- Department of Agricultural Economics, Kansas State University, Manhattan, KS, United States
Research on teaching and learning has demonstrated many benefits from team-and group-based learning for improving content comprehension and meeting learning outcomes in a wide range of disciplines. We help to expand on this research, by shedding additional light on the impact of group formation decisions on group performance during group learning activities in a higher education classroom setting. Our objective is to assess the impact of different group formation strategies, including random assignment, self-selection, and methods based on individual metrics, such as academic performance and individual skill levels. Using an experimental design approach, we find that GPA-based and math-ability based group formation strategies may provide some additional benefit to group performance on the margin when initially forming groups. Self-selection of groups may be more beneficial after students learn more about each other (e.g., skills sets and work ethic) during the progression of the course. Our study contributes to the literature by providing an assessment of different group formation strategies that are accessible to a wide range of instructors for small to large classes, as well as guidance on use of different group formation strategies within classes, specifically for classes with more quantitative and analytical tasks.
1 Introduction
There is a strong trend in higher education for instructors to continue to adopt strategies designed to promote active and experiential learning, as well as greater student engagement. Team-or group-based learning is one such strategy that has proven successful and has become widely adopted (Michaelson et al., 2008). This approach involves students working together in teams or groups to complete a learning activity that consists of preparation, application and assessment components (Michaelson et al., 2002). Research on teaching and learning has demonstrated many benefits from team-and group-based learning for improving content comprehension and meeting learning outcomes in a wide range of disciplines including science, education, economics and business (Haberyan, 2007; Hernandez, 2002; Mcinerney and Fink, 2003). Additionally, this approach has proven to be an effective way for students to practice and refine their ability to work in a team structure and communicate effectively with peers, which happens to be among the most important qualities that employers increasingly want in job candidates in the market (NACE, 2022).
While the benefits of team-based learning strategies are evident, a number of important concerns are still being raised by instructors with regards to team activity design in general and team composition and group dynamics in particular. For example, group composition may unfairly affect one group over another due to skills make-up, personality clashes, differing perspectives and levels of motivation of individual members (Livingstone and Lynch, 2000). Consequently, some important questions that need deeper examination are: What are the optimal strategies for forming learning and project groups? Should students be allowed to self-select into groups? Should students be assigned into groups by the instructor based on individual characteristics? Which configuration of individual characteristics (e.g., GPA, skills, gender, diversity, area of interest) within a group may result in higher group and individual performance? Answers to these questions can provide important and useful insights for instructors currently using or planning to incorporate team-or group-based learning methods in their courses and curriculums. Even with a large academic literature on group and team learning, instructors continue to assess these questions to the present (e.g., Connell et al., 2023; Fischer et al., 2023; Karimi and Manteufel, 2020;Samudra et al., 2024), as answers to these questions will be course and context dependent, differing across disciplines and educational settings.
Student learning within small groups has been well documented in the literature, but this can be enhanced through thoughtful construction of groups to help improve development of skills and learning of content within the group (Hwang et al., 2008; Moreno et al., 2012). Wang and Kojima (2018) explore the formation of groups using different characteristics from a survey of students to capture learning characteristics. They propose the use of mathematical programming tools to formulate the groups using this data. Hwang et al. (2008) and Moreno et al. (2012) both consider the use of genetic algorithms in formulating learning groups based on learning characteristics of the students and learning criteria set by the instructor. Graf and Bekele (2006) develop an ant colony optimization problem for the formation of heterogenous groups to help improve group performance based on personality traits and student performance characteristics. Romanow et al. (2020) examined group formation for a business intelligence class for group term projects. They based group formation on students’ technology skill set. The authors find that forming groups based on skill level helped to improve learning outcomes and performance on the group term project. Many of these approaches require significant data collection and analytical effort for developing groups, which may be prohibitive for instructors with heavy teaching loads, research programs, among other commitments. In addition, the approaches defined may be out of reach for instructors that do not have the prerequisite background to use more advanced quantitative techniques.
The purpose of this paper is to assess the impact of different group formation strategies on group performance of group activities in upper-level undergraduate courses. To achieve this objective, the study utilizes a classroom experimental design approach to assess the impact of alternative team compositions, based on random assignment, academic performance, background skills, and self-selection on group performance. We specifically limit the number of characteristics examined in order to research more practical approaches that a wider group of instructors could employ in small to large classroom settings. Group performance data is collected for two core upper division undergraduate classes in agricultural economics to assess the differences in group performance across treatment groups and in relation to a control group. Both of the courses selected (AGEC 501 – Data Analysis and Optimization and AGEC 599 – Food and Agribusiness Management Strategies) incorporate group-based activities that were modified accordingly for conducting the classroom experiment. AGEC501 uses group-based activities requiring students to apply quantitative skills (linear programming and regression techniques) and approaches to solve applied economics problems, while AGEC599 uses group-based activities to simulate real-life managerial decision-making process through semester-long strategic analysis and strategy formulation projects.
We contribute to the literature on this topic in two ways. First, we provide an assessment of different group formation strategies that are potentially accessible to a wide range of instructors. Second, we provide some guidance on the use of different group formation strategies within classes, specifically for classes with more quantitative and analytical tasks. We find that the GPA-based and math-ability based group formation strategies may provide some additional benefit to group performance on the margin when initially forming groups in quantitative and analytical classes. In addition, we find self-selection of groups may be more beneficial after students learn about each other (e.g., skills sets and work ethic) during the progression of the course.
2 Methods
2.1 Conceptual framework
We adopt the conceptual model of group developmental stages advanced by Tuckman and Jensen (1977) and reviewed by Bonebright (2010). The framework helps to identify the underlying pathways and mechanisms through which group assignment strategies can affect group performance, learning outcomes, and how group formational approaches can be beneficial. The Tuckman and Jensen (1977) revised model of small group development has five stages: (1) forming, (2) storming, (3) norming, (4) performing, and (5) adjourning. In the “forming” stage, group members establish relationships with each other, become familiarized with the task assigned, set ground rules and guidelines, and test boundaries for interpersonal communications. The second stage, “storming,” is characterized by interpersonal conflict, lack of unity in the group, and polarization, as the group learns to work together. The third stage, “norming,” is when the group begins to develop cohesion and a set of roles and norms for interacting, behaving, and completing tasks. The fourth stage, “performing,” is where the group finds cohesion and tasks get completed. In this stage, members may have defined roles, but the roles are flexible and functional. In the final stage, “adjourning,” separation occurs, and the task is completed (Bonebright, 2010; Tuckman and Jensen, 1977). In this study, we chose to specifically focus on the forming stage of group formation to assess its impact on group performance, which has a subsequent impact on the storming stage of group formation. We recognize that mechanisms and strategies exist that can help to improve engagement, dynamics, learning, and group performance in the norming, performing and adjourning stages (Borek and Abraham, 2018; Merlin et al., 2020; Srba and Bielikova, 2014), but that was beyond the scope of this study.
During the first stages of group formation, particularly the “forming” and “storming” stages, significant transaction costs of forming groups or participating in groups that are randomly assigned or are determined through self-selection may result. This can increase the time in these two stages before groups can move onto subsequent, potentially more productive, stages of the small group developmental process (Riebe et al., 2016). Transaction costs can include additional time getting to know (potential) group members and assessments of skill sets. This uncertainty can lead to additional conflict during the “storming” phase, due to conflicting ideas about the views of the expected operations in the small group due to what is called interpretive uncertainty (Weber and Mayer, 2014). Thus, random assignment of group member or group formation via self-selection by students may result in additional transaction costs and conflict during the “forming” and “storming” phases, especially at the beginning of the semester when students may not know or be very familiar with each other. If these transaction costs and conflict can be reduced, then more time can be devoted to the “norming” and “performing” stages of the small group development process, improving group performance.
Based on this conceptual framework, we examine a number of hypotheses related to how groups are formed during the forming stage of group development and the subsequent impact on group performance. These hypotheses include: (H1) group assignment based on grade-point average (GPA) of students improves overall group performance; (H2) group assignment based on math ability of students improves overall group performance; and (H3) group formation based on self-selection improves group performance only if students had prior opportunities to work together and learn about each other’s abilities. Hypotheses H1 and H2 relate to individual characteristics used to form balanced groups (e.g., achieving a mix of mathematical abilities) and are relative to group assignment based on random-assignment and self-selection of groups. Hypothesis H3 is based on the idea that if students had prior opportunities to work together and learn about each other’s abilities (e.g., small group activities have already been used in the class), then the transaction costs associated with self-selection will be reduced relative to random assignment. Group performance is measured by the group grade earned on the group term project and is measured at the group level. As mentioned earlier, assessments of group dynamics are left for future research and were beyond the scope of the current study.
2.2 Experimental approach
2.2.1 Courses, group projects, and sample
The study examined performance of small groups based on different group assignment treatments for two courses: AGEC501 Data Analysis and Optimization and AGEC599 Food and Agribusiness Management Strategies. These two courses provide an opportunity to assess our hypotheses in two courses that have different types of group learning projects. The primary data collected was obtained during the period 2014 to 2017. While the data was collected prior to the pandemic, we feel the results obtained here are still relevant, as similar research questions to those addressed in this study are still being asked in the academic teaching literature related to undergraduate education (e.g., Connell et al., 2023; Fischer et al., 2023; Karimi and Manteufel, 2020; Samudra et al., 2024). In addition, current and past students (e.g., millennials) have worked in groups and teams in their classes as a norm over the past two decades (Kendall et al., 2014). Thus, we believe the results from this study are still pertinent for group formation approaches in undergraduate classrooms today.
2.2.1.1 AGEC501 data analysis and optimization
This is a junior/senior level course required for all undergraduate majors in the Department of Agricultural Economics. The course introduces students to two quantitative methods used in agricultural economics, mathematical programming and regression analysis. The first 8 weeks of the course focus on methods and application of mathematical programming (e.g., linear programming) to agricultural and agribusiness problems and the final 7 weeks of the course focus on methods and applications of linear regression to agricultural economics, farm management and agribusiness problems. The course was taught by one of the co-authors in which the study was conducted for two semesters in Fall 2014 and Fall 2015. In 2014, the course had 44 students (1 sophomore, 9 juniors, 33 seniors, and 1 graduate), of which 19% were female, 93% were between the ages of 20 to 24, and 2 were not majoring in agricultural economics or agribusiness. The average cumulative GPA of students was 3.2 with a standard deviation of 0.6. In 2015, the course had 68 students (1 sophomore, 13 juniors, 53 seniors, and 1 graduate student), of which 28% were female, 93% were between the ages of 20 to 24, and 1 was not majoring in agricultural economics or agribusiness. The average cumulative GPA of students was 3.2 with a standard deviation of 0.5.
For the math programming and regression sections of the course, students had to complete a group term project that applied the skills that they learned. The group term project for the mathematical programming portion of the course (LP term project) required groups to build and solve a linear programming model in EXCEL for an applied case study problem provided by the instructor. In addition, groups were required to write a 3-page report that explained the problem, presented the linear programming model developed, and discussed the results and implications of the solution found by the linear programming model. Case studies examined applied problems related to product mix, logistic, farm management, financial, and scheduling problems. The group term project for the regression portion of the course (regression term project) required students to develop a lecture that could be presented to the class on an applied regression problem related to crop production, livestock production, or an agribusiness problem. The project required students to develop a Power Point lecture that introduced the topic chosen, presented a relevant economic approach to analyzing the chosen problem, presented relevant regression and statistical concepts, and presented an applied example with data. The applied example portion of the project required students to find their own data and work through a regression problem in EXCEL using the regression concepts taught in the course. For both years in which the course was taught, the group term projects represented 30% of a student’s final grade, 15% for each term project. Students were not graded individually but received a group grade for each term project. For the group projects, students were placed in groups of 3 (with 1 group of 2). The groups for each group term project were different.
2.2.1.2 AGEC599 food and agribusiness management strategies
This is a senior level capstone course required for all agribusiness majors in the Department of Agricultural Economics. The course is designed to prepare students for professional endeavors that demand skills in critical thinking, strategic analysis, and managerial decision-making. Students learn how the economic principles underscore strategic management concepts and frameworks used in strategic analysis and strategy formulation. The course content draws on knowledge from multiple sources including a textbook, business case studies, real-world examples, and guest lectures by agribusiness executives. Learning activities include lectures, in-class discussions, strategic analysis of real-world agribusiness firms, strategic plan presentations and evaluations. Student learning is assessed through homework, exams, individual and group assignments, and term project reports. The course was taught by one of the co-authors in which the study was conducted for three semesters in Spring 2015, Spring 2016, and Spring 2017. In 2015, the course had 68 students (1 juniors and 67 seniors), of which 26% were female, all students were between the ages of 20 to 24, and all students were majoring in agricultural economics or agribusiness. The average cumulative GPA of students was 3.2 with a standard deviation of 0.5. In 2016, the course had 65 students (6 juniors and 59 seniors), of which 28% were female, 97% were between the ages of 20 to 24, and all students were majoring in agricultural economics or agribusiness. The average cumulative GPA of students was 3.2 with a standard deviation of 0.5. In 2017, the course had 70 students (8 juniors and 62 seniors), of which 41% were female, all students were between the ages of 20 to 24, and 1 was not majoring in agricultural economics or agribusiness. The average cumulative GPA of students was 3.3 with a standard deviation of 0.4.
The group project in AGEC 599 involves a series of business research activities, written reports, and oral presentations. At the beginning of the semester, students are assigned to groups with four or five members in each team. Each group is assigned a business case study describing an agribusiness company that is facing a significant strategic issue. The groups are responsible for applying concepts learned in class to conduct a Strategic Analysis (SA) of the situation and based on their findings from the SA, formulate Strategic Plan (SP) designed to help improve the firm’s performance. The groups’ performance is assessed based on two sets of oral presentations and written reports. The first set of presentations and reports is on Strategic Analysis due around the midpoint of the semester and the second set of presentations and reports is on Strategic Plan due at the end of the semester. The group project represents 50% of students’ total grade in the course, with 20% allocated to the SA portion and 30% allocated to the SP portion. Students are not graded individually for the group project but received a group grade for each aspect of the project.
2.2.2 Study design
We examine four different treatments for assigning small groups in the two classes for the group projects described. The treatments included: (1) random assignment, (2) GPA-based, (3) math ability based, and (4) self-selection. This follows other experimental methods (e.g., use of treatments and a control) used in the classroom to assess the effects of group formation on student and group performance (Springer et al., 1999). Use of the group formation strategies adopted here as treatments for our study have been discussed in the literature (Chen and Gong, 2018; Donovan et al., 2018; Smith and Spindle, 2007; Vasquez et al., 2020). Each of the treatments are summarized in Table 1.
To help in forming the groups for each treatment, a survey was given to students in both courses at the beginning of the course. The survey was used to collect demographic information, GPA, academic rank, self-rating of student abilities (e.g., mathematical and quantitative), people they may like to work with in the course, and types of applied problems they may be interested in. This information was used to help with group and treatment assignments for the course and study. The study was reviewed, approved and found to be exempt by the IRB Committee for human subjects research at Kansas State University (IRB#7549). All participating students signed an informed consent document indicating the purpose of the project, potential risks and benefits, confidentiality, and that participation was voluntary.
To determine what students would be assigned to each treatment we used the following procedure. We first assigned each student in the class a random number using a uniform random number generator and then sorted the students based on the random number assigned. Given that we wanted an equal number of students and groups assigned to each treatment, we first assigned students to the self-selection treatment, given the nature of this treatment. This was done by taking a number of students at the top of the sorted list equal to the number of groups needed for this treatment and building the self-selected groups based on the survey responses for these students of who they would like to work with in the class. For example, for AGEC501 in 2014, there were 44 students, allowing for the formation of up to 15 groups, 3 of which were assigned to the self-selection treatment. These three groups were based on groups chosen by 3 randomly selected students in the class. The students put into these groups were removed from the sorted list. The remaining students were then randomly assigned to the remaining treatments so that an equal number of small groups could be formed for each treatment.
2.3 Data and statistical analysis
The dependent variable and variable of interest in our study is the overall grade received by a group for their group project. Thus, the outcome variable is measured at the group level and the group is our unit of observation. Group grades were reported as a percentage from 0 to 100. We pooled the data for all years for the analyses. For the AGEC501 course, there are two group projects, the LP term project and the regression term project. The LP term project dataset had 32 group observations with an average group score of 88.7%, standard deviation of 5.3%, and range of 76 to 95.6%. The Regression term project dataset had 37 group observations with an average group score of 86.9%, standard deviation of 5.1% and range of 77.0 to 98.7%. For the AGEC599 there was one group project with two parts, Project Part 1 and Project Part 2. The Project Part 1 dataset had 39 groups with an average group score of 94.1%, standard deviation of 2.8% and range of 87.3 to 100%. The Project Part 2 dataset had 39 groups with an average group score of 95.8%, standard deviation of 2.9% and range of 88 to 100%. As discussed in the results section, there was needed variation across group types and years in the data.
To examine the impact of the group assignment treatment on group performance we used linear regression (Greene, 2012). We estimated linear regressions for each term project assignment in AGEC501 and AGEC5099, resulting in four regression models. Given that random assignment was the control, we included binary variables (or fixed effects) to model the marginal effect of the other group treatments relative to the control as regressors in the regression models. In addition, to correct for potential correlation amongst groups in a given year, we estimated standard errors using a cluster robust estimator with the cluster being designated by the year the class was taught (Greene, 2012).
To assess if the differences in the project scores are statistically significant, we conduct two sets of hypothesis tests. Standard t-tests of the coefficient estimates on the treatment fixed effects provide a way to examine if the GPA-based, Math ability based, and Self-selection treatments were significantly different than the control treatment, random assignment (Greene, 2012). To test differences between the GPA-based, Math ability based and Self-selection treatments, we conduct Wald tests of the difference between the estimated coefficients of the treatments (Greene, 2012).
3 Results and discussion
Table 2 provides the average group grade as a percentage for each treatment for each term project in each of the classes examined. For AGEC501, grades differ by year and project type. In 2014, students performed better on the LP term project compared to the regression term project. In 2015, grades were similar between the two projects. In both years, the average grade for the LP term project for the GPA-based, Math ability-based, and Self-selection treatments were higher than the control (random assignment). In 2014, the GPA-based and Math ability-based treatments had higher averages than self-selection, another often used technique for group formation. In 2015 though, self-selection had the highest average grade. For the regression term project, in 2014 only the self-selection treatment average grade was higher than the control (random assignment), but in 2015 all the treatments resulted in higher average grades than the control.
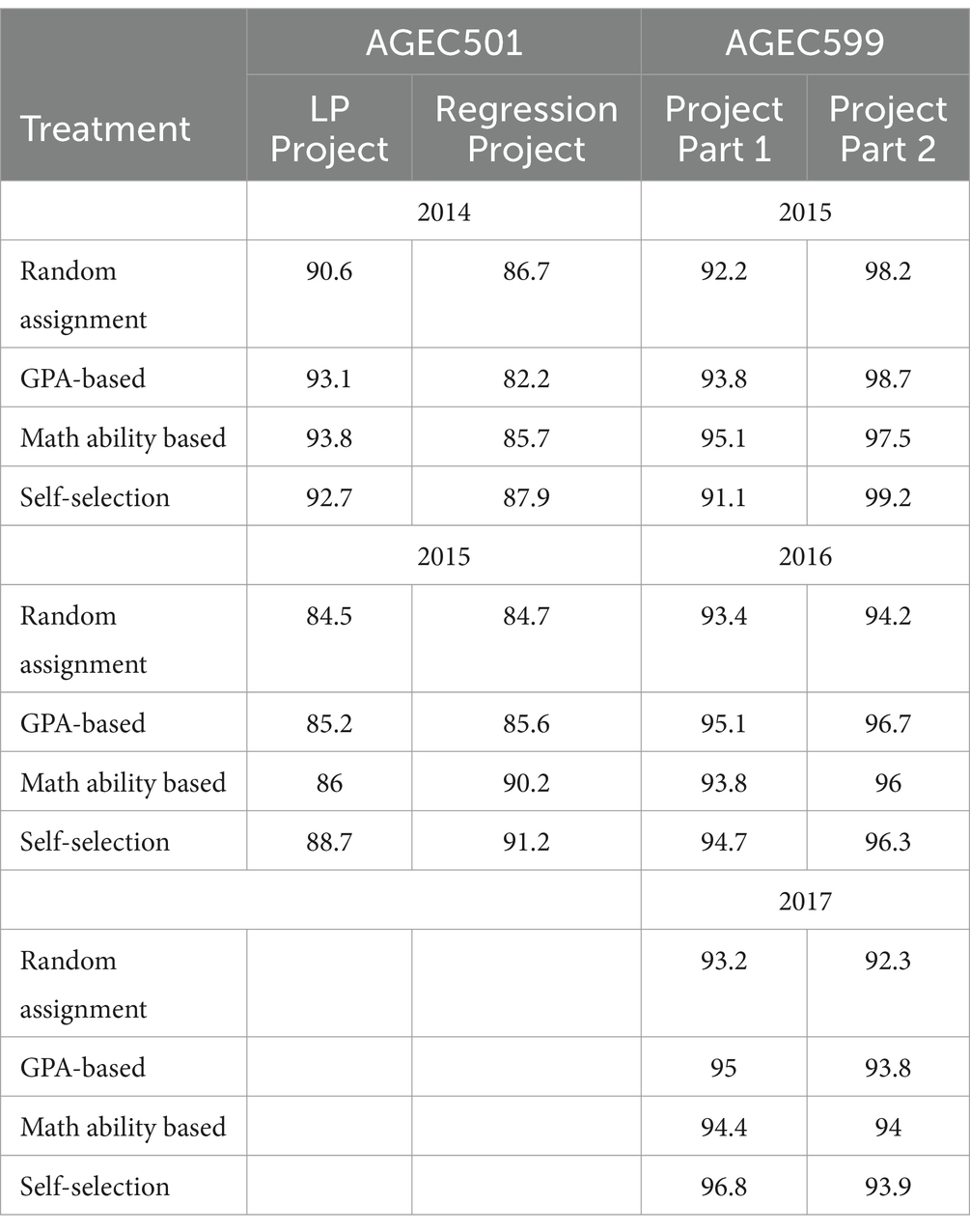
Table 2. Average group project grades as a percentage by group formation treatment for AGEC501 data analysis and optimization and AGEC599 food and agribusiness management strategies by year.
For AGEC599, for the first project, both in 2016 and 2017, all three treatments (GPA-based, Math ability based and Self-Selection) performed better on average than the control, random assignment. In 2015, the Self-Selection average group performance was lower than the random assignment control. These trends hold for the second part of the group project (Project Part 2), but in 2015 the Self-selected groups performed better on average than randomly assigned groups and groups formed based on math ability performed worse on average than those formed based on random assignment of group members. The differences in average group performance across treatment in 2015 may arise from differences in class make-up and classroom dynamics.
Table 3 reports estimation results for linear regressions of group project performance for each group project in each of the classes. Coefficient estimates on the treatment effects can be interpreted as the marginal change in the group grade relative to the control of random assignment. Table 4 reports p-values of t-tests and Wald tests used to test if treatment effects were significantly different from the control and between each treatment. p-values that are less 0.10 are bolded and indicate that the estimates obtained are significantly different at the 10 percent level of significance.
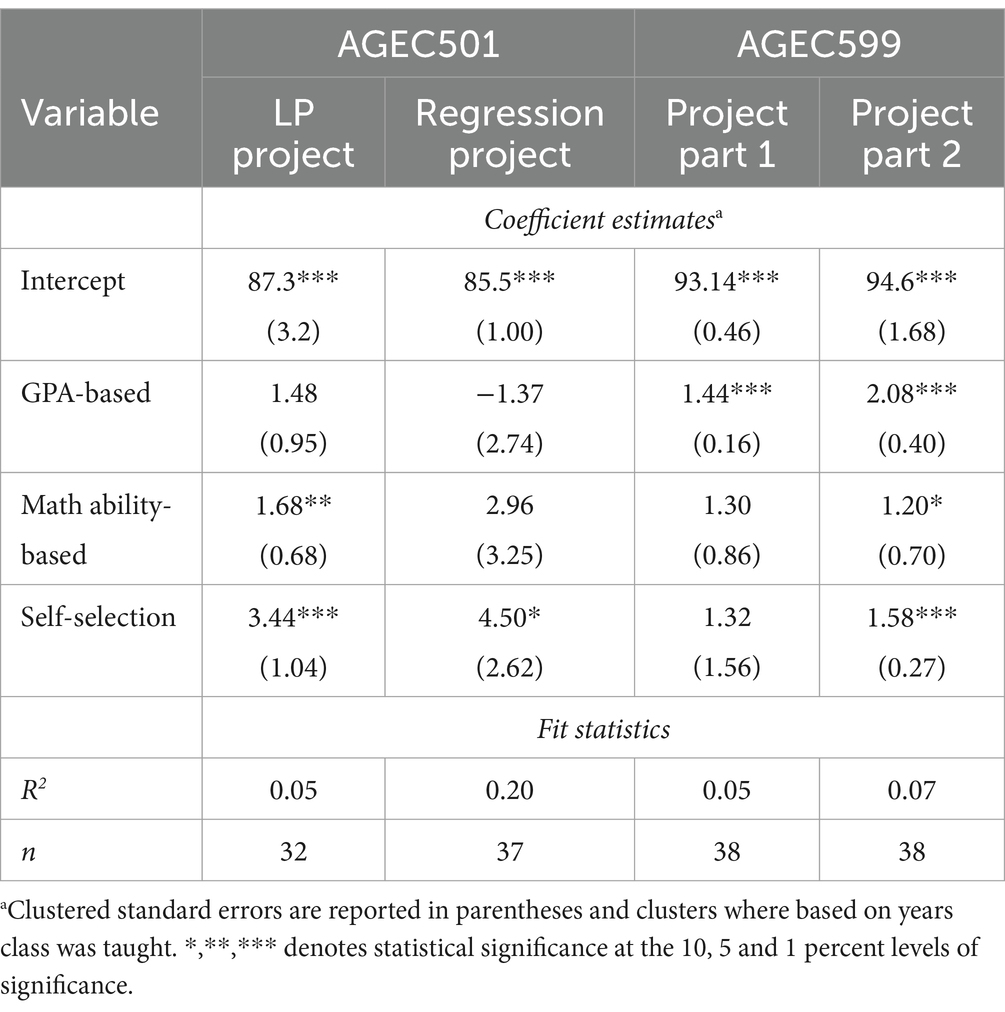
Table 3. Linear regression estimation results and fit statistics for group projects for AGEC501 data analysis and optimization and AGEC599 food and agribusiness management strategies.
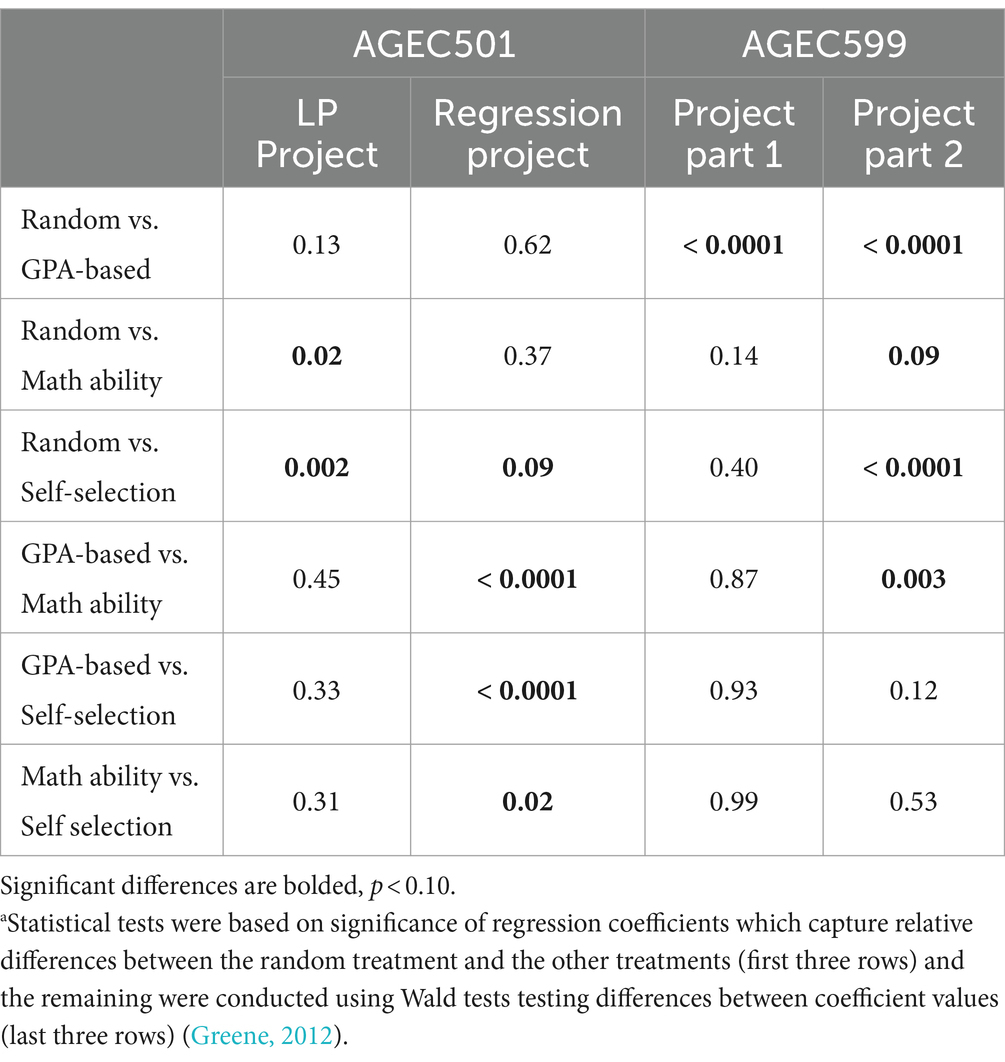
Table 4. P-values of statistical tests examining differences between group formation treatments using linear regression estimation results for group projects in AGEC501 data analysis and optimization and AGEC599 food and agribusiness management strategiesa.
For AGEC501, regression results and statistical tests indicate that for the LP project, over the 2 years, the Math ability-based and Self-selection treatments resulted in statistically significant higher grades than those groups formed using random assignment by approximately 1.7 and 3.4%, respectively. No statistical difference was found between the GPA-based, Math ability-based, and Self-Selection treatments. For the regression term project, the Self-Selection treatment resulted in a statistically higher grade by approximately 4.5% relative to having groups randomly assigned. These results provide support for hypothesis H3, as the regression term project came after the linear term project during the semester. That is, self-selected groups formed after an initial group project in the class performed better than random assignment, and possibly even better relative to the GPA-based and math-based treatments. This result may arise due to the permanence for group members in self-selected groups for the second term project in the class (Samudra et al., 2024). That is, the self-selected groups for the regression term project may have taken advantage of knowing who they work well with in the class and chose to continue to work with those students.
Groups formed based on math ability and self-selection had grades approximately [2.96 – (−1.37)] = 4.3% and [4.5 – (−1.37)] = 5.9% higher than groups formed using a student’s GPA. In addition, the groups formed using self-selection had grades that were (4.50–2.96) = 1.5% (statistically significant at a 5% level) higher than those formed using students’ math ability. Based on results for AGEC501, there is evidence in support of H2, but evidence for H1 is less clear. Our results are comparable to those in the literature. Moreno et al. (2012) found that forming groups based on group member traits and skill levels resulted in better group grades for a problems-based exercise in a computer programming class, similar to the type of term project used in AGEC501. This finding was relative to performance using more traditional group formation methods of random assignment and self-selection.
For AGEC599, for the first part of the term project in the course only the GPA-based formed groups had a grade that was statistically greater than the control (by 1.4%). Grades from groups formed using non-control treatments were not statistically different from each other at a 10% level of significance. For the second part of the group term project in AGEC599, the story changes. The GPA-based, Math ability-based and Self-selected groups performed statistically better than randomly assigned groups by approximately 2.1, 1.2 and 1.6%, respectively. When comparing the three treatments against each other, only the GPA-based group formations performed statistically better when compared to groups formed using math ability (by 0.7%). The results in AGEC599 do provide some evidential support for hypotheses H1 and H2. Chen and Gong (2018) find that group selection based on self-selection or traits/skills resulted in better overall group performance when compared to randomly assigned groups. Their study examined a group project in a large undergraduate class at the National University of Singapore. The group project was worked on throughout the class and represented a significant portion of the students’ grade, similar to the group project for AGEC599.
4 Conclusion
We examine the impact of different group formation treatments on group performance for group projects in two upper-level undergraduate courses at a land grant university. We examined different group formation strategies, including random assignment as a control, GPA-based, math ability-based, and self-selection. We sought to test three hypotheses and found that group formation based on GPA, math-ability, and self-selected based groups can improve group performance, which has been confirmed in other studies examining instructor-based group formation strategies (e.g., Vasquez et al., 2020). In addition, self-selected groups may perform better than the other treatments for group projects that are formed after initial group projects have been conducted in a class.
From the research conducted there are a few key takeaways. First, group performance for initial group projects in a course (e.g., at the beginning of the semester) may benefit from being based on student attributes, such as GPA or math ability, or social networks (i.e., self-selection). Such an approach helps to reduce transaction costs to students, helping to minimize time and potential conflicts during the forming and storming phases of group formation. Second, when forming groups, after initial group projects, assignments or activities have taken place, (i.e., later on in a semester), instructors may consider groups self-selecting. Results here support the benefits of this, as the self-selected groups for the regression term project (the second group project that semester) in AGEC501 performed better than the other treatments on average. In this situation, students may have formed social networks that allow them to assess other students’ abilities and build relationships that substantially lower the transaction costs and processes associated with the forming and storming phases of group formation. Premo et al. (2022) and Gašević et al. (2013) indicate the importance of learning about your classmates through your progression of courses and social ties that form through social networks in group and class performance. Third, we provide an approach that allows for group formation based-off readily available or surveyable data, rather than more complex group formation approaches proposed in the literature. While we do provide a math programming model for helping with group formation that can be implemented, instructors could adopt this approach putting pen to paper with some simple calculations to achieve the same results.
There do exist some limitations to the study conducted that should be taken into consideration, as well as suggestions for future research. The study does examine junior/senior level core agricultural economics courses at a land grant university. While there are some diffferences in student rank and experience, past studies have found at times that this may not always result in significant differences in performance (Springer et al., 1999). Results may differ though in other courses, such as in the humanities or natural sciences. Application of the approaches utilized in this study may be dependent on learning, cultural, educational, and social contexts. Thus, results should be interpreted in light of differences across these contexts (Smith and Spindle, 2007). We do expect though that some of the findings here will still be pertinent for instructors to consider for group composition when using group activities in their courses. The cited literature, which examines some of these other settings, supports the findings in this study. Differences in learning, cultural, educational and social contexts provide different avenues for future research, as well. We did not fully explore the impact of group composition or dynamics on group performance, which is another important factor and an area for future research. Past literature has shown that diverse and purposeful group composition and internal group dynamics can have a positive impact on student learning, engagement and performance. In addition, other practical methods for forming groups that have low transaction costs (e.g., reduced time in and out of class for setting-up groups, no requirement for significant data collection, data is readily available through a learning management system if needed, group formation procedures are clear, etc.), are not too computationally or time intensive, and improve group performance, such as student interests, personality metrics, amongst others, should continue to be explored. Our study adds to this growing literature by providing a practical and useful way to form groups in a course that we feel has lower transaction costs than other proposed methods suggested in the literature. In addition, our approach can help improve group functioning and performance at the beginning of a semester or term by helping to reduce the transaction costs for students in working as a group in the forming and storming phases of group formation.
Data availability statement
The raw data supporting the conclusions of this article will be made available by the authors, without undue reservation.
Ethics statement
The studies involving humans were approved by the Institutional Review Board, University Research Compliance Office, Kansas State University. The studies were conducted in accordance with the local legislation and institutional requirements. The participants provided their written informed consent to participate in this study.
Author contributions
JB: Conceptualization, Data curation, Formal analysis, Investigation, Methodology, Writing – original draft, Writing – review & editing. AS: Conceptualization, Data curation, Formal analysis, Investigation, Methodology, Writing – original draft, Writing – review & editing.
Funding
The author(s) declare financial support was received for the research, authorship, and/or publication of this article. Partial funding for this study came from a Scholarship of Teaching and Learning Mini-Grant, College of Agriculture, Kansas State University, Manhattan, KS, United States.
Acknowledgments
The authors would like to thank all undergraduate students who participated in our project and the support of the College of Agriculture at Kansas State University.
Conflict of interest
The authors declare that the research was conducted in the absence of any commercial or financial relationships that could be construed as a potential conflict of interest.
Publisher’s note
All claims expressed in this article are solely those of the authors and do not necessarily represent those of their affiliated organizations, or those of the publisher, the editors and the reviewers. Any product that may be evaluated in this article, or claim that may be made by its manufacturer, is not guaranteed or endorsed by the publisher.
Supplementary material
The Supplementary material for this article can be found online at: https://www.frontiersin.org/articles/10.3389/feduc.2024.1362211/full#supplementary-material
References
Bonebright, D. A. (2010). 40 years of storming: a historical review of Tuckman’s model of small group development. Hum. Resour. Dev. Int. 13, 111–120. doi: 10.1080/13678861003589099
Borek, A. J., and Abraham, C. (2018). How do small groups promote behavior change? An integrative conceptual review of explanatory mechanisms. Appl. Psychol. Health Well Being 10, 30–61. doi: 10.1111/aphw.12120
Chen, R., and Gong, J. (2018). Can self-selection create high-performing teams? J. Econ. Behav. Organ. 148, 20–33. doi: 10.1016/j.jebo.2018.02.004
Connell, G. L., Donovan, D. A., and Theobald, E. J. (2023). Forming groups in a large-enrollment biology class: group permanence matters more than group size. CBE Life Sci. Educ. 22. doi: 10.1187/cbe.22-08-0172
Donovan, D. A., Connell, G. L., and Grunspan, D. Z. (2018). Student learning outcomes and attitudes using three methods of group formation in a nonmajors biology class. CBE Life Sci. Educ. 17. doi: 10.1187/cbe.17-12-0283
Fischer, M., Rilke, R. M., and Yurtoglu, B. B. (2023). When, and why, do teams benefit from self-selection? Exp. Econ. 26, 749–774. doi: 10.1007/s10683-023-09800-2
Gašević, D., Zouaq, R., and Janzen, R. (2013). “Choose your classmates, your GPA is at stake!”: the association of cross-class social ties and academic performance. Am. Behav. Sci. 57, 1460–1479. doi: 10.1177/0002764213479362
Graf, S., and Bekele, R. (2006). “Forming heterogeneous groups for intelligent collaborative learning systems with ant colony optimization” in Intelligent tutoring systems. Proceedings of the 8th international conference, ITS 2006. Jhongli, Taiwan, June 26–30, 2006. Lecture Notes in Computer, vol. 4053 (Berlin, Heidelberg: Springer), 217–226.
Haberyan, A. (2007). Team-based learning in an industrial/organizational psychology course. N. Am. J. Psychol. 9, 143–152.
Hernandez, S. A. (2002). Team learning in a marketing principles course: cooperative structures that facilitate active learning and higher level thinking. J. Mark. Educ. 24, 73–85. doi: 10.1177/0273475302241009
Hwang, G. J., Yin, P. Y., Hwang, C. W., and Tsai, C. C. (2008). An enhanced genetic approach to composing cooperative learning groups for multiple grouping criteria. J. Educ. Technol. Soc. 11, 148–167.
Karimi, A., and Manteufel, R. D. (2020). Student study group and design team formation based on academic performance metrics. J. High. Educ. Theory Practice 20, 139–153.
Kendall, C., Etheredge, J., Moody, D., and Ashley, C. (2014). Group projects with millennials: the question of not why … but how. J. Learn. High. Educ. 10, 53–58.
Livingstone, D., and Lynch, K. (2000). Group project work and student-centered active learning: two different experiences. Stud. High. Educ. 25, 325–345. doi: 10.1080/713696161
Mcinerney, M. J., and Fink, L. D. (2003). Team-based learning enhances long-term retention and critical thinking in an undergraduate microbial physiology course. Microbiol. Educ. 4, 3–12. doi: 10.1128/me.4.1.3-12.2003
Merlin, M. D., Lavoie, S., and Gallagher, F. (2020). Elements of group dynamics that influence learning in small groups in undergraduate students: a scoping review. Nurse Educ. Today 87:104362. doi: 10.1016/j.nedt.2020.104362
Michaelson, L. K., Knight, A. B., and Fink, L. D. (2002). Team-based learning: a transformative use of small groups. Westport, CT: Praeger Publishers.
Michaelson, L. K., Sweet, M., and Parmelee, D. X. (2008). Team-based learning: small-group Learning’s next big step, new directions for learning. Hoboken, NJ: Wiley Periodicals, Inc.
Moreno, J., Ovalle, D. A., and Viccari, R. M. (2012). A genetic algorithm approach for group formation in collaborative learning considering multiple student characteristics. Comp. Educ. 58, 560–569. doi: 10.1016/j.compedu.2011.09.011
NACE . (2022). Job Outlook 2022: Spring Update. Available at: https://www.naceweb.org/store/2021/job-outlook-2022/ (Accessed November 1, 2023).
Premo, J., Wyatt, B. N., Horn, M., and Wilson-Ashworth, H. (2022). Which group dynamics matter: social predictors of student achievement in team-based undergraduate science classrooms. CBE–Life Sciences Education 21. doi: 10.1187/cbe.21-06-0164
Riebe, L., Girardi, A., and Whitsed, C. (2016). A systematic literature review of teamwork pedagogy in higher education. Small Group Res. 47, 619–664. doi: 10.1177/1046496416665221
Romanow, D., Napier, N. P., and Cline, M. K. (2020). Using active learning, group formation, and discussion to increase student learning: a business intelligence skills analysis. J. Inf. Syst. Educ. 31, 218–231.
Samudra, S., Walters, C., Williams-Dobosz, D., Shah, A., and Brickman, P. (2024). Try before you buy: are the benefits to a random trial period before student choose their collaborative teams? CBE Life Sci. Educ. 23. doi: 10.1187/cbe.23-01-0011
Smith, J. L., and Spindle, R. M. (2007). The impact of group formation in a cooperative learning environment. J. Account. Educ. 25, 153–167. doi: 10.1016/j.jaccedu.2007.09.002
Springer, L., Stanne, M. E., and Donovan, S. S. (1999). Effects of small-group leaning on undergraduates in science, mathematics, engineering, and technology: a meta-analysis. Rev. Educ. Res. 69, 21–51. doi: 10.3102/00346543069001021
Srba, I., and Bielikova, M. (2014). Dynamic group formation as an approach to collaborative learning support. IEEE Trans. Learn. Technol. 8, 173–186. doi: 10.1109/TLT.2014.2373374
Tuckman, B. W., and Jensen, M. A. C. (1977). Stages of small-group development revisited. Group Organiza. Stud. 2, 419–427. doi: 10.1177/105960117700200404
Vasquez, E. S., Dewitt, M. J., West, Z. J., and Elsass, M. J. (2020). Impact of team formation on teamwork effectiveness and performance in an upper-level undergraduate chemical engineering laboratory course. Int. J. Eng. Educ. 36, 491–501.
Wang, J., and Kojima, K. (2018). Exploring an approach for grouping through predicting group performance from analysis of learner characteristics. Paper presented at the 2018 7th international congress on advanced applied informatics, Yonago: IEEE, Japan, July, vol. 8-13, 2018 Available at: https://ieeexplore.ieee.org/abstract/document/8693462 (Accessed October 1, 2023).
Keywords: group formation, group project, higher education, linear programming, student performance
Citation: Bergtold JS and Shanoyan A (2024) Assessment of group formation methods on performance in group-based learning activities. Front. Educ. 9:1362211. doi: 10.3389/feduc.2024.1362211
Edited by:
Alfonso Garcia De La Vega, Autonomous University of Madrid, SpainReviewed by:
Eva Jereb, University of Maribor, SloveniaCarlos C. Goller, North Carolina State University, United States
Copyright © 2024 Bergtold and Shanoyan. This is an open-access article distributed under the terms of the Creative Commons Attribution License (CC BY). The use, distribution or reproduction in other forums is permitted, provided the original author(s) and the copyright owner(s) are credited and that the original publication in this journal is cited, in accordance with accepted academic practice. No use, distribution or reproduction is permitted which does not comply with these terms.
*Correspondence: Jason S. Bergtold, bergtold@ksu.edu