- Centre for Change and Complexity in Education, University of South Australia, Adelaide, SA, Australia
“Closing the loop” in Learning Analytics (LA) requires an ongoing design and research effort to ensure that the technological innovation emerging from LA addresses the actual, pragmatic problems of educators in everyday learning environments. An approach to doing so explored in this paper is to design LA as a part of the human systems of activity within an educational environment, as opposed to conceptualising LA as a stand-alone system offering judgement. In short, this paper offers a case-study of how LA can generate data representations that can provide the basis for expansive and deliberative decision-making within the learning community. The case-study provided makes use of Social Network Analysis (SNA) to monitor the changing patterns of decision making around teaching and learning in a very large Australian college over several years as that college embarked on an organised program of practitioner research. Examples of how the various SNA metrics can be translated into matters of pragmatic concern to the college, its leaders, teachers and students, are provided and discussed.
1 Introduction
In the call for this special collection of papers on the topic of Actionable Learning Analytics, we have been invited to think about how we might “close the loop” in the learning analytics cycle. In essence, the editors of the collection have suggested that in its extant work, the field of LA has done a good job of collecting and representing diverse learning data, but that a gap still remains in leveraging these representations to inform and improve learning. An appropriate purpose of LA generated feedback, they contend, would be to support processes of self-reflection and self-evaluation, ultimately helping to improve students’ Self-Regulation of Learning (SRL).
In offering this contribution to the collection, we heartily agree with the position proposed by the editors, and we seek to go further with the line of thinking they have started. Too often, we would suggest, the “real” challenges of teaching and learning are overlooked in favour of the challenges that are (relatively) easy to understand (Lupton and Hayes, 2021). “Closing the loop” in Learning Analytics (LA), we will therefore argue, requires an ongoing design and research effort to ensure that the technological innovation emerging from LA addresses the actual, pragmatic problems of educators in everyday learning environments.
The editors’ choice of SRL as a context for thinking about LA is a useful one for the argument we are making. SRL is a multi-faceted and, indeed, complex construct (Dever et al., 2023). It is influenced by what might be seen as “regular” educational interventions of the “student does activity and is changed” kind (Dunlosky et al., 2013; Lee et al., 2023). However, the extant research also shows that the development of SRL can—and should—be thought of as part of a more complex system. SRL is impacted, for example, by such things as teacher questioning technique (Wong et al., 2023), by school climate (De Smul et al., 2019a, 2019b) and teacher beliefs (Steinbach and Stoeger, 2016; Barr and Askell-Williams, 2019; Vosniadou et al., 2021). This body of research points to an opportunity and, indeed, a need for LA to expand its scope and to pay greater attention to learning systems as opposed to learning actions. If we are to improve SRL in a school, for example, we need to attend not only to the actions of the students, we also need to know about school climate, teacher beliefs, and how educational decisions are being made. LA can help make such aspects of a complex learning environment known.
1.1 What do we mean when we ask, “what works?”
Learning Analytics is defined as the “measurement, collection, analysis and reporting of data about learners and their contexts, for purposes of understanding and optimising learning and the environments in which it occurs” (Society for Learning Analytics Research (SoLAR), 2024). It should provide the tools to rapidly determine if what we are doing is “working”, and to guide our responses when we find that things are not working.
Like all innovation, however, LA should be understood in the context of its time (Williams and Edge, 1996), and it is important to recognise that LA has emerged at a time when dominant educational policy discourses have too strongly positioned education as synonymous with the work-skills development of the individual (Zipin et al., 2020). In doing so, what might be seen as “mainstream” educational systems have come to valorise a “reductive” (Wrigley, 2019) approach to the gathering of evidence that informs decision making in our schools and universities. In this context, LA has been wielded as a singular tool that tends to narrow rather than broaden the educational landscape conforming to the constraints imposed by the prevailing policy discourse focused on standardised best practices. In short, the context of the times has meant that LA has tended to focus on data related to individual learning actions and is not yet fully accounting for the conditions of learning (Gašević et al., 2014).
Our argument in this paper is that this reductionist understanding of the role of evidence in educational decision making is simply insufficient for purpose. If we are to “close the loop” and ensure that LA is actionable, then we need to rethink how and where LA is used and perceived within educational decision-making systems. The purpose of this paper is to contribute to such a rethink, which we will do via a case study. In presenting this case study we seek to demonstrate an application of analytics in providing novel insights into an evolving learning environment, rather than merely serving as a tool for identifying the implementation of a pre-defined “best practice” in a static environment. It is intended to be an example of how LA can provide a basis for expansive, deliberative and pragmatic decision-making within a learning community as its members act within an uncertain world (Callon et al., 2009).
The chief reason we believe that the reductive policy discourse is an insufficient framework for the development of effective LA is that it—the policy discourse—is derived from neoliberal economic theory (Hall, 2016; Prinsloo and Slade, 2016; Littlejohn and Hood, 2018). It tends to forget about, or even actively ignore, what we know about education as a complex and social system. This argument has been made by many others within the critical analysis of education policy, although none more accessible to an in-expert readership than Lupton and Hayes (2021). In their excellent book they set out the history, and identify the consequences, of the shift to what we are labelling “reductionism”, but which also goes under more attractive names such as “what works” or “evidence-based education”. The arguments for a more expansive vision, though, have come from leading scholars in LA too (see, for example, Gašević et al., 2014; Wiley et al., 2023).
The evidence base being referred to in this “what works” policy discourse is almost exclusively about the identification of successful and unsuccessful practices through randomised control trials (RCTs) of quasi-experimental studies and the calculation of effect sizes based on the synthesis of these studies through meta-analysis. This kind of research is essential to improving what we do in our educational work, and we applaud the extensive and detailed work of those who undertake it. However, relying on any one kind of evidence in human decision making has consequences.
One major consequence of this reductive approach is that when it is consumed by stakeholders who lack expertise on research methodology—and this includes most policy makers, teachers, students and parents—the apparent “objectivity” of the meta-analytic technique can lead to this evidence being widely misunderstood and vastly over-valued (Olejnik and Algina, 2000). The approach generally relies on testing, which is a very particular and by no means neutral approach to assessing student learning. It also erroneously assumes that all tests are equal. Clear, simple conclusions derived from controlled testing can mask other factors that contribute to learning outcomes, causing an uninformed decision maker to inaccurately assume the whole school system mirrors the controlled test classroom environment. It is not widely understood, for example, that we tend to see far greater effect sizes being generated by studies making use of bespoke tests targeting a specific construct (say, phonics) than we do from studies testing more holistic function (say, reading) (Simpson, 2017). A key point we wish to make here when thinking about the actionability of LA is that we must not repeat this mistake of appearing to provide unwarranted truth claims. Myriad factors influence the how, what and why of learning, and we need to ensure that a narrow selection of LAs does not drive undue attention to some elements, while obscuring the importance of others.
Another consequence of the hegemonic position of reductive evidence-use in educational decision making is the way that it structures educational problems. In this construction, educational improvement comes through intervention at the individual and “classroom” level rather than systemic or structural intervention; and learning is a linear developmental process with an essentially known destination. This construction of the nature of educational problems, we would contend, is limiting the capacity of LA to close the loop and to fulfil its long-held promise of improving education in everyday contexts. In contrast, the case study provided in this paper begins with an expansive understanding of education (Engeström, 2016).
1.2 Expansive learning
The reductive understanding of educational evidence and decision making that has dominated educational policy and subsequent work in LA in recent decades stands in stark contrast to the more expansive understandings that are available elsewhere in educational scholarship. For example, the reductive approach assumes that the subject will acquire some identifiable and pre-determined knowledge or skill, and that in doing so a long-lasting and observable change in behaviour will occur. Learning is understood as an isolated transaction. Implicit in this understanding is that the knowledge or skill to be acquired is reasonably stable, and that there will be a competent and expert teacher available who can define what is to be learned, and judge that it has been learned. The danger of this approach, Engeström argued, is that the “[i]nner contradictions, self-movement, and agency from below are all but excluded. It is a paternalistic conception of learning that assumes a fixed, Olympian point of view high above, where the truth is plain to see” (Engeström, 2000, p. 530).
An expansive approach, on the other hand, contends that—once we get past some basic skills—people, organisations and societies are all the time learning and needing to learn things that are actually not very well defined. Or, as Engeström (2016) puts it, we have a need to learn what is not yet there. When we consider the underlying goals of education as the development of life-long learners able to resolve the many tensions of the modern world, we find the need to teach capabilities like SRL, as the editors of this collection suggest. Also, we would argue, we need to respond to learning needs that come “from below”, and in parallel to the creation of new kinds of activity. In this expansive vision of education, learning should be transformative rather than conformative.
Research in the expansive tradition has tended to focus on the transformation of artefact-mediated cultural activity. It holds that the individual cannot be understood in isolation from his or her cultural and historical context, and neither can a community be understood without the agency of individuals who use and produce artefacts, be they concrete or conceptual (Roth, 2016). Viewed from this expansive perspective, LA takes on different purpose. Rather than providing information about isolated educational “transactions”, the expansive perspective suggests a role for LA in both understanding and hence pragmatically adapting complex systems of learning.
Figure 1 illustrates the kinds of learning system that LA can help to optimise. Based on third generation Activity Theory (Sannino et al., 2016), this model provides a framework for looking more deeply at the kinds of complex educational activity needed to improve SRL. It allows that as a learner (actor) seeks to transform a skill, understanding or disposition (object), they do so in the context of the tools available, the rules and traditions of their context, and in a social environment where the community engaged in the learning activity influence both what is learned and how learning occurs. In short, it is a framework for learning in context. This model also allows for a theory-based articulation of both immediate and longer-term goals of a learning design. When understood in a deeply contextual way, the opportunities for LA to be used to optimise the system expand rapidly.
1.3 Expansive learning and SRL
The short version of the approach we promote in this paper is that every arrow in Figure 1 is a LA opportunity, but we will elaborate with respect to SRL. Research on SRL is new and is continually generating new approaches for teaching and learning practice. It calls on teachers to adapt their practice, to implement new things. Using the traditional or “reductive” approach to evaluating such change, we would focus on the top part of the triangle in Figure 1. That is, we would effectively ask how the primary actor—normally the student—has made use of the new “tool”, and the extent to which that transformed their SRL and we would look for a relevant LA signal. The difficulty with this approach within the context of everyday schooling is that the new objective may be in tension with other the aspects of the teaching and learning activity system outlined in the figure. The teacher considering the use of a new pro-SRL pedagogy, for example, may see it as being in tension with an existing rule or tradition of practice relating to, say, next week’s mathematics test and the need to improve domain specific context knowledge. Or, by asking students to take a greater role in the regulation of their own learning behaviours, it may be in tension with the traditional “divisions of (learning) labour”. It may, for example, ask that teachers also learn and implement new behaviour management strategies.
With an expansive outlook, the core questions on what is “working” move from “is this pedagogical approach working?”, towards “how is this pedagogy working within this context?” And it is here that we think that we may find a way for LA to “close the loop”. In the example explored in this paper, our interest is in how an analytics approach can assist us to understand changes in the decision-making processes within a school that are occurring as a result of a wider implementation project focussed on improving SRL. Within that project we do, of course, have more direct measures of changes in constructs more directly related to SRL. Early in the project, however, we found that those measures were of limited utility as the most pressing issue was simply getting the teachers to adopt the pedagogical strategies the project promoted at all, and then to do so in ways that were appropriate for their own classroom. That is, we found that analytics concerned with the arrow from “primary actor” to “object of transformation” was necessary, but not sufficient.
A real challenge for managing learning as a complex system rather than a simple transaction is that the systems are never likely to reach an ideal state. New tools are constantly introduced to our learning systems, the object of learning shifts over time as both knowledge and policy change, people come and go from our communities of practice, and so on. This evolutionary nature of learning activity also presents an ongoing challenge for the designers of LA. That is, just as expansive learning asks us to design learning for what is not yet there, it asks LA to help optimise learning within an emergent system whose ideal state is also not quite known.
This paper continues in the expansive tradition and makes novel use of social network analysis as an example of the kind of LA that can provide the members of an educational community with artefacts through which to mediate their activity. As such, this paper should not be read for the network analyses provided in the results section, per se. Rather, it should be read as an example of how LA can be used to raise the kinds of real and important questions-as-conceptual-artefacts that can be used to improve learning by guiding the pragmatic and adaptive leadership of a learning system. Our purpose here is not to improve on the kinds of LA that exist now. Rather, we are seeking to explore different ways of using LA to improve learning, particularly through taking greater account of the external context of learning.
With the illustrative purpose of the paper in mind, the reader is invited to note that through sections 2, 3 and 4—the methods, results and discussion sections, respectively,—we will set out an analysis of educational decision-making structures in a very large Australian college organised into five sub-schools and catering to students ranging from pre-school to Year 12. The point of interest in this study was the emergence of an informal leadership and decision-making network that formed around an initiative of the college to develop an internal “research and inquiry” institute in partnership with a local university. As such, it provides important information about changes to the community of practice and the divisions of labour within the learning activities of the college, and so can be used as part of a process of pragmatically, adaptively, and iteratively optimising learning at the college.
2 Materials and methods
Our purpose in this paper is to illustrate how LA might “look” and “feel” when we use it to generate questions rather than provide judgements. As we have noted, we imagine the questions we are generating as prompts for deliberation in a manner similar to the hybrid forums as prompted by Callon et al. (2009), or for use in ongoing processes developmental evaluation (Leonard et al., 2017). With this purpose in mind, we will now describe the context for the LA implementation and briefly describe the method used in the generation of the data representations. The process used in this case was quite labour intensive but many aspects of it—including the generation of deliberative questions we undertake in the following “discussion” section of this paper—could be more fully automated.
2.1 A different kind of game
When considering the ambition of the work here, it can be instructive to consider the difference between simple and complex games. Traditionally, games had predefined “win conditions” where, if these specific conditions were met in a linear fashion, the player completes the game. One is obliged to conform to the conditions of the game to succeed, and few other approaches to the rules of the game will be successful (Devolder et al., 2012). In games where the win conditions favour points and badges, learner motivation is almost entirely extrinsic (Abramovich et al., 2013).
However, this is a narrow view of achievement that only applies to simple game systems. Instead, more complex systems will allow the player to generate their own pathways to success or, within a more expansive game system, create their own conditions of success alongside the game’s predefined goals (Devolder et al., 2012). Instead of entering the game expecting just one linear path to the “win state”, players choose how they solve each challenge and continuously evaluate their approach to the challenge in non-linear way (Schrader and McCreery, 2012). When they are unsuccessful at a task, they try again using a different approach, continuing to refine or completely disregard their approach as they understand what their previous barriers to success were.
In complex games, creativity and agency are encouraged, so the approaches may be vastly different between players (Nguyen, 2020a). For example, if the goal is to enter a castle, one player may approach the front door, another may parachute from the sky, and a third player may choose to climb through a window. There is no best practise approach to entering the castle, but all three approaches are “what works” for that specific player due to their previous progress in the game, their skills and abilities, and their personalities (Devolder et al., 2012; Krath et al., 2021). The learning and decision making is in the hands of the player, not the game itself.
Here, there is little scaffolded process, so the player must consider the entire game—and their own agency within and outside of the game—at a holistic level before deciding which steps to take in what order (Nguyen, 2020b; Dever and Azevedo, 2022). Essentially, the self-regulation of learning (SRL) is implicit in the games design, so the player scaffolds how they approach the game instead of the other way around (Zap and Code, 2009). The player must learn the mechanics and then utilise them in a way that meets their decided goal—a more transformative experience than traditional games (Devolder et al., 2012). The game never changes, but new pathways to success will emerge as the player’s choices and goals change. The engagement then comes from the questioning of the game’s environment instead of the defined answer or win state.
SRL follows a similar format to complex games—the learner scaffolds their own learning instead of following a specific, conformative way of doing (Nietfeld, 2017; Xian, 2021). Like in a game, a strong self-regulated learner is better able to adapt to unexpected and new challenges, provided that their learning environment allows them to be emotionally safe to follow “what works” for them (Zap and Code, 2009).
LA can benefit from this perspective. Instead of using LA to understand how to fit learning into a specific model or why a predetermined goal has not been met, the complex data can be used to see how new goals and ways of doing emerge within the context and rules of a specific environment. Here, LA should facilitate asking “what scaffold has emerged?” not “how does this deviate from the ‘best practise’ scaffold?”.
2.2 Social network analysis
The LA used in this study is Social Network Analysis. The study we present provides an analytic representation of data with respect to the networks for educational decision making among the teaching workforce of a large Australian independent college serving Early Years – Year 12 students that we refer to Corroboree Frog College. The College is organised across five campuses of sub-schools, four working with students from Early Years to Year 10, and one catering to students in the final two years of secondary education.
The impetus for establishing SNA as an ongoing “analytic” for monitoring the operation of College was the establishment of an internal research institute (The Institute) within the College. The Institute was resourced through the part-time appointment of a Director of Research and a Research Associate (the first author of this paper), both teachers from the school who, with support from a local university, were to support other teachers wishing to engage in practitioner research to improve their practice or the practices of the College. An early focus for the work of The Institute was to improve SRL practices across the College.
The importance of organisational networks as foundational constituents that profoundly shape operational efficiency, decision-making quality, and resource allocation within the activity of a learning community is well-documented in the literature (Cross et al., 2002; Borgatti and Foster, 2003). This becomes particularly salient in educational contexts where the yardstick for organisational effectiveness primarily hinges on outcomes in teaching and learning (Daly, 2010; Hargreaves and Fullan, 2012). The decision to establish an ongoing analytic through SNA was made to better understand the role of The Institute as an informal decision-making network within the College, and to compare its operation to the College’s existing formal teaching and learning decision making structure. The aim was and is to provide actionable knowledge geared towards refining the educational environment in alignment with the institution’s short-term and long-term objectives of improving educational and broader life outcomes for its students, such as through improving student SRL (Tsai, 2002; Cross and Parker, 2004).
The overall approach taken in this case was based on De Laat et al. (2007) method of combining SNA with content analysis (CA) and critical event recall (CER) to better understand networked learning communities and understand value creation. Data for the ongoing analysis was gathered through monthly surveys taken by teachers associated with The Institute. The participants were asked with whom they had interacted in relation to practitioner research within the previous month, and the nature of that interaction. The survey data was supplemented by field notes taken at group meetings by the lead author.
For the SNA component of the study, the data is coded into an adjacency matrix. An adjacency matrix is a square matrix used to represent a finite graph. The elements of the matrix indicate whether pairs of vertices are adjacent or not in the graph. In the context of this study, the adjacency matrix represented the interactions between participants in The Institute, and beyond (Yang et al., 2017). The adjacency matrix is then run through the Gephi software where the network metrics are calculated, and the networks are visualized (Bastian et al., 2009). This analysis, reported below, provides an array of network metrics for evaluation including average degree, modularity, and clustering coefficient (Latapy, 2008) to provide a nuanced, multi-dimensional portrayal of two different decision-making networks within Corroboree Frog College.
The pragmatic use of each of these metrics is discussed in detail in section 4 of this paper, and more extensively in the Supplementary Figure>Supplementary material. Broadly though, these metrics provide a way to investigate each network’s operational efficiency, susceptibility to structural flaws, and agility in adapting to change (Watts and Strogatz, 1998; Barabási and Albert, 1999; Newman, 2010). Within the College context, the quest for academic excellence is inherently tied to a broader set of objectives, including social, emotional, and developmental facets (Eccles and Roeser, 2011; Roffey, 2012). This underscores the significance of dissecting the formal network structures that either act as facilitators or impediments to effective teaching and learning (Balkundi and Harrison, 2006; Scott, 2017). Metrics such as average degree, modularity, and clustering coefficient are pivotal not merely for deciphering how information and resources traverse the network, but also for understanding the collective organisational capacity for innovation, adaptability, and resilience (Barabási, 2002; Daly, 2010).
The ensuing analysis is committed to unravelling the ramifications of these network characteristics for teaching and learning. The objective is to generate an empirically grounded platform for pragmatic and adaptive professional investigations into what scaffolds have emerged within the “game”.
2.3 Context for the “formal” network
The first network under consideration in this paper is the Formal Network. It represents the foundational mechanism for making teaching and learning decisions within the College, and its membership is defined ex officio. That is, the actors are a part of this network because their job description allows and requires it. In this structure, distinct roles and hierarchies emerge. Notably, for each of the five schools (soon to be six) within the institution, there is a dedicated Head of Teaching and Learning for both the Junior School (JS) and the Middle School (MS). Additionally, each school has a Head of Junior School and a Head of Middle School. These heads come together in specific networks: while the Heads of Teaching and Learning (JS) and the Heads of Junior School convene through the Junior School Formal network, their counterparts for the Middle School convene through the Middle School Formal network. These gatherings typically span between 60 to 120 min, once per term.
2.4 Context for the “informal” network
From the establishment of the initiative that would become The Institute in 2017 through to mid-2020, the teachers who engaged in the initiative did so directly with the Director of Research. In 2020, however, The Institute and the partner university established a cohort-based doctoral research “incubator” program. Essentially, a reading group would gather weekly to discuss their reading, thinking and the development of educational research. The hope was that this would lead to a cohort of staff who would undertake a Doctor of Philosophy program together at a future time. In that year, 9 teachers from three of the College’s sub-schools, along with a varying number of staff with College-wide leadership roles engaged in the program.
From the outset it was clear that members of this group had some very evident opinions about the future of education and could easily express some major problems in their practice. They met every Thursday after school in the College Library. The meetings were led by the College Director of Research and the Institute Research Associate. The group quickly took charge of their own learning. The teachers came from different areas: English, Junior School, Middle School subjects like Geography, PE, Languages, Digital Technologies, and Science, as well as Learning Support and Chaplaincy. As part of their collaboration, they presented their findings at the College’s first research showcase. Here, they shared their research with senior leaders and other teachers. The Heads of the College publicly praised the members of this group, calling them “trailblazers”. Everyone who engaged with the group completed a Professional Certificate offered by the local university, and four have subsequently enrolled in a doctoral research program.
By 2021, a further 11 participants from four of the College’s sub-schools joined the Informal group. The members included a history teacher, Head of Junior School, a junior (primary or elementary) school teacher, a middle (Years 7–10) school teacher, Junior School Head of Pastoral Care, and the college Director of Innovation and Creativity. The Director of Research and Institute Research Associate intentionally took a backseat, hoping this group would take the initiative and self-organise, much like the 2020 group. However, only 5 members finished the program and contributed to the showcase.
Interestingly, instead of a live presentation, they opted for a pre-recorded video. This choice stemmed from the participants’ expressed anxiety about the live presentation, and the decision to pre-record aimed to alleviate this stress. However, feedback from these participants later revealed they felt less appreciated than the 2020 group since they did not present in person. As for the members who did not finish, some reported that they felt unsupported by their school leaders, felt a sense of exclusion and gatekeeping from those who completed the course, and did not expect the course to be so theory-based. The group met every Thursday after school in the Library, with the core group of 5 consistently attending. Three of the 5 who completed were staff members within the same junior school section of the same sub-school. The ongoing stress of the COVID pandemic was also notable in 2021.
In 2022, a group of 23 individuals from across all Corrobboree Frog sub-schools began with an aim to pursue professional learning, considering the Professional Certificate that sat at the heart of the “incubator” program a promising route. Their aspiration spanned achieving a professional certificate to even higher degrees by research. However, the interactions within this cohort demanded substantial scaffolding, indicating their initial dependence on structured guidance. As the meetings progressed, there was a noticeable drop in attendance. From this large group, a dedicated sub-group of three emerged, all keen on obtaining a professional certificate. This trio successfully self-organised and collaborated on action research, targeting specific challenges in their practice. Of those hoping to achieve a higher degree by research, three have remained committed. However, they continue to need substantial guidance, particularly in their meetings, and are yet to achieve the self-organisation seen in previous groups. These meetings are scheduled for Thursdays post-school hours in the Library.
3 Results
This study uses SNA to compare the Formal decision-making network at Corroboree Frog College with the Informal network that developed around the College Research Institute initiative. Table 1 summarises relevant network metrics firstly for the Formal decision-making network in 2022. This network had remained stable for several years, so we have only reported the analysis of this network from the most recent year of data available. Table 1 also summarises the relevant network metrics for the evolving Informal network. As this network was changing rapidly, an annual snapshot of this network has been provided for 2020, 2021 and 2022.
To assist with the interpretation of these metrics, “graphs” or visualisations for each network are provided in Figures 2–5. These graphs are visualisations intended to assist with the interpretation of the calculated metrics provided in the tables. They do not provide new information. In these graphs, the “nodes” or dots represent an individual within the decision-making network. The position of each node is meaningful, with those located towards the centre of the map being more integral to the network’s interactions. That is, they engage more frequently with others or hold pivotal roles in communication. Conversely, dots on the periphery indicate individuals who are less central, engaging less frequently or with fewer members. The “edges” or lines connecting the dots signify interactions. The visual weight of these lines gives us clues about the nature of these interactions. Thick lines denote a higher volume of communication between nodes, suggesting a stronger or more active relationship, while thin lines suggest occasional or less frequent interactions.
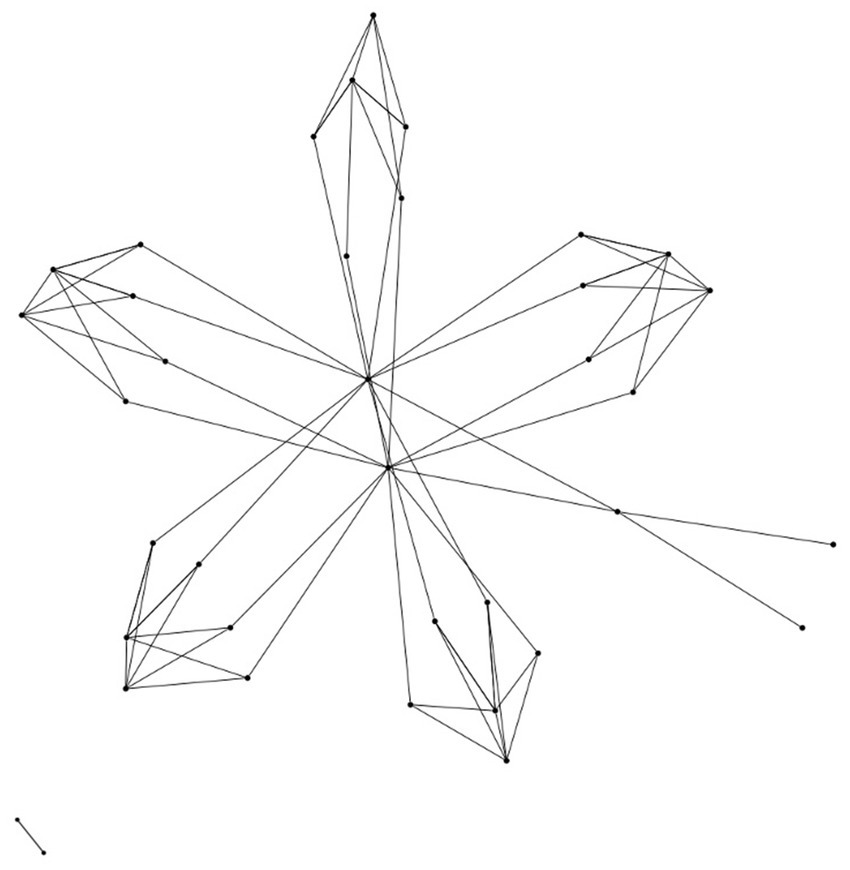
Figure 2. Formal network (including two connected nodes within the formal teaching and learning network with no interactions with the wider network).
Notable in the first of these graphs in Figure 2, is the hierarchical nature of the network. This is what the network with a relatively low Average Degree and high Modularity “looks” like. Each “spoke” in this graph represents people connecting within their own schools—the exception being the spoke to the bottom right which shows the College’s senior leadership. The central hubs in this network are the Junior and Senior Heads of learning. Towards the bottom left side of the Figure, the graph also shows two key members of the network are connected to each other, but are otherwise isolated from the network.
Table 2 provides a summary of key similarities and differences between the formal network and the different snapshots of the evolving informal network. These similarities and differences form the basis for the discussion in the following section.
The following graphs are useful for interpreting these findings. In Figure 3, the informal network shared a hub-and-spoke shape in 2020, the Director of the Institute is central to all activity.
The graphs in Figures 4, 5, in contrast, show that the informal network was tending towards complexity in 2021 and 2022, with what is known as a “small world” structure emerging, although more so in 2021. In this figure, which is the largest network shown in this paper, we see a network that, like the formal structure, has 5 sub-communities forming. Unlike the formal network, however, this network has low modularity with many points of cross-connection between the sub-communities. This kind of visualisation has proven an effective way to present this kind of analytic to school leadership than the table of metrics as it provides a greater feel for what is going on across the learning environment.
Notably, the “small world” nature of the network had begun to break down in 2022. The network is larger, but the centrality of the Director was once again more prominent. If the greater level of peer networking was desirable, then it was being lost and action would be needed to stabilise the more complex network structure. We will return to a possible method of stabilisation in the following section.
4 Discussion
4.1 Beyond the LA dashboard: optimising for learning
When we are thinking about closing the loop in LA, it is important to remember that the purpose is to optimise learning, not to optimise the metric. We’ve been reminded of this as colleagues reviewing early drafts of this paper have asked what is a “good” number for each of the SNA metrics. The argument we are making in this paper, though, is that such an “Olympian point of view” (Engeström, 2000) is not always desirable when it comes to optimising learning. Rather, a more powerful role for LA may lie in how we use the representations it provides to open up our thinking about learning context “from below”.
Returning briefly to Figure 1 and the arguments we began in the introduction, our position is that if our project and our school’s strategic intent is to improve SRL, then measures focussed strictly upon students’ SRL are insufficient. In everyday schooling, the implementation of any change will create tensions within the teaching and learning activity system, and it follows that a more expanded and expansive understanding of the dynamic system is required. As such, and to be clear, the use of the metrics from SNA we outline in this section are not intended to measure SRL directly, but rather to show how we are better understanding the context of learning as we seek to optimise that context with respect to SRL.
In this section we provide an illustration of what we have found to be an important step in “closing the loop” when using LA to understand the complexities surrounding our project. Specifically, we will provide concrete examples of how the different metrics of SNA have led to the generation of questions that can act as epistemic objects (Miettinen and Virkkunen, 2005 Fowler et al., 2022) or the basis for legitimate inquiry by the community of practice within the College. That is, as a basis for internal inquiry seeking to understand what makes the College innovation ready and able to do teaching and learning in new ways that result in improved SRL. The approach that we are promoting here is to use LA to support human decision making about the learning context, rather than as a substitute for that decision making. This is especially important in the context of ongoing advances in machine learning/artificial intelligence. With this technological power, LA is increasingly able to provide cheap although often impenetrable statistical predication (Bankins et al., 2022). What it cannot do, however, is provide the judgement on what predications matter or have value (Goldfarb and Lindsay, 2022). Human judgement is needed for that.
To ensure clarity and coherence in our analysis, we’ll begin by examining key Social Network Analysis (SNA) metrics that shed light on the intricate dynamics within the College’s teaching and learning networks. This discussion will focus on a select few metrics that are especially telling of the current situation, with a more comprehensive exploration of additional metrics available in the Supplementary Figure>Supplementary material. For each chosen metric, we will offer a brief overview grounded in existing literature, detailing its significance and the insights it provides. This will be followed by an analysis of the data specific to the College, expanding upon the preliminary findings outlined in Table 2. From there, we will explore a series of deliberative questions prompted by our analysis. These questions are intended to guide the College’s community of practice and leadership in reflecting on potential avenues for enhancing pedagogical effectiveness and innovation. They are a tool for closing the loop from LA to action.
However, the assessment of connectivity through metrics such as degree centrality is not merely a matter of numerical values but involves a nuanced understanding of the context and dynamics within which the network operates. Crossley (2015), for example, emphasises the importance of context in interpreting network metrics, arguing that what constitutes a “high” or “low” degree of connectivity can only be understood in relation to the size, potential for connections, and the specific objectives of the network. This perspective is critical in educational settings where the goals and scales of networks vary widely, from small professional learning communities to expansive research collaborations across institutions.
Moreover, the application of SNA in educational contexts underscores the importance of purpose and function in defining the value of network connectivity. For instance, (Prell, 2012) highlights how networks designed for intensive collaboration, such as professional learning communities within schools, may require a higher average degree to facilitate effective communication and resource sharing. On the other hand, networks that prioritise diversity of thought, such as interdisciplinary research networks, might benefit from a lower degree of connectivity, thereby encouraging independence and unique contributions from participants.
The dynamic nature of social networks further complicates the assessment of connectivity. Burt (2000) notes that networks evolve over time as new connections are made and existing ones are dissolved, affecting the overall structure and implications of connectivity within the network. This evolution necessitates a comparative approach to understanding high or low degrees of connectivity, where current assessments are contextualised against the historical development and future potential of the network.
The varied impacts of connectivity on network outcomes also demand a nuanced evaluation. Kadushin (2012) argues that while a high degree of connectivity may accelerate the dissemination of information and foster cohesion, it can also lead to challenges such as groupthink or the dilution of individual contributions. Conversely, a lower degree of connectivity might signal isolation or, alternatively, serve as a basis for innovation and the introduction of diverse perspectives.
In short, the assessment of high or low degrees of connectivity within social networks, especially in educational settings, requires a comprehensive understanding that goes beyond simple numerical analysis. It involves considering the specific context, goals, dynamic nature, and potential impacts of network structures on desired outcomes. This comparative approach ensures that evaluations of connectivity are both meaningful and relevant, providing insights that support the strategic development and management of networks to achieve their intended purposes.
4.2 Limited connectivity, sparsity, and fragmentation and implications for teaching and learning in a college setting
The interaction between the formal teaching and learning network of the college, characterised by a limited average connectivity degree of 2.132, and its informal counterparts, suggests significant implications for pedagogical effectiveness and innovation. Scott (2017) highlights that such limited connectivity, while potentially simplifying administrative processes, adversely affects the effectiveness of teaching and learning by creating decision-making bottlenecks and hampering the dissemination of essential resources and innovative teaching methodologies (Tsai, 2002; Borgatti and Foster, 2003). The formal network’s structure, marked by its constrained connectivity, tends to restrict cross-disciplinary collaboration and limits the dynamic exchange of knowledge and best practices, crucial for achieving educational excellence (Kogut and Zander, 1996; Daly, 2010; Eccles and Roeser, 2011).
On the other hand, informal networks, with their slightly higher degrees of connectivity in 2020 (2.3) and significantly more so in 2021 (2.688), suggest a greater potential for resource and knowledge sharing, thereby promoting innovative educational practices. However, the 2022 informal network, with an average degree similar to the formal network’s, indicates a level of connectivity that could potentially mirror the formal network’s challenges in pedagogical advancement and resource distribution.
The analysis reveals a concerning sparsity and fragmentation within the College’s teaching and learning structures, with a low graph density of 0.059 and the presence of separate connected components signifying a fragmented network (Newman, 2010). This fragmentation impedes efficient information sharing and collaboration, leading to disparities in educational quality and outcomes across different schools or faculties within the institution. Moreover, the network’s fragmented nature complicates collective decision-making and the construction of a unified educational vision, potentially resulting in choices that disproportionately benefit specific clusters at the expense of the broader community.
Comparatively, the 2020 informal network’s significantly higher graph density (0.277) and singular connected component highlight a more interconnected and cohesive structure, whereas the subsequent years show a trend towards increased fragmentation, particularly noted in the 2022 informal network’s multitude of strongly connected components (37), suggesting a network fragmented into many small groups.
These observations lead to several deliberative questions to be used in considering how to optimise the College context for promoting the development of SRL:
• How might the formal network integrate the higher connectivity seen in the 2021 informal network to enhance the flow of information, resources, and pedagogical innovations?
• Given the comparable levels of connectivity between the 2022 informal and formal networks, what structural insights from the informal network could inform improvements in decision-making and cross-disciplinary collaboration within the formal network?
• In light of the network’s low density and fragmented nature, what strategies from the more cohesive 2020 informal network could be adopted to enhance connectivity and unity within the formal network, thereby addressing the challenges posed by fragmentation and improving the overall educational environment?
4.3 Localised leadership teams, modularity, silo effects and implications for teaching and learning in a college setting
The integration of localised leadership teams and the impact of modularity and silo effects in college teaching and learning structures presents a nuanced challenge for balancing innovation with cohesive educational objectives. The network’s modularity score of 0.557, indicating distinct clusters within the College’s educational framework (Girvan and Newman, 2002), fosters innovation and effective problem-solving within specific clusters but also contributes to the formation of “silos.” These silos can limit cross-cluster resource sharing and collaborative learning, potentially inhibiting the spread of innovative practices across the College (Krackhardt and Hanson, 1993; Page, 2008; Borgatti et al., 2009).
On the one hand, localised leadership teams, guided by principles of adaptive leadership, can drive agile responses to local challenges and promote innovation in teaching practices (Heifetz, 1994; Gronn, 2009). This structure aligns with the college’s aim for ground-up organisational change. However, the risk of forming silos within these agile, locally focused units may restrict the sharing and scaling of effective methodologies, resources, or curricula, thereby challenging the integration of these units into the College’s broader educational ecosystem (Tushman and O’Reilly, 1996; O’Leary et al., 2011).
The informal network analyses from 2020 to 2022 illustrate a dynamic shift from a unified approach to a more modular one, with 2020 showing a lower modularity (0.213) and 2022 displaying a higher modularity (0.417). This transition suggests a movement towards more localised, autonomous clusters, posing both benefits and challenges for the college’s educational strategies.
In navigating these complexities, it is crucial to foster a balance between local autonomy and organisational cohesion. Regular consultations across different teams and cultivating an organisational culture that prioritises collaboration while maintaining autonomy can help integrate localised strengths into a cohesive strategy for the broader teaching and learning goals of the college.
These observations lead to several deliberative questions to be used in considering how to optimise the College context for promoting the development of SRL:
• How can the college effectively balance the benefits of high modularity and innovation within clusters with the imperative for cross-cluster collaboration and resource sharing?
• What strategies can be employed to ensure effective collaboration across localised leadership teams while maintaining the strengths of local autonomy, especially in light of the balanced approach observed in the 2021 informal network?
• Given the trend towards higher modularity and the formation of autonomous clusters as seen in the 2022 informal network, how can the College facilitate broader collaboration without compromising the innovative potential of local autonomy?
• Considering the risks of silo formation and inconsistent educational experiences due to misalignment with the College’s broader educational philosophy, what initiatives can be introduced to enhance cross-cluster knowledge sharing and ensure a consistent educational approach across different units?
4.4 Small world phenomenon, epistemic bubbles, echo chambers, and implications for teaching and learning in a college setting
The exploration of the network’s small-world characteristics, as detailed by Watts and Strogatz (1998), alongside the modularity and fragmentation aspects highlighted by Newman and Girvan (2004), provides a nuanced understanding of the information flow within the college. These characteristics suggest a network that is agile and capable of rapid information dissemination, which is crucial for a responsive teaching environment that aligns with student needs (Sawyer, 2005). However, the presence of modularity and separate connected components within this network structure also indicates potential challenges in the widespread dissemination of innovative practices and resources across the College (Granovetter, 1973; Daly, 2010), which could lead to the emergence of epistemic bubbles and echo chambers (Jamieson and Cappella, 2010).
This modularity not only affects the flow of information but also raises concerns regarding the alignment of teaching practices with the educational goals of the College (Coburn, 2001). The resultant epistemic bubbles and echo chambers, fueled by the network’s structure, can severely limit pedagogical diversity and innovation (Sunstein, 2017; Nguyen, 2020a) potentially resulting in faculties or schools becoming insular and resistant to new pedagogical approaches. This insularity can have a detrimental impact on the equitable distribution of innovative teaching methods across the student body, thereby hampering efforts towards transformational teacher professionalism and organisational change (Fullan, 2007; Daly, 2010).
In addressing these challenges, strategies such as deploying “boundary spanners” could be instrumental. These individuals or teams could facilitate information flow between different network modules or components, ensuring that innovative practices are evenly disseminated, thus enhancing the teaching and learning experiences across the college (Cross and Parker, 2004). Additionally, the informal network analyses from 2020 to 2022 offer insights into the fluctuating dynamics of information flow and modularity, indicating varying degrees of susceptibility to the formation of epistemic bubbles and echo chambers.
These observations lead to several deliberative questions to be used in considering how to optimise the College context for promoting the development of SRL:
• How can the rapid information flow, as observed in the 2020 informal network, be harnessed to improve teaching and learning practices across the college?
• What strategies could effectively manage the increased modularity seen in 2021 and 2022 to ensure cohesive and comprehensive information dissemination?
• In preventing the formation of silos and fostering a wider dissemination of innovative practices, how can the small-world characteristics of the formal network be optimally utilised?
• Considering the potential for epistemic bubbles and echo chambers, especially highlighted in the 2022 informal network’s high modularity, what measures can the college implement to promote a more inclusive educational environment and align teaching practices with broader organisational goals?
5 Conclusion
In returning to the metaphor of a game, managing a school is a complex game that can be “won” in many ways. Corroboree Frog College has its own unique set of rules—both formal and informal—within its system that can be leveraged by leadership. SNA revealed that the dissemination of information through the informal networks that arose over the period emerged as a response to aiming for the “win state” of effective decision-making, because that was “what worked”. If the original scaffold through formal networks was insufficient, the emergent informal networks may present a new pathway to the same goal. In other words, Corroboree Frog College was “entering the castle” in a different way.
To keep with the theme of “closing the loop” and to ground the work in the everyday realities of managing schools, we will conclude this paper with the reflections of an experienced leader of independent schools with no previous association with Corrobboree Frog College, who we have included as the third author for his work as a “critical friend” of the project. His reflection, reproduced here verbatim, suggest some interesting pathways for future research:
The deliberative approach you suggest could work if and when government acknowledges that the current curriculum is failing children rather than PISA test results show that children are failing their education. This is the mindset shift that needs to occur, in my opinion. While we fail to acknowledge that the metrics in use are flawed, we will be beholden to the criticism and consternation we have seen whenever comparative data across countries that actually have little in common is presented as some sort of empirical judgement. Governments seem open to this at the edges with trade training; but that has had to go through the process of low regulation, collapse and integration, VET in schools, and now back to trade training and the return of technical schools—of sorts. Perhaps a similar pattern for school-based education is possible with a skills driven curriculum meeting the needs of citizens in local communities on the other side.
Regarding the present analysis, I really like the concept and approach. The graphing of the network data is fascinating and identifies a limited few “experts” or “sources of truth” amongst the cohort of teachers. This is unsurprising. What would be interesting is to cross-reference the network data with office locations, meeting schedules, timetables and classes taught to see where, when and why these exchanges are occurring.
I have previously shared my observations regarding teachers viewing professional learning as an addition to their regular duties. To extend on this for a moment, I actually think the base issue is one regarding time: not quantums of time but the passage of time. The 36 week school year creates an essential dichotomy between proactive and reactive tasks for the teacher. Time management is about the most important skill a teacher must develop. The hard stage gates that exist such as school holidays, reports, assessment dates, etc., mean that calendric time clashes with a Bakhtin-like time as it is experienced; the two rarely, if ever, in synchronisation. I actually see this as the greatest change needed to fundamentally shift the culture of school workplaces for a sense of control is what is lost. I wager that the nodes in the diagram who are most influential will also be the most organised: they will be the epitome of the “give a task to a busy person” adage.
Data availability statement
The raw data supporting the conclusions of this article will be made available by the authors, without undue reservation.
Ethics statement
The studies involving humans were approved by University of South Australia Human Research Ethics Committee. The studies were conducted in accordance with the local legislation and institutional requirements. The participants provided their written informed consent to participate in this study.
Author contributions
LJ: Writing – original draft, Investigation, Formal analysis, Data curation. DD: Writing – review & editing, Writing – original draft. CB: Writing – original draft. SL: Writing – original draft, Supervision, Funding acquisition.
Funding
The author(s) declare that financial support was received for the research, authorship, and/or publication of this article. Funding for this project was supplied by the school examined in this paper. Details are withheld to maintain the anonymity of the research participants.
Acknowledgments
We acknowledge the support and open participation of the leadership of the school examined in this paper.
Conflict of interest
The authors declare that the research was conducted in the absence of any commercial or financial relationships that could be construed as a potential conflict of interest.
Publisher’s note
All claims expressed in this article are solely those of the authors and do not necessarily represent those of their affiliated organizations, or those of the publisher, the editors and the reviewers. Any product that may be evaluated in this article, or claim that may be made by its manufacturer, is not guaranteed or endorsed by the publisher.
Supplementary material
The Supplementary material for this article can be found online at: https://www.frontiersin.org/articles/10.3389/feduc.2024.1379520/full#supplementary-material
References
Abramovich, S., Schunn, C., and Higashi, R. M. (2013). Are badges useful in education?: it depends upon the type of badge and expertise of learner. Educ. Technol. Res. Dev. 61, 217–232. doi: 10.1007/s11423-013-9289-2
Balkundi, P., and Harrison, D. A. (2006). Ties, leaders, and time in teams: strong inference about network structure’s effects on team viability and performance. Acad. Manag. J. 49, 49–68. doi: 10.5465/AMJ.2006.20785500
Barabási, A.-L. (2002). Linked: The new science of networks. 1st Edn. Cambridge, MA: Perseus Publishing.
Barabási, A.-L., and Albert, R. (1999). Emergence of scaling in random networks. Science 286, 509–512. doi: 10.1126/science.286.5439.509
Barr, S., and Askell-Williams, H. (2019). Changes in teachers’ epistemic cognition about self–regulated learning as they engaged in a researcher-facilitated professional learning community. Asia Pac. J. Teach. Educ. 48, 187–212. doi: 10.1080/1359866x.2019.1599098
Bankins, S., Formosa, P., Griep, Y., and Richards, D. (2022). AI Decision Making with Dignity? Contrasting Workers’ Justice Perceptions of Human and AI Decision Making in a Human Resource Management Context. Inform. Sys. Front. 24, 857–875. doi: 10.1007/s10796-021-10223-8
Bastian, M., Heymann, S., and Jacomy, M. (2009). Gephi: an open source software for exploring and manipulating networks. Proceedings of the Third international AAAI conference on weblogs and social media, 3, 361–362
Borgatti, S. P., and Foster, P. C. (2003). The network paradigm in organizational research: a review and typology. J. Manag. 29, 991–1013. doi: 10.1016/S0149-2063(03)00087-4
Borgatti, S. P., Mehra, A., Brass, D. J., and Labianca, G. (2009). Network analysis in the social sciences. Science 323, 892–895. doi: 10.1126/science.1165821
Burt, R. S. (2000). The network structure of social capital. Res. Organ. Behav. 22, 345–423. doi: 10.1016/S0191-3085(00)22009-1
Callon, M., Lascoumes, P., and Barthe, Y. (2009). Acting in an uncertain world: An essay on technical democracy : The MIT Press.
Coburn, C. E. (2001). Collective sensemaking about reading: how teachers mediate reading policy in their professional communities. Educ. Eval. Policy Anal. 23, 145–170. doi: 10.3102/01623737023002145
Cross, R., Borgatti, S. P., and Parker, A. (2002). Making invisible work visible: using social network analysis to support strategic collaboration. Calif. Manag. Rev. 44, 25–46. doi: 10.2307/41166121
Cross, R., and Parker, A. (2004). The hidden power of social networks: Understanding how work really gets done in organizations : Harvard Business School Press.
De Laat, M., Lally, V., Lipponen, L., and Simons, R. J. (2007). Investigating patterns of interaction in networked learning and computer-supported collaborative learning: a role for social network analysis. Int. J. Comput. Support. Collab. Learn. 2, 87–103. doi: 10.1007/s11412-007-9006-4
De Smul, M., Heirweg, S., Devos, G., and Van Keer, H. (2019a). It’s not only about the teacher! A qualitative study into the role of school climate in primary schools’ implementation of self-regulated learning. Sch. Eff. Sch. Improv. 31, 381–404. doi: 10.1080/09243453.2019.1672758
De Smul, M., Heirweg, S., Devos, G., and Van Keer, H. (2019b). School and teacher determinants underlying teachers’ implementation of self-regulated learning in primary education. Res. Pap. Educ. 34, 701–724. doi: 10.1080/02671522.2018.1536888
Dever, D. A., and Azevedo, R. (2022). Scaffolding self-regulated learning in game-based learning environments based on complex systems theory. International Conference on Artificial Intelligence in Education
Dever, D. A., Sonnenfeld, N. A., Wiedbusch, M. D., Schmorrow, S. G., Amon, M. J., and Azevedo, R. (2023). A complex systems approach to analyzing pedagogical agents’ scaffolding of self-regulated learning within an intelligent tutoring system. Metacogn. Learn. 18, 659–691. doi: 10.1007/s11409-023-09346-x
Devolder, A., van Braak, J., and Tondeur, J. (2012). Supporting self-regulated learning in computer-based learning environments: systematic review of effects of scaffolding in the domain of science education. J. Comput. Assist. Learn. 28, 557–573. doi: 10.1111/j.1365-2729.2011.00476.x
Dunlosky, J., Rawson, K. A., Marsh, E. J., Nathan, M. J., and Willingham, D. T. (2013). Improving students’ learning with effective learning techniques:promising directions from cognitive and Educational Psychology. Psychol. Sci. Public Interest 14, 4–58. doi: 10.1177/1529100612453266
Eccles, J. S., and Roeser, R. W. (2011). Schools as developmental contexts during adolescence. J. Res. Adolesc. 21, 225–241. doi: 10.1111/j.1532-7795.2010.00725.x
Engeström, Y. (2000). Can people learn to master their future. J. Learn. Sci. 9, 525–534. doi: 10.1207/S15327809JLS0904_8
Engeström, Y. (2016). Studies in expansive learning: learning what is not yet there. New York: Cambridge University Press.
Fullan, M. (2007). The new meaning of educational change. 4th Edn. New York, NY: Teachers College Press.
Fowler, S., Gabriel, F., and Leonard, S. N. (2022). Exploring the effect of teacher ontological and epistemic cognition on engagement with professional development. Profess. Develop. Edu. 1–17. doi: 10.1080/19415257.2022.2131600
Gašević, D., Dawson, S., and Siemens, G. (2014). Let’s not forget: learning analytics are about learning. TechTrends 59, 64–71. doi: 10.1007/s11528-014-0822-x
Girvan, M., and Newman, M. E. J. (2002). Community structure in social and biological networks. Proc. Natl. Acad. Sci. 99, 7821–7826. doi: 10.1073/pnas.122653799
Goldfarb, A., and Lindsay, J. R. (2022). Prediction and Judgment: Why Artificial Intelligence Increases the Importance of Humans in War. Intern. Secu. 46, 7–50. doi: 10.1162/isec_a_00425
Hall, R. (2016). Technology-enhanced learning and co-operative practice against the neoliberal university. Interact. Learn. Environ. 24, 1004–1015. doi: 10.1080/10494820.2015.1128214
Hargreaves, A., and Fullan, M. (2012). Professional capital: Transforming teaching in every school : Teachers College Press.
Jamieson, K. H., and Cappella, J. N. (2010). Echo chamber: Rush Limbaugh and the conservative media establishment : Oxford Univeristy Press.
Kadushin, C. (2012). Understanding social networks: theories, concepts, and findings. Oxford University Press
Kogut, B., and Zander, U. (1996). What firms do? Coordination, identity, and learning. Organiz. Sci. 7, 502–518. doi: 10.1287/orsc.7.5.502
Krackhardt, D., and Hanson, J. R. (1993). Informal networks: the company behind the chart. Harv. Bus. Rev. 74:104,
Krath, J., Schürmann, L., and von Korflesch, H. F. O. (2021). Revealing the theoretical basis of gamification: a systematic review and analysis of theory in research on gamification, serious games and game-based learning. Comput. Hum. Behav. 125:106963. doi: 10.1016/j.chb.2021.106963
Latapy, M. (2008). Main-memory triangle computations for very large (sparse (power-law)) graphs. Theor. Comput. Sci. 407, 458–473. doi: 10.1016/j.tcs.2008.07.017
Lee, M., Lee, S. Y., Kim, J. E., and Lee, H. J. (2023). Domain-specific self-regulated learning interventions for elementary school students. Learn. Instr. 88:101810. doi: 10.1016/j.learninstruc.2023.101810
Leonard, S. N., Belling, S., Morris, A., and Reynolds, E. (2017). Playing with rusty nails: ‘conceptual tinkering’ for ‘next’ practice. EDeR: educational design research 1, 1–22. doi: 10.15460/eder.1.1.1027
Littlejohn, A., and Hood, N. (2018). The [un]democratisation of education and learning. SpringerBriefs in open and distance education, 21–34
Lupton, R., and Hayes, D. (2021). Great mistakes in education policy: And how to avoid them in the future. 1st Edn: Policy Press.
Miettinen, R., and Virkkunen, J. (2005). Epistemic objects, artefacts and organizational change. Organization, 12, 437–456. doi: 10.1177/1350508405051279
Newman, M. E. J., and Girvan, M. (2004). Finding and evaluating community structure in networks. Phys. Rev. E 69:e26113. doi: 10.1103/PhysRevE.69.026113
Nguyen, C. T. (2020a). Echo chambers and epistemic bubbles. Episteme 17, 141–161. doi: 10.1017/epi.2018.32
Nietfeld, J. L. (2017). The role of self-regulated learning in digital games. Handbook of self-regulation of learning and performance, 271–284. Routledge
O’Leary, R., Mortensen, M., and Woolley, A. W. (2011). Collaboration across boundaries: insights and tips from federal senior executives. IBM Center for The Business of Government. Available at: https://www.businessofgovernment.org/report/collaboration-across-boundaries-insights-and-tips-federal-senior-executives
Olejnik, S., and Algina, J. (2000). Measures of effect size for comparative studies: applications, interpretations, and limitations. Contemp. Educ. Psychol. 25, 241–286. doi: 10.1006/ceps.2000.1040
Page, S. (2008). The difference: how the power of diversity creates better groups, firms, schools, and societies. new Edn: Princeton University Press.
Prinsloo, P., and Slade, S. (2016). Big data, higher education and learning analytics: beyond justice, towards an ethics of care, Big data and learning analytics in higher education: Current theory and practice, 109–124
Roffey, S. (2012). Positive relationships: Evidence based practice across the world. 2nd Edn. Netherlands: Springer.
Sannino, A., Engeström, Y., and Lemos, M. (2016). Formative interventions for expansive learning and transformative agency. J. Learn. Sci. 25, 599–633. doi: 10.1080/10508406.2016.1204547
Schrader, P. G., and McCreery, M. (2012). Are all games the same?, D. Ifenthaler, D. Eseryel, and X. Ge (Eds.), Assessment in game-based learning: Foundations, innovations, and perspectives, 11–28. Springer New York
Simpson, A. (2017). The misdirection of public policy: comparing and combining standardised effect sizes. J. Educ. Policy 32, 450–466. doi: 10.1080/02680939.2017.1280183
Society for Learning Analytics Research (SoLAR). (2024). What is learning analytics. Society for Learning Analytics Research (SoLAR). Available at: https://www.solaresearch.org/about/what-is-learning-analytics/
Steinbach, J., and Stoeger, H. (2016). How primary school teachers’ attitudes towards self-regulated learning (SRL) influence instructional behavior and training implementation in classrooms. Teach. Teach. Educ. 60, 256–269. doi: 10.1016/j.tate.2016.08.017
Sunstein, C. R. (2017). #republic: Divided democracy in the age of social media (paperback edition with a new afterword by the author. Ed.) : Princeton University Press.
Tsai, W. (2002). Social structure of "coopetition" within a multiunit organization: coordination, competition, and intraorganizational knowledge sharing. Organiz. Sci. 13, 179–190. doi: 10.1287/orsc.13.2.179.536
Tushman, M. L., and O’Reilly, C. A. (1996). The ambidextrous organizations: managing evolutionary and revolutionary change. Calif. Manag. Rev. 38, 8–29. doi: 10.2307/41165852
Vosniadou, S., Darmawan, I., Lawson, M. J., Van Deur, P., Jeffries, D., and Wyra, M. (2021). Beliefs about the self-regulation of learning predict cognitive and metacognitive strategies and academic performance in pre-service teachers. Metacogn. Learn. 16, 523–554. doi: 10.1007/s11409-020-09258-0
Watts, D. J., and Strogatz, S. H. (1998). Collective dynamics of ‘small-world’ networks. Nature 393:440. doi: 10.1038/30918
Wiley, K., Dimitriadis, Y., and Linn, M. (2023). A human-centred learning analytics approach for developing contextually scalable K-12 teacher dashboards. Br. J. Educ. Technol. 55, 845–885. doi: 10.1111/bjet.13383
Williams, R., and Edge, D. (1996). The social shaping of technology. Res. Policy 25, 865–899. doi: 10.1016/0048-7333(96)00885-2
Wong, S. S. H., Lim, K. Y. L., and Lim, S. W. H. (2023). To ask better questions, teach: learning-by-teaching enhances research question generation more than retrieval practice and concept-mapping. J. Educ. Psychol. 115, 798–812. doi: 10.1037/edu0000802
Wrigley, T. (2019). The problem of reductionism in educational theory: complexity, causality, values. Power Educ. 11, 145–162. doi: 10.1177/1757743819845121
Xian, T. (2021). Exploring the effectiveness of sandbox game-based learning environment for game design course in higher education. Cham: Human Interaction, Emerging Technologies and Future Applications IV.
Yang, S., Keller, F., and Zheng, L. (2017). Social network analysis: Methods and examples. Thousand Oaks, CA: SAGE Publications, Inc.
Zap, N., and Code, J. (2009). Self-regulated learning in video game environments. Handbook of research on effective electronic gaming in education, 738–756. IGI Global
Keywords: social network analysis, learning analytics, actionable learning analytics, expansive education, pragmatic adaptive leadership, what works, complex learning environments
Citation: Johnson L, Devis D, Bacholer C and Leonard SN (2024) Closing the loop by expanding the scope: using learning analytics within a pragmatic adaptive engagement with complex learning environments. Front. Educ. 9:1379520. doi: 10.3389/feduc.2024.1379520
Edited by:
Bart Rienties, The Open University (United Kingdom), United KingdomReviewed by:
José Cravino, University of Trás-os-Montes and Alto Douro, PortugalDirk Tempelaar, Maastricht University, Netherlands
Copyright © 2024 Johnson, Devis, Bacholer and Leonard. This is an open-access article distributed under the terms of the Creative Commons Attribution License (CC BY). The use, distribution or reproduction in other forums is permitted, provided the original author(s) and the copyright owner(s) are credited and that the original publication in this journal is cited, in accordance with accepted academic practice. No use, distribution or reproduction is permitted which does not comply with these terms.
*Correspondence: Lesley Johnson, johnsole@trinity.sa.edu.au; Simon N. Leonard, simon.leonard@unisa.edu.au