- 1Research Institute of Child Development and Education, University of Amsterdam, Amsterdam, Netherlands
- 2Department of Psychology, Education and Child Studies, Erasmus University Rotterdam, Rotterdam, Netherlands
Introduction: Studies pinpoint the importance of exploring factors in the classroom environment that might foster students’ SRL-skills in various domains. This study explored specific teacher and classroom factors, including self-efficacy for SRL-skills (TSE) and teacher–student interactions in relation to students’ self-regulated learning skills in various domains (metacognitive, motivational, and behavioral). Additionally, the moderating role of teacher–student interactions in the relationship between TSE and SRL-skills was examined.
Methods: Third-to sixth grade students (N = 1,278, 46.9% boys) from 63 classrooms of Dutch elementary schools completed reports about their metacognitive, motivational, and behavioral SRL-skills. Teachers reported on their TSE for eight randomly selected students from their classes. In addition, the Classroom Assessment Scoring System was used to observe the quality of teacher–student interactions.
Results: Multilevel analyses generally revealed that individual students reported better metacognitive, motivational, and behavioral SRL-skills when their teachers felt efficacious in teaching SRL-skills. However, none of the associations at the class level were significant. Moreover, when high-quality teacher–student interactions were observed, students reported lower levels of metacognitive, motivational, and behavioral SRL-skills. No moderating effect of teacher–student interactions on the relation between TSE and SRL-skills was found.
Discussion: These findings show that specific measures of TSE at the appropriate level of analysis may help to better explain variation in TSE and students’ SRL-skills.
Introduction
Self-regulated learning skills (SRL-skills) refer to specific abilities, strategies, and behaviors that students employ while they systematically attempt to monitor, regulate, and control their own learning (Pintrich, 2004). Mastering these skills is believed to contribute to effective self-regulated learning, which is widely recognized as a crucial competence for becoming a lifelong learner (Pintrich, 2004; Schunk and Greene, 2018). Previous research has indicated that students who cultivate their self-regulation skills from an early age tend to attain higher levels of performance and exhibit higher levels of motivation towards their academic tasks (e.g., Chung, 2000; Dent and Koenka, 2016; Harding et al., 2019). Accordingly, it seems relevant to understand which factors might foster students’ SRL-skills already in elementary school. Teacher factors are the focus of the current study.
Theoretical model of self-regulated learning
Central to the present study is Pintrich’s (2004) model of self-regulation, which includes metacognitive, motivational, and behavioral components of self-regulation. Students’ metacognition is regarded as the core component of SRL (Veenman, 2017; Usher and Schunk, 2018). It encompasses a range of key skills and strategies for regulating and controlling learning behaviors, such as task-orientation, monitoring, and self-evaluation (Pintrich, 2004; Vandevelde et al., 2013). The regulation of motivation involves the motivational beliefs that students hold regarding a task, including their self-reinforcement, positive self-talk, and interest enhancement (Pintrich, 2004). Last, the regulation of behavior entails the regulation of effort, persistence, time management, and help seeking (Skinner et al., 2008; Usher and Schunk, 2018). The inclusion of the motivational and behavioral components make Pintrich’s model one of the most comprehensive models available and particularly relevant for the elementary school context (Panadero, 2017). These components are considered to be crucial for elementary students’ academic performance (Dignath and Büttner, 2008; Perry et al., 2019).
Teacher self-efficacy and students’ SRL-skills
Two possible factors may promote students’ SRL-skills, including teacher self-efficacy in fostering SRL-skills (Dignath, 2016; Karlen et al., 2023). Teachers’ self-efficacy in SRL-skills, in short TSE for SRL-skills, reflects teachers’ beliefs about their ability to affect students’ SRL-skills (De Smul et al., 2018). Based on social cognitive theory it is commonly believed that TSE for SRL may play a role in students’ SRL-skills by its influence on behaviors and competencies, such as goals and aspirations, outcome expectations, and metacognition (Bandura, 1997). Empirical studies indeed suggest a link between TSE and various SRL-skills, showing that feelings about their own abilities and their own level of self-regulation may be related to students’ self-regulated learning (see Moos and Ringdal, 2012, for an overview).
Despite considerable progress in this literature, current findings seem to call for some nuance. First, it should be noted that general TSE can fluctuate according to the circumstances in the classroom or the tasks at hand (Bandura, 1997; Zee and Koomen, 2016). Unfortunately, however, only a few studies have considered the task-and domain specific nature of TSE in studies on SRL-skills (e.g., Dignath, 2016, 2021; De Smul et al., 2018; Lawson et al., 2019; Karlen et al., 2020). This may be problematic, as the outcome domain of interest specifies the type of TSE that weighs most heavily on a given SRL-skill.
Second, from these studies, the study by Dignath (2021) is the only study that included direct measures of students’ SRL-skills. This study, which involved 191 third-to fourth-grade teachers and their students, assessed TSE for SRL-skills and the level of students’ self-regulatory skills. Results showed that students did not report an increase in their levels of SRL-skills when their teachers reported higher levels of TSE for SRL-skills. These results deviate from the commonly assumed belief that TSE leads to higher SRL-skills in students. It may be that the informant of student SRL-skills (teacher or student reported) might play an important role in whether associations between TSE and students’ SRL-skills appear to be positive. Hence we used student reports to measure SRL-skills in the current study.
Third, in Dignath’s (2021) study the relation of TSE and students’ SRL-skills has only been considered at the class level (Zee and Koomen, 2016). Little is known about the relation at the student level, that is between a teacher’s self-efficacy toward individual students (student-specific TSE) and student’s SRL-skills. Possibly, similar to studies in other teaching domains, the student-specific self-efficacy beliefs teachers hold are a better predictor of individual differences can be removed students’ SRL-skills than teachers’ general feelings of TSE (Zee et al., 2016a, 2018). Given these previous findings, we expect that a large portion of variance in the components of SRL is explained by student-specific TSE and that student-specific TSE has a stronger relationship with individual students outcomes (i.e., the student level), than general forms of TSE (i.e., teachers’ judgments at the class level, based on a subsample of students; Zee et al., 2016a, 2018). Our aim is therefore to examine associations between TSE for SRL-skills and students’ SRL-skills both within and between classes.
Quality of teacher–student interactions and student’s SRL-skills
A second factor that may promote students’ SRL-skills is the quality of teachers’ interactions with their students (Dignath, 2016; de Ruig et al., 2023; Hofkens et al., 2023). The quality of daily interactions between teachers and students is considered a driving force behind learning and development, including the development of SRL-skills (Bronfenbrenner and Morris, 1998; Hamre et al., 2013). These interactions are conceptualized around three broad domains: instructional support, classroom organization, and emotional support (Hamre et al., 2013; Fauth et al., 2021).
Instructional support consists of the degree to which teachers are able to promote inquiry skills, use a variety of learning formats, provide high-quality feedback, involve students in instructional dialogue, and help them understand content at a deeper level (Pianta et al., 2012). It is commonly assumed that if instructional support is well-tailored to students’ prior knowledge and includes real-life examples it will provide opportunities to show current skills, broaden knowledge, and develop new metacognitive SRL-skills (Hamre et al., 2013; Veenman, 2017). Moreover, direct forms of instruction by teachers are believed to help students obtain knowledge about strategy application and its benefits, which might also be related to their self-regulation (Veenman, 2017; Dignath and Veenman, 2021). Different reviews have demonstrated that instructional support can be an effective way through which teachers support students’ metacognitive SRL-skills. However, in these reviews its positive relationship with the motivational and behavioral SRL-skills appears to be less strong (Dignath and Büttner, 2008; Dignath and Veenman, 2021; de Ruig et al., 2023).
The domain of classroom organization represents teachers’ organization of and managerial tasks within the classroom, such as behavioral management, daily routines, and maximization of learning time (Pianta et al., 2012; Fauth et al., 2021; Hofkens et al., 2023). Organized classrooms and daily routines are believed to help students focus on learning tasks such that they can achieve their learning goals (Hamre et al., 2013). In addition, teachers in well-organized classrooms are expected to provide students with enough instructional time so that students encounter sufficient challenges and receive enough strategy instruction. Findings of a recent review by de Ruig et al. (2023) showed that well-organized classrooms also had students with higher metacognitive SRL-skills. The association appeared to be less strong compared to the overall relation for instructional support (de Ruig et al., 2023). Findings were mixed for the relationships between teachers’ classroom organization and motivational and behavioral SRL-skills.
Lastly, teachers who provide high levels of emotional support create a warm classroom climate, are sensitive, and have regard for students’ perspectives by being flexible and providing autonomy (Pianta et al., 2012). Emotionally supportive teachers create a secure and safe learning climate, in which students may feel self-reliant, competent, and willing to take risks to explore the classroom environment (Hamre et al., 2013). Moreover, emotionally supportive teachers are expected to help students feel engaged, autonomous, and motivated to work on a task. It has been suggested that these feelings support students’ SRL-skills (Rieser et al., 2013). Previous research has shown that when teachers were emotionally supportive they were also more likely to promote metacognitive SRL-skills in their students (de Ruig et al., 2023). However, emotionally supportive teachers did not always have students with higher levels of motivational or behavioral SRL-skills (Rimm-Kaufman et al., 2014; Havik and Westergård, 2020).
Two features of current evidence on the relation between teacher–student interactions and SRL-skills are of note. First, most studies in this field predominantly rely on teacher and student reports. de Ruig et al. (2023) found only four studies which included observations of the quality of interactions, all using the Classroom Assessment Scoring System (CLASS; Pianta et al., 2012). This is noteworthy as observations can provide valuable insights about the quality of teacher-student interactions, including real-time classroom dynamics, nonverbal behaviors, and other contextual factors (Pianta et al., 2012; Bell et al., 2019; Wang et al., 2020). It seems therefore likely that observations can provide additional information on how the quality of interactions within a classroom can promote students’ SRL-skills.
A second feature of current evidence is that the four studies that did use classroom observations of the quality of teacher–student interactions were all focused on motivational and behavioral SRL-skills (de Ruig et al., 2023). Metacognitive SRL-skills were not included in these studies. Moreover, findings about the relationship between the quality of interactions and students’ motivational and behavioral SRL-skills appeared to be mixed. Two cross-sectional studies revealed that when high levels of classroom organization were observed, lower and upper elementary school students were also more likely to show higher levels of motivational and behavioral SRL-skills (Cadima et al., 2015; van Dijk et al., 2019). Yet, two other studies did not find association of instructional support, classroom organization, and emotional support with students’ ability to regulate their motivation and behavior (Rimm-Kaufman et al., 2014; Schenke, 2018). Therefore, we go a step further than previous studies by examining whether observed interactions may be associated with all three SRL-skills, thus including metacognitive self-regulation skills.
The moderating role of teacher–student interactions
The role of interaction quality in promotion of SRL-skills seems rather complex, and may generate interaction effects in addition to the expected direct effects. These interaction have, to our knowledge, not been previously researched. This is notable, given the commonly held believe that the potential relationships between student-specific TSE and students’ SRL-skills at different levels of classroom ecology cannot be examined in isolation from the context in which this relationship occurs (Bandura, 1997; Eccles and Wigfield, 2002).
It seems likely that the quality of teacher–student interactions provides teachers with positive and negative information regarding their own ability to teach SRL-skills to students and about their own performance in providing sufficient support. Lower-quality classroom interactions may therefore negatively effect the relationship between TSE and students’ SRL-skills, whereas in classrooms with high-quality interactions a much stronger effect of TSE on SRL-skills may be expected. Hence, our third and final aim to explore the potential moderating effect of the quality of teacher–student interactions on the relationship between TSE and SRL-skills.
Present study
This study addresses three research questions that extend prior research on SRL-skills. The first question is how TSE for SRL-skills and SRL-skills are related at the student and the class level. Rather than taking a class-level only perspective, as an extension to previous research, we also examined these relationships at the student level to gain a deeper insight into how TSE might promote students’ SRL-skills at different levels of classroom ecology. The second question concerns how teacher–student interactions are associated to students’ SRL-skills. We used observations instead of teacher or student reports to obtain information about the quality of teacher–student interactions in class. The third question is whether teacher–student interactions moderate the relationship between TSE and SRL-skills.
Based on prior research, several hypotheses were tested. First, we hypothesized that associations between TSE for SRL-skills and SRL-skills may not be positive (Dignath, 2021). Second, we expected that student-specific TSE may have a positive relationship with individual students’ outcomes (e.g., Zee et al., 2016a). Third, we hypothesized that associations between the three domains of teacher–student interactions and metacognitive SRL-skills may be positive (e.g., Rieser et al., 2013). Given that relationships with motivational and behavioral SRL-skills were mixed in prior research (Rimm-Kaufman et al., 2014; Cadima et al., 2015; Schenke, 2018; van Dijk et al., 2019), we assumed weaker relationships with motivational and behavioral SRL-skills than with metacognitive SRL-skills. Finally, our fourth hypothesis was that a possible relationship between TSE for SRL-skills and SRL-skills was moderated by the quality of teacher–student interactions. We expected that high-quality interactions may positively moderate a potential relationship between TSE and SRL-skills, whereas lower-quality interactions may negatively effect such a association.
Method
Participants
The sample consisted of 1,278 third-to sixth grade students (46.9% boys) from 63 classrooms of 33 Dutch regular elementary schools in both urban and rural areas across the Netherlands. Of these students, 126 were in grade 3 (9.9%), 264 in grade 4 (20.7%), 401 in grade 5 (31.4%), and 452 in grade 6 (35.4%). Another 35 students (2.7%) did not report their grade. Students’ age ranged from 7 to 13 years (M = 10.00, SD = 1.19). Of the participating students, 75.7% identified themselves as ethnically Dutch. Teachers’ age ranged from 22 to 59 years old (M = 34.72, SD = 10.75). Teachers (N = 63) generally had 9.23 years of teaching experience (SD = 7.54) and class sizes ranged from 12 to 37 students per classroom (M = 24.88, SD = 3.18).
Teachers were asked to fill out a questionnaire on student-specific TSE for SRL-skills for eight students who were randomly selected from their classrooms. Completing questionnaires for eight students is considered to be a manageable workload for teachers and a good indication of the class mean for TSE (Zee et al., 2018, 2024; Zee and Rudasill, 2021). Due to illness or time constraints, not all teachers were able to complete the questionnaire for those eight students, resulting in completed questionnaires on teachers’ TSE for SRL-skills for an average seven students per class. This resulted in a subset of 449 students for which information was available on student-specific TSE for SRL-skills.
Procedure
We reached out to school principals and teachers through various channels, including telephone, email, and LinkedIn. Invited teachers received an information brochure and were asked to sign an informed consent form. Of the teachers that were contacted, 13.2% agreed to participate. Moreover, parents were asked to provide consent for their child to participate. The average teacher consent rate per school was 73.3% and the parental consent rate for student participation per class was 84.9%.
Data was gathered in three ways, through: (1) classroom videos, (2) student-report questionnaires, and (3) teacher-report questionnaires. Trained research assistants or the first author made a 45-min video within each participating classroom to capture teacher and student behavior as well as classroom-level interactions (see Instruments). Videos were recorded at the beginning of a regular school day. After the video was recorded, on the same day, teachers and students were asked to complete a questionnaire. Completing the questionnaires took around 30 min. Data was collected during the fall of schoolyear 2019–2020. Teachers and students were allowed to stop at any given moment during the project. The study was approved by the Ethics Review Board of the Faculty of Social and Behavioral Sciences of the University of Amsterdam (project 2019-CDE-10504).
Instruments
Teacher self-efficacy for SRL-skills
All participating teachers were asked to complete the Teacher Self-Efficacy Scale to foster SRL (De Smul et al., 2018) for eight randomly selected students from their classrooms. This scale includes four subscales: (1) Teacher Self-Efficacy for Direct Instruction (5 items), (2) Teacher Self-Efficacy for Providing Choices (5 items), (3) Teacher Self-Efficacy for Providing Challenges and Complex Tasks (5 items), and (4) Teacher Self-Efficacy for Building in Evaluation (3 items). Examples of questions are: “How well can you teach your students which self-regulated learning strategies exist?” and “How well can you let your students reflect on their own learning process?”. Questions for each scale were changed to tap student-specific teacher self-efficacy for SRL by turning the general word “students” into “this student”. For example, the question “How well can you teach your students which self-regulated learning strategies exist?” became “How well can you teach this student which self-regulated learning strategies exist?”. Items were rated by teachers on a 5-point Likert-type scale, ranging from 1 (definitively not true) to 5 (definitively true). A prior study has provided support for the psychometric quality of the Teacher Self-Efficacy Scale to foster SRL, with factor loadings >0.54 and Bentler’s ρ ranging between 0.80 to 0.91 (de Smul et al., 2018). The reliability coefficients for student-specific TSE for Direct Instruction (α = 0.95), Providing Choices (α = 0.90), Providing Challenges and Complex Tasks (α =0.86), and Building in Evaluation (α = 0.89) in the current sample were satisfactory. In line with a previous study that combined the four general scales into a single TSE factor (De Smul et al., 2019), Cronbach’s alpha for the combined Teacher Self-Efficacy for SRL factor was excellent (α = 0.96). The combined scale was used in the current study.
Teacher–student interactions quality
The quality of teacher–student interactions in each of the 63 classrooms was assessed with the Upper Elementary Classroom Assessment Scoring System (CLASS; Pianta et al., 2012). The CLASS is considered one of the most extensively researched observation instruments for assessing the quality of teacher–student interactions (Hafen et al., 2015; Bell et al., 2019). This instrument consists of 11 specific dimensions for the broader domains of Instructional Support, Classroom Organization, and Emotional Support (Pianta et al., 2012). Instructional Support was measured using the dimensions Instructional Learning Formats, Content Understanding, Analysis and Inquiry, Quality of Feedback, and Instructional Dialogue. Classroom Organization was captured by the Behavior Management, Productivity, and Negative Climate subscales. Last, the Emotional Support domain consisted of the dimensions Positive Climate, Teacher Sensitivity, and Regard for Student Perspectives. Prior research has suggested that these domains can be reliably distinguished from one another (e.g., Hafen et al., 2015; Schenke, 2018). Cronbach’s alphas for Instructional Support (α = 0.77), Classroom Organization (α = 0.88), and Emotional Support (α = 0.86) were adequate in the present study.
Each dimension was rated by the first author on a 7-point Likert scale, ranging from low (1–2), middle (3–5), to high (6–7). The first author was a certified CLASS-observer, who attended a three-day coding training and passed yearly reliability tests during the project. Each coding cycle began by carefully noting down observations related to teacher and student behavior, as well as interaction patterns within the classroom. Afterwards, scores were assigned to each dimension using the descriptions provided in the CLASS manual (Pianta et al., 2012).
In total, seven videos (12%) were double coded by a certified CLASS-observer who had no other involvement in the project. Intra-class correlations (ICC) were calculated per dimension to obtain inter-rater reliability (see Table 1). Overall inter-rater reliability ranged between fair (between 0.40 and 0.59), good (between 0.60 and 0.74), and excellent (between 0.75–1.00), except for the Instructional Learning Formats dimension, which was below the critical value of 0.40 (Cicchetti, 1994). This dimension had no negative effect on the internal reliability of the Instructional Support scale, prompting our decision to include it in the score of this scale.
Students’ metacognitive and motivational SRL-skills
Students reported on their Metacognitive and Motivational SRL-skills using four subscales from a slightly adapted version of the Children’s Perceived Use of Self-Regulated Learning Inventory (CP-SRLI; Vandevelde et al., 2013, see Authors et al., for details about the adaptation process): task orientation (7 items), monitoring (8 items), self-evaluation (7 items), and motivational strategies (4 items). The Task Orientation items included students’ approach to learning tasks, including their task demands and representations of learning goals and task requirements (Vandevelde et al., 2013). An example of an item is: “Before I start my schoolwork, I ask myself: will I succeed?”. The Monitoring subscale includes items about students’ monitoring of cognition, behavior, motivation, and the context while conducting learning tasks. An example of an item is: “During my schoolwork, I ask myself: do I still understand everything?”. The Self-Evaluation items consisted of students’ Product and Process Evaluation. Students’ Product Evaluation is the evaluation of learning outcomes, including checks for correctness and completeness. Questions on how students reflect on their approaches, strategies, and their effectiveness were captured by items on students’ Process Evaluation (Vandevelde et al., 2013). Examples are: “After finishing my schoolwork, I check if I have done everything that was asked for” (product evaluation) and “After finishing my schoolwork, I ask myself: How did I feel about it? (fun, difficult, boring, interesting, …)?” (process evaluation). The Motivational Strategies scale consists of four items concerning students’ self-reinforcement, positive self-talk, and interest enhancement. An example of an item is: “During my schoolwork, I motivate myself to keep working”.
Factorial validity, reliability, and measurement invariance across gender have proven to be good in previous research (Vandevelde et al., 2013, 2017; Heirweg et al., 2019). Items from the CP-SRLI for both Metacognitive and Motivational SRL-skills were scored by students on a 5-point Likert-type scale, ranging from 1 (definitively not true) to 5 (definitively true). Average scores were calculated for both scales. Cronbach’s alphas in the present study indicated satisfactory internal consistencies for Metacognitive SRL-skills (α = 0.88) and sufficient for Motivational self-regulation skills (α = 0.62).
Students’ behavioral SRL-skills
Items regarding students’ behavioral SRL-skills were taken from the short Dutch version of the Engagement Versus Disaffection with Learning questionnaire (Skinner et al., 2008; Zee and Koomen, 2020). Behavioral SRL-skills were measured with six self-report items, including items about students’ effort, attention, and persistence before and during a learning task. Examples of items are: “I try hard to do well in school” and “When I am in class, I think about other things”. Items from the Behavioral SRL-skills scale were scored by students on a 5-point Likert-type scale, ranging from 1 (definitively not true) to 5 (definitively true). Average scores were calculated for this scale, based upon the complete sample of students (N = 1,278). The reliability and construct validity for the short Dutch version have been found to be satisfactory (Zee and Koomen, 2020). Moreover, and in line with previous research (e.g., Skinner et al., 2008; Engels et al., 2021), Cronbach’s alpha for the subscale was adequate (α = 0.77).
Data analysis
An intercepts-and-slopes-as-outcomes multilevel model was fitted to test our research questions and corresponding hypotheses (Kreft and de Leeuw, 1998). This model takes the nested data structure of students within classrooms into account (Geiser, 2013). Accounting for the nested data allows for estimation of unbiased standard errors for the regression coefficients. Moreover, variables can be added to this model at both the student (student-specific TSE and SRL-skills) and the class level (aggregated TSE, teacher–student interactions, and class-average SRL-skills). Another advantage of the intercepts-and-slopes-as-outcomes model is that both the intercepts and slopes of student-level regressions can vary across classroom-level variables (Kreft and de Leeuw, 1998; Geiser, 2013). Any differences between classes in intercepts and slopes can, in turn, be explained by variables at the class level. Hence, this model enabled us to answer our first and second research question, that is, whether TSE for SRL-skills and the three interaction domains are associated with students’ SRL-skills at the student and at the class-level.
The third research question, whether teacher–student interactions moderated the relationship between student-specific TSE for SRL-skills and SRL-skills, was examined by adding a cross-level interaction to the intercepts-and-slopes-as-outcomes model. This interaction specifies that the relationship between the student level predictor TSE for SRL-skills and the outcome variables, that is students’ SRL-skills, is dependent on the level of the class-level predictors, that is the quality level of teacher–student interactions.
Maximum likelihood estimation with robust standard errors was employed to deal with potentially non-normal data (Kline, 2016). Missing data was handled using full information maximum likelihood (FIML; Muthén and Muthén, 2017). All student and class-level predictors were grand-mean centered to ease interpretation (Kreft and de Leeuw, 1998).
Modeling procedure
We used a modeling procedure that consisted of four consecutive steps (Hox et al., 2018). First, an intercept-only model was tested with student-specific TSE, Metacognitive, Motivational, and Behavioral SRL-skills as outcome variables and without any of the predictors. This model produced intra-class correlations and served as a baseline model for model comparison (Hox et al., 2018).
Second, the intercepts-and-slopes-as-outcomes model was fitted to the data to test our first and second hypothesis. Within this model, individual SRL-skills scores were regressed on student-specific TSE and the intercepts were allowed to vary across classes. In addition, aggregated TSE was added as a classroom-level predictor of class-average SRL-skills, together with the three domains of teacher–student interactions. This model is based on separate student and class-level correlations and provides unstandardized and standardized regression coefficients at both levels of analysis. The fit of this model was compared to the intercept-only model.
Third, random slopes were separately added to the intercepts-and-slopes-as-outcomes model for each combination of the student level predictor TSE with Metacognitive, Motivational, and Behavioral SRL-skills. Significant random slope coefficients indicate that the relationships between TSE and SRL-skills differed per class (Finch and Bolin, 2017). Only statistically significant random slopes were added to the final model (Hox et al., 2018).
Fourth, cross-level interactions were added to test our third hypothesis. Adding a cross-level interaction allowed the student level predictor TSE to interact with the class-level predictors Instructional Support, Classroom Organization, and Emotional Support (Hox et al., 2018). A significant cross-level interaction indicates a moderating effect of the quality of teacher–student interactions on the relationship between student-specific TSE and SRL-skills. Again, model fit was compared to the previous model and the baseline model.
The Satorra-Bentler adjusted chi-square difference test was used to determine whether alternative models fitted significantly better than the nested models (Finch and Bolin, 2017). The nested model refers to the model that remains when parameters are removed from a more general alternative model (Hox et al., 2018). For this test, log likelihood values were used to calculate the scaling correction factor and the adjusted chi-square difference test. This approach was adopted as Mplus does not produce a chi-square deviance test or deviance values when maximum likelihood estimation with robust standard errors are used. A significant test indicates that the model has a better fit than the previous model.
Results
Descriptive statistics and data screening
Data was screened and descriptive statistics were evaluated before the multilevel models were fitted to the data. Most of the scales were approximately normally distributed following a threshold of ±1.00 for skewness and kurtosis (Kline, 2016). However, for Classroom Organization, skewness (−2.06) and kurtosis (5.87) were considerably higher than ±1.00. To account for this non-normality, we used robust maximum likelihood estimation (Kline, 2016). In addition, no multivariate outliers were found using Cook’s Distance and there was no sign of multicollinearity using variance-infliction factors with a threshold of five (Tabachnick and Fidell, 2014). Probability plots were inspected and showed no violation of the assumption of multivariate normality.
ICC1 and ICC2 values are displayed in Table 2. ICC1 indicates the percentage of the total variance in a variable that can be ascribed to the class-level. These percentages were 7.5% for Metacognitive, 2.2% for Motivational, and 8.8% for Behavioral SRL-skills occurred between classes. Even though these intraclass correlations are relatively small (< 10%), it is still advised to use a multilevel modeling to account for the nested structure of the data (Kreft and de Leeuw, 1998).
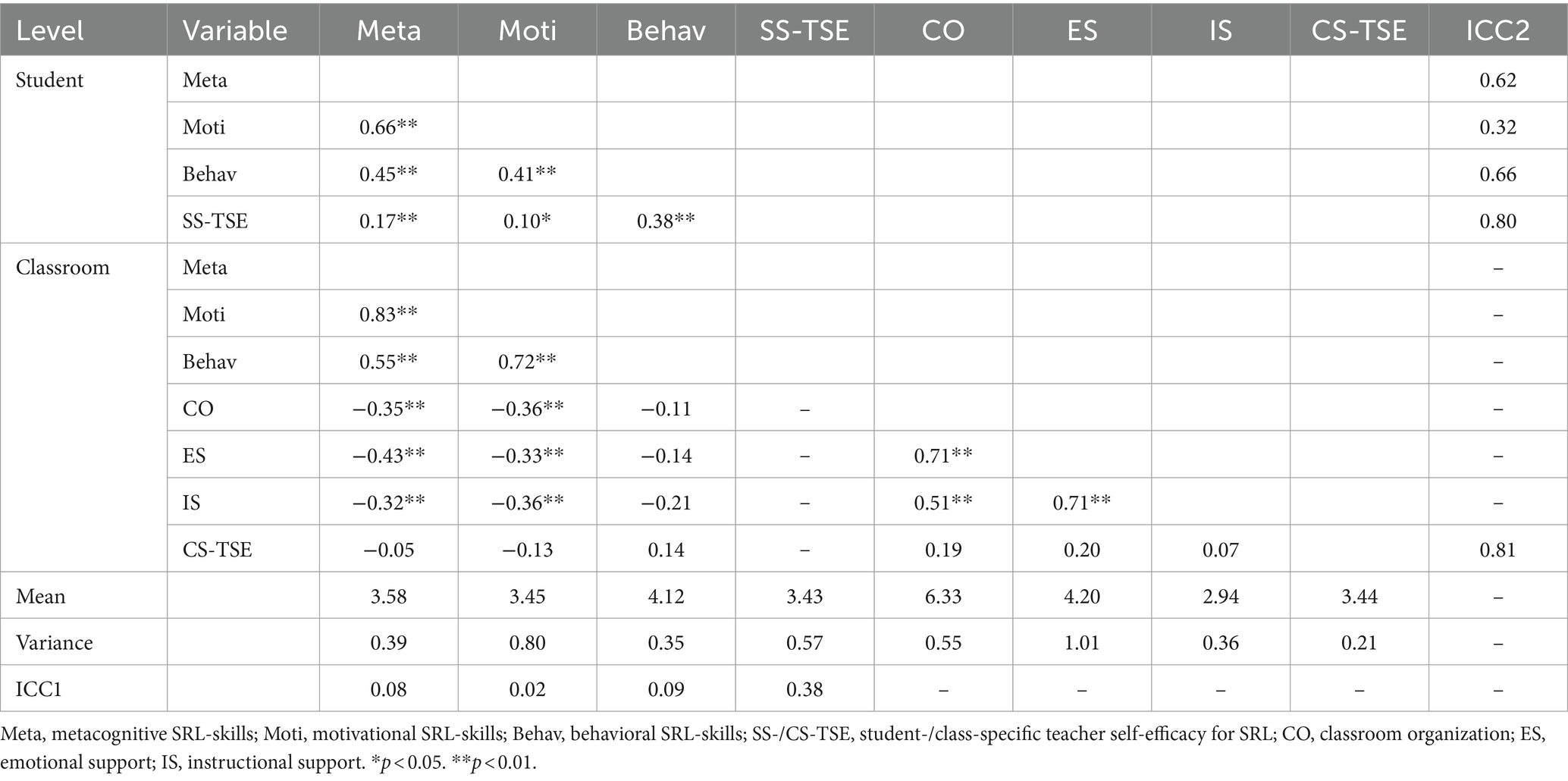
Table 2. Descriptive statistics, intra-class correlations, and correlations among the variables at the student and class level.
In addition, we evaluated the reliability of the aggregated variables (ICC2; Marsh et al., 2012), including Metacognitive, Motivational, and Behavioral SRL-skills, and Student-Specific and Class-specific TSE. Metacognitive, Motivational, and Behavioral SRL-skills were based on the full sample of 1,278 students, whereas Class-specific TSE for SRL was based on eight randomly selected students per class. The ICC2 values were good for the Metacognitive (0.621) and Behavioral SRL-skills (0.664), and even excellent for student-specific (0.803) and Class-specific TSE for SRL (0.807; Cicchetti, 1994). The ICC2 value of 0.316 for the Motivational SRL-skills was not satisfactory. This value indicates that the class-average mean may be unreliable (Marsh et al., 2012). We therefore tested models with and without the Motivation SRL-skills, but did not find significant differences in coefficients at the class level or in model fit. Since the Motivational SRL-skills are considered to be important to the SRL process and also a reliable outcome variable at the within level, we retained this variable in the models that were tested.
Regarding the domains of teacher–student interactions, Instructional Support had the lowest overall mean, while Classroom Organization demonstrated the highest mean among the three interaction domains. This is in accordance with previous research (e.g., Schenke, 2018), and shows that the majority of classrooms are well-organized, while the average quality of instruction falls only in the lower midrange of the CLASS. The variance of Emotional Support shows that the quality varies more among teachers than in the other domains.
The student and class-level correlations are in Table 2. At the class level, the correlations between various domains of classroom interaction quality were high, particularly between Classroom Organization and Emotional Support (r = 0.71, p < 0.001), and Emotional Support and Instructional Support (r = 0.71, p < 0.001). These correlations imply a significant overlap among these interaction domains. The various components of SRL were also correlated at the class level, but, as compared to the student level, several correlations were much stronger, such as the correlations between the Motivational and Metacognitive SRL-skills (r = 0.83, p < 0.001) and Motivational and Behavioral SRL-skills (r = 0.72, p < 0.001). It appears that these SRL-skills are more strongly interconnected between classrooms than within classrooms.
Contrary to expectations, there were negative correlations between the domains of classroom interactions and SRL-skills, including significant negative correlations between the three domains and Metacognitive and Motivational SRL-skills. These negative correlations suggest that as the quality of teacher–student interactions increases, the level of SRL-skills in students decreases.
Multilevel regression models
Our first model was the intercept-only model that served as a baseline model for model comparison. In step two, a random intercept model with the student-level predictor Student-Specific TSE and the classroom-level predictors Instructional Support, Classroom Organization, and Emotional Support and TSE was fitted to the data. This CLASS model with the three measures of the CLASS was a significant improvement over the intercept-only model, according to the Satorra-Bentler adjusted chi-square difference test (TRd = 2326.75 (27), p < 0.01). The standardized coefficients of this model are presented in Table 3. These coefficients demonstrate that student-specific TSE is significantly related to students’ Metacognitive (β = 0.17, p < 0.05), Motivational (β = 0.10, p < 0.05), and Behavioral SRL-skills (β = 0.38, p < 0.05).
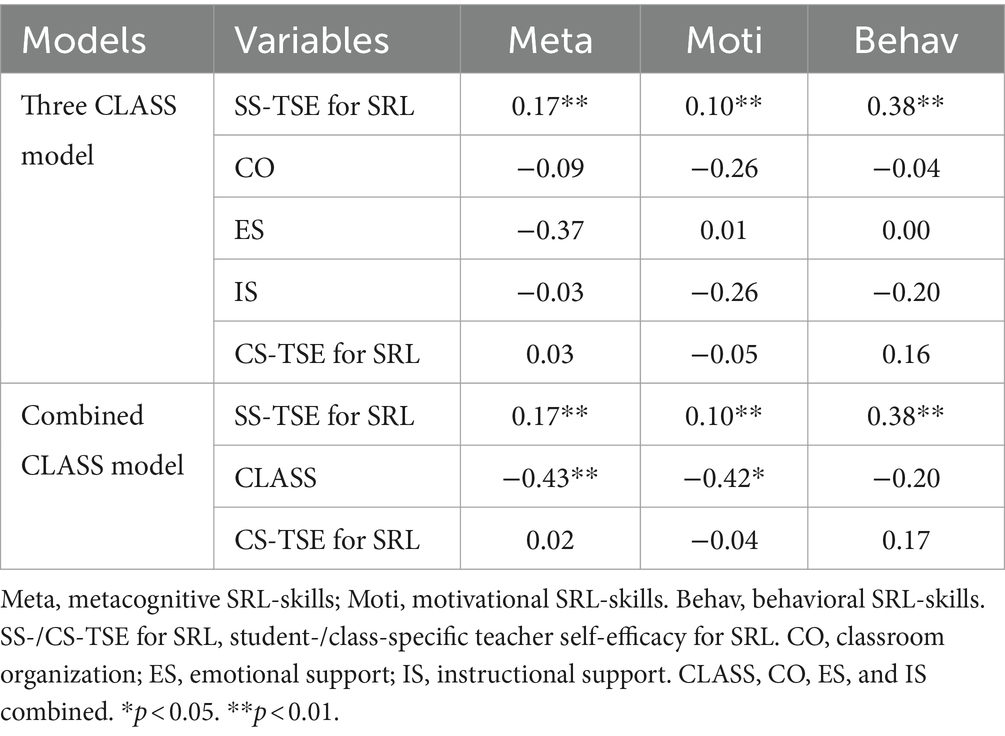
Table 3. Standardized regression coefficients for regression of SRL-skills on TSE and domains of the CLASS.
Standardized coefficients for the relationship between the three domains of class-level interactions and SRL-skills were generally non-significant and predominantly close to zero (see Table 3). The three interaction domains did not have an independent association with each of the SRL-skills. Their effect was likely determined by what they collectively contributed to the dimension of SRL. Therefore, the three domains of teacher–student interactions were combined into a single measure. The combined measure had excellent internal consistency (Cronbach’s α = 0.91). The combined CLASS model appeared to have better model fit than the intercept-only model (TRd = 901.16 (17), p < 0.01). The results of the combined CLASS model showed significant negative standardized coefficients between teacher–student interactions and students’ Metacognitive and Motivational SRL-skills.
In step three, we added random slopes to the random intercept model for each combination of the student level predictor TSE with Metacognitive, Motivational, and Behavioral SRL-skills. Random slopes were non-significant for the association between TSE and students’ SRL-skills when they were separately added to the combined CLASS model. This implies that the variation among classes for students’ SRL-skills is not significant in our data. Hence, there is no indication for a moderating effect of the quality of teacher–student interactions on the relationship between TSE and SRL-skills, given that significant slope variation is a precondition for such an effect (Hox et al., 2018).
An extra step was added to our modeling procedure as we observed positive associations between TSE and SRL-skills at the student level, whereas at the class level the coefficients were near zero and non-significant. Contextual effects for aggregated TSE and the three SRL-skills in the combined CLASS model were therefore calculated, based on three newly created parameters. These parameters match with the differences between the corresponding student and class-level variables for the relationships between TSE at the class level and the three SRL-skills. The contextual effects then reflect the effect of the aggregated class-level variable of TSE, after controlling for the effects of student-specific TSE at the student level (Marsh et al., 2012). Results showed significant negative context effects for TSE at the class level and Metacognitive (B = −0.25, p < 0.001), Motivational (B = −0.21, p < 0.01), and Behavioral SRL-skills (B = −0.37, p < 0.001). These findings indicate that the relationship between student-specific TSE and the three SRL-skills is negatively moderated by TSE at the class level.
Discussion
The current study had three main research questions. Our first question was about the association of TSE for SRL-skills with students’ SRL-skills at both the student-specific and class-level. Our second question was whether observations of the quality of teacher–student interactions were related to students’ metacognitive, motivational, and behavioral SRL-skill. Our third question was whether the quality of teacher–student interactions moderated the relationship between TSE for SRL-skills and students’ SRL-skills.
Results generally showed that teachers who reported higher levels of student-specific TSE for SRL-skills were also more likely to have students in their class with higher levels of SRL-skills, while at the class level such a positive relationship was not found. Moreover, our study revealed that when high-quality teacher–student interactions were observed, students reported lower levels of metacognitive, motivational, and behavioral SRL-skills. In addition, findings indicated no significant moderating effect of teacher–student interactions on the relationship between TSE and SRL-skills.
Relationships between teacher self-efficacy and students’ SRL-skills
Relationships between TSE and students-SRL skills were examined at both the student and the class level. At the student level, teachers who reported higher levels of student-specific TSE for SRL had students who reported higher levels of metacognitive, motivational, and behavioral SRL-skills. These findings are in line with our hypothesis and with previous research in which the effects of student-specific TSE on student outcomes were examined (Zee et al., 2016b, 2018). Such a positive association between TSE and various SRL-skills is not surprising, as teachers may be better able to make judgments about their capacity to support a particular student when they consider the concrete needs, behaviors, and actions of individual students rather than an entire group (Zee et al., 2016b). Moreover, it seems that a domain and student-specific form of TSE is well-tailored to the outcome of interest, increasing the predictive merit of TSE in relation to self-regulation skills (Bandura, 1997). Our study is, to our knowledge, the first to demonstrate that judgments of teachers about whether they are able to foster SRL-skills for specific students are associated with higher levels of SRL-skills. It appears therefore important for teachers to consider how they may enhance their sense of self-efficacy for SRL-skills for individual students, as it seems that individual students’ SRL-skills benefit the most from this approach.
However, at the class level, the average level of TSE for SRL reported by teachers, based on their student-specific TSE, was not related to the average level of SRL of a class as reported by the students. This finding is not in line with the commonly recognized belief that higher levels of TSE lead to higher levels of SRL-skills in students (e.g., De Smul et al., 2018). A main explanation for why our results deviate from most previous findings, is that the level of SRL-skills is often not directly assessed in other studies (e.g., De Smul et al., 2018; Karlen et al., 2020). Rather, in most studies, teachers were asked to report on both their TSE for fostering SRL and SRL-skills in their students. In that case, teachers are the same informant for both the predictor and outcome variables. The use of the same informant for TSE and students’ SRL-skills seems to overestimate their relation (Vandevelde et al., 2013; De Smul et al., 2018). Our findings resonate with the results of a recent study in which the associations between teachers’ self-reported judgments and students’ perceived levels of SRL-skills were nonsignificant as well (Dignath, 2021). In this study and the current study, TSE was based on teacher reports and the level of SRL-skills was directly assessed through student reports.
Another reason for the absence of a relation between student-specific TSE and students’ SRL-skills at the class level, is that in earlier studies teachers rated their TSE based on general judgments about their self-efficacy towards an entire class. In the current study an aggregated measure of student-specific TSE was used. This aggregated measure was based on teachers’ judgments of a random sample of individual students within each class. Previous research has shown that general and aggregated TSE are only moderately correlated, which may result in different relationships with student outcomes (Zee et al., 2016b, 2018). An explanation for these differences is the distinction between aggregated TSE as a classroom context variable and general TSE as a classroom climate variable, which are both based on different referents (Marsh et al., 2012). Aggregated TSE is a representation of teachers’ feelings of self-efficacy towards specific students in a particular classroom, and therefore based on individual students who each receive an individual score. General TSE, on the other hand, uses the entire class as referent (Zee et al., 2018). Hence why students in the same class all receive the same score. The differences in referents may have a fundamental influence on the interpretation of both construct, which is why they may have different relationships with the outcome variables (Marsh et al., 2012; Morin et al., 2014).
The positive associations between TSE and SRL-skills at the student level and the lack of relationships at the class level, suggests a contextual effect of the class (Marsh et al., 2012). The contextual effect is the effect of the class-level variable of TSE, after controlling for the effects of student-specific TSE. Indeed, in our study the contextual effect shows that teachers who feel more confident about fostering SRL-skills in their classes are likely to teach students in those classes who perceive their own self-regulated learning skills as lower. In contrast, in classes where TSE for SRL-skills is reported to be lower, students perceive their SRL-skills on average to be higher. We had not anticipated this effect and it is not straightforward to provide an explanation. One possibility is that teachers use the teacher report scale somewhat differently, resulting in, for instance, one teacher consistently assigning higher ratings than another teacher about their TSE for SRL-skills. Consequently, the relationship between TSE and SRL-skills may be predominantly a within-class effect. Another possible explanation is that teachers’ efficacy beliefs directly influence teachers’ behavior and may therefore serve as a good proxy for how teachers support students within their classrooms (Bandura, 1997; Zee and Koomen, 2016). Thus, if teachers feel highly self-efficacious in teaching SRL, they may invest considerable time in this area and potentially scaffold many SRL-skills for their students. This may have two consequences. First, due to teacher’s emphasis on SRL-skills, students may become more aware of the strategies they do or do not employ, leading to rate themselves lower on these skills. Second, due to the focus on SRL-skills, the students may feel that they need to apply fewer skills themselves to regulate their own learning as the teacher is already doing it for them.
Quality of teacher–student interactions and student’s SRL-skills
A main finding of this study is that in classes with higher quality teacher–student interactions students reported lower mean class-levels of their SRL-skills. This is particularly evident for students’ metacognitive and motivational SRL-skills. Based on previous research that used student and teacher reports, we had expected the opposite outcome (e.g., Rieser et al., 2013). That is, higher teacher-student interaction quality would, at least for the metacognitive SRL-skills, be associated with a higher mean class-level of SRL-skills. Our results deviate from these expectations.
Until now, there were four studies that reported no or weak correlations between domains of the CLASS and students’ SRL-skills (Rimm-Kaufman et al., 2014; Cadima et al., 2015; Schenke, 2018; van Dijk et al., 2019). There are two explanations for this findings. First, absent or weak correlations may be expected because the total score of the CLASS could be disproportionally influenced by a small number of students. Teacher–student interactions consist of both teacher and student behavior that are observed simultaneously. For example, observers score teacher behavior on the Instructional Learning Formats scale, such as promoting involvement and teacher interests, and at the same time also active participation and sustained attention of students (Pianta et al., 2012). Another example is when two students are arguing during the observation, while there is little else happening in the classroom. Their actions probably taint the scores, while this situation may not necessarily impact all students. Hence, it seems that the CLASS, although considered to be a classroom climate measure, also encompasses aspects related to specific student behaviors and actions in class.
Second, another explanation for this unexpected finding is that classroom interaction quality and students’ SRL-skills are measured at different levels of classroom ecology. Whereas interaction quality refers to the overall, more objective atmosphere of the classroom (i.e., contextual variable), the average SRL-skills of a class are based on the subjective ratings of all students in that particular class (i.e., climate variable). Each score is assigned equal weight when aggerated to the class level. In that case, the aggregated outcome measure is based on a different level of analysis than the overall classroom measure. This may potentially lead to correlations and coefficients that are lower than expected (Marsh et al., 2012; Morin et al., 2014). A suggestion for future research may therefore be to design and use an observation instrument that is aimed at the quality of dyadic, one-on-one, interactions instead of the classroom as a whole. The predictor and outcome variables are than both at the student level and reflect the same level of analysis and theory, also when aggregated to the class level (Marsh et al., 2012).
However, in the current study negative relationships between the quality of teacher–student interactions and students’ SRL-skills were found. This may not be fully explained by differences in the levels of analysis. There seem to be two main reasons for the negative relationships. First, it is possible that higher quality interactions made students believe that they have less SRL-skills, because they are provided with relatively better support from their teachers. Second, even though the quality of interactions are high and teachers provide numerous opportunities for learning, these high-quality interactions may also take away some of the necessity for students to regulate their own learning in their experience. For example, when teachers provide high-quality instructional support they demonstrate, guide, and model extensively, which might reduce the necessity for students to actively use their SRL-skills to some extent during instruction. Consequently, when asked to report about their SRL-skills, students may indicate a lower use of these skills. Further research investigating relationships between the domains of the CLASS and SRL-skills seems therefore needed to determine if the negative associations from the current study persist across different classroom and school contexts.
The third and final aim of the study was to examine the moderating role of instructional support, classroom organization, and emotional support on the relationship between student-specific TSE with the three SRL-skills. Notably, none of the random slopes for each combination of TSE with metacognitive, motivational, or behavioral SRL-skills were statically significant. As a result, we were unable to identify any moderation effect for one of the domains of teacher–student interactions. These findings do not align with our hypothesis that a moderation effect would be present. Rather, our findings demonstrate that relationships between TSE and SRL-skills are relatively less sensitive to the influence of the classroom ecology.
Limitations
There are three limitations in the current study that need to be addressed. First, our study is cross-sectional and correlational in nature, preventing us from drawing causal conclusions. Although our findings offer insight into the complex relationships between TSE and SRL-skills at both the student and class level, it is possible that these associations are reciprocal (Bandura, 1997). This would be in line with social-cognitive theory suggesting that teachers’ personal factors, behaviors, and the classroom context interact with each other bidirectionally. Future research could employ longitudinal, cross-lagged multilevel designs to disentangle causal effects and examine whether these relationships are indeed reciprocal.
Second, we did not add a general measure of TSE for SRL to our study in addition to the aggregated TSE variable at the class level. Previous research has demonstrated that general TSE and aggregated TSE are only moderately correlated and may have different relationships with student outcomes (Zee et al., 2016b, 2018). Inclusion of a general measure of TSE would have made our results more comparable with those from previous studies. However, as seen in previous research, relatively weaker relationships are found between general TSE and student outcomes as compared to a specific measure of TSE (Zee and Koomen, 2016; Zee et al., 2018). This is the primary reason why we relied on specific-student measures for TSE.
Third, despite intensive training and a yearly recertification, inter-rater reliability was poor or just fair in two areas of the instructional support domain of the CLASS. These findings are in line with previous studies, in which variability among multiple raters was found (Hamre et al., 2013; Mashburn et al., 2013). However, Cronbach’s alpha for the total score of the instructional support domain appeared to be good in the current study, which is why we still included it and believe it is a reliable scale for the instructional support domain. Yet, it seems necessary for future research to explore methods to enhance inter-rater reliability of the CLASS, by for example increasing the number of raters per classroom or by improving existing observational measures.
Conclusion
The current study’s findings highlight that it is crucial to recognize the multilevel nature of TSE for SRL-skills. Similar to previous studies on TSE (e.g., Zee et al., 2018), relationships with SRL-skills at the student level were positive in the present study, while there were no associations with self-regulation at the class level. These findings demonstrate that the associations between TSE for SRL and students’ SRL-skills in elementary schools are complex and need further attention in future studies. Moreover, it seems that student-specific TSE for SRL is important for promoting SRL, and since TSE serves as a good proxy for teachers’ behaviors and actions in the classroom, it would likely help teachers to provide them with more concrete tools to teach SRL-skills. This does not only apply to metacognitive SRL-skills, but also to motivational and behavioral self-regulation skills. Additionally, it seems that teaching general strategy use may not be as beneficial to SRL-skills at the class level as compared to a student-specific approach. This approach, in which the needs of each individual student is carefully considered, may offer a more effective method for teachers to help there students to learn and apply SRL-skills.
Our study also revealed, contrary to expectations, that high-quality teacher–student interactions were negatively associated to students’ metacognitive, motivational, and behavioral SRL-skills. This unexpected finding may be attributed to our study being among the first to have used classroom observations to measure interaction quality in relation to SRL-skills. Our findings show that the classroom interactions play a highly complex role in promoting SRL-skills. The contextual effect suggests the importance of finding a balance between providing too much support through high-quality interactions and allowing for complete self-regulation. It may also be possible that this balance is also highly dependent on age. Moreover, these findings highlight the importance for future researchers to use other methodologies and instruments than the commonly used self-reports, to better understand the complex dynamics between teacher–student interactions and students’ SRL-skills.
Data availability statement
The datasets presented in this article are not readily available because of restrictions imposed by the ethical committee. Requests to access the datasets should be directed to the corresponding author.
Author contributions
NR: Writing – original draft, Writing – review & editing. MZ: Writing – original draft, Writing – review & editing. PJ: Writing – original draft, Writing – review & editing.
Funding
The author(s) declare financial support was received for the research, authorship, and/or publication of this article. This work was supported by the Dutch Research Council (NWO) (023.011.049).
Conflict of interest
The authors declare that the research was conducted in the absence of any commercial or financial relationships that could be construed as a potential conflict of interest.
The author(s) declared that they were an editorial board member of Frontiers, at the time of submission. This had no impact on the peer review process and the final decision.
Publisher’s note
All claims expressed in this article are solely those of the authors and do not necessarily represent those of their affiliated organizations, or those of the publisher, the editors and the reviewers. Any product that may be evaluated in this article, or claim that may be made by its manufacturer, is not guaranteed or endorsed by the publisher.
References
Bell, C. A., Dobbelaer, M. J., Klette, K., and Visscher, A. (2019). Qualities of classroom observation systems. Sch. Eff. Sch. Improv. 30, 3–29. doi: 10.1080/09243453.2018.1539014
Bronfenbrenner, U., and Morris, P. A. (1998). “The ecology of developmental processes” in Handbook of child psychology. ed. L. RM , vol. (New York, NY: Wiley) 1. 5th ed, 106–112.
Cadima, J., Doumen, S., Verschueren, K., and Buyse, E. (2015). Child engagement in the transition to school: contributions of self-regulation, teacher-child relationships and classroom climate. Early Child. Res. Q. 32, 1–12. doi: 10.1016/j.ecresq.2015.01.008
Chung, M.-K. (2000). The development of self-regulated learning. Asia Pac. Educ. Rev. 1, 55–66. doi: 10.1007/bf03026146
Cicchetti, D. V. (1994). Guidelines, criteria, and rules of thumb for evaluating normed and standardized assessment instruments in psychology. In Psychological assessment. 6, 284–290. doi: 10.1037/1040-3590.6.4.284
de Ruig, N. J., de Jong, P. F., and Zee, M. (2023). Stimulating elementary school students’ self-regulated learning through high-quality interactions and relationships: a narrative review. Educ. Psychol. Rev. 35. doi: 10.1007/s10648-023-09795-5
De Smul, M., Heirweg, S., Devos, G., and Van Keer, H. (2019). School and teacher determinants underlying teachers’ implementation of self-regulated learning in primary education. Res. Pap. Educ. 34, 701–724. doi: 10.1080/02671522.2018.1536888
De Smul, M., Heirweg, S., Van Keer, H., Devos, G., and Vandevelde, S. (2018). How competent do teachers feel instructing self-regulated learning strategies? Development and validation of the teacher self-efficacy scale to implement self-regulated learning. Teach. Teach. Educ. 71, 214–225. doi: 10.1016/j.tate.2018.01.001
Dent, A. L., and Koenka, A. C. (2016). The relation between self-regulated learning and academic achievement across childhood and adolescence: a meta-analysis. Educ. Psychol. Rev. 28, 425–474. doi: 10.1007/s10648-015-9320-8
Dignath, C. (2016). Which components of teacher competence determine whether teachers enhance self-regulated learning? Predicting teachers’ self-reported promotion of self-regulated learning by means of teacher beliefs, knowledge, and self-efficacy. Frontline Learn. Res. 4, 83–105. doi: 10.14786/flr.v4i5.247
Dignath, C. (2021). For unto every one that hath shall be given: teachers’ competence profiles regarding the promotion of self-regulated learning moderate the effectiveness of short-term teacher training. Metacogn. Learn. 16, 555–594. doi: 10.1007/s11409-021-09271-x
Dignath, C., and Büttner, G. (2008). Components of fostering self-regulated learning among students. A meta-analysis on intervention studies at primary and secondary school level. Metacogn. Learn. 3, 231–264. doi: 10.1007/s11409-008-9029-x
Dignath, C., and Veenman, M. V. J. (2021). The role of direct strategy instruction and indirect activation of self-regulated learning—evidence from classroom observation studies. Educ. Psychol. Rev. 33, 489–533. doi: 10.1007/s10648-020-09534-0
Eccles, J. S., and Wigfield, A. (2002). Motivational beliefs, values, and goals. Annu. Rev. Psychol. 53, 109–132. doi: 10.1146/annurev.psych.53.100901.135153
Engels, M. C., Spilt, J., Denies, K., and Verschueren, K. (2021). The role of affective teacher-student relationships in adolescents’ school engagement and achievement trajectories. Learn. Instr. 75:101485. doi: 10.1016/j.learninstruc.2021.101485
Fauth, B., Atlay, C., Dumont, H., and Decristan, J. (2021). Does what you get depend on who you are with? Effects of student composition on teaching quality. Learn. Instr. 71:101355. doi: 10.1016/j.learninstruc.2020.101355
Finch, W. H., and Bolin, J. E. (2017). Multilevel Modeling Using Mplus. Boca Raton: Taylor & Francis Group.
Hafen, C. A., Hamre, B. K., Allen, J. P., Bell, C. A., Gitomer, D. H., and Pianta, R. C. (2015). Teaching through interactions in secondary school classrooms: revisiting the factor structure and practical application of the classroom assessment scoring system-secondary. J. Early Adolesc. 35, 651–680. doi: 10.1177/0272431614537117
Hamre, B. K., Pianta, R. C., Downer, J. T., Dcoster, J., Mashburn, J., Jones, S. M., et al. (2013). Testing a developmental framework of teacher. Elem. Sch. J. 113, 461–487. doi: 10.1086/669616
Harding, S. M., English, N., Nibali, N., Griffin, P., Graham, L., Alom, B., et al. (2019). Self-regulated learning as a predictor of mathematics and reading performance: a picture of students in grades 5 to 8. Aust. J. Educ. 63, 74–97. doi: 10.1177/0004944119830153
Havik, T., and Westergård, E. (2020). Do teachers matter? Students’ perceptions of classroom interactions and student engagement. Scand. J. Educ. Res. 64, 488–507. doi: 10.1080/00313831.2019.1577754
Heirweg, S., De Smul, M., Devos, G., and Van Keer, H. (2019). Profiling upper primary school students’ self-regulated learning through self-report questionnaires and think-aloud protocol analysis. Learn. Individ. Differ. 70, 155–168. doi: 10.1016/j.lindif.2019.02.001
Hofkens, T., Pianta, R. C., and Hamre, B. (2023). “Teacher-student interactions: theory, measurement, and evidence for universal properties that support students’ learning across countries and cultures” in Effective teaching around the world: theoretical, empirical, methodological and practical insights. eds. R. Maulana, M. Helms-Lorenz, and R. M. Klassen (Switzerland: Springer), 399–422.
Hox, J. J., Moerbeek, M., and van de Schoot, R. (2018). Multilevel analysis techniques and applications. (3rd ed.) Edn: New York: Routledge.
Karlen, Y., Hertel, S., and Hirt, C. N. (2020). Teachers’ professional competences in self-regulated learning: an approach to integrate teachers’ competences as self-regulated learners and as agents of self-regulated learning in a holistic manner. Front. Educ. 5:159. doi: 10.3389/feduc.2020.00159
Karlen, Y., Hirt, C. N., Jud, J., Rosenthal, A., and Eberli, T. D. (2023). Teachers as learners and agents of self-regulated learning: the importance of different teachers competence aspects for promoting metacognition. Teach. Teach. Educ. 125:104055. doi: 10.1016/j.tate.2023.104055
Kline, R. B. (2016). Principles and practice of structural equation Modeling. (4th ed.). Edn. New York: The Guilford Press.
Lawson, M. J., Vosniadou, S., Van Deur, P., Wyra, M., and Jeffries, D. (2019). Teachers’ and Students’ Belief Systems About the Self-Regulation of Learning. Educational Psychology Review 31, 223–251. doi: 10.1007/s10648-018-9453-7
Marsh, H. W., Lüdtke, O., Nagengast, B., Trautwein, U., Morin, A. J. S., Abduljabbar, A. S., et al. (2012). Classroom climate and contextual effects: conceptual and methodological issues in the evaluation of group-level effects. Educ. Psychol. 47, 106–124. doi: 10.1080/00461520.2012.670488
Mashburn, A. J., Downer, J. T., Rivers, S. E., Brackett, M. A., and Martinez, A. (2013). Improving the power of an efficacy study of a social and emotional learning program: application of generalizability theory to the measurement of classroom-level outcomes. Prev. Sci. 15, 146–155. doi: 10.1007/S11121-012-0357-3/FIGURES/2
Moos, D. C., and Ringdal, A. (2012). Self-regulated learning in the classroom: a literature review on the teacher’ s role. Educ. Res. Int. 2012, 1–15. doi: 10.1155/2012/423284
Morin, A. J. S., Marsh, H. W., Nagengast, B., and Scalas, L. F. (2014). Doubly latent multilevel analyses of classroom climate: an illustration. J. Exp. Educ. 82, 143–167. doi: 10.1080/00220973.2013.769412
Muthén, L. K., and Muthén, B. O. (2017). Mplus User’s Guide. 8th Edn. Los Angeles, CA: Muthén & Muthén.
Panadero, E. (2017). A review of self-regulated learning: six models and four directions for research. Front. Psychol. 8, 1–28. doi: 10.3389/fpsyg.2017.00422
Perry, J., Lundie, D., and Golder, G. (2019). Metacognition in schools: what does the literature suggest about the effectiveness of teaching metacognition in schools? Educ. Rev. 71, 483–500. doi: 10.1080/00131911.2018.1441127
Pianta, R. C., Hamre, B. K., and Mintz, S. (2012). Classroom assessment scoring system. Charlottesville: Teachstone.
Pintrich, P. R. (2004). A conceptual framework for assessing motivation and self-regulated learning in college students. Educ. Psychol. Rev. 16, 385–407. doi: 10.1007/s10648-004-0006-x
Rieser, S., Fauth, B. C., Decristan, J., and Büttner, G. (2013). Special issue articles the connection between primary school students’ self-regulation in learning and perceived teaching quality. J. Cogn. Educ. Psychol. 12, 138–156. doi: 10.1891/1945-8959.12.2.138
Rimm-Kaufman, S. E., Baroody, A. E., Larsen, R. A. A., Curby, T. W., and Abry, T. (2014). To what extent do teacher-student interaction quality and student gender contribute to fifth graders’ engagement in mathematics learning? J. Educ. Psychol. 107, 170–185. doi: 10.1037/a0037252
Schenke, K. (2018). From structure to process: do students’ own construction of their classroom drive their learning? Learn. Individ. Differ. 62, 36–48. doi: 10.1016/j.lindif.2018.01.006
Schunk, D. H., and Greene, J. A. (2018). “Historical, contemporary, and future perspectives on self-regulated learning and performance” in Handbook of self-regulation of learning and performance. eds. D. H. Schunk and J. A. Greene. 2nd ed (New York: Routledge/Taylor & Francis Group), 1–15.
Skinner, E., Furrer, C., Marchand, G., and Kindermann, T. (2008). Engagement and disaffection in the classroom: part of a larger motivational dynamic? J. Educ. Psychol. 100, 765–781. doi: 10.1037/a0012840
Tabachnick, B. G., and Fidell, L. S. (2014). Using multivariate statistics. (6th ed.). Edn: Boston, MA: Pearson.
Usher, E. L., and Schunk, D. H. (2018). “Social cognitive theoretical perspective of self-regulation” in Handbook of self-regulation of learning and performance. eds. D. H. Schunk and J. A. Greene. 2nd ed (New York: Routledge/Taylor & Francis Group), 19–35.
van Dijk, W., Gage, N. A., and Grasley-Boy, N. (2019). The relation between classroom management and mathematics achievement: a multilevel structural equation model. Psychol. Sch. 56, 1173–1186. doi: 10.1002/pits.22254
Vandevelde, S., Van Keer, H., and Merchie, E. (2017). The challenge of promoting self-regulated learning among primary school children with a low socioeconomic and immigrant background. J. Educ. Res. 110, 113–139. doi: 10.1080/00220671.2014.999363
Vandevelde, S., van Keer, H., and Rosseel, Y. (2013). Measuring the complexity of upper primary school children’s self-regulated learning: a multi-component approach. Contemp. Educ. Psychol. 38, 407–425. doi: 10.1016/j.cedpsych.2013.09.002
Veenman, M. V. J. (2017). Assessing metacognitive deficiencies and effectively instructing metacognitive skills. Teach. Coll. Rec. 119, 1–20. doi: 10.1177/016146811711901303
Wang, M. T., Hofkens, T., and Ye, F. (2020). Classroom quality and adolescent student engagement and performance in mathematics: a multi-method and multi-informant approach. J. Youth Adolesc. 49, 1987–2002. doi: 10.1007/s10964-020-01195-0
Zee, M., de Jong, P. F., and Koomen, H. M. Y. (2016a). Teachers’ self-efficacy in relation to individual students with a variety of social-emotional behaviors: a multilevel investigation. J. Educ. Psychol. 108, 1013–1027. doi: 10.1037/EDU0000106
Zee, M., Jong, P. F., and Koomen, H. M. Y. (2024). The relational side of teachers’ self-efficacy: assimilation and contrast effects of classroom relational climate on teachers’ self-efficacy. J. Sch. Psychol. 103:101297. doi: 10.1016/j.jsp.2024.101297
Zee, M., and Koomen, H. M. Y. (2016). Teacher self-efficacy and its effects on classroom processes, student academic adjustment, and teacher well-being: a synthesis of 40 years of research. Rev. Educ. Res. 86, 981–1015. doi: 10.3102/0034654315626801
Zee, M., and Koomen, H. M. Y. (2020). Engaging children in the upper elementary grades: unique contributions of teacher self-efficacy, autonomy support, and student-teacher relationships. J. Res. Child. Educ. 34, 477–495. doi: 10.1080/02568543.2019.1701589
Zee, M., Koomen, H. M. Y., and de Jong, P. F. (2018). How different levels of conceptualization and measurement affect the relationship between teacher self-efficacy and students’ academic achievement. Contemp. Educ. Psychol. 55, 189–200. doi: 10.1016/j.cedpsych.2018.09.006
Zee, M., Koomen, H. M. Y., Jellesma, F. C., Geerlings, J., and de Jong, P. F. (2016b). Inter- and intra-individual differences in teachers’ self-efficacy: a multilevel factor exploration. J. Sch. Psychol. 55, 39–56. doi: 10.1016/j.jsp.2015.12.003
Keywords: self-regulated learning skills, student-specific teacher self-efficacy, teacher student interactions, elementary schools, multilevel modeling
Citation: de Ruig NJ, Zee M and de Jong PF (2024) Understanding the interplay between teacher self-efficacy, teacher–student interactions, and students’ self-regulated learning skills at different levels of classroom ecology. Front. Educ. 9:1392907. doi: 10.3389/feduc.2024.1392907
Edited by:
Barbara Otto, Fresenius University of Applied Sciences, GermanyReviewed by:
Zhengdong Gan, University of Macau, ChinaElena Mirela Samfira, University of Life Sciences “King Mihai I” from Timisoara, Romania
Copyright © 2024 de Ruig, Zee and de Jong. This is an open-access article distributed under the terms of the Creative Commons Attribution License (CC BY). The use, distribution or reproduction in other forums is permitted, provided the original author(s) and the copyright owner(s) are credited and that the original publication in this journal is cited, in accordance with accepted academic practice. No use, distribution or reproduction is permitted which does not comply with these terms.
*Correspondence: Niels J. de Ruig, n.j.deruig@uva.nl