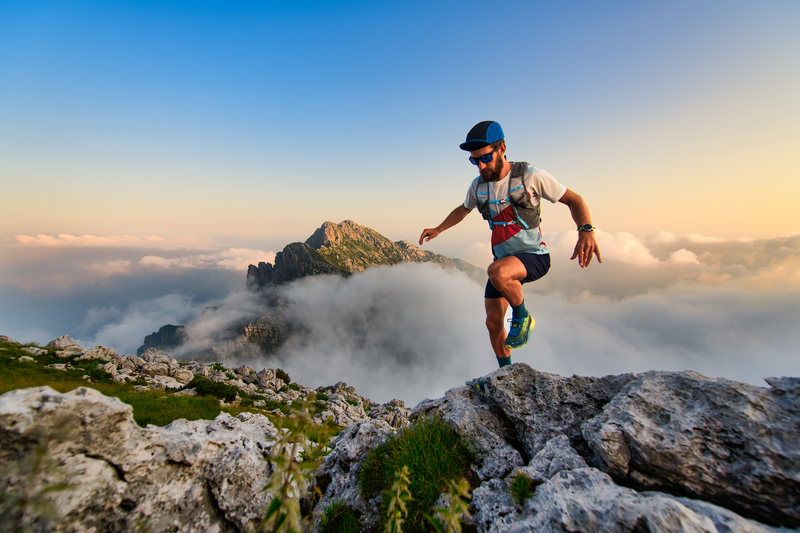
94% of researchers rate our articles as excellent or good
Learn more about the work of our research integrity team to safeguard the quality of each article we publish.
Find out more
ORIGINAL RESEARCH article
Front. Educ. , 11 September 2024
Sec. Digital Learning Innovations
Volume 9 - 2024 | https://doi.org/10.3389/feduc.2024.1365258
This article is part of the Research Topic Emerging Technologies and Digital Innovations: Recent Research and Practices in Technology-enhanced Learning Environments View all 11 articles
Introduction: This study explores the motivators to use learning management systems (LMS) adopted and used in eLearning by Hong Kong universities among Mainland Chinese postgraduate students amid COVID-19 pandemic.
Methods: An adapted and extended UTUAT2 model was first proposed and tested using the structural equation modeling approach. Through self-report online questionnaire, data were collected in 2022 from 352 Mainland Chinese postgraduate students of nine universities in Hong Kong. The reliability and validity of the data were tested using the confirmatory factor analysis, followed by path analysis to test the hypotheses in the proposed model.
Results: The study revealed nine motivators which explained 50% of the variance in LMS use intention. Trust (0.204), instructor characteristics (0.202), performance expectancy (0.181), and facilitating conditions (0.181) were identified as strong motivators of behavioral intention. Other factors such as effort expectancy (0.148), learning value (0.118) and social influence (0.115) also had significant positive effects on LMS use intention. Habit (0.014) and hedonic motivation (−0.016) had no significant direct effect on it.
Discussion: These findings provide inspirations for educational stakeholders to promote the acceptance of LMS platforms among distance and online learners who adopt the cyberspace as the only means of learning. They also offer insights about instructor recruitment and evaluation methods.
Traditionally, learners preferred face-to-face learning for in-depth discussion on course contents, judgement, reasoning and explanation (Chen et al., 2014), collaboration with peers and emotional support from instructors (Thompson and MacDonald, 2005). However, the coronavirus pandemic led to sudden closure of campuses in 192 countries and projected an unprecedented impact on universities worldwide (UNESCO, 2020). The abrupt shift from face-to-face classes to remote online teaching has promoted a “new normality” in university education. According to the Hong Kong government data, in early March 2022, there were over 260,000 local COVID-19 cases recorded in the region (Information Services Department, 2022). Since all universities in Hong Kong were forced to shut down their physical campuses at that time, eLearning became the only workable solution for students to keep on their learning pace. Learning management system (LMS) provided an essential platform which guaranteed the sustainability of learning, teaching and administration for the affected learners and instructors. This research was conducted during a period when postgraduate teaching and learning had shifted entirely to an online-only mode, without any in-person or blended components. There was a heavy reliance on LMS to facilitate the transition to fully remote instruction and administration. As no research has been done on LMS acceptance in Hong Kong targeted at the Mainland Chinese postgraduate students during the pandemic, this study focuses on the motivators to accept LMS among this particular population in the entirely online learning contex.
We hypothesize that trust and instructor characteristics play a crucial role in motivating LMS adoption during the pandemic. Trust plays a crucial role in nurturing personal interactions (Cheung and Lai, 2022) and building users’ confidence to adopt risky technologies such as mobile payment (Khalilzadeh et al., 2017). Therefore, we expect that trust in LMS is an influential factor of LMS adoption among postgraduate students. During the pandemic, students could utilize the LMS to submit assignments, have private video conference calls with instructors for academic discussions, and seek personal guidance on sensitive topics like alternative assessment arrangements, which arose as a result of the impact of COVID-19 on students’ health. However, if students lacked trust in the LMS’s security, data protection capabilities, and overall reliability, they might hesitate to share personal health information, assignments, or discuss their learning challenges on the platform. Instructors’ characteristics are hypothesized to be influential in LMS adoption during the pandemic. As Mainland Chinese students distinguished themselves from Hong Kong students in cultural, economic, and educational backgrounds (Yu and Zhang, 2016), they might find it a stressful experience to adapt to new host learning environments with limited interaction with instructors and classmates at the time under social isolation (Hagedorn et al., 2022). If instructors demonstrated enthusiasm in interacting with students via the LMS, students might be more likely to have higher acceptance to it.
We chose to conduct the questionnaire survey during the pandemic to reach the Mainland Chinese students who studied postgraduate programs in Hong Kong. Through convenience sampling, sufficient samples were collected for structural equation modeling (SEM) analysis and representative results. Mainland Chinese postgraduate students were chosen to be the sample of the study. Hong Kong has become a top location for Mainland Chinese students to pursue their postgraduate study (Vyas and Yu, 2018) due to geographical proximity, mix features of eastern and western cultures, financial support and scholarship (Li and Bray, 2007), international learning environment (Cheng et al., 2015; Vyas and Yu, 2018) and high academic reputation of its universities (Xiong et al., 2020). Over the past two decades, Hong Kong has witnessed a substantial rise in the number of non-local students, predominantly consisting of Mainland Chinese students (Education Bureau, 2020). Furthermore, the number of Mainland Chinese students in Hong Kong is expected to increase further as the Chinese Ministry of Education has approved self-financing institutions to raise their maximum enrolment from 10 percent to 20 percent in 2023 (Ministry of Education, the People’s Republic of China, 2023).
Previous studies have highlighted a lack of comprehensive investigation into the cross-border learning of Mainland Chinese students in Hong Kong (Wu et al., 2024). As this population continues to grow, it becomes crucial to understand the factors that drive their adoption of LMS. Although the pandemic has resulted in a widespread acceptance of online education, with majority of students expressing overall satisfaction with this mode of learning (Chakraborty et al., 2020), the factors contributing to their positive perceptions remain understudied. The results of this study can help to reveal the motivators of LMS adoption and facilitate the development of effective strategies to meet Mainland Chinese postgraduate students’ educational needs, ultimately enhancing the dynamism of both learning and teaching.
The research questions are set as below:
RQ 1: What are the major factors which affected the Mainland Chinese postgraduate students’ experience and level of acceptance to use learning management systems provided by Hong Kong institutions during the COVID-19 pandemic?
RQ 2: To what extent did the factors affect the Mainland Chinese postgraduate students’ acceptance to use learning management systems provided by Hong Kong institutions during the COVID-19 pandemic?
Our study makes the following four contributions. First, the study contributes to the existing empirical studies on eLearning adoption by suggesting a new UTAUT model with nine motivators which explained LMS use intention. We added the “trust” and “instructor characteristics” constructs to the UTAUT2 model to better understand the factors contributing to students’ acceptance on LMS. The two additional factors are indispensable for the use of LMS during the pandemic period. Our findings show that educators and policy makers should improve students’ trust in LMS and provide facilitating conditions of LMS use for enhancing learning quality. We adapt and extend the UTAUT2 model to investigate the determinants of behavioral intention to use LMS adopted by Hong Kong universities. To our best knowledge, this is the first research to examine the impacts of “learning value” in replacement of “price value” in the original UTAUT2 model in the Hong Kong higher education context. This provides insights to educators and system engineers that students are willing to use LMS only if the sacrifice, time and effort spent on it are reasonable. Second, the study focuses on Mainland Chinese postgraduate students, as traditional studies only target at undergraduates despite the growing trend of this target group to study in Hong Kong universities. Our findings provide valuable insights that can help educators design policies to better encourage and support adult learners in using LMS, as the factors that influence their use of LMS may be different from those of undergraduate students. Third, the findings provide a comprehensive picture about factors’ influencing students’ intention of LMS use, which helps institutions to prepare for students’ sustainable learning during unprecedented crises. Last, the results urge the universities to assess instructors’ characteristics that support students’ use of LMS in recruitment and teaching evaluation surveys.
The remaining part of this paper is structured as below. Section 2 provides the theoretical background about the role of LMS in learning, the UTAUT2 framework and recent studies on eLearning technology acceptance in Asian countries. Section 3 presents the measurement model and research hypotheses to be tested. Section 4 discusses the methodology, measuring instrument, sampling and data collection. Section 5 presents and analyzes the results. Section 6 contains discussion of results and Section 7 includes conclusions. Section 8 discusses the research implications and recommendations. Finally, Section 9 includes limitations of the study and provides suggestions for future studies.
A learning management system is an integrated interactive learning environment which integrates communication tools such as private message, notice board, chatroom and provides supplementary multimedia learning materials such as graphics, videos, audio files, references, self-tests and quiz modules (El-Masri and Tarhini, 2017). LMSs are widely adopted by Hong Kong higher institutions to incorporate video communication tools (such as Zoom, Panopto and Cisco Webex), personal response systems (such as Qualtrics, an online survey software), game-based learning assessment tools (such as Kahoot!), anti-plagiarism tools (such as Turnitin and iThenticate) and presentation tools (such as Mentimeter).
Common web-based and mobile-based LMSs adopted by different universities in Hong Kong include Blackboard, Canvas and Moodle, which offer students with flexibility to complete their assignments at their preferred pace and access to course materials (Al-Adwan and Al-Debei, 2024). It is a mandatory requirement for students to attend synchronous (live-streaming) and asynchronous (pre-recorded) classes, access to the printed and multimedia course materials, complete and submit their academic tasks (e.g. exercises or/and quizzes), as well as receive feedbacks through LMSs. Given the use of LMS is mandatory among most Mainland Chinese postgraduate students who pursue their study, it would be vital to understand the drivers which affect students’ intention to use LMS during the emergence of pandemic.
The Unified Theory on Acceptance and Use of Technology (UTAUT) is a classic model for explaining and predicting technology acceptance in organizational setting. This comprehensive model was compiled from eight models and theories including Theory of Reasoned Action (TRA), Theory of Planned Behavior (TPB), Technology Acceptance Model (TAM), combined TAM and TPB (C-TAM-TPB), Model of PC Utilization (MPCU), Motivational Model (MM), Social Cognitive Theory (SCT) and Diffusion of Innovation Theory (IDT) (Venkatesh et al., 2003). The UTAUT2 was later proposed to study the mobile technology acceptance in the Hong Kong consumer context to improve the generalizability of the model (Venkatesh et al., 2012). The UTAUT2 framework consists of four constructs, including performance expectancy, social influence, facilitating conditions and effort expectancy from the UTAUT model and three extra constructs including habit, price value and hedonic motivation as predicators of intention to use and behavior (Figure 1). Whilst the price value construct is added to highlight the importance of product quality-to-price ratio, the habit and hedonic motivation constructs are included for prior behavior and stressing utility respectively.
Figure 1. Unified theory on acceptance and use of technology 2 model (Venkatesh et al., 2012).
A recent quantitative study about the intention and use of eLearning technologies was conducted by Raman and Thannimalai (2021) in a Malaysia university during the COVID-19 pandemic. The researchers carried out a survey with seven constructs (performance expectancy, effort expectancy, social influence, facilitating conditions, hedonic motivation, habit, price value) based on the adapted UTAUT2 model to 159 undergraduate students from Universiti Utara Malaysia. The findings show that habit is the strongest predictor for behavioral intention to use eLearning technologies. Alternatively, social influence also dramatically influences behavioral intention to use eLearning platforms. However, price value is insignificant as students had free access to eLearning technologies such as mobile applications (Google Classroom and Google Meet) and social networking applications (WhatsApp, WeChat and Telegram) when the survey was conducted.
This research adopted the extended UTAUT2 to identify the factors influencing the acceptance of eLearning platforms among medical students during the COVID-19 pandemic. The data were collected by online survey from 360 medical doctorate students aged between 18 and 34. The proposed model is based on UTAUT2 with two additional constructs, learning value (in replacement of price value in UTAUT2) and instructor characteristics. The results have shown that performance expectancy is the most significant predictors to behavioral intention, while learning value and instructor characteristics have a positive effect on behavioral intention. Whether instructors fully utilized the eLearning platform, kept motivating students and answering students’ questions were key factors which affected students’ intention to use the eLearning platforms. Alternatively, social influence, facilitating conditions, effort expectancy, hedonic motivation and habit were insignificant predictors to behavioral intention in this developing country.
This study employed the UTAUT model with extension of two dimensions, mobile self-efficacy and perceived enjoyment to investigate the factors which influence university students’ acceptance on LMS during the COVID-19 pandemic. The quantitative data were collected online from 1,875 graduates, undergraduate and postgraduate students from five different Asian countries, including developing countries such as India, Pakistan, Bangladesh and developed countries like Malaysia and South Korea. The results have demonstrated the high predictability of the UTAUT model, with performance expectancy, effort expectancy, facilitating conditions and social influence as the significant predictors of behavioral intention. While performance expectancy exerted the maximum influence on behavioral intention, mobile self-efficacy also had a significant impact on participants’ acceptance on LMSs. However, perceived enjoyment did not have observable influence on behavioral intention as the utilitarian value of eLearning system was highly treasured during pandemic. The results have confirmed the predictability of the UTAUT model during the global crisis.
Previous research across different Asian countries during the COVID-19 pandemic has yielded inconsistent findings on the factors influencing the adoption of eLearning technologies and platforms. However, performance expectancy and social influence have been commonly identified as the key factors of behavioral intention to use eLearning technologies and platforms. Given the critical role of LMS in facilitating purely online learning, and the unpredictability of future crises, this study investigates the adoption of LMS among the growing population of Mainland Chinese postgraduate students in Hong Kong, within the context of a solely online learning environment.
The UTAUT2 constructs are adopted with modifications for the study. First, the learning value construct replaces the price value construct in the original UTAUT2 model. Second, two new constructs, namely instructor characteristics and trust, are added to our model. The hypotheses are developed as follows to answer the research questions.
Ajzen (2011) defines behavioral intention as an indicator of a person’s readiness to perform a specific behavior. Numerous studies have proven that behavioral intention plays a crucial role in actual behaviors (Burke, 2002). In this study, behavioral intention is associated with the Mainland Chinese postgraduate students’ readiness to use LMSs adopted and used by universities in Hong Kong. It is the only endogenous variable in the model, determined by other exogenous variables.
Performance expectancy is defined as the extent to which a person trusts that adopting the system will assist him or her in improving job performance (Venkatesh et al., 2003). Recent studies have consistently demonstrated the significant effect of performance expectancy on technology adoption among students and teachers (Ahmed et al., 2022; Chao, 2019; Lai et al., 2024; Prasetyo et al., 2021; Raza et al., 2021). In the current study, performance expectancy refers to Mainland Chinese postgraduate students’ beliefs about the usefulness of the LMSs adopted by universities in Hong Kong. Thus, the following hypothesis is developed:
H1: Performance expectancy has a positive effect on the Mainland Chinese postgraduate students’ behavioral intention to use LMSs by Hong Kong universities.
Effort expectancy reflects the level of ease associated with utilizing the system (Venkatesh et al., 2003, p. 450). Existing research has demonstrated that effort expectancy significantly influences students’ willingness to adopt eLearning technologies (Ahmed et al., 2022; Chao, 2019; Raza et al., 2021). However, earlier studies have contended that the mere ease of use of a technology does not necessarily guarantee its acceptance by individuals (Dindar et al., 2021; Çera et al., 2020). Instead, learners are more likely to adopt a technology if they have trust in its ability to provide learning benefits and if it is perceived as user-friendly. In this study, effort expectancy represents Mainland Chinese postgraduate students’ beliefs about the effort or ease related to the LMSs adopted and used by universities in Hong Kong. We assume that students’ behavioral intention to use LMSs is developed through their positive understanding about the LMSs’ easiness. The following hypothesis is proposed:
H2: Effort expectancy has a positive effect on the Mainland Chinese postgraduate students’ behavioral intention to use LMSs by Hong Kong universities.
Social influence represents the degree to which individuals perceive other important people believe if they should adopt the new system (Venkatesh et al., 2003). Extant studies have identified social influence as a significant determinant of eLearning technology and meta-education acceptance (Ahmad et al., 2024; Ahmed et al., 2022; Raman and Thannimalai, 2021). Its effect on behavioral intention may be stronger in the Chinese collectivistic society, where individuals’ tendency to adopt a technology is likely to rely on subjective evaluation of it from other-like-minded peers (Lee et al., 2013). However, recent studies have also indicated insignificant effects of social influence on metaverse technology adoption among Gen Z students (Al-Adwan and Al-Debei, 2024) and on eLearning adoption among doctorate students (Prasetyo et al., 2021). In our study, social influence refers to the impact of other people’s beliefs on Mainland Chinese postgraduate students’ intention to use LMSs in this study. The following relationship is hypothesized:
H3: Social influence has a positive effect on the Mainland Chinese postgraduate students’ behavioral intention to use LMSs by Hong Kong universities.
Facilitating conditions refer to “the degree to which an individual considers that an organization and technical infrastructure exist to support the use of the system” (Venkatesh et al., 2003, p. 453). Technical guidance, system management, training and prompt assistance as well as other logistic arrangements to the users are essential facilitating conditions for using the technology (Mei et al., 2017). Previous studies have shown that facilitating conditions promote students’ intention to learn (Zacharis and Nikolopoulou, 2022) and interact with each other (Wut et al., 2022) on eLearning platforms. Nevertheless, Prasetyo et al. (2021) and Raman and Thannimalai (2021) evidenced no significant relationship between facilitating conditions and behavioral intention in the context of eLearning during the pandemic. In the current research, facilitating conditions relate to the sufficiency of resources, training opportunities, timely assistance and technical support provided to students to facilitate their use of LMSs. The below relationship is proposed:
H4: Facilitating conditions has a positive effect on the Mainland Chinese postgraduate students’ behavioral intention to use LMSs by Hong Kong universities.
Hedonic motivation concerns about the pleasure or fun derived from adopting a technology, which is crucial in predicting use intention of a technology (Venkatesh et al., 2012). Hedonic motivation was commonly recognized as a notable driving force behind behavioral intention in educational settings (Al-Gahtani, 2016; El-Masri and Tarhini, 2017). However, recent studies conducted amidst the COVID-19 pandemic have yielded contrasting findings. Specifically, these studies have indicated that hedonic motivation does not have a direct and significant relationship with the acceptance of LMSs (Prasetyo et al., 2021; Raman and Thannimalai, 2021). In this study, hedonic motivation refers to the pleasure or fun experienced by users of LMSs. The following relationship is hypothesized:
H5: Hedonic motivation has a positive effect on the Mainland Chinese postgraduate students’ behavioral intention to use LMSs by Hong Kong universities.
Since the price value of the eLearning technologies is difficult to be calculated from the tuition fee, we followed Ain et al. (2016) to replace “price value” with “learning value” in the research model to fill in the research gap in Hong Kong. While Harja et al. (2020) and Ain et al. (2016) have found learning value a strong predictor of eLearning technology and LMS adoption among students respectively, Prasetyo et al. (2021) have evidenced that learning value significantly predicts eLearning platform adoption among medical students during the pandemic.
Students devote time and effort (also part of the tuition fees paid to support the development and maintenance of technologies) to gain benefits from LMSs. The use of LMSs holds a value if knowledge is gained from using them. In this study, learning value refers to the cognitive trade-off between the effort and time spent on LMSs and their perceived value by users. Thus, the hypothesis is proposed as follows:
H6: Learning value has a positive effect on the Mainland Chinese postgraduate students’ behavioral intention to use LMSs by Hong Kong universities.
Habit is considered as an individual’s tendency to act spontaneously for learning (Venkatesh et al., 2012). Earlier research has revealed that individuals’ prior experience with technology usage contributes to their ongoing actions, as evidenced by their behavioral intention (El-Masri and Tarhini, 2017; Masa’deh et al., 2016; Moorthy et al., 2019; Raman and Thannimalai, 2021). In this study, habit relates to students’ habitual or automatic behaviors of using LMSs. Students who consistently utilize LMSs are believed to have higher potential in using them. Therefore, the following hypothesis is suggested:
H7: Habit has a positive effect on the Mainland Chinese postgraduate students’ behavioral intention to use LMSs by Hong Kong universities.
Trust refers to one’s readiness to accept vulnerability based on positive expectations about the intentions or the acts of others in an environment featured by risk and interdependence (Ennew and Sekhon, 2007). Trust plays a crucial role in promoting information exchange, which is essential in the knowledge transfer process (Cheung and Lai, 2022). Prior literature has shown dichotomous results about the relationship between users’ decisions to adopt eLearning technologies and their perceived reliability and trustworthiness of system. Recent studies have identified significant effect of trust on behavioral intention in eLearning, m-learning and self-regulated learning context (Chao, 2019; El-Masri and Tarhini, 2017; Harja et al., 2020; Lai et al., 2024). Wang and Yu (2015) have showed that the better educated Chinese are more conscious of precautions and therefore have lower tendency to trust. Despite of these, some have found no direct relationship between trust and behavioral intention in the consumer and information system context (Eneizan et al., 2019; Kabra et al., 2017).
In this study, trust refers to the Mainland Chinese postgraduate students’ understanding towards the trustworthiness and reliability of LMSs. Users’ perception on security and trust are expected to be important factors of LMS acceptance. Therefore, the following hypothesis is set:
H8: Trust has a positive effect on the Mainland Chinese postgraduate students’ behavioral intention to use LMSs by Hong Kong universities.
Instructor characteristics is the extent to which the instructors show care, give advice, and accommodate their students’ learning needs (Ibrahim et al., 2017). Existing literature has shown inconsistent influence of instructor characteristics on behavioral intention. While numerous studies have reported the positive impact of instructor characteristics on perceived usefulness of eLearning technology and behavioral intention to use it (Lee et al., 2009; Prasetyo et al., 2021; Selim, 2007), Ibrahim et al. (2017) have indicated no direct or indirect relationship of instructor characteristics on behavioral intention. In this study, we follow the framework proposed by Prasetyo et al. (2021) to include the instructor characteristics construct to explore the importance of instructors’ support during social isolation at the time of pandemic. In this research, instructor characteristics is defined as the instructors’ tendency to encourage, motivate and facilitate Mainland Chinese postgraduate students to use LMSs. Accordingly, the following hypothesis is set:
H9: Instructor characteristics have a positive effect on the Mainland Chinese postgraduate students’ behavioral intention to use LMSs by Hong Kong universities.
The research model of the study is proposed as follows (Figure 2):
This set of hypotheses covers the direct relationships (i.e., H1 to H9) between the nine exogenous variables (performance expectancy, effort expectancy, social influence, facilitating conditions, hedonic motivation, learning value, habit, trust and instructor characteristics) and the only endogenous variable (behavioral intention) in the proposed research model.
A non-experimental and quantitative research methodology is adopted in this study. Using a SEM approach, the current research tests the relationships among the latent variables adapted and extended from the UTAUT2 framework. First, we conducted a demographic analysis to understand the participant profiles. Then, using IBM SPSS AMOS (version 20) software, we performed the SEM technique in two stages – confirmatory factor analysis (CFA) and path analysis. CFA helps to confirm the uni-dimensionality, reliability and validity of the constructs (MacCallum and Austin, 2000). After that, the structural model was analyzed using path analysis to examine the hypotheses of the research.
An online questionnaire survey including 40 items related to technology acceptance and use is designed to collect descriptive and quantitative samples from Mainland Chinese postgraduate students at different universities in Hong Kong during the pandemic. The measurement instrument represents the theoretical model that relates the latent variables to the manifest indicators, which are measured through questionnaire survey. The survey is divided into two sections. The first section aimed to gather demographic information and the second section then sought to find out participants’ intention to use LMS.
The 40 manifest items and the corresponding descriptions for measuring the 10 latent variables are presented in English in Table 1. Participants were requested to show their levels of agreement towards the descriptions based on a 5-point Likert scale arranging from “strongly disagree” to “strongly agree”.
Before conducting the data analysis, we performed data screening to fulfil the requirement of multivariate analysis. We purified the data by replacing missing values, assessing outliers, data normality and multicollinearity. The common method bias refers to a systematic error in research data which arises from the measurement method itself instead of the constructs being measured (Podsakoff et al., 2003). This bias can occur when data is collected using the same method for all variables in a single study. As all data was collected from self-report questionnaire in our study, Harman’s single-factor test was employed to assess the common method bias.
The survey was conducted to collect samples from postgraduate students at different universities in Hong Kong from March to April 2022. After the pilot study, the revised questionnaire was distributed on a user-friendly survey tool1 through eleven WeChat App groups (group size from 358 to 500) for Mainland Chinese students who had enrolled to taught postgraduate programs in Autumn 2021. A number of 352 questionnaires were returned online with a response rate of 7.6%. From March to April 2022, all the universities in Hong Kong were closed so the use of web-based and mobile-based LMSs (Blackboard, Canvas and Moodle) was mandatory at the time of study. In order to reach a vast number of samples from different universities, convenience sampling method was adopted in this study. For SEM studies, a sample size between 200 and 400 is considered appropriate (Hair et al., 2019; Rehman et al., 2022). Data were collected from postgraduate students who participated in the survey voluntarily without any incentives. All the responses were collected online as social distancing posed a barrier for face-to-face data collection.
In this study, the missing data of the study is less than 2%. As suggested by Cohen (1983), missing data up to 10% may not cause any serious problem in the interpretation of the findings. We adopted the most common treatment to replace the missing data with values of the median responses for each variable (Hair et al., 2019).
For univariate detection, each variable was examined for the standardized (z) score. According to Hair et al. (2019), absolute (z) > 4 is evidenced of an extreme observation for large sample size above 200. The standardized (z) scores of the cases are summarized in Table 2 for the items in each construct. The results reflected that the standardized (z) scores of the manifest items ranged between −3.144 and 3.200, with no variable exceeding the threshold of ± 4. These indicated no univariate outlier among the data from 352 participants.
For identifying the multivariate outliers in the current research, Mahalanobis distance (D2) were generated for each case using AMOS regression with case number as the dependent variable and all non-demographic measures as independent variables. High D2 / df value greater than 3.5 represents potential multivariate outlier (Hair et al., 2019). As shown in Table 3 below, the largest D2 value is 72.40 (belongs to case 40). Regarding the 90 exogenous and endogenous variables together with their relative estimation errors in this study, the maximum D2 / df was equal to 0.804 (72.40 / 90) which was far below the cut-off 3.5. Therefore, it can be concluded that the examination of D2 values for all cases did not indicate the presence of multivariate outliers, so all observations were retained as final data for analysis.
Table 4 shows the assessment results of normality. The skew ranges from −1.662 to 0.467 and the kurtosis ranges from −0.703 to 2.775 which fall within the range of ± 3 and ± 7. These reflect the data set is well-modelled by normal distribution. The results also indicate that critical ration (c.r.) of multivariate normality for all 40 items is 0.320, which is below the threshold of ± 5 as recommended by Yuan et al. (2005).
The participants’ demographic profile is shown in Table 5. The findings show that female participants (73%) are significantly more than the males (27%), reflecting the high proportion of female Mainland Chinese postgraduate students studying in Hong Kong. The majority (93.2%) of participants was less than 40 years old from a variety of higher institutions. Participants were from different disciplines, with most of them having enrolled in business (23.6%), arts, journalism and language (17.9%), engineering and information technology (12.8%). Most of them had good (41.2%) to very good (38.1%) internet connectivity in their study location. They generally possessed some eLearning experience, with the majority having less than two years (27.6%), two to four years (42%) and five to seven years (24.7%) experience.
The findings reveal that one factor model explains 25.255% of the total variance, suggesting that the common method variance is not a serious problem (Hoyle, 1995). The results are presented in Table 6.
This study comprises an overall confirmatory factor analysis (CFA) model with discussion of the measurement model development. To ensure the CFA accuracy, Hair et al. (2019) proposed examining goodness-of-fit indicators, convergent and discriminant validity. The first step of CFA is to evaluate the goodness of fit (GOF) indices of the measurement model. The GOF indices of our measurement model and the recommended values from literatures are listed in Table 7. From the values below, we conclude that the measurement model has good fit of data and requires no adjustments.
The second step of CFA is to examine the reliability and convergent validity of each construct. The reliability was assessed through reviewing the Cronbach’s alpha, composite reliability (CR) and average variance extracted (AVE) whereas the constructs’ convergent and discriminant validity were also examined. The results of CFA are presented in Table 8. The factor loadings for all 40 items are more than 0.5, which shows the meanings of the factors have been preserved by these indicators. The AVEs, ranging between 0.610 and 0.763, exceed the cut-off value of 0.5 (Fornell and Larcker, 1981). The CR values, ranging from 0.862 to 0.928, are above the recommended value of 0.6 (Bagozzi and Yi, 1988) so the measurement is considered reliable. The Cronbach’s Alpha values, ranging between 0.862 and 0.927, are above the threshold of 0.7 (Nunnally and Bernstein, 1994) so the manifest indicators of a latent construct are considered as closely related and sufficiently error-free.
Table 9 below shows the discriminant validity of the measurement model. The inter-factor correlations between the ten constructs range from −0.090 to 0.544, which are less than the threshold of 0.85 (Kline, 2010). The correlations are less than the square root of the AVE by the indicators, which reflects a satisfactory discriminant validity.
After evaluating the goodness to fit indices, convergent and discriminant validity of the measurement model, we can conclude the measurement scale is valid and reliable. Figure 3 presents the measurement model with standardized factor loadings for the 40 items.
To judge if all constructs are suitable for analysis, we adopted the covariance matrix method. The composite scores of the variables were computed by parceling the original measurement item scores. Table 10 presents the standard deviations, the means, the minimum and maximum values of constructs, which were measured on a 5-point Likert scale:
The mean is considered as an indicator of central tendency. The mean values in Table 10 reflect that apart from social influence and learning value, participants’ perception towards all other constructs, with mean values greater than the mid-point of 3, is above the average. Performance expectancy has the greatest mean value of 4.07 whereas social influence has the lowest mean value of 2.683.
The standard deviation serves as a dispersion index which shows the extent to which the values deviate from the mean. Among all the variables, performance expectancy has the greatest deviation from its mean (SD = 0.997), suggesting reasonably high variability in participants’ perception toward performance expectancy. Learning value has the smallest deviation from the mean (SD = 0.668), suggesting relatively low variability in participants’ perception toward performance expectancy. Figure 4 presents a graphical illustration of the mean and standard deviations of all constructs.
Table 11 presents the Pearson’s correlation matrix for all variables in the study. The results reveal predominantly positive and statistically significant correlations. On average, the correlations range from −0.095 to 0.489. As no correlation exceeds the threshold of 0.80 (Cheung and Lai, 2023), there is no evidence of multicollinearity among the variables.
Further, Table 12 demonstrates that all variance inflation factors (VIFs) are below 10 (Cheung and Chung, 2022). Consequently, the study does not encounter any issues related to multicollinearity.
After the measurement model had been validated, we applied the SEM technique on the data to evaluate the hypothesized relationships using IBM SPSS AMOS (version 20) software and maximum likelihood estimate (MLE) technique. We examined the causal effects of performance expectancy, effort expectancy, social influence, facilitating conditions, hedonic motivation, learning value, habit, trust and instructor characteristics on behavioral intention in the proposed model.
The goodness-of-fit indices reflect that the observed data adequately fits the model: χ2 = 907.938, df = 695, p-value = 0.000, GFI = 0.891, AGFI = 0.871, CFI = 0.976, TLI = 0.973, IFI = 0.977, RMSEA = 0.030 and χ2/df = 1.306. Though the chi-square results are statistically significant, this is acceptable as the sample size is large (Bagozzi et al., 1991). The R2 value for behavioral intention is 0.50, which suggests 50 percent of variations in behavioral intention can be explained by its nine determinants. The overall results show that the scores of R2 value meet the condition for the cut off value of 0.10 (Quaddus and Hofmeyer, 2007). Then, the coefficient parameter estimates are reviewed to examine the hypothesized causal relationships of the variables. The hypothesis results of causal relationships are shown in Table 13.
From the above table, except the two paths for hedonic motivation and habit on behavioral intention, all other paths from performance expectancy, effort expectancy, social influence, facilitating conditions, learning value, trust and instructor characteristics on behavioral intention are statistically significant with p-value less than the standardized significant level of 0.05. Thus, the hypotheses H1, H2, H3, H4, H6, H8 and H9 are supported while hypotheses H5 and H7 are rejected. The findings reflect that the strongest predictor of behavioral intention is trust with the standardized path coefficient of 0.204. The second strongest determinant is instructor characteristics with the standardized path coefficient of 0.202. Figure 5 depicts the path analysis results with the standardized coefficients.
H1: Performance expectancy has a positive effect on the Mainland Chinese postgraduate students’ behavioral intention to use LMSs by Hong Kong universities.
From Table 13, the critical ration (c.r.) and p-value of performance expectancy in predicting behavioral intention are 2.719 and 0.007 respectively. It reflects that the probability of having a critical ratio as large as 2.719 in absolute value is 0.007. As the regression weight for performance expectancy in predicting behavioral intention is significantly different from zero at the 0.01 level (two-tailed), H1 is supported. Moreover, the standardized estimate of Beta is 0.181, showing a positive relationship between performance expectancy and behavioral intention.
H2: Effort expectancy has a positive effect on the Mainland Chinese postgraduate students’ behavioral intention to use LMSs by Hong Kong universities.
The regression weight for effort expectancy in predicting behavioral intention is significantly different from zero at the 0.01 level (two-tailed). Thus, H2 is supported. In addition, the standardized estimate of Beta was 0.148, reflecting a positive association between effort expectancy and behavorial intention.
H3: Social influence has a positive effect on the Mainland Chinese postgraduate students’ behavioral intention to use LMSs by Hong Kong universities.
The regression weight for social influence in predicting behavioral intention is significantly different from zero at the 0.05 level (two-tailed). Therefore, H3 is supported. Moreover, the standardized estimate of Beta is 0.115, indicating a positive relationship between social influence and behavorial intention.
H4: Facilitating conditions has a positive effect on the Mainland Chinese postgraduate students’ behavioral intention to use LMSs by Hong Kong universities.
The regression weight for facilitating conditions in predicting behavioral intention is significantly different from zero at the 0.001 level (two-tailed). Thus, H4 is supported. Additionally, the standardized estimate of Beta is 0.181, providing evidence about the positive relationship between facilitating conditions and behavorial intention.
H5: Hedonic motivation has a positive effect on the Mainland Chinese postgraduate students’ behavioral intention to use LMSs by Hong Kong universities.
The finding indicates that there is no significant association between hedonic motivation and behavioral intention. The path coefficient is −0.016, c.r. is −0.293 and p-value is 0.770. Thus, H5 is rejected.
H6: Learning value has a positive effect on the Mainland Chinese postgraduate students’ behavioral intention to use LMSs by Hong Kong universities.
The regression weight for learning value in predicting behavioral intention is significantly different from zero at the 0.005 level (two-tailed). Thus, H6 is supported. Also, the standardized estimate of Beta is 0.118, suggesting a positive association between learning value and behavioral intention.
H7: Habit has a positive effect on the Mainland Chinese postgraduate students’ behavioral intention to use LMSs by Hong Kong universities.
The results show that there is no significant association between habit and behavioral intention. The path coefficient is 0.014, c.r. is 0.298, p-value = 0.766. Thus, H7 is rejected.
H8: Trust has a positive effect on the Mainland Chinese postgraduate students’ behavioral intention to use LMSs by Hong Kong universities.
The regression weight for trust in predicting behavioral intention is remarkably different from zero at the 0.001 level (two-tailed). Thus, H8 is supported. Moreover, the standardized estimate of Beta is 0.204, indicating a positive relationship between trust and behavioral intention.
H9: Instructor characteristics have a positive effect on the Mainland Chinese postgraduate students’ behavioral intention to use LMSs by Hong Kong universities.
The regression weight for instructor characteristics in predicting behavioral intention is significantly different from zero at the 0.001 level (two-tailed). Thus, H9 is supported. Besides, the standardized estimate of Beta is 0.202, demonstrating a positive relationship between instructor characteristics and behavioral intention.
For answering the first research question, nine exogenous variables are hypothesized as the motivators of behavioral intention, the only endogenous variable in this study, to use LMSs adopted by universities in Hong Kong. The regression model shows that 50% of the variation in Mainland Chinese postgraduate students’ behavioral intention is elucidated by the nine predictors. This is comparable to the UTAUT model proposed by Venkatesh et al. (2003) which explained between 17 percent and 53 percent of the variance in user intentions to use information technology.
The results show that seven out of nine hypotheses (H1, H2, H3, H4, H6, H8 and H9) are supported while two of them (H5 and H7) are rejected. The seven major factors, including performance expectancy, effort expectancy, social influence, facilitating conditions, learning value, trust and instructor characteristics, influence the Mainland Chinese postgraduate students’ experience and level of acceptance to use LMSs provided by Hong Kong institutions during the COVID-19 pandemic. They represent technology attributes (i.e., performance expectancy and effort expectancy), contextual factors (i.e., instructor characteristics, facilitating conditions and social influence) and individual perceptions on technology (i.e., trust and learning value).
For answering the second research question, we compared the effect of factors which influence the Mainland Chinese postgraduate students’ acceptance to use LMSs. The standardized estimate coefficients (beta) of the hypotheses are presented in Table 8. The higher the standardized estimate coefficients (beta), the stronger effect of the exogenous latent variables on behavioral intention to accept the use of LMSs. Except the two rejected hypotheses, the effects of exogenous variables are ranked according to their standardized estimate coefficient values from the strongest to the weakest: trust (0.204), instructor characteristics (0.202), facilitating conditions and performance expectancy (both 0.181), effort expectancy (0.148), learning value (0.118) and social influence (0.115).
The rapid shift of eLearning as well as reliance on available course information and task submission on LMS result in new ethical questions about online security and data privacy (García-Morales et al., 2021). In alignment with the results of Chao (2019), El-Masri and Tarhini (2017), Harja et al. (2020) and Lai et al. (2024), our study finds trust as the most important antecedent which influences the well-educated Mainland Chinese student participants’ intention to use LMSs in this research. China, as the largest data pool in the world, used to have grievous trust crisis related to privacy in different fields, with no exception in online learning context (Wang and Yu, 2015). The demand from Chinese citizens for improved data protection is strong (Roberts, 2021). The results of this study are in line with Wang and Yu (2015), which shows that well-educated adults possess strong awareness of data security and trust has direct effect on behavioural intention. However, our findings are in contrast to Eneizan et al. (2019) and Kabra et al. (2017), which identified no positive association between trust and behavioral intention in the mobile marketing and information system context respectively.
In line with most studies on eLearning technology (Lee et al., 2009; Prasetyo et al., 2021; Selim, 2007), instructor characteristics is identified as the second strongest motivator of behavioral intention among Mainland Chinese participants in this research. These findings differ from those of Ibrahim et al. (2017), which suggest no direct or indirect relationship between instructor characteristics and behavioral intention. During the pandemic, the sudden shift of traditional pedagogical approach to online teaching required much preparation by instructors, ranging from designing audio and visual materials, regular planning on duration of study hours, choosing correct assessment tools, to developing interactive and formative class activities for students’ peer collaboration (García-Morales et al., 2021). Under social isolation at the time of pandemic, instructors’ encouragement and motivation were highly correlated with participants’ LMS acceptance. Instructors’ enthusiasm in teaching course contents, encouraging students’ use of LMSs and inviting students to ask or answer questions via the cyberspace is a crucial factor which contributes to students’ positive perception of LMSs.
The findings reflect that technical support and participants’ technological knowledge are significant factors for intention of LMS use during social distancing. The problems associate with eLearning technologies, including downloading errors, issues with installation, login problems, audio and video problems, can discourage students from using LMSs (Dhawan, 2020). Mainland Chinese students may need to use a Chrome browser to enable full functionality of LMS. However, they may face challenges in installing Google Chrome, as access to Google services is often restricted within China. In alignment with the findings of recent studies (Wut et al., 2022; Zacharis and Nikolopoulou, 2022), our study reveals that technical support by universities, students’ resources, knowledge and skills for solving technical problems are vital for their LMS acceptance. This differs from the results by Prasetyo et al. (2021) and Raman and Thannimalai (2021), which suggest no positive relationship between facilitating conditions and behavioral intention during the pandemic.
Performance expectancy has been consistently found to be a strong motivator of behavioral intention in the educational context (Ahmed et al., 2022; Chao, 2019; Lai et al., 2024; Prasetyo et al., 2021; Raza et al., 2021). In line with prior studies, the correlation between performance expectancy and behavioral intention is found to be significant in this study. The implication is that the Mainland Chinese postgraduates are willing to use LMSs by Hong Kong universities as long as the platforms are perceived to be useful for improving their course results. This is not surprising since the online quizzes, polls, written and multimedia course materials on LMSs can help to accomplish their tasks efficiently. Communication channels such as built-in messaging systems and discussion forums can also facilitate academic discussions between instructors and students, which help to improve students’ learning outcomes (Green et al., 2014).
Some prior studies have argued that there is no direct relationship between effort expectancy and behavioral intention, as individuals’ acceptance of a technology is not solely determined by its ease of use but also its performance expectancy (Dindar et al., 2021; Hu et al., 2003). However, our study aligns with other prior studies which have suggested that effort expectancy has a remarkable impact on students’ behavioral intention to adopt eLearning technologies (Ahmed et al., 2022; Chao, 2019; Raza et al., 2021). The results of this study suggest that students’ behavioral intention is developed upon their positive understanding on LMSs’ easiness during the pandemic.
Prior to the pandemic, the world had already experienced substantial growth and adoption in educational technologies, with the online education market projected to reach USD 350 billion by 2025 (World Economic Forum, 2020). Further, the global COVID-19 crisis compelled universities to shift from traditional pedagogical approaches to eLearning. The onset of the pandemic resulted in a sudden and significant surge in the utilization of LMSs (Veluvali and Surisetti, 2022). This transition has led to rapid advancements in the technological infrastructure of education, and students have become increasingly familiar with the use of LMS. Students’ perception of the low degree of effort required to use the LMS contributes to a higher behavioral intention to utilize it. Given that the majority of survey participants were from the younger generation and resided in areas with acceptable internet connectivity, they generally found the well-developed LMSs implemented by Hong Kong universities to be effortless to use. In contrast, those who encountered difficulties in using the LMSs had a lower intention to adopt the system for learning during the pandemic.
Learning value pertains to the cognitive trade-off between the perceived value of LMSs, and the time and effort spent on exploring and adopting them (Ain et al., 2016). Since Mainland Chinese students devote much time and effort (including tuition fee) to enroll into postgraduate programs in Hong Kong, they have expectation on gaining reasonable benefits from using LMSs. Consistent with recent studies in education context (Ain et al., 2016; Harja et al., 2020; Prasetyo et al., 2021), the findings suggest significant impact of learning value on postgraduate student participants’ intention towards LMS use during pandemic. If they find the LMSs convenient and efficient while leading to the desired learning outcome, they have higher motivation to use them.
Social influence concerns user’s perception about the influence of important people. In line with the study conducted by Ahmed et al. (2022) in five different Asian countries (excluding China), the results reflect social influence is a determinant of LMS acceptance. The results also align with Ahmad et al. (2024) and Raman and Thannimalai (2021) which respectively report the positive influence of social influence on behavioral intention to adopt eLearning platforms and metaverse technology in education. Nonetheless, the predicting effect of social influence is the least significant among other determinants in our study. Traditionally, China has been regarded as a collectivistic society where individuals tend to rely on the opinions of their important peers when making decisions about technology adoption (Lee et al., 2013). However, due to the influence of globalization, the younger generation in China has been exposed to Western cultures and has embraced more individualistic values (Hsu and Barker, 2013). As suggested by Al-Adwan and Al-Debei (2024), there was no significant impact of social influence on the adoption of metaverse technology among young students from Generation Z. From Table 5, the majority of our samples fall within the same age group. Further, Prasetyo et al. (2021) found social influence an insignificant predictor of eLearning platform adoption among doctorate students. It is important to consider that our research was conducted on well-educated, young students during a period of social isolation. The influence of peers is not as significant as other important factors in our research findings.
Previous studies have found prior experience with technology use leads to persistent actions reflecting through behavioral intention of individuals (El-Masri and Tarhini, 2017; Masa’deh et al., 2016; Moorthy et al., 2019; Raman and Thannimalai, 2021). However, our finding suggests a contradictive result – habit plays an insignificant role for student participants’ intention of LMS use during the pandemic. As the majority of Mainland Chinese postgraduate students enrolled into one-year full-time taught postgraduate degree programs, it is reasonable for them to perceive their discontinuation of LMS use after graduation, regardless of the positive experience during study.
Hedonic motivation associates with intrinsic motivation such as fun, pleasure or enjoyment of using the system. Before the pandemic, hedonic motivation was frequently identified as a significant motivator of behavioral intention in educational context (Al-Gahtani, 2016; El-Masri and Tarhini, 2017). However, the finding of this study indicates hedonic motivation has no direct significant relationship with the acceptance of LMSs, which is consistent with other prior studies conducted during pandemic (Prasetyo et al., 2021; Raman and Thannimalai, 2021). One possible explanation is that as the use of LMSs became mandatory for accessing online classes (Chen et al., 2013), the effect of hedonic factors like fun and pleasure on behavioral intention became less significant for Mainland Chinese postgraduate students. When these students were required to use the LMSs consistently and repeatedly, their intention to use the systems was likely driven more by utilitarian factors, such as the systems’ functional capabilities and necessity for class participation, rather than by inherent enjoyment or entertainment value.
During pandemic, eLearning was the major mode of class delivery for sustainable learning. It is vital to identify the motivators of students’ acceptance towards the LMSs adopted by universities in Hong Kong for sustainable learning in case of future crisis. In conclusion, the answer to research question 1 reflects that the seven motivators, including performance expectancy, effort expectancy, social influence, facilitating conditions, learning value, trust and instructor characteristics, are significant factors which influence the Mainland Chinese postgraduate students’ acceptance on LMSs adopted and used by universities in Hong Kong. The two factors, hedonic motivation and habit, are not identified as important contributors to behavioral intention to use LMSs. According to the results to research question 2, among the seven exogenous variables, the top motivators of behavioral intention to use LMSs are trust, instructor characteristics, facilitating conditions and performance expectancy, followed by other factors such as effort expectancy, learning value and social influence respectively.
Since there is no relevant study about LMS adoption by Mainland Chinese postgraduate students during pandemic, this research contributes to the literature about technology acceptance model with expansion of a novel context. The findings provide inspirations about LMS use and possible improvements for eLearning. They offer valuable insights for educational stakeholders to promote the acceptance of LMS platforms as part of contingency plans, in preparation for potential future crises which necessitate a solely online mode of learning. They also highlight the acceptance factors of LMS among distance/online learners who adopt the cyberspace as the only means of learning.
For universities and educationalists, given that trust, instructor characteristics and facilitating conditions are the three strongest motivators of LMS acceptance by Mainland Chinese postgraduate students, more resources could be allocated to enhancing the security, guidance, and training of the LMSs and offering technical support to postgraduate students. Specifically, universities can conduct workshops for demonstrating LMS platforms in new student orientation and new instructor training and develop codes of conduct to ensure a safe and trustworthy virtual environment for eLearning, share information about privacy and data protection in LMS and send regular reminders about change of LMS password to students. In case of unexpected crises like the COVID-19 pandemic, more remote resources and timely support should be provided by instructors and technical staff to the affected students to maximize their intention to use LMSs by universities in Hong Kong. Universities should hire instructors with appropriate characteristics. For instance, when interviewing instructors, universities could ask questions to gauge the instructors’ encouragement of student usage of the LMS. This would help the universities better understand the instructors’ characteristics and approach to integrating the LMS into their teaching methods. Instructors’ characteristics could be used as one of the questions in teaching evaluation questionnaire survey which could be used as one of the appraisal criteria for instructors. Prospective Mainland Chinese postgraduate students who may study in Hong Kong or overseas can evaluate their own tendency to accept LMSs by tertiary institutions with reference to the strong motivators and the items in the measurement instrument for making education-related decisions. For example, those who give overwhelmingly low scores to the descriptions in the questionnaire might only consider programs providing much face-to-face support with minimal reliance on LMSs.
This study has certain limitations. First, there is limitation about the generalizability of the results. The population of the study may not be representative enough for all Mainland Chinese postgraduate students who enrolled into postgraduate programs provided by Hong Kong universities. Future investigations can encompass a broader range of participants, including a larger sample size that incorporates a more diverse population, including both males and mature students. Alternatively, researchers can further compare the predictors of LMSs acceptance between undergraduate and postgraduate students, between different genders and age groups. As our study adopted a cross-sectional research design, the data collected only reflect participants’ LMS use intention at the time of the survey. Future research can be conducted in form of longitudinal study to be held at students’ early stage of LMS use and at later stage after an extended period of time. Further, this study adopted a self-administered online questionnaire during pandemic in observation of social distancing measures. For future studies, researchers can incorporate focus groups and face-to-face interviews to explore students’ acceptance of LMS in the post-pandemic era.
The raw data supporting the conclusions of this article will be made available by the authors, without undue reservation.
The studies involving humans were approved by the Research Ethics Committee of Brittany University. The studies were conducted in accordance with the local legislation and institutional requirements. The participants provided their written informed consent to participate in this study.
CL: Conceptualization, Data curation, Formal analysis, Funding acquisition, Investigation, Methodology, Project administration, Resources, Software, Supervision, Validation, Visualization, Writing – original draft, Writing – review and editing. KC: Conceptualization, Formal analysis, Funding acquisition, Investigation, Methodology, Project administration, Resources, Software, Supervision, Validation, Visualization, Writing – original draft, Writing – review and editing. LP: Formal analysis, Methodology, Validation, Writing – review and editing.
The author(s) declare no financial support was received for the research, authorship, and/or publication of the article.
The authors declare that the research was conducted in the absence of any commercial or financial relationships that could be construed as a potential conflict of interest.
All claims expressed in this article are solely those of the authors and do not necessarily represent those of their affiliated organizations, or those of the publisher, the editors and the reviewers. Any product that may be evaluated in this article, or claim that may be made by its manufacturer, is not guaranteed or endorsed by the publisher.
Ahmad, S. A., Alsoud, M., Li, N., Majali, T., Smedley, J., and Habibi, A. (2024). Unlocking future learning: Exploring higher education students’ intention to adopt meta-education. Heliyon 10:E29544. doi: 10.1016/j.heliyon.2024.e29544
Ahmed, R. R., Štreimikienė, D., and Štreimikis, J. (2022). The extended UTAUT model and learning management system during COVID-19: Evidence from PLS-SEM and conditional process modeling. J. Bus. Econ. Manag. 23, 82–104. doi: 10.3846/jbem.2021.15664
Ain, N., Kaur, K., and Waheed, M. (2016). The influence of learning value on learning management system use: An extension of UTAUT2. Inform. Dev. 32, 1306–1321.
Ajzen, I. (2011). The theory of planned behaviour: Reactions and reflections. Psychol. Health 26, 1113–1127.
Al-Adwan, A. S., and Al-Debei, M. M. (2024). The determinants of Gen Z’s metaverse adoption decisions in higher education: Integrating UTAUT2 with personal innovativeness in IT. Educ. Inform. Technol. 29, 7413–7445.
Al-Gahtani, S. S. (2016). Empirical investigation of e-learning acceptance and assimilation: A structural equation model. Appl. Comput. Inform. 12, 27–50.
Bagozzi, R. P., and Yi, Y. (1988). On the evaluation of structural equation model. J. Acad. Market. Sci. 16, 74–94.
Bagozzi, R. P., Yi, Y., and Phillips, L. W. (1991). Assessing construct validity in organizational research. Admin. Sci. Q. 36, 421–458.
Burke, R. R. (2002). Technology and the customer interface: What consumers want in the physical and virtual store. J. Acad. Market. Sci. 30, 411–432.
Çera, G., Pagria, I., Khan, K.A., and Muaremi, L. (2020). Mobile banking usage and gamification: The moderating effect of generational cohorts. J. Syst. Inform. Technol. 22, 243–263.
Chakraborty, P., Mittal, P., Gupta, M. S., Yadav, S., and Arora, A. (2020). Opinion of students on online education during the COVID-19 pandemic. Hum. Behav. Emerg. Technol. 3, 357–365.
Chao, C. M. (2019). Factors determining the behavioral intention to use mobile learning: An application and extension of the UTAUT Model. Front. Psychol. 10:1652. doi: 10.3389/fpsyg.2019.01652
Chen, Y., Lin, Y., Yeh, R., and Lou, S. (2013). Examining factors affecting college students’ intention to use web-based instruction systems: Towards an integrated model. Turk. Online J. Educ. Technol. 12, 111–121.
Chen, Y., Wang, Y., and Kinshuk Chen, N. S. (2014). Is FLIP enough? Or should we use the FLIPPED model instead? Comput. Educ. 79, 16–27. doi: 10.1016/j.compedu.2014.07.004
Cheng, Y. C., Cheung, A. C. K., and Ng, S. W. (2015). Internationalization of higher education: The case of Hong Kong. Singapore: Springer.
Cheung, K. Y., and Chung, C. V. (2022). The impacts of audit committee expertise on real earnings management: Evidence from Hong Kong. Cogent Bus. Manag. 9:2126124.
Cheung, K. Y., and Lai, C. Y. (2022). External auditor’s trust and perceived quality of interactions. Cogent Bus. Manag. 9:2085366. doi: 10.1080/23311975.2022.2085366
Cheung, K. Y., and Lai, C. Y. (2023). The impacts of business ethics and diversity on ESG disclosure: Evidence from Hong Kong. J. Corporate Account. Finance 34, 208–221.
Cohen, J. (1983). The cost of dichotomization. Appl. Psychol. Meas. 7, 249–253. doi: 10.1177/014662168300700301
Dhawan, S. (2020). Online learning: A panacea in the time of COVID-19 crisis. J. Educ. Technol. Syst. 49, 5–22. doi: 10.1007/s11159-021-09889-8
Dindar, M., Suorsa, A., Hermes, J., Karppinen, P., and Näykki, P. (2021). Comparing technology acceptance of K-12 teachers with and without prior experience of learning management systems: A Covid-19 pandemic study. J. Comput. Assist. Learn. 37, 1553–1565. doi: 10.1111/jcal.12552
El-Masri, M., and Tarhini, A. (2017). Factors affecting the adoption of e-learning systems in Qatar and USA: Extending the unified theory of acceptance and use of technology 2 (UTAUT2). Educ. Technol. Res. Dev. 65, 743–763. doi: 10.1007/s11423-016-9508-8
Eneizan, B., Ali, G. M., Alhamzah, A., Anas, S. A., and Odai, E. (2019). Customer acceptance of mobile marketing in Jordan: An extended UTAUT2 model with trust and risk factors. Int. J. Eng. Bus. Manag. 11:1847979019889484. doi: 10.1177/1847979019889484
Ennew, C., and Sekhon, H. (2007). Measuring trust in financial services: The trust index. Consumer Policy Rev. 17, 62–68.
Fornell, C., and Larcker, D. F. (1981). Evaluating structural equation models with unobservable variables and measurement error. J. Market. Res. 18, 39–50.
García-Morales, V. J., Garrido-Moreno, A., and Martín-Rojas, R. (2021). The transformation of higher education after the COVID disruption: Emerging challenges in an online learning scenario. Front. Psychol. 12:616059. doi: 10.3389/fpsyg.2021.616059
Gefen, D., Karahanna, E., and Straub, D. (2003). Trust and TAM in online shopping: An integrated model. MIS Q. 27, 51–90.
Green, R. A., Farchione, D., Hughes, D. L., and Chan, S. P. (2014). Participation in asynchronous online discussion forums does improve student learning of gross anatomy. Anatom. Sci. Educ. 7, 71–76. doi: 10.1002/ase.1376
Hagedorn, R. L., Wattock, R. A., and Olfert, M. D. (2022). My entire world stopped: College students’ psychological and academic frustrations during the COVID-19 pandemic. Appl. Res. Q. Life 17, 1069–1090. doi: 10.1007/s11482-021-09948-0
Hair, J. F., Black, W. C., Babin, B. J., Anderson, R. E., and Tatham, R. L. (2019). Multivariate data analysis, 8th Edn. Boston, MA: Cengage.
Harja, Y. D., Irawan, M. I., and Ambarwati, R. (2020). Measure the significance of learning value and trust factors for online learning technology acceptance in Indonesia. IPTEK J. Technol. Sci. 31, 188–200.
Hoyle, R. H. (1995). “The structural equation modeling approach: Basic concepts and fundamental issues,” in Structural equation modeling, concepts, issues and applications, ed. R. H. Hoyle (Thousand Oaks, CA: Sage), 1–15. doi: 10.3389/fpsyg.2018.00532
Hsu, S. Y., and Barker, G. G. (2013). Individualism and collectivism in Chinese and American advertisements. Int. Commun. Gazette 75, 695–714. doi: 10.1177/1748048513482543
Ibrahim, R., Leng, N. S., Yusoff, R. C. M., Samy, G. N., Masrom, S., and Rizman, Z. I. (2017). E-learning acceptance based on technology acceptance model (TAM). J. Fundamental Appl. Sci. 9, 871–889.
Information Services Department (2022). HK sees 56k COVID-19 cases. Hong Kong: Information Services Department.
Kabra, G., Ramesh, A., Akhtar, P., and Dash, M. K. (2017). Understanding behavioural intention to use information technology: Insights from humanitarian practitioners. Telemat. Inform 34, 1250–1261. doi: 10.1016/j.tele.2017.05.010
Khalilzadeh, J., Ozturk, A. B., and Bilgihan, A. (2017). Security-related factors in extended UTAUT model for NFC based mobile payment in the restaurant industry. Comput. Hum. Behav. 70, 460–474.
Kline, R. B. (2010). Principles and practice of structural equation modeling. New York, NY: Guilford Press.
Lai, C. Y., Cheung, K. Y., Chan, C. S., and Law, K. K. (2024). Integrating the adapted UTAUT model with moral obligation, trust and perceived risk to predict ChatGPT adoption for assessment support: A survey with students. Comput. Educ. Artif. Intell. 6:1000246. doi: 10.1016/j.caeai.2024.100246
Lee, B. C., Yoon, J. O., and Lee, I. (2009). Learners’ acceptance of e-learning in South Korea: Theories and results. Comput. Educ. 53, 1320–1329.
Lee, S. G., Trimi, S., and Kim, C. (2013). The impact of cultural differences on technology adoption. J. World Bus. 48, 20–29.
Li, M., and Bray, M. (2007). Cross-border flows of students for higher education: Push–pull factors and motivations of mainland Chinese students in Hong Kong and Macau. High. Educ. 53, 791–818.
MacCallum, R. C., and Austin, J. T. (2000). Applications of structural equation modeling in psychological research. Annu. Rev. Psychol. 51, 201–226. doi: 10.1146/annurev.psych.51.1.201
Masa’deh, R. M. T., Tarhini, A., Bany, M. A., and Maqableh, M. (2016). Modeling factors affecting student’s usage behaviour of eLearning systems in Lebanon. Int. J. Bus. Manag. 11, 299–312.
Mei, B., Brown, G., and Teo, T. (2017). Toward an understanding of pre-service English as a foreign language teachers’ acceptance of computer-assisted language learning 2.0 in the People’s Republic of China. J. Educ. Comput. Res. 56, 74–104. doi: 10.1177/0735633117700144
Ministry of Education, the People’s Republic of China (2023). 2023 national educational work conference convenes in Beijing. Beijing: Ministry of Education, the People’s Republic of China.
Moorthy, K., Yee, T. T., T’ing, L. C., and Kumaran, V. V. (2019). Habit and hedonic motivation are the strongest influences in mobile learning behaviours among higher education students in Malaysia. Aust. J. Educ. Technol. 35, 174–191. doi: 10.14742/ajet.4432
Nunnally, J. C., and Bernstein, I. H. (1994). Psychometric theory, 3rd Edn. New York, NY: McGraw-Hill.
Podsakoff, P. M., Mackenzie, S. B., Lee, J. Y., and Podsakoff, N. P. (2003). Common method biases in behavioral research: A critical review of the literature and recommended remedies. J. Appl. Psychol. 88, 879–903. doi: 10.1037/0021-9010.88.5.879
Prasetyo, Y. T., Roque, R. A. C., Chuenyindee, T., Young, M. N., Diaz, J. F. T., Persada, S. F., et al. (2021). Determining factors affecting the acceptance of medical education eLearning platforms during the COVID-19 pandemic in the Philippines: UTAUT2 approach. Healthcare 9:780. doi: 10.3390/healthcare9070780
Quaddus, M., and Hofmeyer, G. (2007). An investigation into the factors influencing the adoption of B2B trading exchanges in small business. Eur. J. Inform. Syst. 16, 202–215.
Raman, A., and Thannimalai, R. (2021). Factors impacting the behavioural intention to use e-learning at higher education amid the covid-19 pandemic: UTAUT2 model. Psychol. Sci. Educ. 26, 82–93.
Raza, S. A., Qazi, W., Khan, K. A., and Salam, J. (2021). Social isolation and acceptance of the learning management system (LMS) in the time of COVID-19 pandemic: An expansion of the UTAUT model. J. Educ. Comput. Res. 59, 183–208.
Rehman, A. U., Bashir, S., Mahmood, A., Karim, H., and Nawaz, Z. (2022). Does e-shopping service quality enhance customers’ e-shopping adoption? An extended perspective of unified theory of acceptance and use of technology. PLoS One 17:e0263652. doi: 10.1371/journal.pone.0263652
Roberts, H. (2021). Informational privacy with Chinese characteristics. Dig. Ethics Lab Yearbook 2021, 9–23.
Selim, H. M. (2007). Critical success factors for e-learning acceptance: Confirmatory factor models. Comput. Educ. 49, 396–413.
Thompson, T. L., and MacDonald, C. J. (2005). Community building, emergent design and expecting the unexpected: Creating a quality eLearning experience. Internet High. Educ. 8, 233–249.
Veluvali, P., and Surisetti, J. (2022). Learning management system for greater learner engagement in higher education – A review. High. Educ. Future 9, 107–121.
Venkatesh, V., Morris, M., Davis, G., and Davis, F. (2003). User acceptance of information technology: Toward a unified view. MIS Q. 27, 425–478.
Venkatesh, V., Thong, J., and Xu, X. (2012). Consumer acceptance and use of information technology: Extending the unified theory of acceptance and use of technology. MIS Q. 36, 157–178.
Vyas, L., and Yu, B. (2018). An investigation into the academic acculturation experiences of Mainland Chinese students in Hong Kong. High. Educ. 76, 883–901. doi: 10.1007/s10734-018-0248-z
Wang, Z., and Yu, Q. (2015). Privacy trust crisis of personal data in China in the era of big data: The survey and countermeasures. Comput. Law Secur. Rev. 31, 782–792. doi: 10.1016/j.clsr.2015.08.006
World Economic Forum (2020). The Covid-19 pandemic has changed education forever. This is how. Cologny: World Economic Forum.
Wu, X. I., Bernadette, M. W., and Baker, S. C. (2024). The role of language use and communication. in Mainland Chinese students’ cross-cultural adaptation to Hong Kong: A qualitative investigative study. Lang. Intercult. Commun. 24, 20–34.
Wut, T. M., Lee, W., and Xu, J. (2022). How do facilitating conditions influence student-to-student interaction within an online learning platform? A new typology of the serial mediation model. Educ. Sci. 12:337.
Xiong, W., Mok, K. H., Ke, G., and Cheung, J. O. (2020). Impact of COVID-19 pandemic on international higher education and student mobility: Student perspective from Mainland China and Hong Kong. Int. J. Educ. Res. 105:101718. doi: 10.1016/j.ijer.2020.101718
Yu, B., and Zhang, K. (2016). ‘It’s more foreign than a foreign country’: Adaptation and experience of Mainland Chinese students in Hong Kong. Tertiary Educ. Manag. 22, 300–315. doi: 10.1080/13583883.2016.1226944
Yuan, K. H., Bentler, P. M., and Zhang, W. (2005). The effect of skewness and kurtosis on mean and covariance structure analysis: The univariate case and its multivariate implication. Sociol. Methods Res. 34, 240–258.
Keywords: adult learning, distance education, learning management system, mobile learning, eLearning, UTAUT2, SEM
Citation: Lai CY, Cheung KY and Pang LLL (2024) Examining the motivators affecting acceptance towards learning management systems for sustainable learning amid COVID-19 pandemic. Front. Educ. 9:1365258. doi: 10.3389/feduc.2024.1365258
Received: 04 January 2024; Accepted: 14 August 2024;
Published: 11 September 2024.
Edited by:
Yu-Chun Kuo, Rowan University, United StatesReviewed by:
Pinaki Chakraborty, Netaji Subhas University of Technology, IndiaCopyright © 2024 Lai, Cheung and Pang. This is an open-access article distributed under the terms of the Creative Commons Attribution License (CC BY). The use, distribution or reproduction in other forums is permitted, provided the original author(s) and the copyright owner(s) are credited and that the original publication in this journal is cited, in accordance with accepted academic practice. No use, distribution or reproduction is permitted which does not comply with these terms.
*Correspondence: Chung Yee Lai, dHJhY3l4NDEyOEBnbWFpbC5jb20=
Disclaimer: All claims expressed in this article are solely those of the authors and do not necessarily represent those of their affiliated organizations, or those of the publisher, the editors and the reviewers. Any product that may be evaluated in this article or claim that may be made by its manufacturer is not guaranteed or endorsed by the publisher.
Research integrity at Frontiers
Learn more about the work of our research integrity team to safeguard the quality of each article we publish.