- 1Programs of Master and Doctorate in Psychology, University of Sonora, Hermosillo, Mexico
- 2Bachelor’s Degree Program of Health Psychology, University of Sonora, Cajeme, Mexico
The relationship between career choice and academic achievement associated with science, technology, engineering, and mathematics (STEM) fields has been extensively studied from the Educational Psychology Approach. The present study examined the impact of academic motivation on affective engagement, focusing on the mediating role of self-efficacy in pre-college students. A sample of 324 students between 17 and 19 years (M = 17.41; SD = 0.506; 51% females, 49% males) from different schools located in northwestern Mexico participated in completing a self-report questionnaire about math and science engagement, self-efficacy to science and math, and academic motivation. Statistical analyses were carried out to determine the reliability and validity of the measurement scale; posteriorly, a mediation analysis was used to determinate the direct and indirect effects of the structural model. The results revealed high correlations between the three study variables. Both mediation analysis and structural equation modeling indicated that academic motivation influences affective engagement through its relationship with self-efficacy. Although self-efficacy has a direct effect on affective engagement, it is also affected by academic motivation, so the interaction between these two generates an influence on affective engagement. In this research, a strong relationship was observed between academic motivation and self-efficacy, indicating that students who are motivated and enjoy performing activities related to their science and math classes have previous experiences of success in these tasks. These results provide new insights for the improvement of strategies regarding the training and performance of pre-university students in the areas of science and mathematics by considering their affective states.
1 Introduction
Scientific knowledge, research and technological development are important elements for the development of education and society (Organisation for Economic Co-operation and Development OECD, 2018). Therefore, promoting and disseminating the vocation and engagement to science in educational and social contexts is a strategy that could lead to an increase of university enrollment in undergraduate or engineering degrees related to Science, Technology, Engineering and Mathematics (STEM). In addition, training in science allows the development of specific skills for decision making, critical thinking, and scientific reasoning, which according to Madjar et al. (2017) can be translated into competencies for life.
Understanding the causality of the events surrounding human beings allows the elaboration of explanations and rules of how various events operate and provides the opportunity to intervene at certain moments of the processes. Bunge (2017) mentioned that scientific experimentation allows us to predict consequences and make changes. Hence, the training of students within the field where scientific and technological innovation is produced can reinforce critical thinking and offer contributions for the solution of future conflicts in society.
In the Mexican context, during the last few years, new guidelines and training in science have been implemented to strengthen the scientific community due to the lag in the production of new knowledge and the lack of engagement of new generations (National Council of Humanities, Sciences and Technologies - CONAHCYT, 2023). Moreover, public policies have presented changes in the implementation of new strategies that promote the development of positive skills toward Science and Mathematics for the formation of responsible and informed decisions, the reflection of scientific knowledge, and how this can help to improve people’s life condition in the future (Orendain, 2019). This lack of engagement in scientific and mathematics is justified by the results in the latest international evaluations of basic education levels, such as the Program for International Student Assessment (PISA). The 2018 application yielded an average below the one established by the Organization for Economic Cooperation and Development (OECD) (x = 490) in the mastery of science and mathematics skills in basic education students.
In university education, the National Association of Universities and Higher Education Institutions (ANUIES, 2020) presented in the 2020 report the admission, completion, and graduation rates, which reflect a low enrollment in STEM-related careers. In regard to the graduation rates, from the 100% of students enrolled, only 17% finished their studies, and 13% managed to obtain a university degree. In the case of the productive sector, the National Institute of Statistics and Geography INEGI (2020) mentioned that these types of careers have a high labor supply due to the low demand they present, but why have these issues related to the disciplines of science and mathematics arisen? Studies developed in the Mexican context [i.e., (Romero Bojórquez et al., 2014)] have identified that negative emotional factors play a significant role in the learning of mathematics and science. In particular, frustration and anxiety occur frequently among high school students when facing activities related to science and mathematics affecting their performance and attitude toward these subjects. In addition, García-Santillán et al. (2016) indicated that problem solving in science and mathematics classes can trigger or encourage negative emotions in students and could influence their establishment of professional goals and their academic motivation. Likewise, exploratory studies have found that the presence of positive self-efficacy beliefs in the school context are related to cognitive and affective variables in adolescents (Ozkal, 2019).
In such manner, the study of academic motivation and self-efficacy beliefs in science and mathematics is important because they are considered predictors for student engagement (Azila-Gbettor et al., 2021; Mellado Jiménez et al., 2014; Hampden-Thompson and Bennett, 2011; Singh and Abdullah, 2020; Froiland and Worrell, 2016).
2 Theoretical background
In the field of psychology and education, there has been discussion regarding the factors that determine career choices, academic learning outcomes, and student achievement expectations in the context of undergraduate education (Eccles and Wigfield, 2020; Pedrero and Manzi, 2020). Engagement, motivation, and self-efficacy are some of the psychological constructs most frequently found in studies related to academic achievement and career choice orientation in science (Cerinsek et al., 2012; Holmes et al., 2018; Lichtenberger and George-Jackson, 2012; Stankov and Lee, 2014).
Thus, Eccles and Wigfield (2020) in the situated expectancy-value model established the importance of a cognitive component related to success expectations and value attributable to different academic and/or professional options available to everyone. Their analysis focused on the competencies and beliefs that in turn can be influenced by cultural norms and positive experiences (Eccles et al., 1998; Wang and Degol, 2014). In such a manner, the situated expectancy-value theory is a broad framework that allows exploring factors considered by students to dedicate themselves to the STEM area (Eccles and Wigfield, 2020; Meece et al., 1982; Simpkins et al., 2015).
In addition, directing professional life toward STEM-related fields involves a process of reasoning and psychological factors in students. This decision-making process is often influenced by internal factors such as the degree of engagement, which is referred to as the observable and unobservable qualities of interactions with learning activities and students’ choice processes (Deci and Ryan, 1985; Wang et al., 2016).
2.1 Affective engagement
In the school context, engagement refers to students’ participation in their education, as well as the relationships developed with other people and goals (Pedrero and Manzi, 2020; Skinner et al., 2009). Some studies have referred to student engagement toward academic areas of science and mathematics as part of attitudes toward these subjects, the degree of participation, practical and theoretical training in STEM areas, student self-efficacy, and social perception toward these professions (Lupión et al., 2019; Williams et al., 2021). Also, this construct has been addressed as an important factor in the process of vocational choice related to university careers in the field of science and mathematics (Dopico and Amstrong, 2017), as well as studies that explain students’ motivation to learn about science (Oppermann et al., 2019).
Similarly, according to Fredricks (2011), engagement is composed of three major dimensions: the first is behavioral, related to the performance of activities associated with the goal; followed by the cognitive dimension, related to self-regulation and understanding of topics or activities related to the goal; and finally, the affective component, where positive or negative emotions are manifested toward certain topics or even teachers (Wang and Liou, 2018). Engagement is considered fundamental to academic achievement in subjects such as science and mathematics (Lee et al., 2019; Wang and Degol, 2014), because when a student is engaged, he or she is influenced by motivation and his or her beliefs about achieving the goals set in school. Emotions and feelings are an integral part of students when they experience learning situations, and this can influence their engagement in certain academic activities when they experience positive emotions, interest, or negative emotions such as frustration or boredom (Attard et al., 2020; Reeve, 2012).
Studies have found that student engagement is related to excellent functioning and learning motivation (Fraysier et al., 2020). Additionally, this behavioral and affective engagement is modulated by the level of self-efficacy of students (Olivier et al., 2019).
2.2 Academic motivation
It has been studied that one of the main causes of behavior is the motives that lead people to perform them, since motivation affects the initiation, persistence, change, direction toward goals and termination of any behavior (Reeve, 2018). The sources of a person’s motivation can be intrinsic, derived from internal processes associated with pleasure and enjoyment; or extrinsic, which comes from external forces involving behaviors aimed at meeting specific goals (Deci and Ryan, 1985; Staribratov and Babakova, 2019).
Within Educational Psychology, academic motivation is referred to as the disposition that is related to academic functioning, learning and success, as well as the effort made by students and persistence to achieve the goals, they set for themselves (Schunk, 2008). Research studies have shown how motivation is related with students’ engagement (Schunk and Mullen, 2012). Other studies explain that motivational regulation is a positive predictor of satisfaction to learn and study STEM-related subjects and/or careers; also, studies found indirect effects with affective states, such as stress (Kryshko et al., 2022; Oppermann et al., 2019; Wang and Liou, 2018).
For the present study, greater emphasis is placed on intrinsic motivation for learning because of the relationship found with positive affective states and the development of positive attitudes toward science and mathematics in high school students (De Loof et al., 2019). Also, studies presented relationship between academic motivation with young people’s perceived self-efficacy about their abilities in science and mathematics, which predicts likely what they are to choose and pursue STEM careers (Kıran et al., 2019; Fong-Silva et al., 2017; Silva, et al., 2018; Sáinz et al., 2020). Moreover, the positive relationship between engagement and academic motivation (De Naeghel et al., 2012), and with academic achievement in science and mathematics (Singh et al., 2002).
2.3 Self-efficacy as a mediator between academic motivation and affective engagement
In school contexts and educational psychology, the term self-efficacy proposed by Albert Bandura (1997) has been used to explain how a belief or judgment that a person has toward him/herself about the skills he/she possesses influences the fulfillment of certain activities or goals (Wigfield et al., 2011; Wigfield and Eccles, 2023). In this context, self-efficacy is a belief that affects, on a regular basis in a positive way, students’ academic achievement outcomes resulting in the choice of tasks that represent difficulty for them, greater planning of the strategies to be used and engagement toward certain learning situations (Zeldin et al., 2008; Usher et al., 2019; Zimmerman and Cleary, 2009). These beliefs or judgments stem from sources such as previous experiences of success, peer comparison experiences, social persuasion, and emotional states (Bandura, 1997), which, along with motivation are considered determinants toward choosing STEM careers and engagement to staying in them (Rosenzweig and Wigfield, 2016). In the field of educational psychology, studies have shown that self-efficacy is associated with affective engagement and academic achievement (Olivier et al., 2019), as well as to learning performance in science and mathematics (Ozkal, 2019). Also, it plays a critical role in the self-regulation of motivation and engagement (Azila-Gbettor et al., 2021; Close and Solberg, 2008).
On the other hand, self-efficacy in various studies has been determined as a mediating variable for career choice (Agoes Salim et al., 2023; Hackett and Betz, 1995; Restubog et al., 2010; Song and Chon, 2012; Liu et al., 2023), and to explain learning and school performance associated with parental support (Cattelino et al., 2019). Others have taken up such variable to assess the effects of perceived task-goal structure, perceived ability structure, and academic performance (Høigaard et al., 2015), task appraisal and learning and achievement in mathematics and science (Gao et al., 2020; Yurt, 2022), as well as appraisal of learning environments and academic engagement (Sökmen, 2021). This construct has also been used to explain and predict good performance in science and mathematics, such as the likelihood of choosing a career in the STEM field (Van Aalderen-Smeets et al., 2019; Jansen et al., 2015; Kulakow, 2020).
The aforementioned studies take up this construct as a mediating variable. In statistical terms, mediation refers to a causal link, where it is assumed that the effect of one or more independent variables is transmitted to other dependent variables through a third variable, and this link indicates the effect between these variables (Baron and Kenny, 1986; MacKinnon et al., 2007). Mediation can occur in three different types: complementary mediation, where direct and indirect effects are significant and point in the same direction; competitive mediation, where direct and indirect effects are significant, but go in opposite directions; and indirect mediation, where indirect effects are significant, but the direct effect is not (Hayes, 2013; Hair et al., 2017).
2.4 The present study
Given that, in educational y psychological research, emphasis is placed on motivation, self-efficacy beliefs, and engagement as important constructs for goal achievement and career choice in STEM-related subjects. It was decided to investigate the relationship between these three variables. In addition, since self-efficacy may play a role as a mediating variable for the relationship of academic motivation and affective engagement, the assessment of this mediation was determined. It was also tested how self-efficacy affects this relationship. In Figure 1, we hypothesize that:
H1: Academic Motivation would be positively related to Affective Engagement.
H2: Academic Motivation would be positively related to Self-Efficacy.
H3: Self-efficacy would be positively related to Affective Engagement.
3 Methods
3.1 Sample
The sample was delimited using the non-probability method by quotas, since the selection of participants did not depend on probability but on causes related to the characteristics of the research (Hernández, et al., 2014). A total of 324 high school senior students from the city of Hermosillo, Mexico participated, belonging to four schools: three from the private sector (47.5%) and one from the public sector (52.5%). The sample consisted of 49% male and 51% female students aged between 17 and 19 years old (M = 17.41, SD = 0.506).
3.2 Measurement
Math and Science Engagement Scale: Participants used a 10-item scale modified from Wang et al. (2016), which measures the degree of emotional co-engagement that high school students have toward science and math related classes and activities. Each item was measured with a 5-point Likert scale (1 = agree, 5 = disagree). The items included situations such as “I think that math/science class is not boring,” “I care about learning science/math” and “I often feel good when I am in science/math class.”
Self-efficacy Scale: We developed a scale to assess students’ perceptions of self-efficacy in relation to their previous success experiences during science and mathematics classes, as well as their emotional states in these subjects (Bandura, 1997). The 12-item scale was measured on a 5-point Likert scale (1 = Never, 5 = Always).
Academic Motivation Scale: A motivation scale for science and mathematics was adapted from the Academic Motivation Scale instrument (AMS-Mathematics: Staribratov and Babakova, 2019). The scale consisted of 11 items divided into two factors: intrinsic motivation and extrinsic motivation. The response options ranged from 1 (strongly disagree) to 5 (strongly agree).
3.3 Procedure
After the approval of the Research Ethics Committee of the University of Sonora (CEI-UNISON), we contacted the high schools’ principals with the highest enrollment in the city to request permission to apply the surveys to senior students. Once granted, we approached the classrooms and explained the purpose of the study. Participation was completely voluntary and anonymous, and participants were invited to sign a statement of informed consent. It took an average of 25 min to complete the questionnaires. Data was collected in May 2022, and the participation rate was approximately 78.07%.
3.4 Statistical analysis
The data was captured and analyzed using the Statistical Package for the Social Sciences (SPSS v25.0) to obtain the psychometric properties of each of the scales. Univariate descriptive statistics were calculated for each of the items, as well as the internal consistency through Cronbach’s alpha, and the correlations between each of the variables.
To test the hypotheses, a series of statistical analyses were performed in the framework of Structural Equation Modeling (SEM). First, structure was obtained through Confirmatory Factor Analysis (CFA) for each of the variables; after that, SEM was tested in EQS Software 6.1. The indices used for estimating the goodness of fit of the models were chi-square hypothesis test (x2), normed fit index (NFI), non-normed fit index (NNFI), comparative fit index (CFI) and the root mean square error of approximation (RMSEA), (Hu and Bentler, 1999). Models were considered to have a good fit if all coefficients were marked as significant at the 0.05 level, goodness-of-fit indices scored above 0.95, and RMSEA remained below 0.08 (Bentler and Bonett, 1980; Bentler, 1990; Kline, 2016).
In addition, beta coefficients and mediation confidence intervals were calculated using SPSS v25.0 with the Process add-on (Hayes, 2013) allowing to establish the dependence relationship between two or more variables and the values of a third variable as mediator in the model. To test the role of mediation and its effects, the bootstrapping method was used which involves repeated sampling of the data set and estimates the indirect effect and confidence intervals (CI) for the mediation effect. A 95% CI with 5,000 iterations was used allowing us to find more precise intervals and determine the significance of the effects, with the aim of accepting the raised hypotheses (Hayes, 2013; Hair et al., 2017).
4 Results
4.1 Descriptive statistics and correlation analysis
Table 1 presents the variables correlation matrix and their internal consistency. Cronbach’s alpha values of the different scales used in the study were appropriate (a= > 0.6) indicating adequate consistency in each one. As for the correlations, it was found that self-efficacy has a positive and highly significant correlation with academic motivation (r = 0.767**), followed by the relationship between affective engagement and self-efficacy (r = 0.621**). These observations lead us to question if the scales for self-efficacy and academic motivation measure very similar concepts or refers to a conditional effect. Therefore, the existence of multicollinearity was verified through a regression analysis using variance inflation factors (VIF) and tolerance statistics (Berseley et al., 1980). Through the results, we can determine that there is no evidence of collinearity when entering self-efficacy alongside academic motivation (VIF = 1.000, Tolerance = 1.000). Additionally, in the linear regression analysis between the variables mentioned, Mahalanobis distance and Cook’s distance and distance were assessed to identify influential and outlying observations. Mahalanobis distance reported a minimum value of 0.011, a maximum value of 8.021 (M = 0.997, S.D. = 1.41), indicating that while most observations are close to the multivariate mean, some are potentially outliers and could be significantly distant from the center of the distribution, warranting further examination to ensure the robustness of the model. In contrast, Cook’s distance exhibited a minimum value of 0.00, a maximum value of 0.49 (M = 0.003, S.D. = 0.006), suggesting that most observations exert minimal influence on the estimation of regression coefficients, although one observation with 0.49 might have a relatively greater impact without exceeding the commonly concerning threshold of 1 (Cook and Weisberg, 1982).
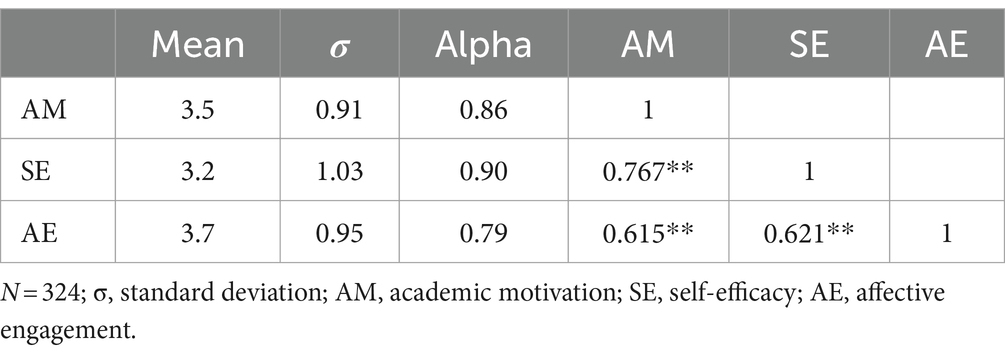
Table 1. Univariate statistics and their relationship with academic motivation, self-efficacy, and affective engagement.
Regarding the results of the regression analysis between affective engagement and self-efficacy, significant patterns emerge. The Mahalanobis distance exhibits moderate variability among observations, ranging from a minimum of 0.000 to a maximum of 4.18, indicating that some observations deviate notably from the centroid of the group. Univariate statistics suggest considerable dispersion around the mean (M = 0.997; S.D. = 1.185). Both VIF and tolerance values are 1.000, indicating no serious issues of multicollinearity. Additionally, the Cook’s distance shows minimal changes due to outlier observations, with a maximum of 0.105 and a mean of 0.004.
In addition, the Durbin-Watson statistic was also considered to test autocorrelation, whose values were found to be d = 1.92 for the relationship between self-efficacy and affective engagement; and d = 2.15 for the relationship between self-efficacy and academic motivation.
4.2 Structural equation model
The confirmatory factor analysis (CFA) was performed using the robust maximum likelihood estimation method to estimate the goodness-of-fit indicators as well as the factor loadings for each of the indicators of the three latent variables of the study (Stommel et al., 1992). The test of the measurement models resulted in satisfactory fits to the data. In addition, all factor loadings on the latent variables were significant (p < 0.05) indicating that the latent constructs were well represented by their indicators.
In our structural model, the mediating role of self-efficacy was tested in relation to academic motivation and affective engagement (Figure 2). All paths were marked as significant. The goodness-of-fit indexes indicated an acceptable fit between the data and the structural model (x2: 80.66, 29 gL, p < 0.05; BBNFI = 0.96; BBNNFI = 0.96; CFI = 0.98; RMSEA = 0.07, explaining 77% of the total variance).
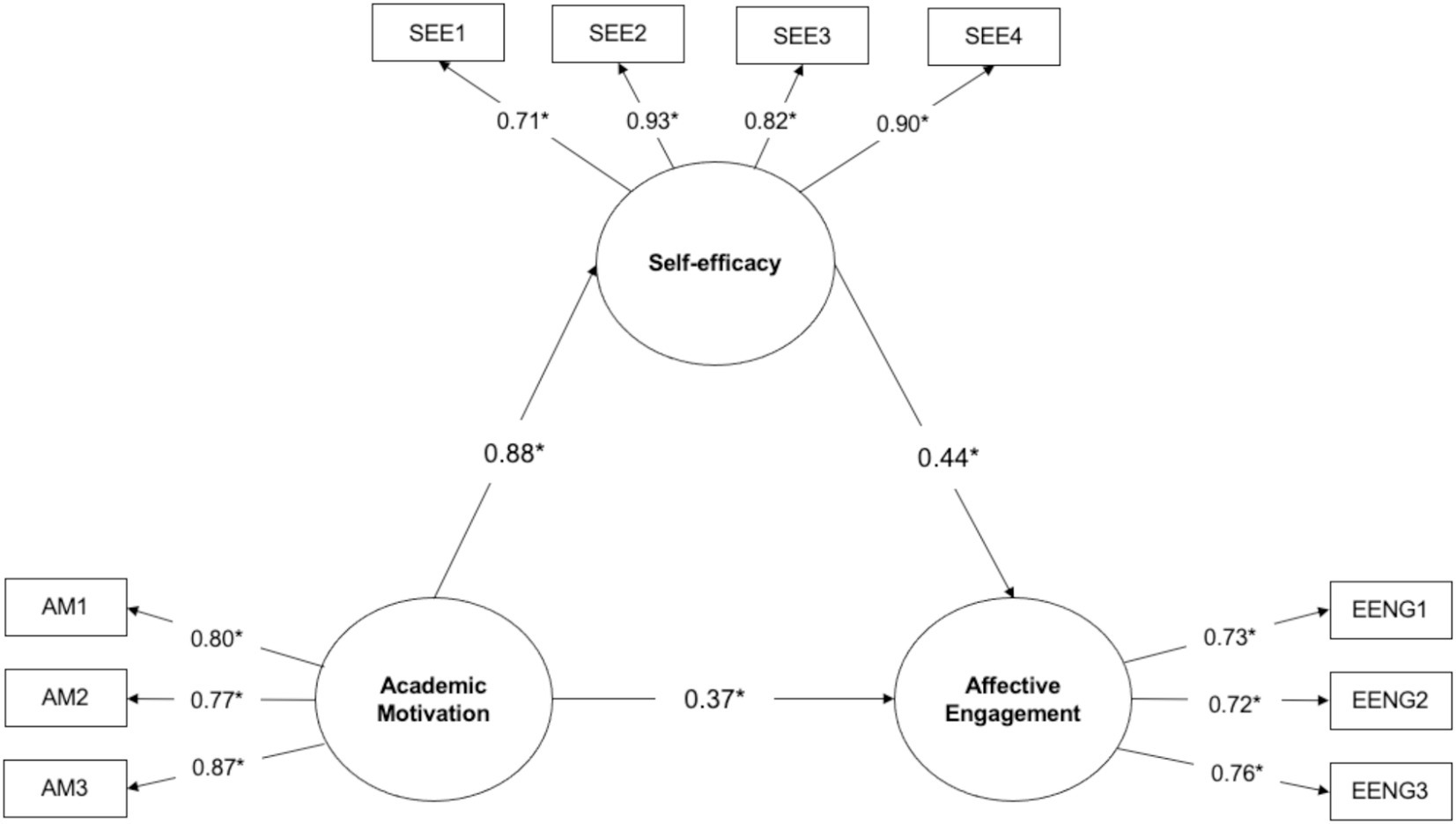
Figure 2. Structural equation model between academic motivation, self-efficacy and affective engagement with standardized estimates. Goodness of fit: X2 = 80.66 (29 df), p = 0.000, BBNFI = 0.96, BBNNFI = 0.96, CFI = 0.98, RMSEA = 0.07. R2 = 0.77.
4.3 Direct and indirect effects
When examining the total effect of academic motivation on affective engagement, a statistically significant value was found [β = 0.648, p = 0.00, 95% CI (0.73; 0.61)]. Thus, the result indicates that academic motivation positively predicts affective engagement toward science and mathematics. As for the direct effect of academic motivation toward self-efficacy, positive and significant values were found [β = 0.601, p = 0.00, 95% CI (1.05; 1.27)] which represents a coefficient very similar to the total effect. The direct effect of self-efficacy on affective engagement also showed a positive and significant result, but with a lower coefficient [β = 0.362, p = 0.00, 95% CI (0.16, 0.34)].
When we examined the indirect effects (Table 2), it was found that the effect of the mediating variable self-efficacy on the relationship between academic motivation and affective engagement was a complementary mediation [β = 0.292, p = 0.00, 95% C (0.16; 0.42)]; since, despite presenting a smaller effect, it did not fail to be statistically significant (Baron and Kenny, 1986; Hayes, 2013).
The results indicated that academic motivation affects affective engagement through its relationship with self-efficacy. Although self-efficacy has a direct effect to affective engagement, it is also affected by academic motivation, so the interaction between these two generate an influence on affective engagement (AM*SE; 0.77*0.36). The interaction gives the indirect effect value of 0.27 and allows to explain the correlation value of 0.61 between academic motivation and affective engagement. That is, to improve engagement it is necessary to affect the academic motivation and self-efficacy, since together generates an indirect effect on affective engagement.
5 Discussion
This study examined the relationship between academic motivation, self-efficacy, and affective engagement. In addition, the mediating role of self-efficacy between the relationship between academic motivation and affective engagement was analyzed. Prior to the implementation of the structural equation model, the constructs were previously validated by obtaining acceptable goodness-of-fit indexes according to the criteria established by Bentler and Bonett (1980) and Kline (2016). The tested model consisted of academic motivation as an independent variable, affective engagement as a dependent variable, and self-efficacy as a mediating variable. It was found that self-efficacy plays the role of complementary mediation in the relationship, as both its direct and indirect effects were significant (Hayes, 2013; Hair et al., 2017).
Firstly, it was found during the measurement models of each variable that students take into consideration their emotional states in their decisions about science and/or mathematics careers and their performance in such subjects during school. Academic motivation presented high factorial loads in the items related to enjoyment of related activities, which is consistent with the studies of Mai et al. (2023), Membiela et al. (2023), Wood (2019). As with the variable of engagement, the dimension of affective states was the only one able to explain engagement with science and mathematics in high school seniors, which is a similar result to other research areas of psychology and STEM education (Jang et al., 2010; Murphy et al., 2019; Lee et al., 2019; Phillips et al., 2019).
As for the mediating variable of self-efficacy, there are numerous studies in the field of educational psychology that take up this psychological variable to explain how students’ beliefs affect their academic performance. In this research, a strong relationship was observed between academic motivation and self-efficacy, indicating that students who are motivated and enjoy performing activities related to their science and math classes have previous experiences of success in these tasks; in addition to experiencing positive affective states (Bellová et al., 2023; Ozkal, 2019; Kwon et al., 2023).
For the full structural model, we can conclude that these three psychological constructs help us to understand and explain motivation, beliefs about learning, fulfillment, and goal orientation of students at different educational levels. In high school students, who are close to making a career choice, it is considered important to address affective states, since are an important element that is not considered in educational settings, although they are an important factor for decision making and for the development of engagement toward science and mathematics (Iztek-Greulich and Vollmer, 2017). Likewise, self-efficacy was found to have an indirect effect in the existing relationship between academic motivation and affective engagement with science and mathematics among high school students, so that the development of engagement to STEM fields and activities depends not only on students’ motivation, but also on their beliefs about their own scientific and mathematical abilities and their previous and enjoyable experiences (Jones et al., 2000).
5.1 Implications for educational psychology
Our findings serve to provide a new perspective on the approach to the development of vocations in science and mathematics, as well as to reinforce and justify the importance of the implementation of programs such as PAUTA, which is a strategy that promotes the development of scientific vocations and the reinforcement of skills in children and adolescents with outstanding interest and aptitude in science and is responsible for accompanying them throughout their school career. This program also carries out the work of teacher training in science and links with the scientific community (PAUTA, 2013). The study also helps to confirm that the intervention of the psychologist in educational contexts is of utmost importance to identify the needs that students may have to achieve goals in science and/or mathematics, and thus provide the necessary strategies for both students and teachers to develop self-efficacy beliefs and thus motivate and engage students in learning various topics in STEM education and educational psychology.
5.2 Limitations
Our limitations are related to the size of the sample used for the study, in addition to the fact that the students were selected for the convenience of the research team, considering only that they were students about to graduate from high school. Therefore, the study is limited to a specific developmental environment due to the characteristics of the school environment where the data collection took place.
Several studies have also been conducted on the development of scientific vocation considering gender differences (Tzu-Ling, 2019; Ugwuanyi et al., 2020; Voica et al., 2020; Li and Singh, 2021; Fredricks et al., 2018; Tellhed et al., 2017; Liou et al., 2021), so for future research, in addition to expanding the sample, one could consider working on analyses that consider the demographic variable of gender and explore whether there are significant gender differences. Moreover, future research could explore the distinct measurement of science self-efficacy and mathematics self-efficacy among Mexican high school students. Although educational system typically integrates these subjects, understanding students’ perception specific to each discipline could provide more nuanced insights.
Data availability statement
The raw data supporting the conclusions of this article will be made available by the authors, without undue reservation.
Ethics statement
The studies involving humans were approved by the Research Ethics Committee of the University of Sonora (CEI-UNISON). The studies were conducted in accordance with the local legislation and institutional requirements. Written informed consent for participation in this study was provided by the participants’ legal guardians/next of kin.
Author contributions
RV-P: Conceptualization, Data curation, Formal analysis, Investigation, Methodology, Writing – original draft. CT-F: Formal analysis, Methodology, Supervision, Writing – original draft, Writing – review & editing. BF-S: Data curation, Methodology, Supervision, Writing – review & editing. JM-B: Data curation, Formal analysis, Methodology, Supervision, Writing – review & editing.
Funding
The author(s) declare that no financial support was received for the research, authorship, and/or publication of this article.
Conflict of interest
The authors declare that the research was conducted in the absence of any commercial or financial relationships that could be construed as a potential conflict of interest.
Publisher’s note
All claims expressed in this article are solely those of the authors and do not necessarily represent those of their affiliated organizations, or those of the publisher, the editors and the reviewers. Any product that may be evaluated in this article, or claim that may be made by its manufacturer, is not guaranteed or endorsed by the publisher.
References
Agoes Salim, R. M., Istiasih, M. R., Rumalutur, N. A., and Biondi Situmorang, D. D. (2023). The role of career decision self-efficacy as a mediator of peer support on students career adaptability. Heliyon 9:e14911. doi: 10.1016/j.heliyon.2023.e14911
Attard, C., Grootenboer, P., Attard, E., and Laird, A. (2020). “Affect and engagement in STEM education” in STEM education across the learning continuum. Eds. A. MacDonald, L. Danaia, and S. Murphy (Singapore: Springer) 195–212.
Azila-Gbettor, E. M., Mensah, C., Abiemo, M. K., Bokor, M., and Awan, R. U. N. (2021). Predicting student engagement from self-efficacy and autonomous motivation: a cross-sectional study. Cogent. Education 8, 1–14. doi: 10.1080/2331186X.2021.1942638
Baron, R. M., and Kenny, D. A. (1986). The moderator-mediator variable distinction in social psychological research: conceptual, strategic and statistical considerations. J. Pers. Soc. Psychol. 51, 1173–1182
Bellová, R., Balážová, M., and Tomčík, P. (2023). Are attitudes towards science and technology related to critical areas in science education? Res. Sci. Technol. Educ. 41, 1117–1132. doi: 10.1080/02635143.2021.1991298
Bentler, P. M., and Bonett, D. G. (1980). Significance test and goodness of fit in the analysis of covariance structures. Psychol. Bull. 88:588.
Cattelino, E., Morelli, M., Baiocco, R., and Chirumbolo, A. (2019). From external regulation to school achievement: the mediation of self-efficacy at school. J. Appl. Dev. Psychol. 60, 127–133. doi: 10.1016/j.appdev.2018.09.007
Cerinsek, G., Hribar, T., Glodez, N., and Dolinsek, S. (2012). Which are my future career priorities and what influenced my choice of studying science, technology, engineering or mathematics? Some insights on educational choice—case of Slovenia. Int. J. Sci. Educ. 35, 2999–3025. doi: 10.1080/09500693.2012.681813
Close, W., and Solberg, S. (2008). Predicting achievement, distress, and retention among lower-income Latino youth. J. Vocat. Behav. 72, 31–42. doi: 10.1016/j.jvb.2007.08.007
Cook, R. D., and Weisberg, S. (1982). Residual and influence in regression. London: Chapman and Hall.
De Loof, H., Struyf, A., Boeve-de Pauw, J., and Van Petegem, P. (2019). Teachers’ motivating style and students’ motivation and engagement in STEM: the relationship between three key educational concepts. Res. Sci. Educ. 1, 1–19. doi: 10.1007/s11165-019-9830-3
De Naeghel, J., Van Keer, H., Vansteenkiste, M., and Rosseel, Y. (2012). The relation between elementary students' recreational and academic reading motivation, reading frequency, engagement, and comprehension: a self-determination theory perspective. J. Educ. Psychol. 104, 1006–1021. doi: 10.1037/a0027800
Deci, E. L., and Ryan, R. M. (1985). Intrinsic motivation and self-determination in human behavior. 1st Edn. New York, NY: Springer.
Dopico, E., and Amstrong, C. (2017). Waking up scientific vocations. New trends and issues Proceedings on Humanities and Social Sciences. 3, 34–40. doi: 10.18844/prosoc.v3i1.1727
Eccles, J. S., and Wigfield, A. (2020). From expectancy-value theory to situated expectancy-value theory: a developmental, social, cognitive, and sociocultural perspective on motivation. Contemp. Educ. Psychol. 61:101859. doi: 10.1016/j.cedpsych.2020.101859
Eccles, J. S., Wigfield, A., and Schiefele, U. (1998). Motivation to succeed. In Handbook of Child Psychology: Vol. 3. Social, Emotional, and Personality Development. 5th ed. Eds. W. Damon and N. Eisenberg (New York: Wiley) pp. 1017–1095.
Fong-Silva, W., Curiel-Gómez, R., and Brito-Carrillo, C. (2017). Aprendizaje significativo y su relación con la motivación intrínseca, escuela de procedencia y estrategias cognitivas en estudiantes de ingeniería. IPSA Scientia Revista científica Multidisciplinaria 2, 55–63. doi: 10.25214/27114406.909
Fraysier, K., Reschly, A., and Appleton, J. (2020). Predicting postsecondary enrolment with secondary student engagement data. J. Psychoeduc. Assess. 38, 882–899. doi: 10.1177/0734282920903168
Fredricks, J. A. (2011). Engagement in school and out of school contexts: a multidimensional view of engagement. Theory Pract. 50, 327–335. doi: 10.1080/00405841.2011.607401
Fredricks, J. A., Hofkens, T. L., Wang, M. T., Mortenson, E., and Scott, P. (2018). Supporting girls’ and boys’ engagement in math and science learning: a mixed methods. J. Res. Sci. Teach. 55, 271–298. doi: 10.1002/tea.21419
Froiland, J. M., and Worrell, F. C. (2016). Intrinsic motivation, learning goals, engagement, and achievement in a diverse high school. Psychol. Sch. 53, 321–336. doi: 10.1002/pits.21901
Gao, S., Long, H., and Li, D. (2020). The mediation effect of student self-efficacy between teaching approaches and scienc achievement: findings from 2011 TIMSS US data. Soc. Psychol. Educ. 23, 385–410. doi: 10.1007/s11218-019-09534-1
García-Santillán, A., Moreno-García, E., Schnell, J., and Hernández, J. R. (2016). Anxiety towards mathematics on undergraduates in a Nautical School (An empirical study in Port Veracruz). Int. Electron. J. Math. Educ. 11, 2418–2429.
Hackett, G., and Betz, N. E. (1995). “Self-efficacy and career choice and development” in Self-efficacy, adaptation and adjustment. The plenum series in social/clinical psychology. Ed. J. E. Maddux (Boston, MA: Springer).
Hair, J. F., Hult, G. T., Ringle, C. M., Sarstedt, M., and Thiele, K. O. (2017). Mirror, mirror on the wall: a comparative evaluation of composite-based structural equation modeling methods. J. Acad. Market. Sci. 45, 616–632. doi: 10.1007/s11747-017-0517-x
Hampden-Thompson, G., and Bennett, J. (2011). Science teaching and learning activities and students’ engagement in science. Int. J. Sci. Educ. 35, 1325–1343. doi: 10.1080/09500693.2011.608093
Hayes, A. (2013). Introduction to mediation, moderation and conditional process analysis: A regression-based approach. New York, NY: Guilford Press.
Høigaard, R., Kovač, V. B., Øverby, N. C., and Haugen, T. (2015). Academic self-efficacy mediates the effects of school psychological climate on academic achievement. Sch. Psychol. Q. 30, 64–74. doi: 10.1037/spq0000056
Holmes, K., Gore, J., Smith, M., and Lloyd, A. (2018). An integrated analysis of school students’ aspirations for STEM careers: which student and school factors are most predictive? J. Sci. Math. Educ. 16, 655–675. doi: 10.1007/s10763-016-9793-z
Hu, L. T., and Bentler, P. M. (1999). Cutoff criteria for fit indexes in covariance structure analysis: conventional criteria versus new alternatives. Struct. Equ. Model. Multidiscip. J. 6, 1–55.
Iztek-Greulich, H., and Vollmer, C. (2017). Emotional and motivational outcomes of lab work in secondary intermediate track: the contribution of a science center outreach lab. J. Res. Sci. Teach. 54, 3–28. doi: 10.1002/tea.21334
Jang, H., Reeve, J., and Deci, E. L. (2010). Engaging students in learning activities: it is not autonomy support or structure but autonomy support and structure. J. Educ. Psychol. 102, 588–600. doi: 10.1037/a0019682
Jansen, M., Scherer, R., and Schroeders, U. (2015). Students’ self-concept and self-efficacy in the sciences: differential relations to antecedents and educational outcomes. Contemp. Educ. Psychol. 41, 13–24. doi: 10.1016/j.cedpsych.2014.11.002
Jones, M. G., Howe, A., and Rua, M. J. (2000). Gender differences in students’ experiences, interests and attitudes toward science and scientists. Sci. Educ. 84, 180–192.
Kıran, D., Sungur, S., and Yerdelen, S. (2019). Predicting sciende engagement with motivation and teacher characteristics: a multilvel investigation. Int. J. Sci. Math. Educ. 17, 67–88. doi: 10.1007/s10763-018-9882-2
Kline, R. B. (2016). Principles and practice of structural equation modeling. New York, NY: Guilford Publications.
Kryshko, O., Fleischer, J., Grunschel, C., and Leutner, D. (2022). Self-efficacy for motivational regulation and satisfaction with academic studies in STEM undergraduates: the mediating role of study motivation. Learn. Individ. Differ. 1, 96–102. doi: 10.1016/j.lindif.2021.102096
Kulakow, S. (2020). How autonomy support mediates the relationship between self-efficacy and approaches to learning. J. Educ. Res. 113, 13–25. doi: 10.1080/00220671.2019.1709402
Kwon, H., Vela, K., Williams, A. M., and Barroso, L. R. (2023). Mathematics and science self-efficacy and STEM careers: a path analysis. J. Math. Educ. 12, 66–81. doi: 10.26711/007577152790039
Lee, Y., Capraro, R. M., and Bicer, A. (2019). Affective mathematics engagement: a comparison of STEM PBL versus non-STEM PBL instruction. Can. J. Sci. Math. Technol. Educ. 19, 270–289. doi: 10.1007/s42330-019-00050-0
Li, Y., and Singh, C. (2021). Effect of gender, self-efficacy, and interest on perception of the learning environment and outcomes in calculus-based introductory physics courses. Phys. Rev. Phys. Educ. Res. 17, 010–143. doi: 10.1103/PhysRevPhysEducRes.17.010143
Lichtenberger, E., and George-Jackson, C. (2012). Predicting high school Student’s interest in majoring in a STEM field: insight into high school students postsecondary. J. Career Tech. Educ. 28, 19–38. doi: 10.21061/jcte.v28i1.571
Liou, P., Wang, C., John, J. L., and Areepattamannil, S. (2021). Assessing student’s motivational beliefs about learning science across grade level and gender. J. Exp. Educ. 89, 605–624. doi: 10.1080/00220973.2020.1721413
Liu, Y., Draper, J., and Dawson, M. (2023). The relationship between work experience and career expectations: career decision-making self-efficacy as mediator. J. Hosp. Tour. Educ. 35, 2013–2224. doi: 10.1080/10963758.2022.2034118
Lupión, T., Franco, A. J., and Girón, J. R. (2019). Predictores de vocación en ciencia y tecnología en jóvenes: estudio de casos sobre percepciones de alumnado de secundaria y la influencia de participar en experiencias educativas innovadoras. Revista Sobre Enseñanza y Divulgación de Las Ciencias 16, 1–21. doi: 10.25267/Rev_Eureka_ensen_divulg_cienc.2019.v16.i3.3102
MacKinnon, D. P., Fairchild, A. J., and Fritz, M. S. (2007). Mediation analysis. Annu. Rev. Psychol. 58, 593–614. doi: 10.1146/annurev.psych.58.110405.085542
Madjar, N., Weinstock, M., and Kaplan, A. (2017). Epistemic beliefs and achievement goal orientations: relations between constructs versus personal profiles. J. Educ. Res. 110, 32–49. doi: 10.1080/00220671.2015.1034353
Mai, M. Y., Yusuf, M., and Saleh, M. (2023). Motivation and engagement as a predictor of students science achievement satisfaction of Malaysian of secondary school students. Eur. J. Educ. 6, 96–107. doi: 10.2478/ejed-2023-0019
Meece, J. L., Parsons, J. E., Kaczala, C. M., and Goff, S. B. (1982). Sex differences in math achievement: toward a model of academic choice. Psychol. Bull. 91:324.
Mellado Jiménez, V., Borrachero, A. B., Brígido Mero, M., Melo, L. V., Dávila Acedo, M., Conde Núñez, M., et al. (2014). Las emociones en la enseñanza de las ciencias. Enseñanza de las Ciencias 32, 0011–0036. doi: 10.5565/rev/ensciencias.1478
Membiela, P., Acosta, K., Yebra, M. A., and González, A. (2023). Motivation to learn science, emotions in science classes, and engagement towards science studies in Chilean and Spanish compulsory secondary education students. Sci. Educ. 107, 939–963. doi: 10.1002/sce.21793
Murphy, S., MacDonald, A., and Wang, C. A. (2019). Towards and understanding of STEM engagement: a review of the literature on motivation and academic emotions. J. Sci. Math Teach. Educ. 19, 304–320. doi: 10.1007/s42330-019-00054-w
National Council of Humanities, Sciences and Technologies - CONAHCYT (2023). Primeros cambios, grandes mejoras : CONAHCYT Avanza.
National Institute of Statistics and Geography INEGI. (2020). Tendencias de Empleo Profesional - Tercer trimestre 2020. Empleabilidad En México. https://www.inegi.org.mx/temas/empleo/ (Accessed November 18, 2023).
Olivier, E., Archambault, I., De Clercq, M., and Galand, B. (2019). Student self-efficacy, classroom engagement, and academic achievement: comparing three theoretical frameworks. J. Youth Adoles. 48, 326–340. doi: 10.1007/s10964-018-0952-0
Oppermann, E., Brunner, M., and Anders, Y. (2019). The interplay between preschool teacher’s science self-efficacy beliefs, their teaching practices, and girl’s and boy’s early science motivation. Learn. Individ. Differ. 70, 86–99. doi: 10.1016/j.lindif.2019.01.006
Orendain, V. (2019). Mujeres STEM: Un reto educativo en México. Revista de Evaluación para Docentes y Directivos RED INEE. 5 (2). Instituto Nacional para la Evaluación de la Educación: México. Available at: https://historico.mejoredu.gob.mx/mujeres-stem-un-reto-educativo-en-mexico/
Organisation for Economic Co-operation and Development OECD (2018). Informe del Programa para la Evaluación Internacional de los Estudiantes PISA 2018 : OCDE.
Ozkal, N. (2019). Relationships between self-efficacy beliefs, engagement and academic performance in math lessons. Kıbrıslı Eğitim Bilimleri Dergisi 14, 190–200. doi: 10.18844/cjes.v14i2.3766
Pedrero, V., and Manzi, J. (2020). Self-beliefs, engagement and motivation in science and mathematics: are they universal? Int. J. Educ. Res. 101, 1–15. doi: 10.1016/j.ijer.2020.101562
Phillips, T. B., Ballard, H. L., Lewenstein, B. V., and Bonney, R. (2019). Engagement in sicence through citizen science: moving beyond data collection. Sci. Educ. 103, 665–690. doi: 10.1002/sce.21501
Reeve, J. (2012). “A self-determination theory perspective on student engagement” in Handbook of research on students engagement. Eds. S. Christenson, A. Reschly and C. Wylie (Boston, MA: Springer), 149–172.
Restubog, S. L. D., Florentino, A. R., and Garcia, P. R. J. M. (2010). The mediating roles of career self-efficacy and career decidedness in the relationship between contextual support and persistence. J. Vocat. Behav. 77, 186–195. doi: 10.1016/j.jvb.2010.06.005
Romero Bojórquez, L., Utrilla Quiroz, A., and Utrilla Quiroz, V. M. (2014). Las actitudes positivas y negativas de los estudiantes en el aprendizaje de las matemáticas, su impacto en la reprobación y la eficiencia terminal. Ra Ximhai 10, 291–319.
Rosenzweig, E. Q., and Wigfield, A. (2016). STEM motivation interventions for adolescents: a promising start, but further to go. Educ. Psychol. 51, 146–163. doi: 10.1080/00461520.2016.1154792
Sáinz, M., Fàbregues, S., Rodó-de-Zárate, M., Martínez-Cantos, J.-L., Arroyo, L., and Romano, M.-J. (2020). Gendered motivations to pursue male-dominated STEM careers among spanish young people: a qualitative study. J. Career Dev. 47, 408–423. doi: 10.1177/0894845318801101
Schunk, D. H. (2008). Metacognition, self-regulation, and self-regulated learning: research recommendations. Educ. Psychol. Rev. 20, 463–467. doi: 10.1007/s10648-008-9086-3
Schunk, D. H., and Mullen, C. A. (2012). “Self-efficacy as an engaged learner” in Handbook of research on student engagement. eds. S. Christenson, A. Reschly, and C. Wylie (Boston, MA: Springer).
Silva, W. F., Redondo, R. P., and Cardenas, M. J. (2018). Intrinsic motivation and its association with cognitive, actitudinal and previous knowledge processes in engineering students. Contemp. Eng. Sci. 11, 129–138. doi: 10.12988/ces.2018.79114
Simpkins, S. D., Price, C. D., and Garcia, K. (2015). Parental support and high school students' motivation in biology, chemistry, and physics: understanding differences among Latino and Caucasian boys and girls. J. Res. Sci. Teach. 52, 1386–1407. doi: 10.1002/tea.21246
Singh, K., and Abdullah, B. (2020). Influence of self-efficacy on student engagement of senior secondary school students. Ind. J. Pub. Health Res. Dev. 11:119. doi: 10.37506/v11/il/2020/ijphrd/193797
Singh, K., Granville, M., and Dika, S. (2002). Mathematics and science achievement: effects of motivation, interest, and academic engagement. J. Educ. Res. 95, 323–332. doi: 10.1080/00220670209596607
Skinner, E. A., Kindermann, T. A., and Furrer, C. J. (2009). A motivational perspective on engagement and disaffection: conceptualization and assessment of children’s behavioral and emotional participation in academic activities in the classroom. Educ. Psychol. Meas. 69, 493–525. doi: 10.1177/0013164408323233
Sökmen, Y. (2021). The role of self-efficacy in the relationship between the learning environment and student engagement. Educ. Stud. 47, 19–37. doi: 10.1080/03055698.2019.1665986
Song, Z., and Chon, K. (2012). General self-efficacy’s effect on career choice goals via vocational interests and person–job fit: a mediation model. Int. J. Hosp. Manag. 31:798. doi: 10.1016/j.ijhm.2011.09.016
Stankov, L., and Lee, J. (2014). Quest for the best non-cognitive predictor of academic achievement. Educ. Psychol. 34, 1–8. doi: 10.1080/01443410.2013.858908
Staribratov, I., and Babakova, L. (2019). Development and validation of a math-specific version of the academic motivation scale (AMS-mathematics) among first-year university students in Bulgaria. STEM J 8, 317–328. doi: 10.18421/TEM82-01
Stommel, M., Wang, S., Given, C. W., and Given, B. (1992). Focus on psychometrics confirmatory factor analysis (CFA) as a method to assess measurement equivalence. Res. Nurs. Health 15, 399–405
Tellhed, U., Bäckström, M., and Björklund, F. (2017). Will I fit in and do well? The importance of social belongingness and self-efficacy for explaining gender differences in interest in STEM and HEED majors. Sex Roles 77, 86–96. doi: 10.1007/s11199-016-0694-y
Tzu-Ling, H. (2019). Gender differences in high-school learning experiences, motivation, self-efficacy, and career aspirations among Taiwanese STEM college students. Int. J. Sci. Educ. 41, 1870–1884. doi: 10.1080/09500693.2019.1645963
Ugwuanyi, C. S., Okeke, C. I., and Ageda, T. A. (2020). Psychological predictors of physics learners’ achievement: the moderating influence of gender. Cypriot J. Educ. Sci. 15, 834–842. doi: 10.18844/cjes.v15i4.4635
Usher, E. L., Ford, C. J., Li, C. R., and Weidner, B. L. (2019). Sources of math and science self-efficacy in rural Appalachia: a convergent mixed methods study. Contemp. Educ. Psychol. 57, 32–53. doi: 10.1016/j.cedpsych.2018.10.003
Van Aalderen-Smeets, S. I., Walma van der Molen, J. H., and Xenidou-Dervou, I. (2019). Implicit STEM ability beliefs predict secondary school students’ STEM self-efficacy beliefs and their intention to opt for a STEM field career. J. Res. Sci. Teach. 56, 465–485. doi: 10.1002/tea.21506
Voica, C., Singer, F. M., and Stan, E. (2020). How are motivation and self-efficacy interacting in problem-solving and problem-posing? Educ. Stud. Math. 1, 487–517. doi: 10.1007/s10649-020-10005-0
Wang, M. T., and Degol, J. (2014). Staying engaged: knowledge and research needs in student engagement. Child Dev. Perspect. 8, 137–143. doi: 10.1111/cdep.12073
Wang, M.Te, Fredricks, J. A., Ye, F., Hofkens, T. L., and Linn, J. S. (2016). The math and science engagement scales: scale development, validation, and psychometric properties. Learn. Instr., 43, 16–36. doi: 10.1016/j.learninstruc.2016.01.008
Wang, C., and Liou, P. (2018). Patterns of motivational beliefs in the science learning of Total, high-, and low-achieving students: evidence of Taiwanese TIMSS 2011 data. Int. Ernational J. Sci. Math Educ. 16, 603–618. doi: 10.1007/s10763-017-9797-3
Wigfield, A., and Eccles, J. S. (2023). “The relevance of situated expectancy-value theory to understanding motivation and emotion in different contexts” in Motivation and emotion in learning and teaching across educational contexts (United Kingdom: Routledge), 3–18.
Wigfield, A., Klauda, S. L., and Cambria, J. (2011). Influences on the development of academic self-regulatory processes. In: Handbook Self-regulation Learn. Performance. Vol. 1. Eds. B. J. Zimmerman and D. H. Schunk (Routledge/Taylor and Francis Group). 33–48. Available at: https://psycnet.apa.org/record/2011-12365-003
Williams, A. J., Danovitch, J. H., and Mills, C. M. (2021). Exploring sources of individual differences in Children’s interest in science. Mind Brain Educ. 15, 67–76. doi: 10.1111/mbe.12263
Wood, R. (2019). Students’ motivation to engage with science learning activities through the lens of self-determination theory: results from a single-case school-based study. Eur. J. Math. Sci. Technol. Educ. 15, 1–22. doi: 10.29333/ejmste/106110
Yurt, E. (2022). Mathematics self-efficacy as a mediator between task value and math anxiety in secondary school students. Int. J. Curr. Instruct. 14, 1204–1221.
Zeldin, A. L., Britner, S. L., and Pajares, F. (2008). A comparative study of the self-efficacy beliefs of successful men and women in mathematics, science, and technology careers. J. Res. Sci. Teach. 45, 1036–1058. doi: 10.1002/tea.20195
Keywords: STEM education, self-efficacy, academic motivation, affective engagement, science and math, pre-college students, educational psychology
Citation: Valenzuela-Peñuñuri R, Tapia-Fonllem CO, Fraijo-Sing BS and Manríquez-Betanzos JC (2024) Academic motivation and affective engagement toward science and math: the mediating role of self-efficacy. Front. Educ. 9:1385848. doi: 10.3389/feduc.2024.1385848
Edited by:
Paitoon Pimdee, King Mongkut’s Institute of Technology Ladkrabang, ThailandReviewed by:
Mustafa Özgenel, Istanbul Sabahattin Zaim University, TürkiyeMaura Pilotti, Prince Mohammad bin Fahd University, Saudi Arabia
Copyright © 2024 Valenzuela-Peñuñuri, Tapia-Fonllem, Fraijo-Sing and Manríquez-Betanzos. This is an open-access article distributed under the terms of the Creative Commons Attribution License (CC BY). The use, distribution or reproduction in other forums is permitted, provided the original author(s) and the copyright owner(s) are credited and that the original publication in this journal is cited, in accordance with accepted academic practice. No use, distribution or reproduction is permitted which does not comply with these terms.
*Correspondence: César O. Tapia-Fonllem, Y2VzYXIudGFwaWFAdW5pc29uLm14