to differences in characteristics and coefficients between urban and rural áreas (Sakellariou, 2008; Fortin et al., 2011; Akita and Miyata, 2013; Vujicic and Nasseh, 2013; Jackson and VanderWeele, 2018; Luh and Wei, 2018; Berri et al., 2021; Fu et al., 2021; Nagi and Gummadi, 2022; Tadesse et al., 2023). Additionally, the technique has been extended to nonlinear models, providing a comprehensive approach to decompose differences in outcomes between groups (Bauer and Sinning, 2008).
The Oaxaca-Blinder decomposition is a powerful tool for understanding and quantifying disparities between groups, providing valuable insights into the factors contributing to these differences. Its applications span across diverse fields, making it a versatile and widely used method for analyzing various forms of inequality and disparity.
The Oaxaca-Blinder decomposition method, aims to explain the difference in mean outcomes across two groups by dissecting the contributions of group differences in explanatory variables and differences in the magnitude of regression coefficients (Jann, 2008). This method has been widely applied in various fields, such as labor market analysis, financial literacy, and health disparities, to understand the factors contributing to differences in outcomes between different groups.
The application of the Oaxaca-Blinder decomposition has provided valuable insights into gender disparities in financial literacy, wage differentials, and health outcomes. For instance, in the analysis of gender gaps in financial literacy, the decomposition revealed that the majority of the gap was attributed to differences in coefficients rather than differences in the characteristics of men and women (Fonseca et al., 2012). Similarly, in the study of immigrant health disparities, the method allowed for the examination of disparities at various quantiles of the health distributions, providing a nuanced understanding of the determinants of health disparities among immigrants (Berloffa and Paolini, 2022).
Moreover, the Oaxaca-Blinder decomposition has been extended to non-linear models, enabling its application to a broader range of research questions and statistical models (Bauer and Sinning, 2008). This extension has facilitated the examination of wage discrimination, poverty differentials, and industry income gaps, providing a comprehensive understanding of the factors contributing to disparities across various domains (Agrawal, 2013; Chen et al., 2017; Matuszewska-Janica, 2018).
The Oaxaca-Blinder decomposition method is a widely used technique in economics and social sciences to analyze the difference in mean outcomes between two groups, denoted as Group A and Group B (Jann, 2008). This method allows for the decomposition of the mean outcome difference into several components, providing insights into the factors contributing to the observed differences (Firpo et al., 2009). The procedure is known in the literature as the Oaxaca-Blinder decomposition and has been applied to various fields such as labor market discrimination, educational attainment, income inequality, and disparities in informal care intensity (Powers et al., 2011; Arteaga and Glewwe, 2019; Zarei et al., 2021).
Threefold decomposition Oaxaca-Blinder for rural–urban gap in learning achievement
Denoting the two groups as urban (U) and rural (R), the difference in means can be explained simply as the difference in means of the results for the observations of group U and group R
Threefold decomposition
In the context of a linear regression, the mean outcome for Group G ∈ {U, R} can be expressed as , where contains the mean values of explanatory variables and are the estimated regression coefficients. Hence, can be rewritten as:
This expression can, in turn, be written as the sum of the following three terms:
Equation 4 of the Oaxaca-Blinder decomposition breaks down the mean outcome difference into three components: the endowments term, the coefficients term, and the interaction term. The endowments term accounts for differences in explanatory variables across groups, the coefficients term represents the part due to group differences in the coefficients, and the interaction term captures the simultaneous occurrence of cross-group differences in explanatory variables and coefficients (Fairlie, 2005; Bauer and Sinning, 2008; Jann, 2008).
Twofold decomposition
The Oaxaca-Blinder decomposition method is a valuable tool for understanding the factors contributing to differences in mean outcomes between groups. This method allows for the disentanglement of the portion of the difference in mean outcomes that can be explained by cross-group differences in the explanatory variables from the portion that remains unexplained (Bauer and Sinning, 2008). It has been widely used to identify and quantify the separate contributions of group differences in measurable characteristics to racial and gender gaps in outcomes (Fairlie, 2005). Furthermore, this method has been extended to nonlinear models, allowing for the decomposition of differences in a non-linear outcome variable between two groups into explained and unexplained parts (Bauer and Sinning, 2008). The Oaxaca-Blinder decomposition method is particularly useful in situations where there is a need to control for confounding variables to ensure the validity of the analysis (Attia et al., 2017). It allows for the prediction of the effect of changes in policy or other variables on quantiles, providing a comprehensive understanding of the impact of different explanatory variables on outcomes (Firpo et al., 2009) The unexplained portion of the mean outcome gap can be further decomposed into sub-components, measuring the part of the mean difference in outcomes originating from discrimination in favor of one group and the part that comes from discrimination against the other group (Powell et al., 2012).
Assuming that the group suffering discrimination is rural students, then the wage gap between urban and rural students is given by Equation 3, that is:
By adding and subtracting in the previous expression,
According to Equation 5, the wage difference between groups U and R equals the sum of two components. The first component is interpreted as the part explained by differences among observed variables, while the second component represents the unexplained part or that associated with unobserved variables.
Suppose it is the non-discriminatory condition or the reference coefficient, the general equation for the decomposition of will be:
The Oaxaca-Blinder model is used to decompose differences in educational achievement between groups, such as urban and rural, identifying the explained part (attributable to observable differences) and the unexplained part (associated with discrimination).
Data
The data correspond to the Student Census Evaluation (ECE) of second grade secondary school students, which is a standardized evaluation carried out by the Ministry of Education to know the learning achievements achieved by the country’s students. This evaluation is carried out in all public and private schools in the country that have more than five students in the grade to be evaluated. The Census evaluation contains data on learning achievements, the type of school management, the geographic area, and the socioeconomic index of the evaluated students.
The evaluation encompasses various factors such as learning achievements, school management type, geographic area, and socioeconomic status of students. To assess the learning gap between urban and rural students, data from the entire sample was used, as there were no missing data, and no exclusions were made. If you need further assistance with analyzing or interpreting the data, feel free to provide more specific details or questions. The information has also been filtered at the district level reported by the Ministry of Education of Peru and UNDP, which are shown in Table 1,
Results
The mathematics achievement gap between urban and rural areas has been the subject of significant research and analysis. The study revealed that even after controlling for socioeconomic variables and school contributions, the urban area maintained significantly higher learning performance in mathematics compared to the rural area. This positive gap in favor of the urban area was estimated at 63.28 points, indicating a persistent disparity in learning performance between urban and rural areas.
The outcomes of the analysis conducted using the Oaxaca-Blinder triple decomposition (Equation 4) and the provided dataset are as follows: Endowments: These represent the observable attributes of individuals that contribute to the learning achievement gap. In this instance, the data illustrates that disparities in endowments contribute 52.25 points to the learning achievement gap between urban and rural students. Coefficients: These delineate how differences in observable attributes influence the learning achievement gap. Here, the coefficients suggest that discrepancies in observable attributes account for 19.91 points of the learning achievement gap between the two groups. Interactions: This component encapsulates how disparities in endowments affect the learning achievement gap between the groups divergently. In this context, the interaction manifests a value of −8.89, indicating a negative correlation between observable attributes and the learning achievement gap between urban and rural students. These findings imply that variances in endowments (observable attributes) and coefficients (the impact of those attributes on learning achievement) are pivotal in elucidating the learning achievement gap between urban and rural students. Moreover, the interaction between endowments and the learning achievement gap suggests that disparities in observable attributes wield distinct effects on the two groups.
On the other hand, according to the double decomposition of the learning achievement gap using the Oaxaca-Blinder methodology, it is shown that the difference of 63.28 points in mathematics achievement between urban and rural areas, in favor of urban area students, consists of 54.96 points explained by observable variables and 8.32 points unexplained (Table 2).
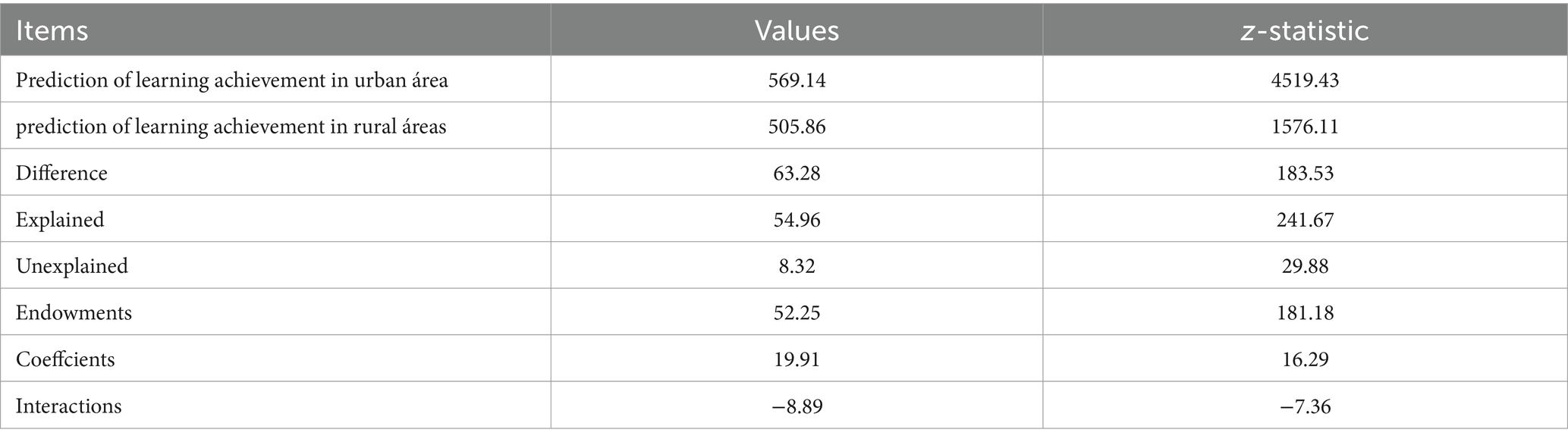
Table 2. Learning achievement gap between urban and rural areas through the Oaxaca-Blinder methodology.
The Oaxaca-Blinder two-part decomposition method decomposes the learning achievement gap into two parts. One part attributed to the explained part related to observable characteristics (such as socioeconomic characteristics of students, school resources and teachers’ qualifications) and another part that remains unexplained, often attributed to factors such as discrimination, cultural differences or unexplained variables (Observed Equation 9). The Oaxaca-Blinder model stands as a widely embraced approach for dissecting disparities in educational achievement between distinct groups, such as urban and rural cohorts, into discernible segments of explained and unexplained variations. This model serves as a pivotal tool in delineating the portion of the discrepancy that can be ascribed to observable differences, accounting for 86.85% (54.96/63.28), and the segment entwined with discriminatory factors, totaling 13.14% (8.32/63.28). The twofold decomposition derived from the Oaxaca-Blinder methodology (Table 3; Equation 9) elucidates that among the observable variables, socioeconomic determinants elucidate 56.40% of the variance, trailed by educational inputs at 12.15%. Notably, key socioeconomic variables exerting significant influence include the years of education among individuals aged 25 and over (EDU25), contributing 37.32%, followed by the socioeconomic index (ISH) at 33.73%, and the per capita family income of the district (IPC) at 23.22%. Remarkably, the years of education among the adult populace notably mitigate the disparity, while household socioeconomic indices and district-level family income per capita exacerbate it. Among educational inputs, access to basic amenities such as water, sanitation, and electricity contributes 11.19%, followed by the proportion of school facilities in satisfactory condition at 6.98% (Table 3).
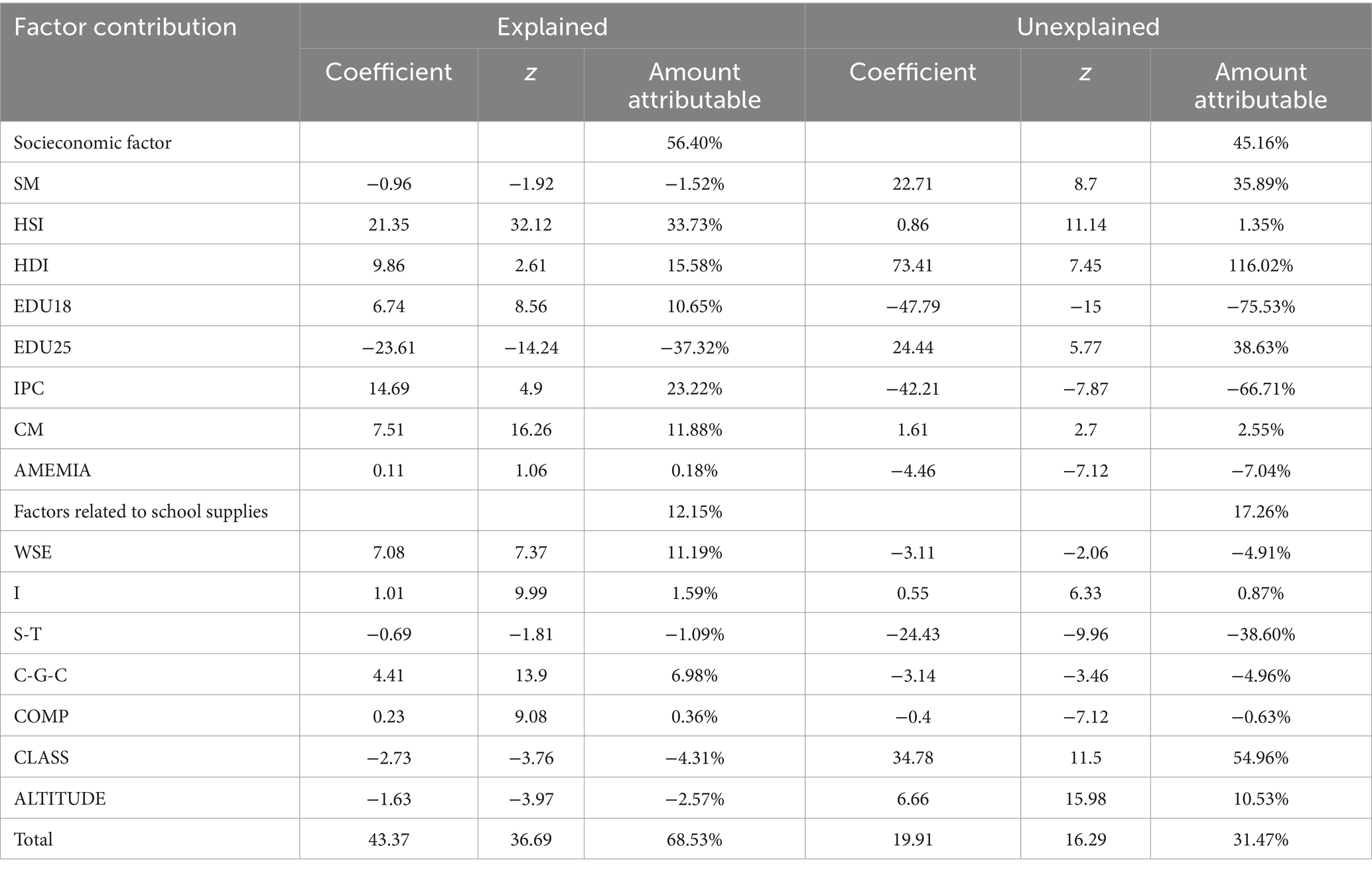
Table 3. Explained and unexplained component of the gap in mathematics learning achievement between urban and rural áreas.
The estimated results shown in Equation 9 (Table 3) correspond to Equation 6, which describes the contribution of different factors to mathematics learning achievement between urban and rural areas, divided into explained and unexplained components.
Socioeconomic factors explain 56.40% of the gap in mathematics achievement between urban and rural areas. The main factors contributing to this explained portion include the Health Situation Index (HSI) with 33.73% and the Human Development Index (HDI) with 15.58%. In contrast, some factors, like the percentage of adults with higher education (EDU25), contribute negatively to this explained portion with −37.32%. Regarding the unexplained portion, socioeconomic factors account for 45.16% of the gap. The HDI is the largest contributor with 116.02%, followed by SM with 35.89%.
The positive contribution of the HDI at 116.02% reflects its ability to mitigate the negative impacts of other factors that contribute negatively to the unexplained portion. Essentially, when combined with the negative contributions of factors such as EDU18 and IPC, the HDI’s positive impact results in a net percentage that exceeds 100%. This does not imply that more than 100% of the gap is explained solely by these factors, but rather illustrates their combined effect after accounting for positive and negative influences across the model.
Factors like EDU18 (secondary education completion for those over 18 years old) and IPC have significant negative contributions, with −75.53% and −66.71%, respectively.
School resources-related factors explain 12.15% of the gap in mathematics achievement. Among these, the availability of school materials (WSE) is the largest contributor with 11.19%, followed by school infrastructure (I) with 1.59%. However, some factors have negative contributions, such as class size (CLASS) with −4.31% and altitude (ALTITUDE) with-2.57%. In the unexplained portion, school resources contribute 17.26% to the gap. Here, class size (CLASS) is a significant factor with a contribution of 54.96%, while other factors, such as the student-to-computer ratio (COMP) and altitude (ALTITUDE), have smaller contributions, with-0.63 and 10.53%, respectively.
In total, the explained factors account for 68.53% of the gap in mathematics achievement between urban and rural areas, while the unexplained factors account for 31.47%. The explained portion is mainly attributed to socioeconomic factors, while in the unexplained portion, both socioeconomic factors and school resources-related factors play important roles, with major contributions from specific factors such as HDI and class size.
Table 4 shows that in all regions, with the exception of Madre de Dios and Moquegua, the explained part of the learning achievement gap is greater than the unexplained part.
The table presents the explained and unexplained learning achievement gap by departments, expressed in both points and percentages, using the Oaxaca-Blinder methodology. The explained column shows the explained contribution to the learning achievement gap, while the unexplained column indicates the unexplained portion of the gap. In the in points column, absolute values of the explained and unexplained gap are displayed. In the in percentage column, percentages of the explained and unexplained gap relative to the total learning achievement gap are shown. For example, in the Amazonas department, out of the total gap of 47.50 points, 40.52 points are explained by observable variables, representing 85.30% of the total gap. Meanwhile, 6.98 points are unexplained, representing 14.70% of the total gap. These results allow for the identification of the contribution of observable variables (such as socioeconomic factors, access to basic services, among others) and unobservable variables (such as discrimination, institutional inequality, among others) to the learning achievement gap across different departments.
The triple decomposition (Table 5) shows that the gap in learning performance in mathematics is explained in 82.58% (52.25/63.28) by the endowment effect, in 31.47% (19.91/63.28) due to the coefficient effect and-14.04% (−8.89/63.28) due to the interaction effect. That is, the gap between the urban environment is explained mainly by the endowment effect, followed by the coefficient effect and the interaction effect. The endowment effect, which accounted for the largest portion of the difference, suggests that inherent characteristics and resources available in urban and rural areas significantly impact mathematics achievement (Li et al., 2017). Additionally, the coefficient effect and interaction effect identified in the decomposition analysis indicate the influence of specific factors and the interplay between different variables on mathematics achievement in urban and rural settings (Li et al., 2017).
The socioeconomic variables that most contribute to increasing the gap in favor of students from urban areas are: district human development index (65.26%) and household socioeconomic index (46.26%). While the variables that reduce the gap are: the average years of education of the population aged 25 and over and per capita family income at the district level (−16.33%). On the school supplies side, the main variables that contribute to increasing the gap are: class size (10.26%), the provision of water, drainage and electricity (7.92%) and school premises in good condition (5.14%).
Table 6 provides insights into the disparities in educational achievement between urban and rural areas across diverse departments. Positive differentials signify superior performance in urban settings, whereas negative differentials indicate better outcomes in rural regions. For instance, in Ancash, urban students achieve 557.58 points compared to 491.72 points for their rural counterparts, resulting in a 65.87-point advantage for urban areas. The “Decomposition” column further dissects this gap into three fundamental components: Endowments, Coefficients, and Interaction. Endowments delineate variances in initial resources or characteristics between urban and rural students. For instance, in Ancash, urban areas exhibit endowments of 52.58 points, while rural areas demonstrate 16.80 points. Coefficients elucidate the impact of these initial disparities on educational outcomes. In the context of Ancash, coefficients amount to 16.80 points for urban areas and − 3.51 points for rural areas. The Interaction term delineates any supplementary influence stemming from the interplay between urban/rural status and other factors. In Ancash, this interaction is represented as-3.51 points, suggesting an additional effect beyond endowments and coefficients. Consistently, the data reveal a prevailing gap in educational performance favoring urban locales across most departments, with distinctive contributions from endowments, coefficients, and interactions in each scenario.
Discussion
The findings of this study have significant implications for public policy. Firstly, observable characteristics account for 83% of the learning achievement gap between urban and rural environments in Peru. Secondly, this gap is largely explained by socioeconomic characteristics and school resources across all departments in Peru, with the exceptions of Madre de Dios and Moquegua. To address the learning achievement gap between urban and rural areas in Peru, it is crucial to consider the impact of socioeconomic characteristics and school resources. Disparities in educational performance between urban and rural areas stem from a combination of socioeconomic factors and the availability of educational resources (Şirin, 2005; Sun and Du, 2021).
Several studies support the hypothesis that the level of socioeconomic status in both the household and the surrounding environment contributes to the achievement gap between urban and rural students. Reeves (2012) emphasizes the impact of family socioeconomic status on the rural math achievement gap in high school, indicating a correlation between socioeconomic status and academic achievement. Similarly, Ababneh and Kodippili (2020) found that students attending rural schools were not performing as well as students from urban schools, highlighting the association of variables with the mathematics achievement gap between rural and urban students. The difference in learning achievement between urban and rural areas according to socio-economic factors and school resources has been found in other studies (Murphy, 2019; Dean et al., 2023), mainly in rural áreas (Dean et al., 2023), where students from low socio-economic backgrounds often face challenges such as limited access to resources, inadequate healthcare, and unstable home environments. Urban and rural areas often differ in terms of resources, opportunities, and community support. Urban areas may have more access to educational resources, extracurricular activities, and cultural institutions, which can positively impact student achievement. On the other hand, rural areas may face challenges such as limited access to quality schools, transportation barriers, and a lack of diverse learning opportunities.
Other studies emphasize the importance of socioeconomic variables, such as the Human.
Development Index (HDI) and household socioeconomic status, in the learning gap favoring urban students. For example, Sharaf and Rashad (2016) found that these variables are determinants of regional inequalities in child malnutrition in Egypt, Jordan, and Yemen. Additionally, another study reveals that socioeconomic factors, such as access to educational resources, significantly influence the difference in educational performance between urban and rural areas. Sun and Du (2021) highlight that teacher training can reduce this educational gap by providing better tools and methodologies for rural teachers, thus improving the academic performance of students in these areas.
Furthermore, the study highlights that the portion of the gap in educational performance attributable to observable factors exceeds the unexplained portion in most departments, emphasizing the importance of addressing these disparities (Jacobs and Harvey, 2010).
Research has shown that these factors play an instrumental role in adolescents’ intellectual and social–emotional development (Eccles and Roeser, 2011). Additionally, the efficiency of secondary schools and the appropriate allocation of public resources to support diverse students have been highlighted as crucial evidence for policymakers and stakeholders (Muñoz, 2016). Furthermore, the socioeconomic environment has been found to have a significant effect on inferential reasoning of students, emphasizing the importance of public policies in addressing these disparities (Flores-Mendoza et al., 2017).
Moreover, the gender gap in educational achievement has been studied in relation to school context, indicating that school resources and practices can influence boys’ and girls’ performance differently (Hek et al., 2017). This highlights the importance of considering the specific needs of different student groups when addressing educational disparities. Additionally, the effect of bonuses on teacher retention and student learning in rural schools has been investigated, providing insights into the indirect effects of incentives aimed at attracting teachers to disadvantaged schools (Castro and Esposito, 2022).
Furthermore, the role of contextual and compositional characteristics of schools in health inequalities has been examined, emphasizing the need to consider the broader impact of school environments on student well-being (Herke et al., 2020). Additionally, the contribution of early childhood and schools to cognitive gaps has been highlighted, emphasizing the importance of both home a learning outcomes (Castro and Rolleston, 2018).
In the specific context of Peru, the study of teachers’ pedagogical content knowledge and mathematics achievement of students has been conducted, shedding light on the role of teachers in influencing student achievement (Cueto et al., 2016). Additionally, the importance of parental involvement in education as a moderator of family and neighborhood socioeconomic context on school readiness among young children has been emphasized, indicating the significance of family and community support in educational outcomes (Kingston et al., 2013).
The findings of this study have important implications for public policy. The significant influence of socioeconomic characteristics and school resources on the achievement gap suggests that targeted interventions in these areas could help reduce the gap. By addressing the disparities in resources and support available to students in rural areas, policymakers can work towards narrowing the achievement gap in mathematics learning.
The study’s findings align with previous research that has emphasized the impact of socioeconomic status on academic achievement. It has been noted that family socioeconomic status plays a crucial role in shaping students’ educational outcomes, with lower family socieconomic status being associated with poorer academic achievement (Şirin, 2005).
The achievement gap between urban and rural students is a multifaceted issue influenced by various factors. Research indicates that disparities in child nutrition, income, and educational resources play a significant role in perpetuating this gap (Montgomery and Hewett, 2005; Sicular et al., 2008; Baker and Greer, 2010; Pribesh et al., 2011; Jeynes, 2012; Liao et al., 2013; Liu et al., 2013; Srinivasan et al., 2013; Yang, 2015; Khan et al., 2017; Liddle, 2017; Wang and Tao, 2017; Mason et al., 2019; Tine, 2019; Wang et al., 2019; Ju et al., 2022; Masud, 2022).
Factors such as family socioeconomic status, educational expectations, and access to educational resources have been identified as key contributors to the achievement gap (Baker and Greer, 2010; Pribesh et al., 2011; Tine, 2019). Furthermore, the urban-rural income gap and inequality in the allocation of educational resources have been highlighted as contributing factors to the learning achievement gap (Sicular et al., 2008; Liddle, 2017; Wang and Tao, 2017; Wang et al., 2019).
Furthermore, the quality of school management, household socioeconomic development, and access to essential resources such as water, electricity, and educational facilities have been identified as critical determinants of the achievement gap (Pribesh et al., 2011; Srinivasan et al., 2013; Wang et al., 2019). It is also important to consider the impact of cultural and ethnic differences, as well as the distinct cognitive and academic profiles of students from rural versus urban poverty (Jeynes, 2012; Mason et al., 2019).
The learning achievement gap in mathematics between urban and rural areas in Peru is a significant issue, with urban students consistently outperforming their rural counterparts. This gap varies across different departments of Peru, with the largest gap observed in the mountainous regions such as Ancash, Junín, and Cusco, and the smallest gap in coastal and jungle areas like Tacna, Madre de Dios, Ica, and San Martin. The disparity in learning achievement is a cause for concern and requires attention to ensure equitable access to quality education for all students, regardless of their geographical location. Table 5 shows the learning achievement gap in mathematics between urban and rural areas. The results suggest that there is a positive gap in favor of students from urban areas in all departments of Peru, which varies between 8.09 points in the department of Tacna and 65.87 points in the department of Ancash. The regions with the greatest gap are located in the mountains of Peru (Ancash, Junín and Cusco); while those with the smallest gap are found in the Coast and Jungle (Tacna, Madre de Dios, Ica and San Martin). The regions with the greatest gap are located in the mountains of Peru, while those with the smallest gap are found in the Coast and Jungle.
The learning achievement gap in mathematics between urban and rural areas in Peru is a significant concern, with urban students consistently outperforming their rural counterparts. This gap varies across different departments of Peru, with the largest gap observed in the mountainous regions such as Ancash, Junín, and Cusco, and the smallest gap in coastal and jungle areas like Tacna, Madre de Dios, Ica, and San Martin (Ramsden and Entwistle, 1981). The disparity in learning achievement is a cause for concern and requires attention to ensure equitable access to quality education for all students, regardless of their geographical location. This issue is not unique to Peru, as similar disparities have been observed in other countries, such as China, where increasing attention has been paid to regional differences in education between and within provinces (Qian and Smyth, 2007). Furthermore, the migration from rural to urban areas, fueled by political instability in rural regions, has also been identified as a contributing factor to the disparities in learning achievement.
Addressing the urban-rural learning achievement gap requires a multifaceted approach, including focused programmatic interventions in rural settings to improve access to quality education. Furthermore, the role of education in reducing income inequality within urban and rural areas has been highlighted, emphasizing the need to reduce not only the urban-rural educational gap but also the educational inequalities within urban and rural áreas (Mahmud and Akita, 2018).
Furthermore, the influence of urbanization on the urban-rural gap in elementary education has been recognized as a significant variable warranting further study. Urbanization has been identified as a key factor affecting the social process and its impact on the urban-rural gap in elementary education is considered worthy of additional investigation (Ju et al., 2022). Additionally, the concentration of industries such as culture, education, science, and health in urban areas has been identified as a contributing factor to the widening income gap between urban and rural áreas (Liu and Long, 2021).
Understanding the educational achievement gap between urban and rural areas is crucial for designing effective interventions to address educational inequalities. This article reviews the psychopedagogical implications and future research directions of this gap. We examine how the Oaxaca-Blinder methodology elucidates the contributions of observable variables to the gap and discuss its implications for educational policy and practice. Additionally, we propose future research directions, including longitudinal studies to assess the long-term impact of interventions and investigations into the role of other factors, such as teacher quality and family environment, in the achievement gap.
The disparity in educational achievement between urban and rural areas is a persistent challenge in education systems worldwide. While numerous studies have documented this gap, understanding its underlying causes and implications is essential for developing targeted interventions to address it. In this article, we explore the psychopedagogical implications of the educational achievement gap between urban and rural areas and outline potential future research directions to inform policy and practice.
The Oaxaca-Blinder methodology provides valuable insights into the factors contributing to the educational achievement gap between urban and rural areas. By decomposing the gap into explained and unexplained components, this approach helps identify the role of observable variables, such as socioeconomic factors and school resources, in driving disparities in educational outcomes. Understanding these factors can inform the development of evidence-based interventions aimed at narrowing the gap.
Despite advances in understanding the educational achievement gap, several areas warrant further investigation. Longitudinal studies are needed to assess the effectiveness of interventions aimed at reducing the gap over time and to identify potential long-term outcomes for students from urban and rural areas. Additionally, research exploring the influence of other factors, such as teacher quality, family support, and community resources, on the achievement gap is essential for developing comprehensive strategies to address educational inequalities.
Addressing the educational achievement gap between urban and rural areas requires a multifaceted approach that considers the complex interplay of individual, family, school, and community factors. By exploring the psychopedagogical implications of this gap and identifying future research directions, this article aims to contribute to ongoing efforts to promote educational equity and improve outcomes for all students, regardless of their geographic location.
Conclusion
To address the conclusion that there is a positive gap in favor of urban students in mathematics achievement in Peru, it is essential to consider the impact of various factors on student learning outcomes. The positive gap in favor of urban students in mathematics achievement in Peru is influenced by various factors, including teaching methods, teacher-student rapport, socioeconomic status, and disparities in the quality of education between rural and urban schools. Addressing these disparities and implementing targeted interventions, such as improving teaching quality and investing in educational reforms, may help reduce the achievement gap and promote equitable learning outcomes for all students.
In the context of educational achievement in mathematics between students from urban and rural areas, the Oaxaca-Blinder decomposition has been applied to reveal that observable characteristics account for a substantial portion (86.85%) of the achievement gap. This approach provides a comprehensive understanding of the observed gap, shedding light on the influence of various factors such as access to resources, teaching quality, and socio-economic background. By quantifying the contribution of observable characteristics to the achievement gap, this methodology offers valuable insights for policymakers and educators to design targeted interventions aimed at reducing disparities in mathematics achievement between urban and rural students.
Based on the Oaxaca Blinder decomposition methodology, the gap in mathematics achievement was explained by the effect of endowment with 82.57%, the coefficient effect with 31.46%, and the interaction effect with −14.05%. The learning achievement gap is influenced by a complex interplay of socioeconomic factors at both the household and district levels. These factors include income, parental education, access to public services, and class size. Addressing these disparities is crucial for narrowing the achievement gap and promoting educational equity.
Understanding the educational achievement gap between urban and rural areas is crucial for addressing educational inequalities and comprehensively considering the psychopedagogical implications associated with this gap. By reviewing these implications and proposing future research directions, policymakers and educators can design evidence-based interventions that account for the unique needs of students from diverse geographic backgrounds. The utilization of the Oaxaca-Blinder methodology provides valuable insights into the psychopedagogical aspects of the gap by elucidating the contributions of observable variables, such as socioeconomic factors and school resources, to educational outcomes. Despite progress, further investigation into psychopedagogical factors is warranted, including longitudinal studies to assess intervention effectiveness and exploring the influence of factors like teacher quality and family environment. By recognizing and addressing these implications, policymakers and educators can advance efforts to promote educational equity and improve outcomes for all students, regardless of their geographic location.
In conclusion, addressing the learning achievement gap between urban and rural environments in Peru requires a comprehensive understanding of the multifaceted factors influencing student outcomes. Policymakers and stakeholders need to consider the interplay of socioeconomic characteristics, school resources, teacher quality, and community involvement to develop effective interventions and policies aimed at reducing educational disparities.
Data availability statement
Publicly available datasets were analyzed in this study. This data can be found here: http://umc.minedu.gob.pe/evaluaciones-censales/.
Author contributions
RR: Writing – original draft. RP: Methodology, Writing – review & editing. RA: Validation, Writing – review & editing. CQ: Conceptualization, Writing – original draft. EC-Z: Data curation, Writing – review & editing.
Funding
The author(s) declare that financial support was received for the research, authorship, and/or publication of this article. This work was supported by the Universidad Nacional del Altiplano de Puno.
Conflict of interest
The authors declare that the research was conducted in the absence of any commercial or financial relationships that could be construed as a potential conflict of interest.
Publisher’s note
All claims expressed in this article are solely those of the authors and do not necessarily represent those of their affiliated organizations, or those of the publisher, the editors and the reviewers. Any product that may be evaluated in this article, or claim that may be made by its manufacturer, is not guaranteed or endorsed by the publisher.
References
Ababneh, E. G., and Kodippili, A. (2020). Investigation the association of some variables with mathematics achievement gap between rural and urban Jordanian students. J. Educ. Pract. 11, 147–158. doi: 10.7176/JEP/11-21-17
Agrawal, T. (2013). Gender and caste-based wage discrimination in India: some recent evidence. J. Labour Mark. Res. 47, 329–340. doi: 10.1007/s12651-013-0152-z
Akita, T., and Miyata, S. (2013). The roles of location and education in the distribution of economic well-being in Indonesia: hierarchical and non-hierarchical inequality decomposition analyses. Lett. Spat. Resour. Sci. 6, 137–150. doi: 10.1007/s12076-013-0093-8
Arteaga, I., and Glewwe, P. (2019). Do Community factors matter? An analysis of the achievement gap between indigenous and non-indigenous children in Peru. Int. J. Educ. Dev. 65, 80–91. doi: 10.1016/j.ijedudev.2017.08.003
Attia, J., Jones, M., and Hure, A. (2017). Deconfounding confounding part 1: traditional explanations. Med. J. Aust. 206, 244–245. doi: 10.5694/mja16.00491
Backer, F. D., Lombaerts, K., Mette, T. D., Buffel, T., and Elias, W. (2012). Creativity in artistic education: introducing artists into primary schools. Int. J. Art Design Educ. 31, 53–66. doi: 10.1111/j.1476-8070.2012.01715.x
Baker, R. D., and Greer, F. R. (2010). Diagnosis and prevention of Iron deficiency and Iron-deficiency Anemia in infants and young children (0–3 years of age). Pediatrics 126, 1040–1050. doi: 10.1542/peds.2010-2576
Bauer, T., and Sinning, M. (2008). An extension of the blinder–Oaxaca decomposition to nonlinear models. Asta Adv. Stat. Anal. doi: 10.1007/s10182-008-0056-3
Befort, C. A., Nazir, N., and Perri, M. G. (2012). Prevalence of obesity among adults from rural and urban areas of the United States: findings from NHANES (2005-2008). J. Rural. Health 28, 392–397. doi: 10.1111/j.1748-0361.2012.00411.x
Berloffa, G., and Paolini, F. (2022). Going “beyond the mean” in Analysing immigrant health disparities. Demogr. Res. 47, 161–198. doi: 10.4054/DemRes.2022.47.7
Berri, D. J., Burdekin, R. C. K., and Deutscher, C. (2021). Nationality effects on the allocation of playing time in the Chinese basketball association: Xenophilia or xenophobia? J. Sports Econ. 23, 156–174. doi: 10.1177/15270025211034824
Budianur, B., and Setiawan, A. (2021). Participation model of Society in Education at Islamic elementary school. J. Al-Qiyam 2, 128–139. doi: 10.33648/alqiyam.v2i1.134
Caponera, E., and Losito, B. (2016). Context factors and student achievement in the IEA studies: evidence from TIMSS. Large Scale Assess. Educ. 4:12. doi: 10.1186/s40536-016-0030-6
Castro, J. F. (2020). Revisiting the contribution of schools to cognitive gaps: evidence from Peru. Rev. Dev. Econ. 24, 1256–1278. doi: 10.1111/rode.12713
Castro, J. F., and Esposito, B. (2022). The effect of bonuses on teacher retention and student learning in rural schools: a story of spillovers. Educ. Finan. Policy 17, 693–718. doi: 10.1162/edfp_a_00348
Castro, J. F., and Rolleston, C. (2018). The contribution of early childhood and schools to cognitive gaps: new evidence from Peru. Econ. Educ. Rev. 64, 144–164. doi: 10.1016/j.econedurev.2018.03.009
Chatterjee, A., and Burns, S. (2021). Links between disadvantage and educational achievement in a low-income urban setting in Bangalore, India. Int. J. Psychol. Educ. Stud. 8, 223–236. doi: 10.52380/ijpes.2021.8.4.620
Chen, M., Lu, K., and Liang, E., (2017). Decomposition analysis of China’s industry income gap-based on macroscopic perspective.
Cueto, S., León, J., Sorto, M. A., and Miranda, A. (2016). Teachers’ pedagogical content knowledge and mathematics achievement of students in Peru. Educ. Stud. Math. 94, 329–345. doi: 10.1007/s10649-016-9735-2
Dean, J., Roberts, P., Downes, N., and Goldsmith, A. (2023). The spatial implications of academic achievement in year 12: rethinking discourses of disadvantage in rural locations. Aust. J. Educ. 67, 94–114. doi: 10.1177/00049441231155708
Djibu, R., and Duludu, U. A. (2020). Impact of the work environment and work motivation in influencing the performance of non-formal educators. J. Nonformal Educ. 6, 92–100. doi: 10.15294/jne.v6i1.24170
Dwiwarna,, and Rahadian, R. B. (2018). The Most considered type of student characteristics by primary school teacher. Int. J. Integrat. Technol. Educ. 7, 29–42. doi: 10.5121/ijite.2018.7303
Eccles, J. S., and Roeser, R. W. (2011). Schools as developmental contexts during adolescence. J. Res. Adolesc. 21, 225–241. doi: 10.1111/j.1532-7795.2010.00725.x
Fairlie, R. W. (2005). An extension of the blinder-Oaxaca decomposition technique to logit and Probit models. J. Econ. Soc. Meas. 30, 305–316. doi: 10.3233/JEM-2005-0259
Firpo, S., Fortin, N. M., and Lemieux, T. (2009). Unconditional Quantile Regressions. Econometrica 77, 953–973. doi: 10.3982/ecta6822
Flores-Mendoza, C., Benigno Saraiva, R., Vilela Câmara, G. C., Guimarães Lopes, W. M., Carvalho Pereira Passos, A. P., Valladão Pires Gama, A. M., et al. (2017). Socioeconomic environment effect on inferential reasoning of Latin American students. Salud Mental 40, 183–190. doi: 10.17711/SM.0185-3325.2017.024
Fonseca, R., Mullen, K. J., Zamarro, G., and Zissimopoulos, J. (2012). What explains the gender gap in financial literacy? The role of household decision making. J. Consum. Aff. 46, 90–106. doi: 10.1111/j.1745-6606.2011.01221.x
Fu, X., Sun, Q., Sun, C., Xu, F., and He, J. (2021). Urban-rural differences in catastrophic health expenditure among households with chronic non-communicable disease patients: evidence from China family panel studies. BMC Public Health 21:874. doi: 10.1186/s12889-021-10887-6
Gadsden, V. L., and Dixon-Román, E. J. (2016). “Urban” schooling and “urban” families. Urban Educ. 52, 431–459. doi: 10.1177/0042085916652189
Garira, E. (2020). A proposed unified conceptual framework for quality of education in schools. SAGE Open 10:215824401989944. doi: 10.1177/2158244019899445
Gimbert, B., Cristol, D., and Sene, A. M. (2007). The impact of teacher preparation on student achievement in algebra in a “hard-to-staff” urban PreK-12-university partnership. Sch. Eff. Sch. Improv. 18, 245–272. doi: 10.1080/09243450601147528
González Canché, M. S. (2023). The geography of mathematical (dis)advantage: an application of multilevel simultaneous autoregressive (MSAR) models to public data in education research. AERA Open 9, 1–38. doi: 10.1177/23328584231198452
Hek, M. V., Kraaykamp, G., and Pelzer, B. (2017). Do schools affect girls’ and boys’ Reading performance differently? A multilevel study on the gendered effects of school resources and school practices. Sch. Eff. Sch. Improv. 29, 1–21. doi: 10.1080/09243453.2017.1382540
Herke, M., Moor, I., Winter, K., Hoffmann, S., Spallek, J., Hilger-Kolb, J., et al. (2020). Role of contextual and compositional characteristics of schools for health inequalities in childhood and adolescence: protocol for a scoping review. BMJ Open 10:e038999. doi: 10.1136/bmjopen-2020-038999
Irvin, M., Byun, S.-Y., Smiley, W. S., and Hutchins, B. C. (2017). Relation of opportunity to learn advanced math to the educational attainment of rural youth. Am. J. Educ. 123, 475–510. doi: 10.1086/691231
Jackson, J., and VanderWeele, T. J. (2018). Decomposition analysis to identify intervention targets for reducing disparities. Epidemiology 29, 825–835. doi: 10.1097/EDE.0000000000000901
Jacobs, N., and Harvey, D. (2010). The extent to which teacher attitudes and expectations predict academic achievement of final year students. Educ. Stud. 36, 195–206. doi: 10.1080/03055690903162374
Jann, B. (2008). The blinder–Oaxaca decomposition for linear regression models. Stata J. Promot. Commun. Statist. Stata. doi: 10.1177/1536867X0800800401
Jeynes, W. H. (2012). School choice and the achievement gap. Educ. Urban Soc. 46, 163–180. doi: 10.1177/0013124512447101
Ju, F., Wang, S., and Lin, W. (2022). Nonlinear effect of urbanization on the gap between urban and rural elementary education in China. Math. Probl. Eng. 2022, 1–10. doi: 10.1155/2022/7025433
Khan, J., Ahmed, S., and Evans, T. (2017). Catastrophic healthcare expenditure and poverty related to out-of-pocket payments for healthcare in Bangladesh—an estimation of financial risk protection of universal health coverage. Health Policy Plan. 32, 1102–1110. doi: 10.1093/heapol/czx048
Kingston, S., Huang, K. Y., Calzada, E. J., Dawson-McClure, S., and Brotman, L. M. (2013). Parent involvement in education as a moderator of family and neighborhood socioeconomic context on school readiness among young children. J. Commun. Psychol. 41, 265–276. doi: 10.1002/jcop.21528
Lee, G. Y., and Um, Y. J. (2021). Factors affecting obesity in urban and rural adolescents: demographic, socioeconomic characteristics, health behavior and health education. Int. J. Environ. Res. Public Health 18:2405. doi: 10.3390/ijerph18052405
Li, J., Shi, L., Li, S., Xu, L., Qin, W., and Wang, H. (2017). Urban-rural disparities in hypertension prevalence, detection, and medication use among Chinese adults from 1993 to 2011. Int. J. Equity Health 16:50. doi: 10.1186/s12939-017-0545-7
Liao, P., Chang, H., Wang, J.-H., and Horng, T.-H. (2013). Do rural students really perform worse than urban students do? Empirical evidence from a university entrance program in <scp>T</Scp>aiwan. Rural. Sociol. 78, 109–131. doi: 10.1111/j.1549-0831.2012.00096.x
Liddle, B. (2017). Urbanization and inequality/poverty. Urban Sci. 1:35. doi: 10.3390/urbansci1040035
Liu, H., Fang, H., and Zhao, Z. (2013). Urban-rural disparities of child health and nutritional status in China from 1989 to 2006. Econ. Hum. Biol. 11, 294–309. doi: 10.1016/j.ehb.2012.04.010
Liu, Y., and Long, C. (2021). Urban and rural income gap: does urban spatial form matter in China? SAGE Open 11:215824402110022. doi: 10.1177/21582440211002215
Luh, Y.-H., and Wei, M.-F. (2018). Distributional effect of the farmer pension program in Taiwan. China Agric. Econ. Rev. 11, 180–205. doi: 10.1108/caer-05-2017-0104
Mahmud, S. S., and Akita, T. (2018). Urban and rural dimensions of the role of education in income inequality in Bangladesh. Rev. Urban Reg. Dev. Stud. 30, 169–183. doi: 10.1111/rurd.12089
Marks, G. (2010). Improvements over the educational career of immigrant students. Aust. J. Educ. 54, 133–154. doi: 10.1177/000494411005400203
Mason, C. P., Hughey, A. W., and Burke, M. G. (2019). Closing the achievement gap in P-12 schools by implementing advanced leadership training and comprehensive school counseling programs. J. Educ. Soc. Policy 6. doi: 10.30845/jesp.v6n3p6
Masud, A. (2022). A study of the relationship between self-regulated learning strategies and academic achievement of secondary school learners. Pakist. Lang. Hum. Rev. 6, 802–816. doi: 10.47205/plhr.2022(6-ii)69
Matuszewska-Janica, A. (2018). Differences in Men’s and Women’s wages in the education sector in the Baltic Sea region states. Folia Oecon. Stetinensia 18, 157–168. doi: 10.2478/foli-2018-0012
May, (2022). The implementation of independent learning activity units in the semester credit system improves learning achievement. Int. J. Educ. Comp. Stud. 2, 1–9. doi: 10.35870/ijecs.v2i1.616
McEwan, P. J., and Marshall, J. H. (2004). Why does academic achievement vary across countries? Evidence from Cuba and Mexico. Educ. Econ. 12, 205–217. doi: 10.1080/0964529042000258572
Mondal, D. (2021). Basic service provisioning in Peri-urban India: a regional perspective from Kolkata Metropolis. Indian J. Hum. Dev. 15, 97–116. doi: 10.1177/09737030211000930
Montgomery, M. R., and Hewett, P. C., (2005). Poverty and children’s schooling in urban and rural senegal.
Muñoz, J. M. (2016). Distribución Local De La Inversión De Decisión Regional: El Caso Del FNDR en Chile 1997-2012. Rev. Estudios Polít. Públicas 1, 152–169. doi: 10.5354/0719-6296.2015.38433
Muñoz, D. A., and Queupil, J. P. (2016). Assessing the efficiency of secondary schools in Chile: a data envelopment analysis. Qual. Assur. Educ. 24, 306–328. doi: 10.1108/QAE-05-2015-0022
Murawska, I., Przyborowska, B., Kopińska, V., and Błajet, P. (2019). Settlement type and educational effectiveness of polish schools on the example of the Kujawsko-Pomorskie Voivoideship. Bullet. Geograp. Soc. Econ. Ser. 43, 45–55. doi: 10.2478/bog-2019-0003
Murphy, S. (2019). School location and socioeconomic status and patterns of participation and achievement in senior secondary mathematics. Math. Educ. Res. J. 31, 219–235. doi: 10.1007/s13394-018-0251-9
Nagi, K. S., and Gummadi, S. (2022). Unveiling gender gap in educational performance: evidence from India. Indian Econ. J. 70, 472–489. doi: 10.1177/00194662221104755
Nugba, R. M., Amedahe, F. K., and Asamoah-Gyimah, K. (2022). Role of input, process factors and school context on the effectiveness of junior high school education in the central region of Ghana. Int. J. Sec. Educ. 10:1. doi: 10.11648/j.ijsedu.20221001.11
Nyirenda, M. K. (2021). Impact of resource distribution and availability on primary schools pupil learning outcomes: case study of Chintheche education zone, Nkhata Bay, Malawi. J. Dev. Commun. Stud. 8, 239–260. doi: 10.4314/jdcs.v8i1.12
Odera, O., Sika, J. O., and Olel, M. (2022). Selected school based factors’ influence on value addition in secondary education in public secondary schools in Rachuonyo south Sub-County, Kenya. Afr. J. Educ. Pract. 8, 19–33. doi: 10.47604/ajep.1617
Owoeye, J. S., and Yara, P. O. (2011). School location and academic achievement of secondary School in Ekiti State, Nigeria. Asian Soc. Sci. 7. doi: 10.5539/ass.v7n5p170
Paciorek, C. J., Stevens, G. A., Finucane, M. M., and Ezzati, M. (2013). Children’s height and weight in rural and urban populations in low-income and middle-income countries: a systematic analysis of population-representative data. Lancet Glob. Health 1, e300–e309. doi: 10.1016/S2214-109X(13)70109-8
Piyaman, P., Hallinger, P., and Viseshsiri, P. (2017). Addressing the achievement gap. J. Educ. Adm. 55, 717–734. doi: 10.1108/JEA-12-2016-0142
Powell, L. M., Wada, R., Krauss, R., and Wang, Y. (2012). Ethnic disparities in adolescent body mass index in the United States: the role of parental socioeconomic status and economic contextual factors. Soc. Sci. Med. 75, 469–476. doi: 10.1016/j.socscimed.2012.03.019
Powers, D. A., Yoshioka, H., and Yun, M. S. (2011). Mvdcmp: multivariate decomposition for nonlinear response models. Stata J. Promot. Commun. Statist. Stata 11, 556–576. doi: 10.1177/1536867X1201100404
Pribesh, S., Gavigan, K., and Dickinson, G. (2011). The access gap: poverty and characteristics of school library media centers. Libr. Q. 81, 143–160. doi: 10.1086/658868
Qian, X., and Smyth, R. (2007). Measuring regional inequality of education in China: widening coast–inland gap or widening rural–urban gap? J. Int. Dev. 20, 132–144. doi: 10.1002/jid.1396
Ramos, R., Duque, J., and Sandra, N. (2016). Estudios de Economía Aplicada decomposing the rural-urban differential in student achievement in Colombia using PISA microdata. Estudios Econ. Aplicada 34, 379–412. doi: 10.25115/eae.v34i2.3044
Ramsden, P., and Entwistle, N. (1981). Effects of academic departments on students’ approaches to studying. Br. J. Educ. Psychol. 51, 368–383. doi: 10.1111/j.2044-8279.1981.tb02493.x
Reeves, E. B. (2012). The effects of opportunity to learn, family socioeconomic status, and friends on the rural math achievement gap in high school. Am. Behav. Sci. 56, 887–907. doi: 10.1177/0002764212442357
Rivkin, S. G., Hanushek, E. A., and Καιν, J. F. (2005). Teachers, schools, and academic achievement. Econometrica 73, 417–458. doi: 10.1111/j.1468-0262.2005.00584.x
Rudy, R., Silalahi, W. H. H., and Sinaga, T. F. (2021). The influence of online learning on students’ characteristics. Idebahasa 3, 93–108. doi: 10.37296/idebahasa.v3i2.59
Sahlan, A. (2014). Enhancement of culture in education: research on Indonesian high school. Procedia Soc. Behav. Sci. 143, 117–121. doi: 10.1016/j.sbspro.2014.07.371
Sakellariou, C. (2008). Peer effects and the indigenous/non-indigenous early test-score gap in Peru. Educ. Econ. 16, 371–390. doi: 10.1080/09645290802133065
Shala, A., and Latifi, F. (2021). Does socioeconomic status influence achievement? An analysis of the performance of Kosovar students on the 2015 and 2018 PISA assessment. Rev. Element. Izobraževanje 4, 393–408. doi: 10.18690/rei.14.4.393-408.2021
Sharaf, M. F., and Rashad, A. S. (2016). Regional inequalities in child malnutrition in Egypt, Jordan, and Yemen: a blinder-Oaxaca decomposition analysis. Heal. Econ. Rev. 6:23. doi: 10.1186/s13561-016-0097-3
Sicular, T., Yue, X., Gustafsson, B., and Li, S., (2008). The urban-rural income gap and income inequality in China.
Şirin, S. R. (2005). Socioeconomic status and academic achievement: a Meta-analytic review of research. Rev. Educ. Res. 75, 417–453. doi: 10.3102/00346543075003417
Srinivasan, C. S., Zanello, G., and Shankar, B. (2013). Rural-urban disparities in child nutrition in Bangladesh and Nepal. BMC Public Health 13:581. doi: 10.1186/1471-2458-13-581
Steyn, G. M. (2005). Implementing professional development in invitational education. Afr. Educ. Rev. 2, 258–278. doi: 10.1080/18146620508566304
Stoll, L., and Kools, M. (2017). The school as a learning organisation: a review revisiting and extending a timely concept. J. Prof. Capital Commun. 2, 2–17. doi: 10.1108/JPCC-09-2016-0022
Suastini, N. W., and Sumada, I. M. (2022). Character education in school based on local wisdom. Soc. Persp. J. 354, 365–368. doi: 10.53947/tspj.v1i2.106
Sumida, S., and Kawata, K. (2021). An analysis of the learning performance gap between urban and rural areas in sub-saharan africa. S. Afr. J. Educ. 41, 1–17. doi: 10.15700/saje.v41n2a1779
Sun, S., Chen, J., Johannesson, M., Kind, P., Xu, L., Zhang, Y., et al. (2011). Regional differences in health status in China: population health-related quality of life results from the National Health Services Survey 2008. Health Place 17, 671–680. doi: 10.1016/j.healthplace.2011.01.007
Sun, R., and Du, P. (2021). Does teacher training narrow the educational gap between urban and rural students? Empirical evidence from CEPS baseline data. Best Evid. Chin. Educ. 9, 1243–1261. doi: 10.15354/bece.21.ar036
Tadesse, S. E., Mekonnen, T. C., Dewau, R., Zerga, A. A., Kebede, N., Feleke, Y. W., et al. (2023). Urban-rural disparity in stunting among Ethiopian children aged 6–59 months old: a multivariate decomposition analysis of 2019 mini-EDHS. PLoS One 18:e0284382. doi: 10.1371/journal.pone.0284382
Tine, M. (2019). Location matters: distinct cognitive and academic profiles of students from rural versus urban poverty. J. Adv. Educ. Res. 4. doi: 10.22606/jaer.2019.41001
Vujicic, M., and Nasseh, K. (2013). A decade in dental care utilization among adults and children (2001–2010). Health Serv. Res. 49, 460–480. doi: 10.1111/1475-6773.12130
Wang, W., and Tao, Y., (2017). Research on differences of fairness of educational resource allocation between urban and rural areas in China.
Wang, Z., Wang, X., and Liang, L. (2019). Green economic efficiency in the Yangtze River Delta: spatiotemporal evolution and influencing factors. Ecosyst. Health Sustain. 5, 20–35. doi: 10.1080/20964129.2018.1559000
Wulandari, S., Hermansyah, H., and Pratiwi, Y. (2021). The influence of learning motivation on mathematics learning achievement in terms of gender of class VIII students of SMP Negeri 5 Tarakan. J. Educ. Lear. Math. Res. 2, 42–50. doi: 10.37303/jelmar.v2i2.63
Xiang, L., and Stillwell, J. (2023). Rural–urban educational inequalities and their spatial variations in China. Appl. Spat. Anal. Policy 16, 873–896. doi: 10.1007/s12061-023-09506-1
Yang, J. (2015). The effects of urbanization process on urban-rural income gap based on the feasible generalized least squares. Int. J. U-And E-Ser. Sci. Technol. 8, 385–394. doi: 10.14257/ijunesst.2015.8.10.37
Zarei, E., Pakzad, R., Yekta, A., Amini, M., Sardari, S., and Khabazkhoob, M. (2021). Economic inequality in visual impairment: a study in deprived rural population of Iran. J. Curr. Ophthalmol. 33, 165–170. doi: 10.4103/2452-2325.288936
Zhang, J., Deininger, K., Torero, M., and Li, T. (2018). Teachers and urban-rural gaps in educational outcomes. Am. J. Agric. Econ. 100, 1207–1223. doi: 10.1093/ajae/aay009
Keywords: achievement gap, Oxaca-Blinder methodology, public policy intervention, mathematics learning, socioeconomic characteristics, school resources, urban student
Citation: Rojas Apaza R, Paredes RP, Arpi R, Quispe Lino CN and Chura-Zea E (2024) Urban-rural gap in education performance in Peruvian public institutions during 2018: an analysis using the Oaxaca-Blinder decomposition. Front. Educ. 9:1394938. doi: 10.3389/feduc.2024.1394938
Edited by:
Juan Pedro Martínez-Ramón, University of Murcia, SpainReviewed by:
Eduardo Hernández-Padilla, Autonomous University of the State of Morelos, MexicoFrancisco Manuel Morales, University of Granada, Spain
Copyright © 2024 Rojas Apaza, Paredes, Arpi, Quispe Lino and Chura-Zea. This is an open-access article distributed under the terms of the Creative Commons Attribution License (CC BY). The use, distribution or reproduction in other forums is permitted, provided the original author(s) and the copyright owner(s) are credited and that the original publication in this journal is cited, in accordance with accepted academic practice. No use, distribution or reproduction is permitted which does not comply with these terms.
*Correspondence: Rene Paz Paredes, cnBwYXJlZGVzQHVuYXAuZWR1LnBl