- 1Department for Chemistry Education, University of Duisburg-Essen, Essen, Germany
- 2Paluno – The Ruhr Institute of Software Technology, University of Duisburg-Essen, Essen, Germany
- 3Kekulé-Institute of Organic Chemistry and Biochemistry, University of Bonn, Bonn, Germany
- 4Department for Organic Chemistry, University of Duisburg-Essen, Essen, Germany
A convincing e-learning system for higher education should offer adequate usability and not add unnecessary (extraneous) cognitive load. It should allow teachers to switch easily from traditional teaching to flipped classrooms to provide students with more opportunities to learn and receive immediate feedback. However, an efficient e-learning and technology-enhanced assessment tool that allows generating digital organic chemistry tasks is yet to be created. The Universities of Bonn and Duisburg-Essen are currently developing and evaluating an e-learning and technology-enhanced assessment tool for organic chemistry. This study compares the effectiveness of traditional paper-pencil-based and digital molecule-drawing tasks in terms of student performance, cognitive load, and usability—factors that all contribute to learning outcomes. Rasch analysis, t-tests, and correlation analyses were used for evaluation, revealing that the developed system can generate digital organic chemistry tasks. Students performed equally well on simple digital and paper-pencil molecule-drawing tasks when they received an appropriate introduction to the digital tool. However, using the digital tool in two of three studies imposes a higher extraneous cognitive load than using paper and pencil. Nevertheless, the students rated the tool as sufficiently usable. A significant negative correlation between extraneous load and tool usability was found, suggesting room for improvement. We are currently concentrating on augmenting the functionality of the new e-learning tool to increase its potential for automatic feedback, even for complex tasks such as reaction mechanisms.
1 Introduction
In recent decades, the success and dropout rates in higher education have attracted particular interest (Aulck et al., 2016; Fischer et al., 2021; Fleischer et al., 2019; Heublein et al., 2020). For chemistry students, prior knowledge (in addition to satisfaction with the study content) is especially important for success (Fischer et al., 2021; Fleischer et al., 2019). It is therefore particularly important to promote the knowledge of students with little prior knowledge. This can be achieved by providing principle-based (feedback that provides learners with an explanation of why an answer is right or wrong, rather than just informing them whether an answer is right or wrong), just-in-time feedback (Eitemüller et al., 2023). In addition to knowledge of content, mastery of representations (e.g., routine use of skeletal formula) is essential for understanding organic chemistry. Representational competence mediates the relationship between prior knowledge and content knowledge in organic chemistry (Dickmann et al., 2019). Research indicates that students face challenges in producing representations at a very basic level such as drawing the correct molecule when given an IUPAC (International Union of Pure and Applied Chemistry) name (Bodé et al., 2016; Farhat et al., 2019) and connecting representations with relevant concepts (e.g., Anzovino and Lowery Bretz, 2016; Asmussen et al., 2023; Graulich and Bhattacharyya, 2017). Instructional support that promotes the integration of explanations and representations can enhance learning outcomes (Rodemer et al., 2021). Furthermore, the duration and level of previous chemistry education has been found to be a significant predictor for representational competence at university (Taskin et al., 2017).
Organic chemistry can be very complex, and hence, demands a high degree of the learners’ working memory capacity to cope with it. To prevent cognitive overload, additional extraneous load, e.g., due to the processing of unfavorable instructions should be avoided to not impair learning (Paas and Sweller, 2014). Therefore, an e-learning system with sufficient usability that does not initiate extraneous processing through confusing operation is needed to enhance learning, by enabling learners to work independently on tasks at their own pace and receive personalized and timely error-related feedback. This type of learning system would present learners who have lower prior knowledge with an opportunity to keep up with those who have higher prior knowledge while providing all learners with more opportunities to practice the skills they have acquired. Additionally, implementing such an e-learning system could facilitate a shift from traditional courses that promote a one-size-fits-all approach to a flipped classroom, where students have more opportunities to engage in dialogue, receive feedback, and interact with educators (van Alten et al., 2019). However, an effective non-commercial and adequate e-learning and e-assessment tool that can be integrated into learning platforms such as Moodle or Ilias, allows teachers to create digitally those typical organic chemistry tasks they need for their courses, can evaluate student responses automatically and provide explanatory feedback; and whose handling does not induce extraneous cognitive load, that is, offers good usability, is still missing. This paper offers insights into the primary stages of the development and evaluation of an e-learning and e-assessment tool for organic chemistry. First, we summarize the findings of related studies. Next, we present an overview of the functionality of the developed learning tool. Then, we describe the design of the evaluation studies before presenting their results, and finally, discuss our findings.
1.1 Challenges in learning organic chemistry
Students’ prior knowledge significantly predicts their success in chemistry studies (Fischer et al., 2021; Fleischer et al., 2019). Although students’ knowledge of organic chemistry generally increases over the course of a semester, regardless of their prior knowledge (Averbeck, 2021), learners with low prior knowledge do not learn enough to catch up, so prior knowledge remains a significant predictor of course grade in basic organic chemistry courses. Thus, it is possible to identify students at a high risk of not passing the course through prior knowledge assessment (Hailikari and Nevgi, 2010). Research suggests that novices in organic chemistry tend to focus on surface structures of representations (such as geometric shapes) and therefore have difficulty establishing the relationships between structural features and concepts (Anzovino and Lowery Bretz, 2016). Furthermore, dependence on rote learning instead of meaningful learning (Grove and Lowery Bretz, 2012) and focus on the surface level of representations (Graulich and Bhattacharyya, 2017) appear to be problematic.
An analysis of students’ difficulties in predicting products or comparing mechanisms using a modified version of Bloom’s taxonomy provides an overview of the various types of difficulties that students encounter (Asmussen et al., 2023). While difficulties with factual knowledge are limited to the cognitive process of remembering, difficulties with conceptual knowledge arise in the cognitive processes of remembering, understanding, applying, analyzing, and evaluating. If students encounter difficulties in analyzing concepts, they may not be able to identify the relevant parts of a molecule or deduce relevant concepts from the representation. Consequently, even if information is depicted, students may not be able to use it because of their inability to comprehend it. Contrary to the other difficulty categories, this category leads to no or incorrect solutions for the task. Thus, it is essential to encourage students to use representations as useful tools, instead of viewing them as a burden. An analysis of student quizzes at the beginning of an advanced organic chemistry course revealed that approximately one-third of the students encountered challenges in drawing Lewis structures based on the name of the molecule. Of the errors detected, the prominent ones included (1) failure to draw a Lewis structure, instead of opting for some structural formula that often violated the octet rule, and (2) drawing molecules with incorrect carbon chains of varying lengths (Farhat et al., 2019). Further evidence suggests that students struggle with fundamental skills in organic chemistry, such as identifying functional groups, drawing molecules from their IUPAC names, and drawing meaningful structures that adhere to the octet rule (Bodé et al., 2016). Evidence shows that representational competence mediates the relationship between prior and content knowledge in organic chemistry (Dickmann et al., 2019). Research also suggests that instructional support, which facilitates the integration of explanations and representations, mediates learning outcomes (Rodemer et al., 2021). Furthermore, the duration and level of previous chemistry education has been found to be a significant predictor for representational competence at university (Taskin et al., 2017).
In summary, although representations are crucial in organic chemistry, evidence suggests that students face difficulties in using and comprehending basic representations. Consequently, students experience problems when attempting more advanced tasks such as reaction mechanisms, as these rely on the ability to use and comprehend representations. Previous studies have demonstrated the importance of prior knowledge for achieving success in the field of organic chemistry. It is thus crucial for students lacking prior knowledge to receive adequate instructional support to bridge their knowledge gaps and prevent them from dropping out.
This paper uses tasks that ask students to draw molecules based on their IUPAC names to explore the differences between paper-based and digital tasks as part of an initial evaluation of an e-learning and e-assessment tool that is currently being developed
1.2 Cognitive load and schema construction
Cognitive Load Theory concerns learning domains with high intrinsic cognitive load (Paas et al., 2005) and considers the limitation of working memory as a critical factor in creating learning materials (Sweller et al., 1998). Learners acquire cultural knowledge that has to be acquired with conscious effort, such as organic chemistry by actively constructing mental models incorporating previously attained knowledge and presented information (Paas and Sweller, 2014). Acquired knowledge can be stored in long-term memory without time or quantity limitations in the form of cognitive schemata. Schemata serve to organize not only pieces of information, but also the relations between them. Basic schemata can be combined to form more complex schemata (Paas et al., 2003a). Unlike long-term memory, working memory has a strictly limited capacity, meaning that only a few pieces of new information can be processed simultaneously in working memory (Paas and Sweller, 2014). Despite its complexity, a schema that transfers from long-term memory into working memory is considered a single element; therefore, it is not limited by working-memory constraints (Sweller et al., 1998). Hence, schemata not only organize and store knowledge, but also serve to relieve working memory. Within a given domain, experts and novices diverge in the progression and automation of domain-related schemata (Sweller, 1988). Experts use highly developed and automated schemata that enable them to perform complex tasks. Consequently, to acquire cultural knowledge such as organic chemistry, one should aim to develop coherent and highly automated cognitive schemata.
For the successful acquisition of cultural knowledge, a sufficient cognitive load during learning is crucial (Paas et al., 2005). The processing of new information in the working memory induces a cognitive load (Paas and Sweller, 2014). Cognitive load theory distinguishes between the intrinsic cognitive load that arises from learning content and the extraneous load that results from unfavorable processing with regard to learning (Paas and Sweller, 2014; Sweller, 2010; Sweller et al., 1998; Sweller et al., 2019). Intrinsic cognitive load is determined by the number of elements that must be processed simultaneously and their interrelatedness. In the domain of organic chemistry, remembering the structural formula of ethanol may overload the working memory capacity of someone who is completely unfamiliar with chemistry, chemical representations, and structural formulas. Without a cognitive schema that assigns chemistry-specific meaning to the representation, one would need to process every letter and line and their spatial arrangement in the working memory to draw the structural formula for ethanol when requested. An organic chemistry expert can rely on a number of fully automated chemistry-specific cognitive schemata that include knowledge about the alkyl group and hydroxy group, element symbols, the octet rule that informs about plausible and implausible bonding, limitations of the structural formula as a form of representation, and other unrepresented information such as the three-dimensional arrangement of molecular components, hybridization, and possibilities for rotation around sigma bonds. Therefore, learners’ prior knowledge plays a crucial role in their individual intrinsic cognitive load. Higher prior knowledge allows learners to process more information simultaneously because they have already constructed fundamental schemata on which they can rely and expand, whereas learners with lower prior knowledge have fewer rudimentary or non-automated chemistry-specific schemata on which to rely (Paas et al., 2003b). For effective learning, it is essential that the learning material is not overly complex and aligns with the learner’s prior knowledge to prevent an overwhelming intrinsic cognitive load. In the field of organic chemistry, learners need a good grasp of specific concepts such as the representation of atoms by element symbols, the octet rule, basic functional groups, hybridization, and molecular orbitals, which enable them to draw, for example, the Lewis structures of organic molecules without risking intrinsic cognitive overload by trying to remember meaningless letters and strings. These schemata are necessary to advance further skills such as understanding and predicting reaction mechanisms.
Extraneous cognitive load arises from the inappropriate processing of learning material, such as providing unnecessary and distracting information or misdirecting learners’ attention. An example of distracting information is information on the effects of alcohol intake on humans when asked to recall the name and draw the Lewis structure of the corresponding molecules of the first 10 primary n-alcohols. An example of distracting attention at this stage and in the context of the task might be informing learners about alternative representations or about the inaccurate representations of the bond angle by the structural formula. As the extraneous cognitive load competes with the intrinsic cognitive load, reducing the extraneous cognitive load is crucial for successful learning in complex fields such as organic chemistry. Minimizing these distractions optimizes the load imposed on the working memory and releases the working memory capacity for schema construction and automation, which is essential for effective learning.
In summary, learning in complex domains, such as organic chemistry, induces a high intrinsic cognitive load. Learners require working memory, which is limited, to proceed with the intrinsic load. After the initial construction of the cognitive schemata, learners also need time to practice and automate their schemata. Additional extraneous load can impair learning (Paas and Sweller, 2014). The present study therefore investigates the cognitive load of students when working on tasks in which molecules are to be drawn on the basis of their IUPAC names.
1.3 Requirements for an effective e-learning system
As previously explained, learning environments must strive to minimize learners extraneous load. This is also true for e-learning tools, where such a load can result from non-intuitive handling, splitting attention as a consequence of multiple or overlapping windows, or other technical hindrances to the solution of the primary task. Therefore, an adequate learning environment must provide sufficient usability. Additionally, e-learning systems should present further opportunities for learners to practice newly acquired skills and thus promote schema automation. An e-learning system that automatically evaluates students’ responses and provides feedback beyond that on knowledge of results can enhance learning by offering explanatory feedback that provides principle-based explanations (Johnson and Priest, 2014). Explanatory feedback supports learning by (1) informing learners about the correctness of their answers, (2) identifying gaps in their existing knowledge, and (3) creating opportunities to adjust mental models. One advantage of e-learning systems over traditional written homework is the opportunity to offer immediate feedback to learners rather than delayed feedback after the teacher has corrected the homework. Empirical studies suggest that students value immediate feedback (Malik et al., 2014). Another benefit of an e-learning system over presenting a sample solution in class is its ability to provide principle-based feedback for errors that students have actually made instead of discussing common errors or misunderstandings. Eitemüller et al. (2023) demonstrated this advantage in a general chemistry course, in which students who received individual error-specific feedback outperformed those who received only corrective feedback, after adjusting for prior knowledge. Moreover, an e-learning system enables students to study independently and take advantage of individually paced learning sessions and the option to pause if necessary. Several studies on the segmenting principle have revealed that in addition to presenting meaningful segments that aid in organizing information, pausing and providing extra time to process information enhances learning (Mayer and Pilegard, 2014; Spanjers et al., 2012).
In summary, an e-learning system with adequate usability and a low extraneous cognitive load can facilitate learning by enabling learners to work individually on tasks at their own pace and receive personalized real-time feedback on errors. This will provide learners who have low prior knowledge with a chance to catch up with those who have higher prior knowledge, and thereby promote schema automation by presenting opportunities to practice. Additionally, an e-learning platform can support the transformation of conventional courses that rely on uniformity into flipped classrooms, which encourages increased opportunities for questioning, feedback, and interaction between students and teachers (van Alten et al., 2019). This article presents the results of initial evaluation studies carried out as part of the development of an e-learning tool for organic chemistry.
1.4 Digital learning tools for organic chemistry
A digital learning environment for organic chemistry should offer opportunities to create typical tasks for the subject and allow an automatic evaluation of student responses. Presently, transferring manually drawn molecular structures to computerized systems remains impossible (Rajan et al., 2020). Hence, digital learning tools for organic chemistry are restricted to using images for multiple-choice items (Da Silva Júnior et al., 2018) or must offer the possibility of digitally drawing molecules. Efforts have been made to develop such a platform (Malik et al., 2014; Penn and Al-Shammari, 2008). For example, the University of Massachusetts and University of Kentucky, which collaborated with Pearson, developed such a tool (Chamala et al., 2006; Grossman and Finkel, 2023). Another example is orgchem101, an e-learning tool offered by the University of Ottawa, which consists of modules that can stand alone or be combined with courses (Flynn, 2023; Flynn et al., 2014). Meanwhile, the University of California has developed the Reaction Explorer, which is currently distributed by Wiley PLUS (Chen and Baldi, 2008, 2009; Chen et al., 2010; WileyPLUS and University of California, 2023). However, no adequate, non-commercial e-learning and e-assessment tools currently exists that can be integrated into learning platforms such as Moodle or Ilias. This tool should allow teachers to create digitally their own organic chemistry tasks necessary for their courses, automatically assess students’ responses, provide explanatory feedback, and not induce an extraneous cognitive load through its use, thus providing good usability.
1.5 Development goals and research questions
The Universities of Bonn and Duisburg-Essen are currently developing and evaluating an e-learning and e-assessment tool for organic chemistry.
Hence, our goal (G) is to develop and evaluate an e-learning and e-assessment tool with the following characteristics:
G1: Is non-commercial,
G2: Can be integrated into learning platforms like Moodle or Ilias,
G3: Allows teachers to create digitally organic chemistry tasks that are typical and needed for their courses,
G4: Can evaluate student responses automatically, provide explanatory feedback, and offer students additional opportunities to study at their own pace, and
G5: Does not induce an extraneous cognitive load due to its handling, thus providing good usability.
The development of the tool followed the instructional path that students take when learning organic chemistry. Therefore, we developed a digital tool that can perform simple drawing tasks for organic molecules. This study’s objective was to evaluate the tool and establish guidelines for the implementation of complex tasks involving chemical reaction equations, mechanisms, and the transformation of molecule A to molecule B. It evaluated the (1) performance of students on simple molecule-drawing tasks using paper and pencil versus the digital tool, (2) perceived cognitive load of the students, and (3) usability of the tool. Goals 3 and 4 were therefore not the subject of this first evaluation study.
We examined the following research questions (RQ):
RQ1: To what extent are students’ abilities to solve digital molecule-drawing tasks comparable with their abilities to solve paper-pencil-based molecule-drawing tasks?
RQ2: To what extent do cognitive load ratings for digital molecule-drawing and paper-pencil-based molecule-drawing tasks differ?
RQ3: How do students rate the usability of the developed tool?
We assume that the digital format has several characteristics that can be decisive for learning. We therefore assume that a comparative media approach (Buchner and Kerres, 2023) can be helpful in gaining valuable insights into changing processes. We therefore explicitly do not use the comparative media approach based on a purely technology-centered understanding of teaching and learning. The first implication is that digital tasks necessitate the use of skeletal formulae rather than allow students to choose the formula they wish to use. The ability to use the skeletal formula without difficulty is a learning objective for a basic course in organic chemistry. The skeletal formula is a rather abstract representation in which a lot of essential information is not explicitly represented (e.g., carbon and hydrogen atoms or free electron pairs), compared to the valence structure formula or the Lewis structure, for example. This level of abstraction can pose a challenge to novice learners who struggle to read this representation. In addition, learners challenged by the task may be overwhelmed by the additional requirement of using the tool rather than drawing their ideas directly. Moreover, the use of a digital tool could lead to a tool-driven solution (e.g., avoiding repeated switching between required functions such as the option to draw single bonds or the option to insert heteroatoms). It is not necessary to mentally plan the drawing in paper format. Learners can solve tasks here in a content-driven way by developing their drawing step by step along the name of the molecule. Furthermore, the digital tool evaluated in this study lacks a notepad function for drawing or elaborating components before assembling them. Additionally, there is no option to cross off already-named components. Finally, the digital tool automatically adds or removes hydrogen atoms if the octet rule is violated, which can confuse students who are not aware of the violation and distract them from drawing. Hence, we considered that several aspects relevant to learning and performance differ between media and therefore assumed that the media comparison approach would be helpful in investigating whether learning or performance differs between media. Moreover, because we assumed that any differences in media could potentially affect cognitive load, we decided to collect data on cognitive load. Notably, any potential differences in performance or cognitive load are not a direct result of the medium used but rather of the changes in task processing presented above. Should this study yield evidence of disparities in students’ abilities or cognitive load across different media, further investigation is necessary to establish which of the aforementioned factors is responsible for these differences (as the media itself is not regarded as a causal factor).
2 The e-learning and e-assessment tool called JACK
JACK (Striewe, 2016) is an e-learning and e-assessment tool developed at the University of Duisburg-Essen. Unlike general learning management systems (LMS) that are designed to perform general tasks related to running courses (e. g. group formation, dissemination of learning materials, provision of simple quizzes), JACK is specialized for conducting formative and summative assessments with complex assessment items and individual, instant feedback. Nevertheless, JACK can be integrated into any LMS that supports the Learning Tools Interoperability (LTI) Standard. To do so, teachers define learning activities within the LMS that actually serve as links to the JACK server. Students clicking on these links automatically get logged in to JACK and forwarded to the set of exercises or assignments defined by the teacher. Elaborate feedback is displayed directly in JACK, while result points can also be reported back to the LMS for further processing.
To develop an e-learning and e-assessment environment for organic chemistry, JACK was complemented with Kekule.js (Jiang et al., 2016), an open-access web-based molecular editor available under the MIT license. The editor is based on current web-technologies (i. e. HTML5) and compatible with all major browsers on desktop and mobile clients. This enhancement enables us to work on a new type of task (called: molecule) within JACK, which allows the drawing of organic molecules in skeletal formulas and automatic checking of whether a student’s response is correct. Teachers can create molecule-based tasks using JACK. For instance, these tasks can require learners to draw a single molecule (e.g., “Draw the molecule described by the following IUPAC-name: (S,S)-pentane-2,4-diol,” Figure 1, upper left) or multiple molecules (e.g., “Draw all isomers of 1,2-dibromocyclohexane, including stereoisomers”). To evaluate student responses automatically, teachers can provide a sample solution by drawing the expected molecules in a Kekule.js window in the feedback section of JACK. Feedback for correct (e.g., “Your answer is correct.”; Figure 1, bottom right) and incorrect (“Unfortunately, your answer is incorrect. Please try again.”) answers as students respond may be included. E-learning teachers can also record further optional feedback rules; for example, to check student responses for typical mistakes (e.g., whether a student has drawn (R,S)-pentane-2,4-diol, Figure 1, upper right, or just pentan-2,4-diol without providing further information regarding stereochemistry) and provide principle-based feedback to their students to correct typical mistakes (Johnson and Priest, 2014).
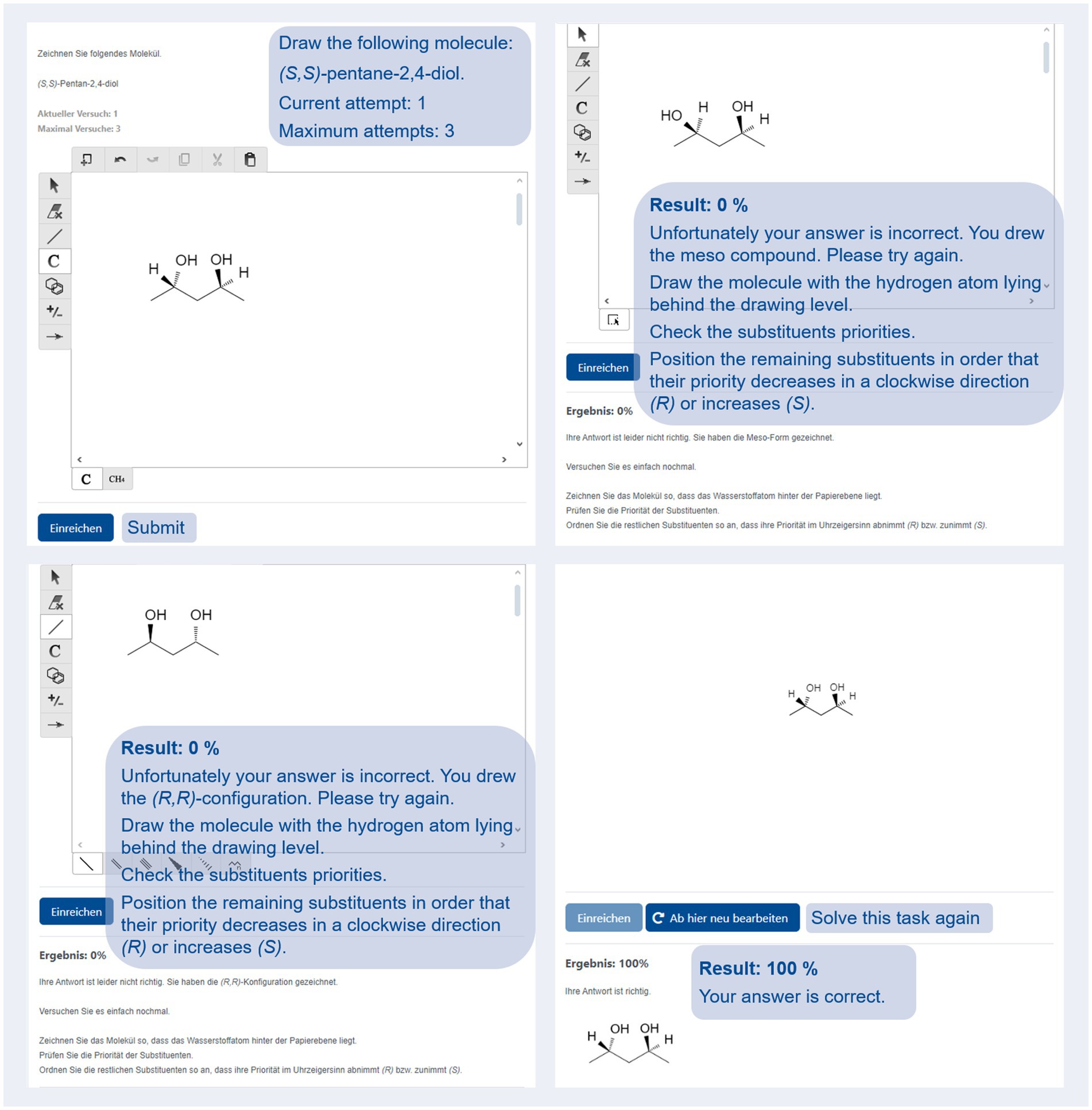
Figure 1. Screenshots from JACK. The figure shows a task (top left), two incorrect student answers with principle-based feedback (top right and bottom left), and a correct answer with a sample solution (bottom right). English translation of the originally German text is presented in blue.
The recorded sample solution and student responses are transformed from graphical information (drawn molecules) into InChI codes (Heller et al., 2015; IUPAC, 2023), which are standardized strings. The InChI code (international chemical identifier) derived from the student response is compared with the recorded sample solution and with additional feedback rules; in the case of correspondence, the respective feedback is provided to the student. If there is no correspondence, feedback for an incorrect solution is provided to the student. The transformation from graphical information to InChI allows comparing the essential features of molecules such as stereochemistry, and is independent of extraneous features such as writing direction. The original drawing is also stored in JACK in a machine-readable format. Hence, students and teachers can always inspect the original drawings when reviewing and discussing responses. In addition, JACK can further analyze the drawing to search for features that cannot be expressed in InChI codes, such as the arrangement of molecules around the reaction arrows.
Hence, integrating Kekule.js into JACK enables the design of tasks that require students to draw organic molecules, thus automatically comparing their responses to correct sample solutions or well-known mistakes, and providing feedback. JACK offers additional types of tasks such as fill-in-the-blanks and multiple-choice tasks, as well as various course settings to create appropriate learning or assessment courses.
3 Methods
This section discusses the development of the aforementioned e-learning and e-assessment tool and presents the framework for the evaluation studies.
3.1 Framework for the evaluation studies
The initial version of the tool was available in the summer of 2022 and underwent an initial evaluation. Throughout the subsequent year, the system became more sophisticated, following user feedback and feature requests from teachers. The e-learning mode of the tool (multiple attempts to solve the task, principle-based feedback, and knowledge of the results being available) was subsequently used for exercises in the beginner course on organic chemistry, and additional evaluations were conducted using the e-assessment mode (characterized by one attempt to solve the task, no principle-based feedback, and no knowledge of the results being available). All evaluation studies had the shared objective of examining (RQ1) to what extent students’ abilities to solve digital molecule-drawing tasks compare to their abilities to solve paper-pencil-based molecule-drawing tasks (RQ2) to what extent cognitive load ratings for digital molecule-drawing and paper-pencil-based molecule-drawing tasks differ, and (RQ3) if their usability ratings were satisfactory. Our assumption for the study was that students who have the ability to draw molecules based on their IUPAC names should perform equally well in both formats. Cognitive load ratings were interpreted as indicators of perceived demand. In other words, comparable cognitive load ratings were interpreted in such a way that digital molecule-drawing tasks are perceived as being as demanding as paper-pencil molecule-drawing tasks and do not induce any further (extraneous) load (which might not change performance but might have a negative impact, for example, on motivation). Satisfactory usability ratings are those that are at least above the midpoint of the scale and are interpreted to mean that students do not dislike the digital molecule-drawing tasks.
For all studies, students were asked to participate in class. Students were informed that their participation was voluntary and did not affect their course grades. They were also informed about data protection and the use of their data for scientific research, and any questions were answered prior to data collection. At the end of each study, students were given the opportunity to ask questions again. Although IRB approval was not necessary at German universities, the guidelines concerning the ethical scientific practice of the Federal German Research Foundation (DFG) were applied.
To compare students’ abilities to solve paper-pencil-based molecule-drawing tasks to digital molecule-drawing tasks, the following tasks in tandem with two very similar tasks were constructed. The digital task asks students to “Draw a 1,3-Dichloro-2-methylbutane molecule.” The corresponding paper-pencil-based task asks students to “Draw a 1,2-Dichloro-2-methylpropane molecule.” All tasks were originally constructed in German language. An overview of all IUPAC names used in study 4 is provided in Table 1. The students’ answers were coded as correct (1) or incorrect (0). In the case of the paper-pencil format, this coding was performed by the first author of this study. The coding for the digital format was automatically done by the developed system.
Cognitive load ratings were collected using nine-point, labeled rating scales. For the first, second, and fourth studies, a German adaptation of the intrinsic and extraneous load items provided by Leppink et al. (2013) was used (Table 2) to measure delayed two-dimensional cognitive load (Schmeck et al., 2015; van Gog et al., 2012; Xie and Salvendy, 2000) after completing all molecule-drawing tasks in one format (paper-pencil-based or digital). Owing to the unsatisfactory reliability of the two-dimensional cognitive load rating scales in the third study, immediate cognitive load measures were subsequently collected after each molecule-drawing task using a unidimensional cognitive load instrument (Kalyuga et al., 2001).
The usability ratings for the first and second studies were collected using nine-point, labeled rating scales and a German adaptation (Hauck et al., 2021) of the nine items originally provided by Brooke (1996). For the fourth study, a German version of the user experience questionnaire (UEQ, Laugwitz et al., 2008), which measures attractiveness (six items), perspicuity, efficiency, dependability, stimulation, and novelty (four items for each scale) using semantic differentials and seven-point rating scales, was administered.
The initial data analysis was performed using Winsteps (1-pl Rasch model, version 5.2.4.0; Boone et al., 2014). Data analysis was done separately for data from paper-pencil-based and digital material. Analyses in Winsteps were conducted in item-centered fashion. Rating scales were analyzed using a partial credit model. According to the Rasch model, there is a probabilistic relationship between the observed response behavior (item solution probability) and a latent characteristic (ability of the person) (Bond and Fox, 2007; Boone, 2016; Boone and Scantlebury, 2006). Within the Rasch model, the solution probabilities, ranging from 0 to 1, are transformed into logit values, having a value range from minus infinity to plus infinity (Boone and Staver, 2020; Boone et al., 2014). Typical measures range from +3 to −3. Easy items and people with lower personal abilities are indicated with negative values. Difficult items and individuals with higher person abilities are indicated with positive values (Boone et al., 2014; Linacre, 2023). In comparison to a raw sum score of correctly solved items, the person ability also considers whether the correctly solved items were easy or difficult items. An identical logit value for a person ability and an item difficulty corresponds to a 50% probability of solving an item by this person. A student who solves only very few and very easy items correctly will gain a low person ability. A student who solves the majority of items correctly and is even able to solve the difficult items will gain a high person ability. Hence, the person ability is a measure of how well a student performed. Person abilities were imported into IBM SPSS (26th version), and further analyses were performed (paired t-tests and correlation analyses). The Rasch analysis provides two reliability measures: person and item reliabilities. Person reliability is used to classify the sample. A person reliability below 0.8 in an adequate sample means that the instrument is not sensitive enough to distinguish between high and low person ability. The person reliability depends on the sample ability variance, the length of the test, the number of categories per item and the sample-item targeting. A high person reliability is obtained with a wide ability range, many items, more categories per item and optimal targeting. If the item reliability is below 0.8 and the test length is adequate, this indicates that the sample size or composition is unsuitable for a stable ordering of items by difficulty (Linacre, 2023). Item reliability depends on item difficulty variance and person sample size. A wide difficulty range and a large sample result in higher item reliability. The item reliability is widely independent of test length.
Please note that Rasch values for person abilities for drawing molecules and cognitive load ratings are contrary to each other. Students who have drawn a large number of molecules correctly have high positive person abilities with regard to drawing molecules. Students who reported low cognitive load in the cognitive load ratings have high negative person abilities for the cognitive load ratings.
For all analyses, we report bias-corrected and accelerated 95% bootstrap confidence intervals (BCa 95% CI) from 1,000 bootstrapped samples in square brackets (Field, 2018). The effect size d for repeated measures of one group (dRM, pooled) was calculated using the website statistica (Lenhard and Lenhard, 2023).
4 Results
4.1 Study 1
The first evaluation study was conducted at the end of the summer of 2022. University students (22 chemistry B.Sc. and water science B.Sc. majors taking the obligatory basic organic chemistry course, which only used paper-pencil-based exercises) were first asked to complete eight paper-pencil-based molecule-drawing tasks. Afterwards, they reported their perceived cognitive load using a multidimensional instrument adapted from Leppink et al. (2013). In the second part of the study, students were given an iPad and a brief introduction (10 min) to drawing molecules using JACK and Kekule.js, including a follow-along sample task. Once all the technical questions were answered, the students were asked to complete eight digital molecule-drawing tasks. Cognitive load and usability ratings were then collected.
The average mean for person ability regarding drawing molecules person ability (Nstudents = 22, npp items = 8 Person Reliability: 0.35, Item Reliability: 0.90, ndigital items = 8, Person Reliability: 0.63, Item Reliability: 0.85) in paper-pencil (M = 0.69, SE = 0.34) and digital (M = −0.63, SE = 0.46) tasks were compared using a t-test, which revealed a significant difference, t(21) = 2.59, p = 0.017, dRM, pooled = 0.438, favoring the paper-pencil molecule-drawing tasks with higher person abilities, ΔM = 1.33, BCa 95% CI [−2.268, −0.328]. Figure 2 provides two boxplot diagrams. Person abilities are plotted on the y-axis using the logit scale of the Rasch model. High person abilities are represented by high positive values. A mid-level person ability is represented by a value around zero. Low person abilities in drawing molecules are represented by negative values. The two formats (paper-pencil, digital) are plotted on the x-axis. The two boxplot diagrams show the distribution of person abilities for drawing molecules for both formats. The bold black lines represent the median for person abilities for each format (Field, 2018). The blues boxes represent the area into which the person abilities of half of the observed students fall (interquartile range). The whiskers represent the top and bottom 25% of person abilities. Differences regarding the position of the bold black line, the shape of the blue boxes and the positions of whiskers indicate differences between the formats. A lower position of the bold black line for the digital format in the diagram at the upper left (study 1, summer 2022) indicates that the students’ person ability to solve digital tasks was lower than their ability to solve paper-pencil-based tasks.
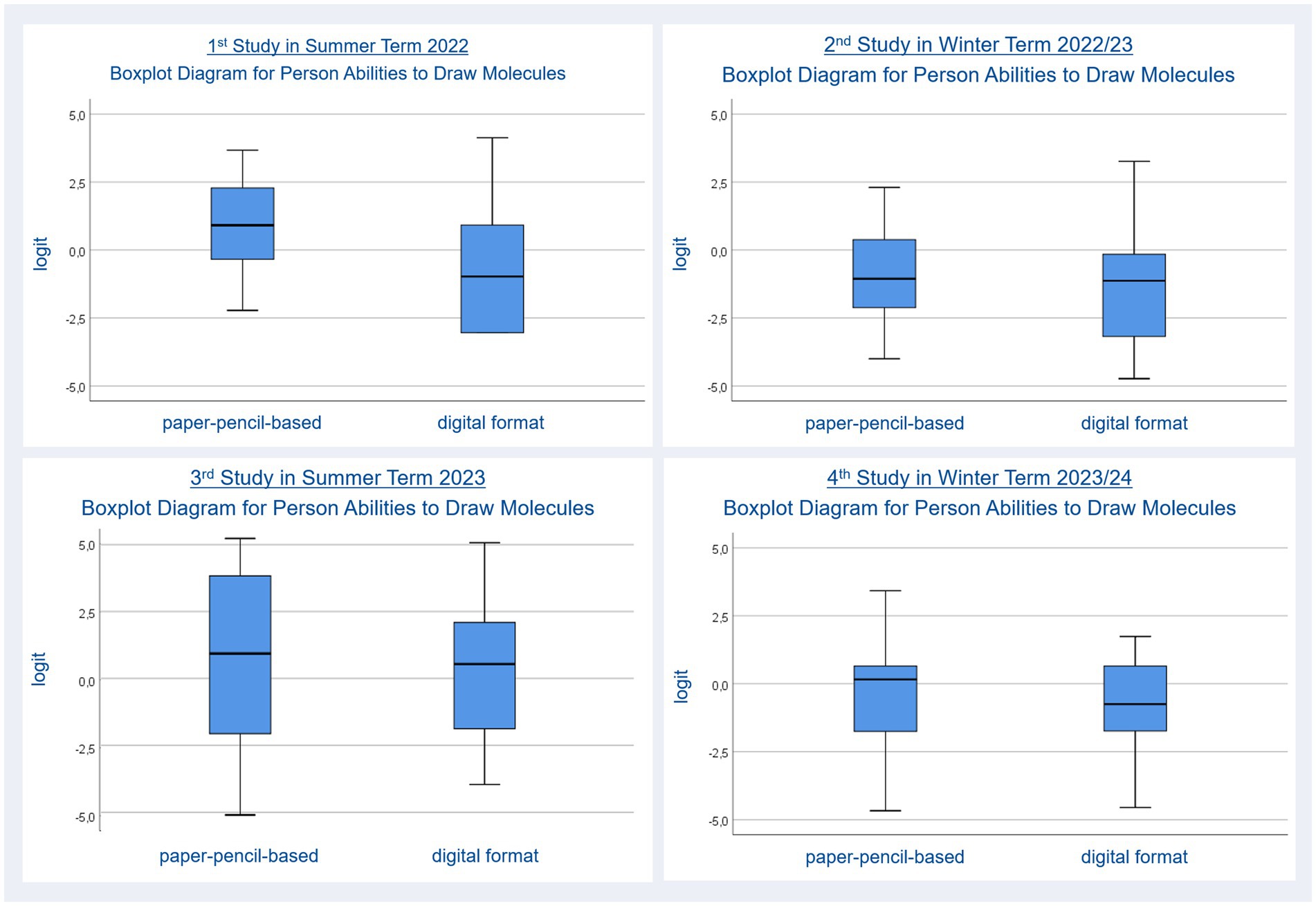
Figure 2. Person abilities in drawing molecules for paper-pencil-based and digital molecule-drawing tasks.
Intrinsic cognitive load ratings were used to inspect the cognitive load which arises from the content, namely drawing molecules based on their IUPAC name. The more interacting elements a student has to process at the same time, such as the number of carbon atoms related to the name of the carbon chain, the functional group related to the name of a substituent, the number of alkyl groups and their positions, the higher the intrinsic cognitive load will be. Comparing the average mean for person ability regarding intrinsic load ratings (Nstudents = 22, nil items pp = 3, Person Reliability: 0.71, Item Reliability: 0.87, nil items digital = 3 Person Reliability: 0.70, Item Reliability: 0.96) for paper-pencil (M = −0.78, SE = 0.32) and digital (M = −0.43, SE = 0.25) tasks using a t-test revealed no significant differences, t(21) = 1.06, p = 0.300, ΔM = 0.36, BCa 95% CI [−1.032, −0.238], which means students perceived equal intrinsic load while working on the paper-pencil and digital molecule-drawing tasks. Figure 3 (upper left) shows the comparison between formats regarding intrinsic cognitive load using boxplot diagrams. The comparable position of the median (bold black line) and the shape of the boxes and whiskers indicate no differences between formats. The single dot labeled with an 8 underneath one whisker represents an outlier (a student whose ratings are not fitting to the other students’ ratings).
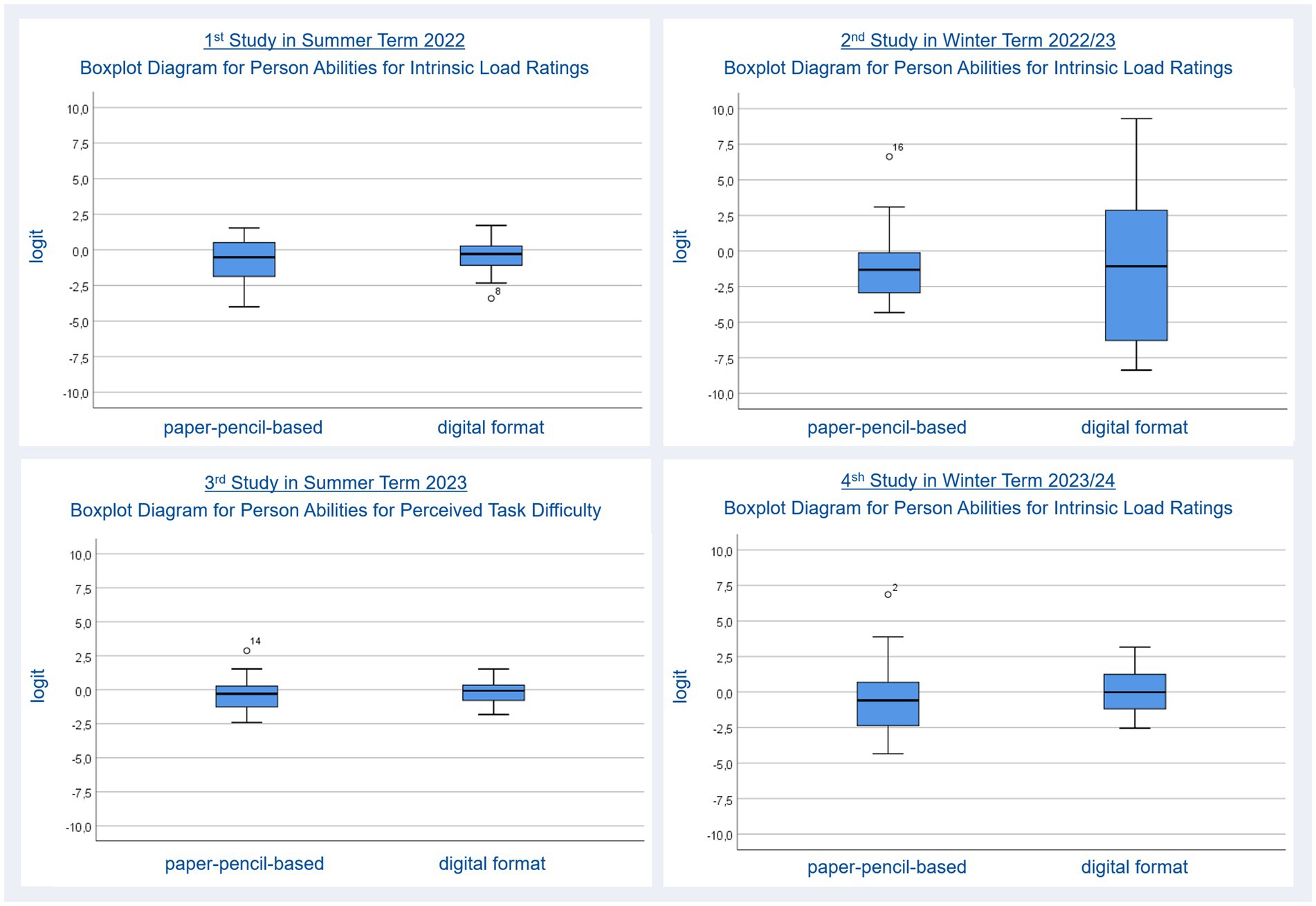
Figure 3. Person abilities for cognitive load ratings for paper-pencil-based and digital molecule-drawing tasks.
Extraneous cognitive load ratings were used to inspect the cognitive load which arises from processing not content related information. For example, a student maybe knows which functional group she has to draw to depict a molecule but she cannot remember where to find the option to add a heteroatom within the user interface of Kekule.js. Therefore, she has to try different options. In case she chooses a wrong user interface element on her first try, she has to remove the changes she made to her molecule, before she can come back to searching for an option to add the heteroatom. This testing by trial-and-error will require cognitive resources which are not available to solving the original task. The average mean for person ability regarding extraneous load ratings (Nstudents = 22, nel items pp = 3, Person Reliability: 0.47, Item Reliability: 0.56, nel items digital = 3, Person Reliability: 0.54, Item Reliability: 0.97) for paper-pencil (M = −1.77, SE = 0.42) and digital (M = −0.38, SE = 0.17) tasks were also compared using a t-test. The results revealed significantly lower mean person abilities for paper-pencil tasks, t(21) = 3.21, p = 0.004, dRM, pooled = 0.430, ΔM = 1.49, BCa 95% CI [−2.268, −0.648], meaning that students perceived lower extraneous load while working on such tasks. Again, boxplot diagrams were used to show differences between formats regarding extraneous cognitive load (Figure 4, upper left). Like in Figure 2 the differences regarding the position of the median and the distribution of person abilities regarding agreement with extraneous cognitive load ratings indicate differences between the two formats.
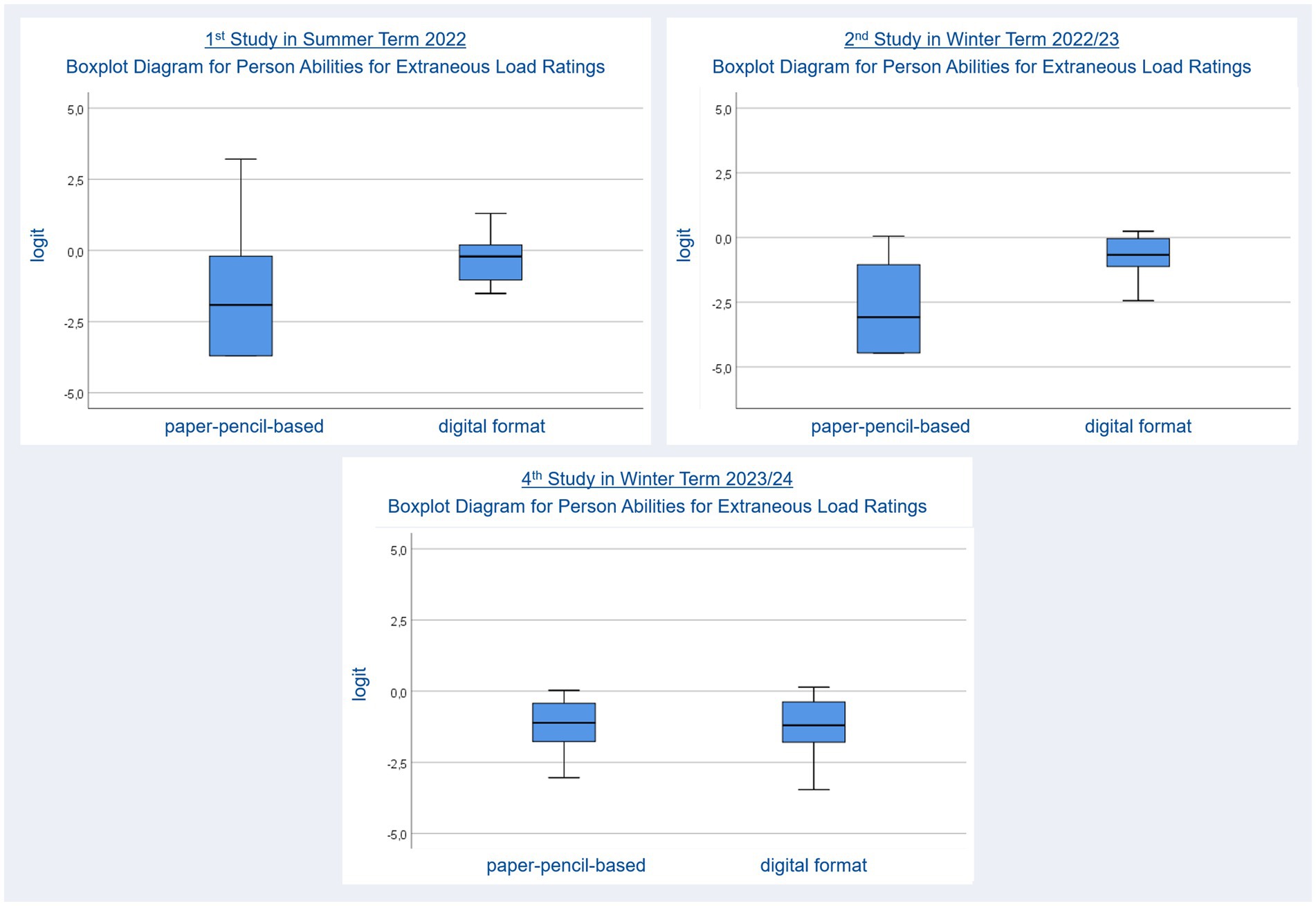
Figure 4. Person abilities for extraneous cognitive load ratings after working on paper-pencil-based and digital molecule-drawing tasks.
Person abilities for usability ratings for the digital tasks (Nstudents = 22, NItems = 9, Person Reliability: 0.88, Item Reliability: 0.89) amounted to M = −0.37, SE = 0.25, slightly below the middle of the scale. Hence, the students’ usability experience was slightly less than medium.
Person ability for cognitive load ratings and the usability of digital tasks were correlated. The intrinsic cognitive load was significantly correlated with usability, r = −0.477, p = 0.025, BCa 95% CI [−0.758, −0.082]. Hence, students who reported higher intrinsic load experienced lower usability. The correlation between extraneous load and usability was in line with this finding, r = −0.455, p = 0.033, BCa 95% CI [−0.762, −0.041]. Hence, students who reported higher extraneous load experienced lower usability.
Overall, the first study showed that students’ ability to solve paper-pencil molecule-drawing tasks was higher than their ability to solve comparable digital tasks, and that students reported higher extraneous cognitive load for digital molecule-drawing tasks and unsatisfactory usability. Hence, students who received very little instruction regarding the tool and previously learned to draw molecules in a paper-pencil format were hindered in their ability to solve molecule-drawing tasks and perceived an extraneous load when trying to solve digital molecule-drawing tasks.
4.2 Study 2
To investigate whether the results of the first study would change if students were used to drawing molecules digitally, we extended the introduction to the digital tool. The first session of the organic chemistry course in the winter term was used to provide a longer introduction to the tool, with a training phase of eight tasks and personal support for problems and questions (approximately 30 min in total). Additionally, we asked students to bring their own devices and offered faculty iPads to students who did not bring their own devices. During the following weeks, the students were continuously asked to complete digital molecule-drawing tasks at home as part of the course exercises. After 1 month, data were collected from 21 bachelor’s degree students preparing to become chemistry teachers. In Germany, these students study two subjects (in our case chemistry and another subject, e.g., mathematics) and educational science. Students first worked on paper-pencil molecule-drawing tasks and rated their perceived cognitive load before working on the digital tasks and reporting their perceived cognitive load and usability. In this study, we used 17 molecule-drawing tasks in tandem. Again, we collected cognitive load and usability ratings.
The average mean for person ability regarding performance (Nstudents = 21, npp items = 17, Person Reliability: 0.74, Item Reliability: 0.83, ndigital items = 17, Person Reliability: 0.78, Item Reliability: 0.86) in paper-pencil (M = −1.03, SE = 0.39) and digital (M = −1.39, SE = 0.48) tasks were compared using a t-test; this revealed no significant difference, t(20) = 1.10, p = 0.285. Boxplot diagrams (Figure 2, upper right) support this finding.
Item Reliability for intrinsic cognitive load ratings for the digital format is not acceptable. Low item reliability implies that the person sample is not large enough to confirm the item difficulty hierarchy (=construct validity) of the instrument; hence, our work excluded item analysis. A comparison of the average mean for person ability regarding intrinsic load ratings (Nstudents = 21, nil items pp = 3, Person Reliability: 0.87, Item Reliability: 0.66, nil items digital = 3 Person Reliability: 0.94, Item Reliability: 0.00) for paper-pencil (M = −1.04, SE = 0.55) and digital (M = −1.05, SE = 1.25) tasks using a t-test revealed no significant differences, t(20) = 0.01, p = 0.990, ΔM = 0.01, BCa 95% CI [−1.851, −1.863], which means that students perceived equal content-related (intrinsic) load while working on the paper-pencil and digital molecule-drawing tasks. Boxplot diagrams (Figure 3, upper right) support this finding. Again, the single dot labeled with 16 above the left whisker represents an outlier.
The average mean for person ability regarding not content-related (extraneous) load ratings (Nstudents = 21, nel items pp = 3, Person Reliability: 0.32, Item Reliability: 0.71, nel items digital = 3, Person Reliability: 0.53, Item Reliability: 0.88) for paper-pencil (M = −2.68, SE = 0.37) and digital (M = −0.77, SE = 0.19) tasks were also compared using a t-test. This revealed significantly lower mean ratings for person ability regarding extraneous load in paper-pencil tasks, t(20) = 4.90, p ≤ 0.001, dRM, pooled = 1.421, ΔM = −1.91, BCa 95% CI [−2.686, −1.106], meaning that students perceived lower extraneous load while working on the paper-pencil molecule-drawing tasks. Boxplot diagrams (Figure 4, upper right) support this finding.
Person abilities for usability ratings for the digital tasks (Nstudents = 21, Nitems = 9, Person Reliability: 0.85, Item Reliability: 0.88) amounted to M = 0.19, SE = 0.27, slightly above the middle of the scale. Hence, the students’ usability experience was slightly above medium level.
Person ability for cognitive load ratings and usability of the digital tasks were correlated. The correlation between extraneous load and usability is significant, r = −0.738, p ≤ 0.001, BCa 95% CI [−0.883, −0.479]. Hence, students who reported higher extraneous load experienced lower usability. The correlation between person ability for intrinsic cognitive load and usability was not significant. Hence, no significant relationship was found between intrinsic cognitive load and usability.
In conclusion, the results showed that the extended introduction, continuous use of digital molecule-drawing exercises during the course, and opportunity to work with a personal device helped reduce differences between the ability to draw molecules either paper-pencil-based or digitally. Equally perceived intrinsic cognitive load pointed to the same direction. However, students still perceived a higher extraneous load when working on the digital molecule-drawing tasks. Students’ usability experience was a bit above medium level.
4.3 Study 3
Due to the further development of the tool, we have made various changes to the design of the organic chemistry exercise for the cohort that took part in the third study. However, these changes did not alter the tasks we used for the study.
In the summer of 2023, students were again introduced to the tool during the first session of the term; this included a training phase on their own devices and an opportunity to ask questions and receive personal support. Students were continuously asked to work on digital exercises as homework for the course during the following weeks. Three weeks later, data were collected from 26 undergraduate students (majoring in chemistry or water science). We used 14 molecule-drawing tasks in tandem this time. Students first worked on the digital drawing tasks before switching to the paper-pencil format. Considering the unsatisfactory reliability of the cognitive load rating scales, we used an immediate unidimensional single-item measure (Kalyuga et al., 2001). This item measures an overall cognitive load. Thus, it is not able to differentiate between extraneous or intrinsic load. In the third study, no usability ratings were collected (usability was investigated at a later point in the course; therefore, no usability ratings were reported for the third study).
The average mean for person ability regarding drawing molecules (Nstudents = 26, npp items = 14, Person Reliability: 0.86, Item Reliability: 0.85, ndigital items = 14, Person Reliability: 0.81, Item Reliability: 0.88) in paper-pencil (M = 0.73, SE = 0.62) and digital (M = 0.23, SE = 0.50) tasks were compared using a t-test; this revealed no significant difference: t(25) = 1.21, p = 0.238. The boxplot diagrams in Figure 2 (upper left) represent this finding.
The average mean for person ability regarding perceived task difficulty ratings (Nstudents = 26, npp item = 1 answered 14 times, Person Reliability: 0.94, Item Reliability: 0.95, nitem digital = 1 answered 14 times, Person Reliability: 0.72, Item Reliability: 0.94) of paper-pencil (M = −0.28, SE = 0.23) and digital (M = −0.18, SE = 0.15) tasks were also compared using a t-test. This revealed no significant difference, t(25) = 1.05, p = 0.306, ΔM = 0.10, BCa 95% CI [−0.292, 0.101], which means that perceived task difficulty did not differ between digital and paper-pencil molecule-drawing tasks. The boxplot diagrams in Figure 3 (upper left) represent this finding. As these boxplots are based on perceived task difficulty ratings they are not directly comparable to the other boxplot diagrams in Figure 2 which are based on intrinsic cognitive load ratings. The dot labeled with 14 again represents an outlier.
In summary, the results of the third study support the findings of the second study and provide further evidence that students’ performance in simple molecule-drawing tasks is independent of the format (paper-pencil or digital). Regarding extraneous cognitive load, it remains unclear whether the item used failed to detect differences or whether students did not perceive a higher level of extraneous cognitive load while working on digital molecule-drawing tasks. The findings require further investigation.
4.4 Study 4
Before the start of the winter term (2023/24), we attempted to improve the usability of the digital tool for an elementary organic chemistry course by deleting user interface elements that were irrelevant for working on the tasks (e.g., templates for heterocycles). At the beginning of the course, students were introduced to the tool during the first session of the term; this included a training phase on their own devices and the opportunity to ask questions and receive personal support. Afterwards, they had the opportunity to work on the first organic chemistry tasks in class, ask questions, or receive support. Students were continuously asked to work on digital exercises as homework for the course during the following weeks. Two weeks later, data were collected from 25 bachelor’s degree students preparing to become chemistry teachers. Again, we used 14 molecule-drawing tasks in tandem, and the students first worked on the digital drawing tasks before switching to the paper-pencil format. We switched back to the multidimensional cognitive load measure we already used for the first and the second study and used the UEQ (Laugwitz et al., 2008) to measure usability.
The average mean for person ability regarding drawing molecules (Nstudents = 25, npp items = 14, Person Reliability: 0.78, Item Reliability: 0.86, ndigital items = 14, Person Reliability: 0.73, Item Reliability: 0.85) in paper-pencil (M = 0.37, SE = 0.42) and digital (M = −0.86, SE = 0.39) tasks were compared using a t-test, revealing no significant difference, t(24) = 1.67, p = 0.107. Again, boxplot diagrams were used to represent this finding (Figure 2, bottom right).
A comparison of the average mean for person ability regarding content-related (intrinsic) load ratings (Nstudents = 25, nil items pp = 3, Person Reliability: 0.87, Item Reliability: 0.71, nil items digital = 3 Person Reliability: 0.75, Item Reliability: 0.71) for paper-pencil (M = −0.64, SE = 0.51) and digital (M = 0.07, SE = 0.32) tasks using a t-test revealed no significant differences, t(24) = 1.80, p = 0.085, meaning that students perceived equal intrinsic load while working on the paper-pencil and digital molecule-drawing tasks (Figure 3, bottom right). Again, boxplot diagrams were used to represent this finding (Figure 3, bottom right). An outlier is shown by the dot labeled with 2.
We also compared the average mean for person ability regarding not content-related (extraneous) load ratings (Nstudents = 25, nel items pp = 3, Person Reliability: 0.05, Item Reliability: 0.47, nel items digital = 3, Person Reliability: 0.35, Item Reliability: 0.90) for paper-pencil (M = −1.25, SE = 0.21) and digital (M = −1.50, SE = 0.25) tasks using a t-test. This revealed no significant differences, t(24) = 0.76, p = 0.458. Hence, the students perceived equal extraneous load while working on the paper-pencil and digital molecule-drawing tasks. Again, boxplot diagrams were used to represent this finding (Figure 4, bottom right).
The mean person abilities for all subscales of the UEQ were above zero. Hence, students perceived usability as acceptable. The attractiveness of the tool was merely sufficient, but students’ perceptions of efficiency and stimulation were very good as shown in Table 3.
Extraneous cognitive load was significantly negatively correlated with attractiveness: r = −0.475, p = 0.016, BCa 95% CI [−0.739, −0.114]; perspicuity: r = −0.399, p = 0.048, BCa 95% CI [−0.696, 0.169]; efficiency: r = −0.552, p = 0.004, BCa 95% CI [−0.796, −0.151]; and stimulation: r = −0.585, p = 0.002, BCa 95% CI [−0.802, −0.266], indicating that students who perceived high extraneous load rated the tool’s attractiveness, perspicuity, efficiency, and stimulation to be lower and vice versa. We found no significant correlation between intrinsic load and tool usability.
In summary, the results of the fourth study support the findings of the second and third studies and provide further evidence that students’ performance in simple molecule-drawing tasks is independent of the format (paper-pencil or digital). Regarding extraneous cognitive load, the fourth study provided evidence that after reducing the user interface, students perceived equal levels of extraneous cognitive load in both the paper-pencil and digital molecule-drawing tasks. Students considered the tool’s usability sufficient, with values above zero for all usability subscales.
5 Discussion
5.1 Discussion of the evaluation studies and their limitations
Figure 5 summarizes the results of the evaluation studies. The comparison between paper-pencil-based format and digital format regarding drawing molecules and cognitive load is represented individually. Undesirable differences between formats are marked with an X, missing statistical differences between formats are marked with a check mark. Additionally, results regarding usability are included. Figure 5 shows that we were able to improve the tool to reach satisfying results regarding drawing molecules, cognitive load, and usability over time.
The first study showed significant differences in person ability to solve paper-pencil-based and digital molecule-drawing tasks. This finding is consistent with the significantly higher extraneous cognitive load and equal intrinsic load observed for both formats. Please remember that intrinsic load arises from the content-related complexity of a task (e.g., drawing an ethane molecule is less complex than drawing a 3-ethylhexanal molecule because more structural elements have to be considered for drawing) whereas extraneous cognitive load arises from processing information which are not content-related (e.g., searching for an option to add a heteroatom required for depicting a functional group by trial-and-error). Thus, although the participants perceived the chemical content of the molecule-drawing task to be equally difficult (no significant difference regarding intrinsic cognitive load), their ability to solve such tasks was higher for the paper-pencil format. We used the Rasch model to calculate students’ person ability to solve tasks asking them to draw molecules based on their IUPAC name. The person ability is based on students’ performance. In comparison to a raw sum score of correctly solved items, the person ability also considers whether the correctly solved items were easy or difficult items. The lower ability to solve digital molecule-drawing tasks can be explained by the higher extraneous cognitive load of this format. Accordingly, the usability ratings were unsatisfactory, as they were below the scale center. That usability was significantly negatively correlated with intrinsic cognitive load meant that a higher intrinsic cognitive load was associated with reduced experienced usability and vice versa. Additionally, the extraneous cognitive load was significantly negatively correlated with usability. Specifically, students who perceived high extraneous load reported lower usability. These findings align with the cognitive load theory, suggesting that students who already perceive a high load from processing the relevant information to solve a task are at risk of perceiving cognitive overload due to unintuitive and unfamiliar handling of the tool, resulting in poorer usability. By contrast, participants who perceive a lower intrinsic load may have more working memory capacity to process the necessary information regarding tool handling, leading to better usability. Overall, the first study showed that students who had only minimal instruction in using the tool, previously learned to draw molecules in a traditional paper-pencil format, and used foreign devices experienced difficulty in solving molecule-drawing tasks and faced additional extraneous cognitive load when attempting to solve digital molecule-drawing tasks. The high extraneous load implies that the difficulty did not lie in the task itself but was induced by the unfamiliar medium or format.
Based on this assumption, we extended the participants’ introduction to the digital tool in the next term and transitioned from using faculty devices to using students’ personal devices, which were more familiar to them. Additionally, during the course of the term, we used the digital tool for knowledge acquisition. As there were no significant differences in person ability to complete the digital or paper-pencil-based molecule-drawing tasks, students were able to perform at similar levels when given their own devices, extended instructions, and regular training with the digital tool. Nevertheless, there was still a significantly higher extraneous cognitive load reported by the students when performing the digital drawing tasks. Again, no significant differences were found in intrinsic cognitive load. The usability rating slightly improved from the first to the second study and was found to be moderate. A significant correlation between intrinsic load and usability was no longer observed. Hence, when students were accustomed to using the tool, the perceived usability appeared unrelated to the perceived difficulty of the task. Similar to the first study, we found a negative correlation between the extraneous cognitive load and tool usability. This suggests that the tool’s unintuitive handling places a load on the students’ working memory. In summary, the results showed that the extended introduction, regular use of digital molecule-drawing exercises during the course, and the opportunity to work with personal devices helped decrease the gap in students’ ability to draw molecules using either paper and pencil or a digital tool. In conclusion, these findings indicate that the implemented measures effectively reduced the differences in performance. Nevertheless, the students still perceived a higher extraneous load when working on digital molecule-drawing tasks.
Students’ feedback revealed that they found a combination of digital and paper-pencil homework exercises challenging to organize. However, the results from the second study showed no difference in person ability between the two formats when given an extended introduction, opportunities for regular use, and personal devices for students’ use. The tool’s ongoing improvements encouraged us to switch to entirely digital homework exercises for the basic organic chemistry course. The results of the third study confirmed the findings of the second study, which showed no differences in person abilities to solve molecule-drawing tasks using digital or paper-pencil-based formats. Unidimensional cognitive load measurements (which measure an overall load without being able to distinguish between intrinsic and extraneous load) did not detect differences in cognitive load between formats. However, it remained unclear whether the instrument was unable to detect differences or whether students, being used to drawing molecules digitally, did not perceive differences in the cognitive load. No usability ratings were collected in the third study.
To improve usability and reduce the extraneous load, we have customized the user interface by removing elements that are not necessary for processing the current tasks (e.g., templates for drawing heterocycles). Moreover, instead of working at home, we gave students the chance to work on the first organic chemistry tasks in class and provided support when needed. The fourth study showed equal abilities to solve molecule-drawing tasks, intrinsic load, and extraneous load for both formats, and ratings of sufficient to good for tool usability.
Overall, the evaluation studies demonstrated that the developed tool is functional. We provided evidence that students can accomplish digital drawing tasks using the tool as proficiently as using paper and pencil, provided that they are sufficiently familiar with the tool (RQ1). Nevertheless, the tool appeared to induce an extraneous cognitive load (RQ2) that should be reduced to relieve students’ working memory. Reducing the extraneous cognitive load shows promise in improving unsatisfactory tool usability (RQ3), as these factors are closely related. An appropriate configuration of the Kekule.js widgets to accommodate task requirements involves hiding unnecessary controls and options, along with reducing extraneous cognitive load and improving usability (fourth study).
One limitation is that the chosen study design did not allow us to statistically determine the factors that cause a higher extraneous load for the digital drawing tool. Any adjustments we made were therefore based solely on assumptions derived from experience and discussions with students. One of these assumptions is that students who would normally not choose skeletal formula because of its abstract nature were forced by the digital tool to do so. Moreover, the use of a digital tool could lead to a tool-driven solution (e.g., avoiding repeated switching between required functions such as the option to draw single bonds or the option to insert heteroatoms). Unlike the paper format where students can develop their drawing step by step along the name of the molecule, a tool-driven solution requires a mental pre-structuring and planning of the drawing. We also deem the absence of a notepad function a deficiency, because this could help relieve working memory through note-taking. Although students were also allowed to take pen and paper to solve the digital tasks in the current format, this was only used spontaneously by a few individuals (so it could also be a strategy problem for the students). We can also imagine that the automatic correction of violations of the octet rule causes confusion among students. Occasionally, questions were asked in the tests that point in this direction (If I insert an oxygen atom here, suddenly a hydrogen atom also appears. How do I get rid of that?). Various designs for evaluation studies are required to understand the causes of this extraneous load. A comparison of the representation form used between paper-pencil and digital formats appears to be a productive method for obtaining information on students’ preferred representation format. In situations in which students do not use the skeletal formula for the paper-pencil format, differences between media may occur because of the obligation to use an unfamiliar representation form for the digital format. Additional training for the use of skeletal formulas, the commonly used representation format for presenting chemical structures among professionals, offers the potential for improvement. Further investigation is necessary to better understand the causes of the extraneous cognitive load. Reliable instruments are required to measure both extraneous and intrinsic cognitive load. Aside from those used in the evaluation studies presented, other multidimensional cognitive load measurement instruments are available for learning scenarios with unknown potential (Klepsch et al., 2017; Krieglstein et al., 2023); however, comparable instruments for performance are still missing.
Our findings are limited by the unsatisfactory reliability of the multidimensional measurements of cognitive load used here as well as the potential inability of the unidimensional instrument to identify variances in extraneous cognitive load. Furthermore, the results are limited in that they rely on a small number of molecule-drawing tasks and the inclusion of students from only one university. In addition, our results are limited by the fact that the students study different subjects in the summer and winter semesters (Chemistry B. Sc. and Water Science B. Sc. in the summer semester, students preparing to become chemistry teachers in the winter semester). We recommend that future studies include students from other universities, which would require synchronization of basic organic chemistry courses and the use of the same exercises. Another limitation is the use of a single task format, specifically molecule-drawing tasks. Future research should investigate whether these results can be replicated for other tasks such as those dealing with chirality or reaction mechanisms. The results of our work are limited to assessments and do not consider learning, bearing in mind that the main objective of developing the digital tool was to enhance learning.
In summary, based on initial evaluations, it was feasible to implement a digital tool for organic chemistry courses. We developed a noncommercial (G1) e-learning and e-assessment tool that (G2) can be integrated into learning platforms such as Moodle or Ilias. Further efforts are needed to reduce the system-induced extraneous cognitive load and thereby enhance usability (G5). The next step is to assess whether integrating the tool’s features—such as receiving individualized, explanatory feedback in real time, working at a self-paced rate, and having extra opportunities to practice—enhance students’ learning outcomes and overall performance in organic chemistry (G4). A further step could be to look for different teachers who create their own typical organic chemistry tasks that they need for their courses (G3).
In addition to evaluating the use of a digital tool for an organic chemistry course, various other findings have emerged from discussions with students. For example, students expressed a dislike for the combination of digital and paper-pencil homework exercises, as they made the tasks challenging to manage. Students told us that working on digital and paper-pencil-based tasks makes it hard to remember whether they have solved all tasks as they had to check two sources. Additionally, they had problems with integrating paper-pencil-based and digital notes when preparing for exams. The participants also expressed their desire to export tasks, their responses, and the feedback they received to document their course progress and prepare for exams. Because offline copies of exams are required for exam documentation, the export-as-PDF feature was added to JACK, allowing students to save their results. Experiences from the summer of 2023 demonstrated that the implementation of a digital learning environment failed to improve student motivation to complete course exercises. Only a minority of the students completed the weekly exercises at home, while the majority appeared unprepared for the in-person sessions, expecting the teacher to provide them with a sample solution for review before the end-of-semester examination. As we decided to provide a worked-out example after three unsuccessful solution attempts, the format of the course’s in-person sessions will change when the weekly homework exercises are done digitally. It is no longer necessary to use in-person sessions to provide sample solutions for all tasks. Hence, the use of digital homework exercises has the potential to enhance in-person sessions towards a more advanced involvement with organic chemistry, provided that students are willing and adequately prepared to attend classes. In short, the digital tool does facilitate the implementation of flipped classroom approaches (van Alten et al., 2019), with student motivation being the main challenge.
5.2 Technical development and next steps
Overall, the integration of Kekule.js into JACK has resulted in a powerful tool that enables the digital implementation of a sufficient number of standard organic chemistry tasks and has potential for further enhancement, such as automating the evaluation of complete reaction mechanisms. Although further challenges must be addressed (e.g., automatic evaluation of mesomerism, transition states, arrows for electron transition, or the option to create and evaluate tasks that ask to mark parts of a molecule), the existing tool already offers additional learning opportunities and explanatory feedback that cannot be offered in the same amount during an in-person course. System development activities thus far have focused on the proper technical integration of Kekule.js into JACK, including the possibility of configuring the editor interface and providing essential features for teachers to define rule-based feedback. Thus, the current state of development has not yet explored the potential additional benefits that digital drawing may provide with respect to automated analysis. The next development steps will extend the capabilities for automated answer analysis and rule-based feedback in two ways. First, JACK will not only analyze the InChI code generated from drawings, but also inspect the machine-readable representation of the drawing itself. This will not only allow the analysis of reactions with respect to the positions of molecules in relation to the reaction arrow, as already mentioned above, but also solve some other problems such as the representation; for example, it will also allow detecting additional annotations and color markings. Second, JACK will be linked to a Chemistry Development Kit (Steinbeck et al., 2006), allowing for the automatic detection of certain differences between molecules. This will save teachers the burden of listing all well-known errors in their feedback rules and instead allow them to write these rules on a conceptual level, defining feedback on types of errors instead of individual errors.
Automatic analysis of molecule-drawing tasks is based of comparing InChI-codes. During the 2 years in which evaluation studies were set, an additional function to automatically analyze reaction equations was implemented. This function is based on searching for reaction arrow and checking which molecules are placed in front (educts) and behind (products) or above (minor educts) or underneath (minor products) this reaction arrow. An extension of this function, which is currently under technical evaluation, allows to automatically evaluate reactions based on multiple reaction equations. The next step will be to inspect the machine-readable representation of the drawing itself, which will allow to inspect for lone electron pairs and electron pushing arrows. Hence, this function will enable us to automatically analyze reaction mechanisms. Figure 6 provides an overview over the tool’s development with example tasks.
Data availability statement
The raw data supporting the conclusions of this article will be made available by the authors, without undue reservation.
Ethics statement
Ethical approval was not required for the studies involving humans because although IRB approval was not necessary at German universities, the guidelines concerning the ethical scientific practice of the Federal German Research Foundation (DFG) were applied. The studies were conducted in accordance with the local legislation and institutional requirements. The participants provided their written informed consent to participate in this study.
Author contributions
KS: Conceptualization, Investigation, Methodology, Visualization, Writing – original draft. MS: Software, Writing – original draft. DP: Conceptualization, Writing – review & editing. AL: Funding acquisition, Project administration, Supervision, Writing – review & editing. MGo: Project administration, Software, Supervision, Writing – review & editing, Resources. MGi: Investigation, Project administration, Supervision, Writing – review & editing, Validation. MW: Conceptualization, Funding acquisition, Project administration, Resources, Supervision, Writing – review & editing.
Funding
The author(s) declare that financial support was received for the research, authorship, and/or publication of this article. This project was funded by Foundation Innovations in Higher Education. We thank the University of Bonn for funding the development of the e-learning tool within the Hochschulpakt and ZSL program of North Rhine-Westfalia State.
Conflict of interest
The authors declare that the research was conducted in the absence of any commercial or financial relationships that could be construed as a potential conflict of interest.
Publisher’s note
All claims expressed in this article are solely those of the authors and do not necessarily represent those of their affiliated organizations, or those of the publisher, the editors and the reviewers. Any product that may be evaluated in this article, or claim that may be made by its manufacturer, is not guaranteed or endorsed by the publisher.
References
Anzovino, M. E., and Lowery Bretz, S. (2016). Organic chemistry students’ fragmented ideas about the structure and function of nucleophiles and electrophiles: a concept map analysis. Chem. Educ. Res. Pract. 17, 1019–1029. doi: 10.1039/C6RP00111D
Asmussen, G., Rodemer, M., and Bernholt, S. (2023). Blooming student difficulties in dealing with organic reaction mechanisms – an attempt at systemization. Chem. Educ. Res. Pract. 24, 1035–1054. doi: 10.1039/d2rp00204c
Aulck, L., Velagapudi, N., Blumenstock, J., and West, J. (2016). Predicting student dropout in higher education. 2016 ICML Workshop on #Data4Good: Machine Learning in Social Good Applications. doi: 10.48550/arXiv.1606.06364
Averbeck, D. (2021). “Zum Studienerfolg in der Studieneingangsphase des Chemiestudiums: Der Einfluss kognitiver und affektiv-motivationaler Variablen [study success of chemistry freshmen: the influence of cognitive and affective variables]” in Studien zum Physik- und Chemielernen: Vol. 308. ed. A. Daniel (Berlin: Logos Berlin).
Bodé, N. E., Caron, J., and Flynn, A. B. (2016). Evaluating students’ learning gains and experiences from using nomenclature101.com. Chem. Educ. Res. Pract. 17, 1156–1173. doi: 10.1039/c6rp00132g
Bond, T. G., and Fox, C. M. (2007). Applying the Rasch model: Fundamental measurement in the human sciences. 2nd Edn. New York: Lawrence Erlbaum Associates Publishers.
Boone, W. J. (2016). Rasch analysis for instrument development: why, when, and how? CBE Life Sci. Educ. 15:148. doi: 10.1187/cbe.16-04-0148
Boone, W. J., and Scantlebury, K. (2006). The role of Rasch analysis when conducting science education research utilizing multiple-choice tests. Science 90, 253–269. doi: 10.1002/sce.20106
Boone, W. J., and Staver, J. R. (2020). Advances in Rasch analyses in the human sciences. Cham: Springer International Publishing.
Boone, W. J., Staver, J. R., and Yale, M. S. (2014). Rasch analysis in the human sciences. Heidelberg: Springer Netherlands.
Brooke, J. (1996). “SUS: a ‘quick and dirty’ usability scale” in Usability evaluation in industry. eds. P. W. Jordan, B. Thomas, B. A. Weerdmeester, and I. L. McClelland. First ed (London, GB: Taylor and Francis: Bristol, PA).
Buchner, J., and Kerres, M. (2023). Media comparison studies dominate comparative research on augmented reality in education. Comput. Educ. 195:104711. doi: 10.1016/j.compedu.2022.104711
Chamala, R. R., Ciochina, R., Grossman, R. B., Finkel, R. A., Kannan, S., and Ramachandran, P. (2006). EPOCH: an organic chemistry homework program that offers response-specific feedback to students. J. Chem. Educ. 83, 164–169. doi: 10.1021/ed083p164
Chen, J. H., and Baldi, P. (2008). Synthesis explorer: a chemical reaction tutorial system for organic synthesis design and mechanism prediction. J. Chem. Educ. 85, 1699–1703. doi: 10.1021/ed085p1699
Chen, J. H., and Baldi, P. (2009). No electron left behind: a rule-based expert system to predict chemical reactions and reaction mechanisms. J. Chem. Inf. Model. 49, 2034–2043. doi: 10.1021/ci900157k
Chen, J. H., Kayala, M. A., and Baldi, P. (Eds.). (2010). Reaction Explorer: Towards a Knowledge Map of Organic Chemistry To Support Dynamic Assessment and Personalized Instruction. In: Enhancing Learning with Online Resources, Social Networking, and Digital Libraries. eds. R. E. Belford, J. W. Moore, and H. E. Pence 1060, 191209. doi: 10.1021/bk-2010-1060.ch011
da Silva Júnior, J. N., Nobre, D. J., do Nascimento, R. S., Torres, G. S., Leite, A. J. M., Monteiro, A. J., et al. (2018). Interactive computer game that engages students in reviewing organic compound nomenclature. J. Chem. Educ. 95, 899–902. doi: 10.1021/acs.jchemed.7b00793
Dickmann, T., Opfermann, M., Dammann, E., Lang, M., and Rumann, S. (2019). What you see is what you learn? The role of visual model comprehension for academic success in chemistry. Chem. Educ. Res. Pract. 20, 804–820. doi: 10.1039/C9RP00016J
Eitemüller, C., Trauten, F., Striewe, M., and Walpuski, M. (2023). Digitalization of multistep chemistry exercises with automated formative feedback. J. Sci. Educ. Technol. 32, 453–467. doi: 10.1007/s10956-023-10043-2
Farhat, N. J., Stanford, C., and Ruder, S. M. (2019). Assessment of student performance on Core concepts in organic chemistry. J. Chem. Educ. 96, 865–872. doi: 10.1021/acs.jchemed.8b00913
Fischer, V., Walpuski, M., Lang, M., Letzner, M., Manzel, S., Motté, P., et al. (2021). Studienerfolg und Studienabbruch [what influences the decision to drop out? Longitudinal analyses of the interplay between study satisfaction, content knowledge and dropout intention in chemistry, engineering and social sciences]. ZeHf 4, 55–80. doi: 10.3224/zehf.v4i1.05
Fleischer, J., Leutner, D., Brand, M., Fischer, H., Lang, M., Schmiemann, P., et al. (2019). Vorhersage des Studienabbruchs in naturwissenschaftlich-technischen Studiengängen [prediction of student drop-out in STEM study programs]. Z. Erzieh. 22, 1077–1097. doi: 10.1007/s11618-019-00909-w
Flynn, A. B. (2023). Orgchem101 learning lab. Available at: https://www.orgchem101.com (Accessed July 20, 2023).
Flynn, A. B., Caron, J., Laroche, J., Daviau-Duguay, M., Marcoux, C., and Richard, G. (2014). Nomenclature101.com: a free, student-driven organic chemistry nomenclature learning tool. J. Chem. Educ. 91, 1855–1859. doi: 10.1021/ed500353a
Graulich, N., and Bhattacharyya, G. (2017). Investigating students’ similarity judgments in organic chemistry. Chem. Educ. Res. Pract. 18, 774–784. doi: 10.1039/C7RP00055C
Grossman, R. B., and Finkel, R. (2023). ACE - achieving chemistry excellence organoc. Lexington: University of Kentucky.
Grove, N. P., and Lowery Bretz, S. (2012). A continuum of learning: from rote memorization to meaningful learning in organic chemistry. Chem. Educ. Res. Pract. 13, 201–208. doi: 10.1039/C1RP90069B
Hailikari, T. K., and Nevgi, A. (2010). How to diagnose at-risk students in chemistry: the case of prior knowledge assessment. Int. J. Sci. Educ. 32, 2079–2095. doi: 10.1080/09500690903369654
Hauck, D., Melle, I., and Steffen, A. (2021). Molecular orbital theory—teaching a difficult chemistry topic using a CSCL approach in a first-year university course. Educ. Sci. 11:485. doi: 10.3390/educsci11090485
Heller, S. R., McNaught, A., Pletnev, I., Stein, S., and Tchekhovskoi, D. (2015). Inchi, the IUPAC international chemical identifier. J. Chem. 7:23. doi: 10.1186/s13321-015-0068-4
Heublein, U., Richter, J., and Schmelzer, R. (2020). Die Entwicklung der Studienabbruchquoten in Deutschland [The development of student drop-out rates in Germany] (DZHW Brief 3|2020). Hannover, Germany: DZHW. doi: 10.34878/2020.03.dzhw_brief
IUPAC (Ed.) (2023). The IUPAC international chemical identifier (INCHI) : International Union of Pure and Apllied Chemistry. Avilable at: https://iupac.org/who-we-are/divisions/division-details/inchi/
Jiang, C., Jin, X., Dong, Y., and Chen, M. (2016). Kekule.Js: an open source JavaScript Chemoinformatics toolkit. J. Chem. Inf. Model. 56, 1132–1138. doi: 10.1021/acs.jcim.6b00167
Johnson, C., and Priest, A. H. (2014). “The feedback principle in multimedia learning” in The Cambridge handbook of multimedia learning. ed. R. E. Mayer (Cambridge: Cambridge University Press).
Kalyuga, S., Chandler, P., Tuovinen, J., and Sweller, J. (2001). When problem solving is superior to studying worked examples. J. Educ. Psychol. 93, 579–588. doi: 10.1037/0022-0663.93.3.579
Klepsch, M., Schmitz, F., and Seufert, T. (2017). Development and validation of two instruments measuring intrinsic, extraneous, and germane cognitive load. Front. Psychol. 8:1997. doi: 10.3389/fpsyg.2017.01997
Krieglstein, F., Beege, M., Rey, G. D., Sanchez-Stockhammer, C., and Schneider, S. (2023). Development and validation of a theory-based questionnaire to measure different types of cognitive load. Educ. Psychol. Rev. 35. doi: 10.1007/s10648-023-09738-0
Laugwitz, B., Held, T., and Schrepp, M. (2008). “Construction and evaluation of a user experience questionnaire” in Lecture notes in computer science. HCI and usability for education and work. ed. A. Holzinger (Berlin Heidelberg: Springer), 63–76.
Lenhard, W., and Lenhard, A. (2023). Psychometrica. Availabel at: https://www.psychometrica.de/effektstaerke.html (Accessed July 20, 2023).
Leppink, J., Paas, F., van der Vleuten, C. P. M., van Gog, T., and van Merriënboer, J. J. G. (2013). Development of an instrument for measuring different types of cognitive load. Behav. Res. Methods 45, 1058–1072. doi: 10.3758/s13428-013-0334-1
Linacre, J. M. (2023). A User’s guide to Winsteps® Ministep: Rasch-model computer programs. Available at: https://www.winsteps.com/winman/copyright.htm (Accessed July 23, 2024).
Malik, K., Martinez, N., Romero, J., Schubel, S., and Janowicz, P. A. (2014). Mixed-methods study of online and written organic chemistry homework. J. Chem. Educ. 91, 1804–1809. doi: 10.1021/ed400798t
Mayer, R. E., and Pilegard, C. (2014). “Principles for managing essential processing in multimedia learning: segmenting, pre-training, and modality principles” in The Cambridge handbook of multimedia learning. ed. R. E. Mayer (Cambridge: Cambridge University Press), 316–344.
Paas, F., Renkl, A., and Sweller, J. (2003a). Cognitive load theory and instructional design: recent developments. Educ. Psychol. 38, 1–4. doi: 10.1207/S15326985EP3801_1
Paas, F., and Sweller, J. (2014). “Implication of cognitive load theory for multimedia learning” in The Cambridge handbook of multimedia learning. ed. R. E. Mayer (Cambridge: Cambridge University Press).
Paas, F., Tuovinen, J. E., Tabbers, H., and van Gerven, P. W. M. (2003b). Cognitive load measurement as a means to advance cognitive load theory. Educ. Psychol. 38, 63–71. doi: 10.1207/S15326985EP3801_8
Paas, F., Tuovinen, J. E., van Merriënboer, J. J. G., and Aubteen Darabi, A. (2005). A motivational perspective on the relation between mental effort and performance: optimizing learner involvement in instruction. Educ. Technol. Res. Dev. 53, 25–34. doi: 10.1007/BF02504795
Penn, J. H., and Al-Shammari, A. G. (2008). Teaching reaction mechanisms using the curved arrow neglect (CAN) method. J. Chem. Educ. 85:1291. doi: 10.1021/ed085p1291
Rajan, K., Brinkhaus, H. O., Zielesny, A., and Steinbeck, C. (2020). A review of optical chemical structure recognition tools. J. Chem. 12:60. doi: 10.1186/s13321-020-00465-0
Rodemer, M., Eckhard, J., Graulich, N., and Bernholt, S. (2021). Connecting explanations to representations: benefits of highlighting techniques in tutorial videos on students’ learning in organic chemistry. Int. J. Sci. Educ. 43, 2707–2728. doi: 10.1080/09500693.2021.1985743
Schmeck, A., Opfermann, M., van Gog, T., Paas, F., and Leutner, D. (2015). Measuring cognitive load with subjective rating scales during problem solving: differences between immediate and delayed ratings. Instr. Sci. 43, 93–114. doi: 10.1007/s11251-014-9328-3
Spanjers, I. A., van Gog, T., Wouters, P., and van Merriënboer, J. J. (2012). Explaining the segmentation effect in learning from animations: the role of pausing and temporal cueing. Comput. Educ. 59, 274–280. doi: 10.1016/j.compedu.2011.12.024
Steinbeck, C., Hoppe, C., Kuhn, S., Floris, M., Guha, R., and Willighagen, E. L. (2006). Recent developments of the chemistry development kit (CDK) - an open-source java library for chemo- and bioinformatics. Curr. Pharm. Des. 12, 2111–2120. doi: 10.2174/138161206777585274
Striewe, M. (2016). An architecture for modular grading and feedback generation for complex exercises. Sci. Comput. Program. 129, 35–47. doi: 10.1016/j.scico.2016.02.009
Sweller, J. (1988). Cognitive load during problem solving: effects on learning. Cogn. Sci. 12, 257–285. doi: 10.1207/s15516709cog1202_4
Sweller, J. (2010). Element interactivity and intrinsic, extraneous, and germane cognitive load. Educ. Psychol. Rev. 22, 123–138. doi: 10.1007/s10648-010-9128-5
Sweller, J., van Merrienboer, J. J. G., and Paas, F. G. W. C. (1998). Cognitive architecture and instructional design. Educ. Psychol. Rev. 10, 251–296. doi: 10.1023/A:1022193728205
Sweller, J., van Merriënboer, J. J. G., and Paas, F. (2019). Cognitive architecture and instructional design: 20 years later. Educ. Psychol. Rev. 31, 261–292. doi: 10.1007/s10648-019-09465-5
Taskin, V., Bernholt, S., and Parchmann, I. (2017). Student teachers’ knowledge about chemical representations. Int. J. Sci. Math. Educ. 15, 39–55. doi: 10.1007/s10763-015-9672-z
van Alten, D. C., Phielix, C., Janssen, J., and Kester, L. (2019). Effects of flipping the classroom on learning outcomes and satisfaction: a meta-analysis. Educ. Res. Rev. 28, 100281–100218. doi: 10.1016/j.edurev.2019.05.003
van Gog, T., Kirschner, F., Kester, L., and Paas, F. (2012). Timing and frequency of mental effort measurement: evidence in favour of repeated measures. Appl. Cogn. Psychol. 26, 833–839. doi: 10.1002/acp.2883
WileyPLUS and University of California. (2023). Reaction Explorer: Synthesis Explorer and Mechanism Explorer Reaction Tutorial System. Available at: https://cdb.ics.uci.edu/cgibin/tutorial/ReactionTutorialSetupWeb.py (Accessed July 20, 2023).
Keywords: e-learning system, technology-enhanced assessment, higher education, cognitive load, organic chemistry, feedback
Citation: Schuessler K, Striewe M, Pueschner D, Luetzen A, Goedicke M, Giese M and Walpuski M (2024) Developing and evaluating an e-learning and e-assessment tool for organic chemistry in higher education. Front. Educ. 9:1355078. doi: 10.3389/feduc.2024.1355078
Edited by:
Gautam Bhattacharyya, Missouri State University, United StatesReviewed by:
Pamela Lundin, High Point University, United StatesJosé Hernández-Ramos, Metropolitan University of Educational Sciences, Chile
Copyright © 2024 Schuessler, Striewe, Pueschner, Luetzen, Goedicke, Giese and Walpuski. This is an open-access article distributed under the terms of the Creative Commons Attribution License (CC BY). The use, distribution or reproduction in other forums is permitted, provided the original author(s) and the copyright owner(s) are credited and that the original publication in this journal is cited, in accordance with accepted academic practice. No use, distribution or reproduction is permitted which does not comply with these terms.
*Correspondence: Katrin Schuessler, katrin.schuessler@uni-due.de