- 1RDI & Competences, Haaga-Helia University of Applied Sciences, Helsinki, Finland
- 2Haaga-Helia School of Vocational Teacher Education, Haaga-Helia University of Applied Sciences, Helsinki, Finland
- 3International Business, Haaga-Helia University of Applied Sciences, Helsinki, Finland
- 4Department of Social Sciences, University of Eastern Finland, Kuopio, Finland
Introduction: The purpose of this empirical research was to map the capabilities and perceptions of undergraduate business administration students about artificial intelligence (AI) and its potential to answer questions related to sustainable transition in society, and to obtain information about the suitable pedagogical solution to increase the knowledge and understanding related to these themes.
Methods: The data was gathered among higher education (HE) students in a workshop that consisted of introductory lecture, answering surveys, questionnaire, group discussions, and reflective narratives on the relationship and possibilities of AI and sustainable development. In data analysis an abductive qualitative research methodology was adopted.
Results: Through abduction new insights were obtained and new knowledge was created new knowledge regarding AI literacy in the context of sustainable development. This brought new knowledge in the context of HE studies. The taxonomy of AI literacy in sustainable development created a new reference framework for learning tasks, and course planning in HE. The findings showed that the students had difficulties solving the actual problem because they lacked knowledge and understanding of the basics of AI and sustainable development. However, in groups where one person had a deeper understanding of the concepts, the whole group began to understand the task and work on both meta-level ethical questions and practical examples.
Discussion: The assistance of AI potentially creates opportunities for developing solutions supporting sustainable development. However, utilizing this potential requires AI literacy. In this task HE plays a significant role. This study contributes to the pedagogical approach where AI and sustainable development are integrated in HE curricula.
1 Introduction
The escalating global crises have created a remarkable threats to civilization. Human-induced climate change, all the depletion of natural resources, and declining biodiversity call for actions. Effective solutions are needed at all levels of society and the global community. The versatile utilization of Artificial Intelligence (AI) and fast development of AI literacy have raised both hope and fear. This study is motivated by the question we have asked ourselves: what pedagogical solutions should be developed within Higher Education (HE) to incorporate the needs of the 21st century whilst embracing AI literacy?
The definition of AI literacy is still evolving and there is no generally accepted definition thereof (Laupichler et al., 2022). One definition suggests it could be “a set of competencies that enables individuals to critically evaluate AI technologies; communicate and collaborate effectively with AI; and use AI as a tool online, at home, and in the workplace” (Long and Magerko, 2020). Thus, AI literacy refers to capability that enables people to deal with artificial intelligence, enhanced solutions or products, thus enabling them to use basic AI-powered software and evaluate its impact on humans, societies and the planet.
It is difficult to recognize the operation of artificial intelligence in everyday life. The development of artificial intelligence has been fast, and it brought about consequences, not yet understood. Following AI uncritically may lead to dichotomous thinking and one-dimensional solutions. Awareness and knowledge of AI helps to guide its use for ethically weighed purposes. For example, privacy concerns while using digital services can present dangers while AI is used (Alamäki et al., 2023). On the other hand, awareness and knowledge of AI may help to guide its use for ethically weighed purposes. The task of education is to correct biases and increase inclusion in utilizing AI. The use, awareness and development of AI is still the activity of a small group, although the use is extensive. The application of AI is also globally very unevenly distributed.
This study focuses on challenges from the viewpoint of AI literacy in sustainable development in the context of HE. Prior research on AI literacy is scarce, and there is little or any study about the characteristics of AI literacy in the context of sustainable development. Therefore, in this study we focus on the state of AI literacy in the context of HE institutions with the aim of obtaining not only a situational picture but also with the aim of creating pedagogical models that will help promoting artificial intelligence literacy in teaching and learning. The purpose of the study is to map the current capabilities and perceptions of the HE students about artificial intelligence and its potential to answer questions related to sustainability transition in society. The study also aims at obtaining information about the suitable pedagogical solutions for increasing the knowledge and understanding related to these themes.
2 Theoretical framework
2.1 Sustainable development
Sustainable development is an imperative of our time. Humans’ pursuit of good life permanently changes the socio-ecological system on which our everyday life depends (Steffen et al., 2015; Figueres et al., 2017; Ripple et al., 2017). This is a reason the period between 2005 and 2014 marked a decade of “Education for Sustainable Development” (UNDESD), which emphasized the role of education in global sustainable development. After that period, in September 2015, the United Nations (UN) formulated and adopted the 17 Sustainable Development Goals (SDGs). Whereas the former promoted implementation of overall quality of education that would ensure a sustainable future, the latter (SDG 4) called for quality education for all, rooted in the Universal Declaration of Human Rights.
SDG 4 calls for ensuring “inclusive and equitable quality education” and promoting “lifelong learning opportunities for all.” It also calls for ensuring that the acquisition of skills and knowledge, vital to leading sustainable lives, is available to all. Therefore, the role of education, especially in promoting communication, research and innovations, access to information and networking opportunities are critical strategies for achieving the SDGs. HE faces fundamental questions about re-skilling and updating people’s competencies and ways of pursuing a good life without compromising future generations’ ability to meet their needs (Redecker et al., 2011). In practical terms, this means ensuring the pedagogical solutions for acquiring key competencies of 21st century, such as sustainable lifestyles, work, and habitat (Van den Branden, 2015) whilst utilizing fossil-free renewable resources.
Research on the integration of AI and sustainable development has rapidly increased during the last few years (Leal Filho et al., 2023). The advancements of AI provide several significant technological opportunities to sustainable development and its initiatives (Goralski and Tan, 2020; Vinuesa et al., 2020), and their integration is also a main priority of policymakers (e.g., Gailhofer et al., 2021).
2.2 Artificial intelligence literacy
Gašević et al. (2023) raise key questions regarding AI literacy: (1) the knowledge of AI among students, teachers, faculties, and general public, (2) the potential of AI, (3) AI’s implications on individuals and societies. Most definitions of AI literacy focus on diverse types of “literacies” and those definitions have skill sets in varied disciplines (Ng et al., 2021a). Ng et al. (2021b) classified AI literacy into four aspects which are: to know and understand, to use and apply, to evaluate and create, and to be aware of ethical issues. Those four aspects represent also the level of capabilities that individuals have whilst dealing with AI applications. A challenge in the current conceptualization of AI literacy is its application and usage-orientation and their lack of pedagogical guidance. However, individuals should also have capabilities to critically evaluate the impacts and effects of AI as a part of social, economic, and environmental context, not only from ethical viewpoint but also a broad perspective, as AI will be an integral part of our everyday life in any sector. AI is often referred to as a computational agent (Alamäki et al., 2019), thus it is not just a technological application, but a significant actor of communities, environments, and societies. Yi (2021) connects metacognition as a primacy competence to AI literacy, whose aim is to assist individuals in anticipation of the future of AI by adopting functional, social, and technology literacies in this process.
Educators and teachers should have awareness and understanding of the AI literacy skills, essential abilities for AI-human interaction in developing and integrating curriculum into educational practices (Nguyen et al., 2022). AI literacy is seen as a generic learning skill in education (Laupichler et al., 2022; Su and Ng, 2023). In addition to educators and teachers, students should develop awareness and understanding related to AI from various viewpoints. For example, AI literacy improves students’ abilities to evaluate fairness, accountability, transparency, ethics, and safety of AI (Ng et al., 2021b). Yi (2021) defines AI literacy as the basic ability that helps individuals to become independent citizens in the AI era. Hornberger et al. (2023), in a study in Germany, found that students with prior experience in AI or studies in computer science or STEM studies had a higher level of AI literacy than students with other backgrounds. They also revealed that most students were interested in learning more about AI and they had a positive attitude toward AI.
The capability of AI literacy should be seen as the end of education which aims to increase students’ capability to evaluate AI’s various features and consequences. Students already have an opportunity to adopt AI solutions in their studies (e.g., Mononen et al., 2023) which requires technological capabilities to use AI in their studies and daily life. AI literacy requires technological capability which is the combination of ability and motivation (c.f. Kimbell et al., 1996) that promotes understanding and enables use of AI in responsible and sustainable ways.
Technological capability, as a concept, is embedded in many areas from equipment to human skills (Archibugi and Coco, 2005). It is also seen as the potential for efficient, practical, and quality work in designing technological solutions (Petrina, 1998). Further, it is also associated with knowledge-based competencies at the firm level (Bustinza et al., 2019). Technological capability combines technological knowledge and understanding about concepts and principles, and personal qualities and technological skills (Alamäki, 2018). Thus, a technologically capable student is a technologically literate one. Similarly, a student capable of understanding basic concepts and principles related to AI, and responsible for using or adapting AI can be considered as an AI literate person.
In this empirical study we were interested in the level of awareness and knowledge of the role of AI among young undergraduate HE students. Secondly, we were interested in gauging the participants’ awareness of the future of AI and its role in their future professional life. We also wanted to identify how the participants valued the ethical impact of AI in the future professional life. As there are no established learning goals for AI literacy yet, we utilised the already defined learning goals of technology literacy ITEA (2007). ITEA has defined the standards of technological literacy for K12 education in the United States and they are widely used taxonomies across western countries. ITEA’s definition contains 20 standards for technological literacy that are the main learning objectives for students’ skill acquisition. These standards are formulated in five generic learning goals: (1) understanding of the nature of technology, (2) understanding of technology and society, (3) understanding of design, (4) having abilities for technological world, (5) understanding of designed world in selecting and using various technologies. These learning goals are adapted to our AI literacy model. In addition to utilizing ITEA’s (2007) model of technological literacy, we adapted the revised Bloom’s taxonomy (Krathwohl, 2002) in our conceptualization of AI and sustainable development in education taxonomy. The Bloom’s taxonomy provides cumulative hierarchy of learning objects where students proceed from basic knowledge and understanding to higher level of capabilities such as analyzing, synthetizing, creation, and evaluation. This revised Bloom taxonomy emphasizes the meta that is essential in ensuring continuous learning and self-directed critical thinking and reflection.
Our specific research questions were: Q1: What do undergraduate students understand of AI? Q2: How did the workshop promote understanding of the potential of artificial intelligence in solving challenges related to sustainable development? Q3: What are the greatest challenges in enhancing AI literacy in higher education context, and what pedagogical solutions could be useful?
3 Methodology
3.1 Data gathering
The participants of our study were 22 first semester undergraduate business administration students following a BBA English language program. The group was multicultural and multigender. We assured the students of the data’s confidentiality and obtained permission to use it for the study’s purpose.
The research data was gathered in a workshop that consisted of several parts. At the beginning of the workshop, the students were asked to fill in a questionnaire where they answered several questions concerning their current knowledge, understanding and perceptions of artificial intelligence and its use in solving challenges related to sustainable development. The students were also asked to assess the visibility of these themes in their studies, as well as their expectations for their future employers regarding sustainability issues.
After that, the students were introduced to the basics of AI and the concept of AI literacy. We described the concept of AI to students by presenting it as the capability of a digital computer or computer-controlled robot that performs tasks commonly associated with intelligent beings (Copeland, 2023). We also extended this definition by presenting three other practical short definitions of AI which described AI as intelligent entity, independent executor and rational actor. In addition, we presented the following abilities of AI: it performing human-like tasks, enables creation of real-time controlling and monitoring systems, ability enables building automatic guidance and support for humans and making predictions and recommendations for decision-making (Table 1).
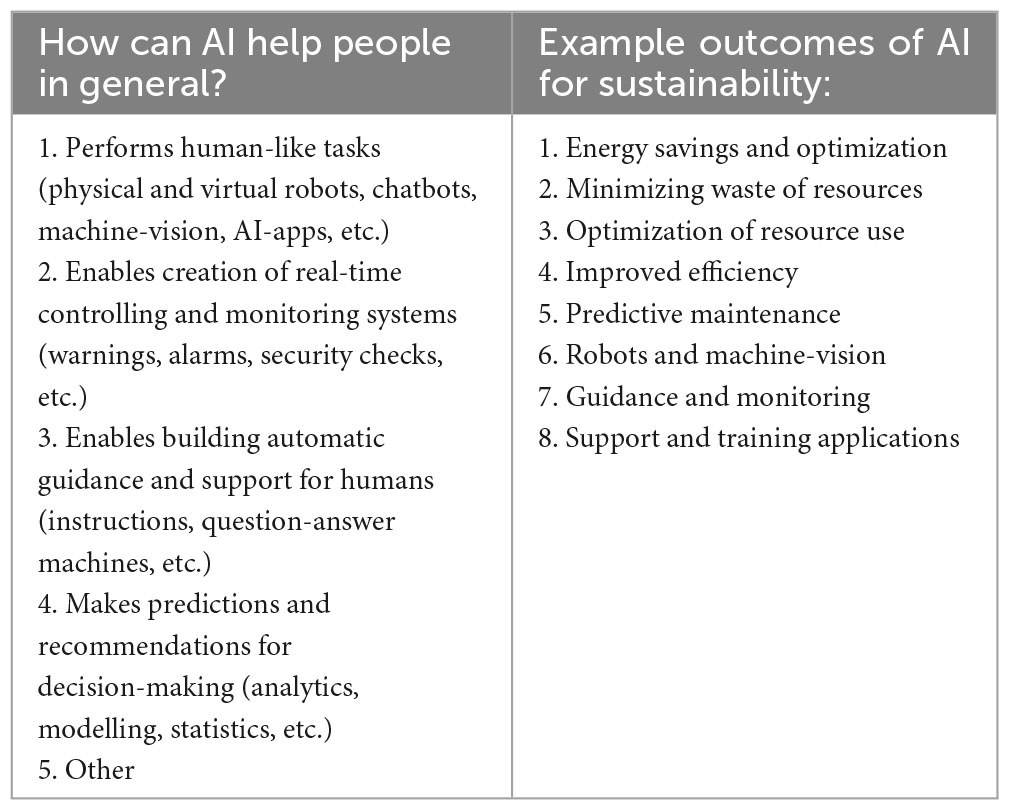
Table 1. The generic illustrative examples that we presented to students to assist them to accomplish the group work assignment.
The goal of presenting the practical definitions and abilities of AI was to make sure that the students have similar understanding of AI and its abilities. Then, in groups of 4–5 students were asked to share and discuss their answers related to various levels of understanding and application of artificial intelligence.
In defining “definition in sustainable development context” (see column 3 Table 2) we suggested the students get familiar with UN Sustainable Development Goals 1–17 and ideate a definition for it in small groups. The students were able to create concrete examples of how AI could provide value for sustainable development. We used the levels of AI literacy and their definitions according to the study of Ng et al. (2021b).
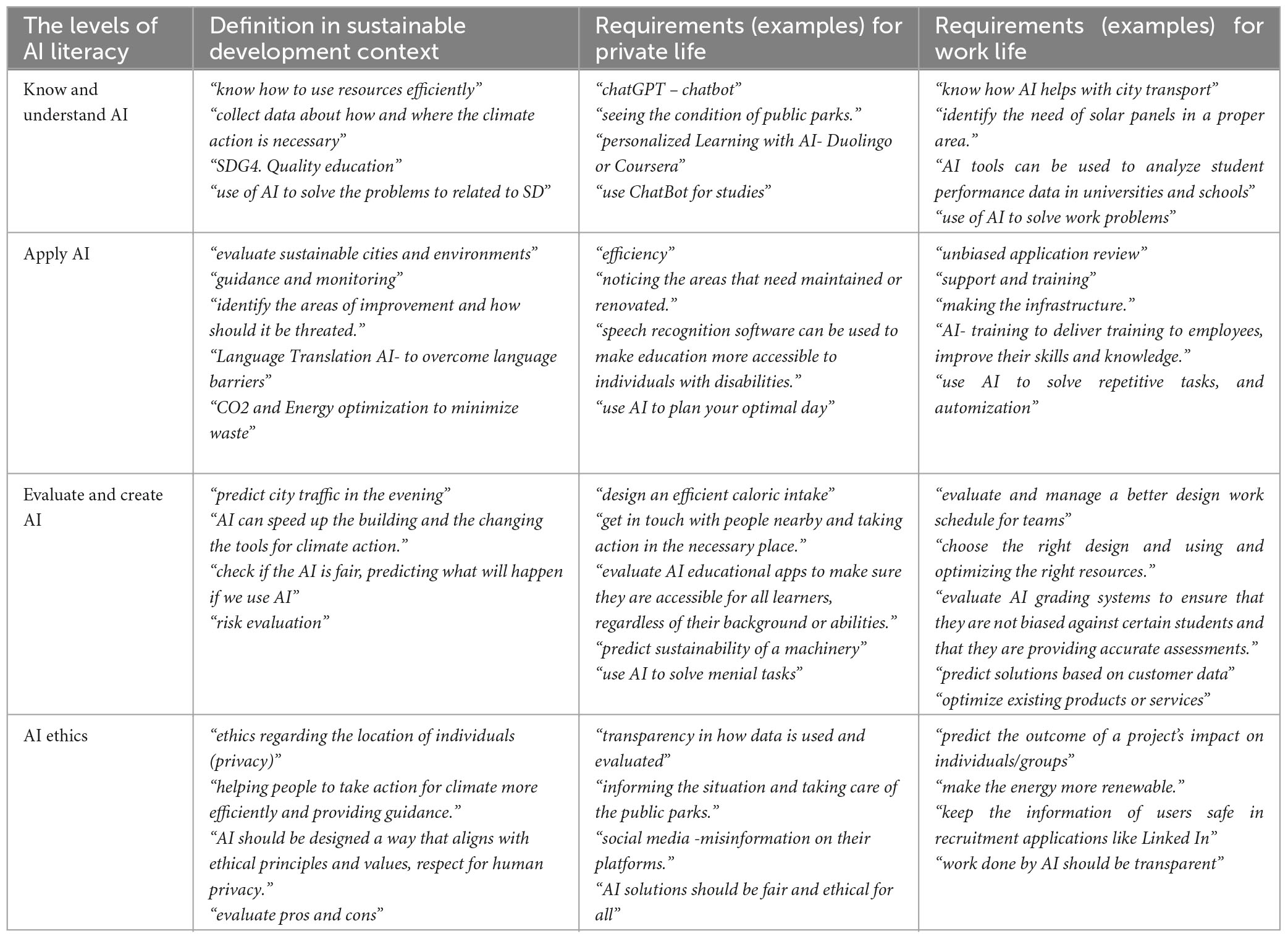
Table 2. Artificial Intelligence (AI) literacy (Ng et al., 2021b) for sustainable development with the example ideas of student groups.
In the final part of the workshop the students filled out another questionnaire inquiring into their learning experiences. The questions asked for the students’ reflections and evaluations on their current experiences, knowledge, skills and expectations regarding artificial intelligence and sustainability. They were also asked to reflect on their learning experiences, in short narratives. Their answers gave us insights and allowed us to assess their knowledge of the role of AI in everyday situations.
3.2 Data analysis
We adopted an abductive qualitative research methodology approach while analyzing data (Dubois and Gadde, 2002). The characteristics of abduction in research are logical and scientific inferences that extend into the realm of profound insight which generates new knowledge (Reichertz, 2004). In practical terms, through the perspectival approaches of explanatory abduction (Alvesson and Sköldberg, 2009) we simultaneously processed the theoretical framework and analyzed empirical data in an iterative manner. Thus, through abduction, we created insights regarding AI literacy in the context of sustainable development, and such knowledge has not before associated with each other in educational studies.
The reflective learning narratives were analyzed using thematic analysis, and following method suggested by Gioia et al. (2013). The themes were first identified, coded (Saldana, 2009), and categorized into first level categories. Thus, analyzing open-ended responses of the pre- and post-questionnaires, open coding was applied without pre-defined coding categories for the literature review. This facilitated an understanding of AI literacy in the sustainable development context (Strauss and Corbin, 1998).
In practice, we carried out the data analysis in such a way that first, we carefully read through all the writings to understand them as complete narratives. After this, we identified thoughts, sentences, or parts of sentences related to the same themes from the respondents’ writings. We combined these thoughts, sentences, or parts of sentences into categories. After that, we continued by breaking down the categories identified from the material into subcategories, generic categories, and further into main categories, which form the answers to our research questions (Elo and Kyngäs, 2008). We did not quantify the material while analyzing it. We did not count the number of expressed thoughts, sentences, or parts of sentences. Still, we identified the differences and similarities of the expressions used by the respondents when comparing them to others (Silverman, 1993).
In the beginning of the first data analysis round, we had an initial conceptual understanding of the AI literacy and sustainable development literacy. From this perspective, we started the data analysis by analyzing the pre-questionnaire and the workshop results. The current understanding and capabilities of the students’ AI knowledge and understanding in sustainable development redirected our study both theoretically and empirically. Based on our empirical observations from the workshop and students’ material produced in the workshop, our interests turned to the designing of the AI literacy taxonomy in the sustainable development context. First, we used the results of empirical workshop where the students applied their current AI understanding to sustainable development cases. In the second phase of the analysis, we focused on specific educational goals, learning objectives and perspectives of sustainable development of AI literacy whilst forming the new taxonomy in this field. Based on the created AI literacy in sustainable development taxonomy (Table 3), we developed pedagogical model that identified distinct types of teaching practices that relate to education of AI literacy in sustainable development.
4 Results
4.1 What do undergraduate students understand about AI?
The thematic analysis of the learning narratives completed at the beginning of the workshop revealed the following categories.
Limited knowledge of an important subject. The students’ assessments of their own skills in relation to artificial intelligence were realistically critical. The importance of the matter was recognized, but the limitations of one’s own skills were also acknowledged. The skills identified by the students were limited to awareness of the role of artificial intelligence, and the usage of the skills in some contexts. The lack of deeper understanding was clearly recognized. The students who followed additional courses in AI evaluated their skills to be better.
“Occasionally we use tools that utilize AI to handle information.” I like to think about philosophical way of it but not any competence whatsoever.” “I don’t think I’ve really consciously done anything AI related.” “I have no expertise related to AI whatsoever.” “I have done a course on AI.”
Acknowledgments of both the value of AI and human agency. The students’ reflections conveyed an enlightened understanding of the effectiveness of AI, but also its dependence on human thinking. Although the students believed in the usefulness of artificial intelligence, they also emphasized the importance of human decision-making.
“I would not consider AI to be the absolute solver of world problems. AI is only a tool for professionals to help them solve problems.” “AI is a tool to execute the plan.” “AI is programmed intelligence.” “Yes, partially AI can help solving these problems, but I think we need also human creativity.”
Absence of AI in the curriculum. The reflections clearly conveyed the view that AI has not been discussed in the studies. A few students mentioned elements of AI in their studies, but their learning took place outside of the regular studies included in the curriculum.
“We do not have any classes or studies on AI. All I know is coming from individual research.” “I started to need AI more, so I got better. “I have learned a lot from my classmates during these three months.” “Schools could do a much better job at teaching and increasing our knowledge of AI.” “Nobody talked about AI until today.”
Varied levels of presence of sustainability in the curriculum. The reflections showed strong disagreements about the impact of the studies on the understanding of promotion of sustainable development. Some students gained a lot of understanding of sustainable development from their studies, some none at all. The explanations suggested that those students who were interested in the issue both recognized the related themes in their studies and applied for courses that focused specifically on the themes of sustainability.
“I have learned about methods much.” “Since I started my studies I learnt a lot about sustainable development.” “It is always appreciated but my studies are not related to the matter.” “Most projects are based on sustainability.” “Maybe a little bit.” “In every subject, teachers always emphasize on the importance of developing sustainability and how to make it effectively.” “Sustainable development hasn’t been a hot topic during my studies.”
Importance of sustainability promoted by future employers. Some reflective narratives showed strong positions for and some against the impact the future employers should exert on sustainability. In general, the employer’s actions were considered especially important. On the other hand, the students were ready to compromise their ideals when faced with the realities of life. They stressed the importance of the opportunities to choose potential employers, whilst applying for work. If they need work urgently, the students are more ready to compromise on the demands regarding the employer’s actions to promote sustainable development.
“It would be a decisive feature for me because it shows the value of the company.” “It is a hard decision that I can make based on the situation.” “As a jobless student the main motivation is to find work no matter what the company is.” “It would be a positive metric but not something I consider a priority.” “It will certainly be a plus.”
Our analysis also revealed the students considered their future employer’s actions to promote sustainable development to be important. The importance of the issue was also emphasized when the ratings were lower, but the lower rating was based on scepticism about companies’ desire to promote sustainability or emphasizing the issue as a positive addition, but not necessary.
“…I do not know much about such topic. But it is important for my future employer to discuss sd for me.” “Of course, I hope they take those things seriously, but it is not mandatory.” “It is definitely important, But not the biggest factor for me.” “Employers actions do have direct influence on earth.” “I want to… promote sustainability by the best of my ability.”
4.2 How did the workshop promote understanding of the possibilities of artificial intelligence to solve challenges related to sustainable development?
During the part of the workshop where the students worked in groups the students were asked to elaborate on the connections between AI and sustainable development. They were able to create concrete examples of how AI could provide value for sustainable development. However, they did not link them to the SDGs except for two of the groups. The students also had difficulties ideating definitions based on the hierarchical levels of AI literacy, namely knowing, and understanding AI, applying AI, evaluating, and creating AI and AI ethics. This showed that we should put special focus on the hierarchical levels of AI literacy if we use the same template in the same way. The ideas for the requirements for private and work life provided concrete suggestions (columns 4–5 in Table 2). This indicated that the students can create innovative ideas for AI-based value creation opportunities in sustainable development. Although the connection to the hierarchical levels of AI literacy was missing in most outcomes, the examples showed that the students were able to define how AI will create value for different SDGs.
4.3 What are the greatest challenges in enhancing AI literacy in higher education context, and what kind of pedagogical solutions could be useful?
When asked about the value of the workshop in knowledge creation and understanding of the potential of AI to enhance sustainable development twelve students (out of 22) acknowledged gaining more knowledge about AI. The examples below illustrate the students learning.
“I came to know the different abilities of AI that we can use to prevent climate change and to enhance sustainable development”. “I did some research about AI and see it extremely fascinating.” “Yes, I learned about it through my discussion with my teammate.”
On the other hand, ten students described their learning as follows:
“I did not really (learn). I would need to learn about the whole concept first before I can combine sustainability to it.” “The workshop confused me.”
When asked about their assessment of their learning on a scale 1–5, on average the students rated their learning as 2, 1. When asked for suggestions for workshop improvement from the learning perspective, the students pointed out a lack of prior introduction to the subject. They assessed the introductory lecture as general and expressed the need for more in-depth lecture on AI and sustainability in general. They also mentioned confusion due to the lack of clarity of the instructions and questions in the assignments. The following excerpts illustrate the students’ suggestions:
“Give us more examples on how AI is used in real life. Define AI better for the ones of us who have no knowledge of it, so working on the issues would be easier.” “I think it is a clever idea to have a lecture about AI before this workshop.”
When asked about the most useful parts of the workshop they mentioned the possibility of opening the topic, learning about it, as well as brainstorming, researching, and discussing it with their peers.
“The evaluation part because it helped me to reflect on ways AI could help with sustainable development.” “The part where we had to invent ideas, because it made us think more.”
The students evaluated the importance of future employer’s relationship to sustainability and responsibility on average as 3, 2 on a scale 1–5. Some students expressed the importance of caring about the future generations, some expressed the need for earning money as their primary focus.
“I think it’s really important because the issue otherwise is going to be there forever.” “It shows their (employer’s) values when they care about sustainable development.” “I need the work, so sustainability isn’t that important.”
As can be seen from the examples above the students’ learning about AI and sustainability was somehow compromised because of a combination of two factors: students’ various initial knowledge of the concept, and the unclarity of the knowledge and the instructions implemented during the workshop. It can be therefore concluded that when introducing a new concept, a more in-depth, and illustrated by examples lecture should be delivered prior to the workshop. Moreover, the results of the post-evaluation questionnaire showed that the concept of sustainability and its connection to AI was new to the students. However, it must be considered that artificial intelligence and sustainable development are exceptionally difficult topics, for which it can be difficult to assess the depth of the introduction.
Based on identified themes we formed the following pedagogical implications (See Table 3)
The main problem from the pedagogical point of view to be the great variation in students’ prior knowledge. This was a presupposition regarding artificial intelligence, but the weak knowledge of the concept of sustainable development came as a surprise. This was visible in the students’ additional questions and need for help during the workshop. This finding is a result of public debate focusing on the concept of climate change instead of sustainable development.
5 Discussion and concluding remarks
5.1 Conceptual model for AI literacy for sustainable development
The opportunities of artificial intelligence and the goals of sustainable development are not mutually exclusive but rather complementary. AI is a significant technological advancement in information and communication technologies. AI will also significantly change work, education and health care in the near future. From the perspective of sustainable development, many expectations are placed on the opportunities of AI. It can produce new necessary information that otherwise could not be found or analyzed in the environmentally oriented processes. Thus, it will be an important method and tool in sustainable development. This sets new requirements for HE as it is essential to provide skills, such as how AI and sustainable development could be combined in innovative ways. In this study, we have conceptualized this phenomenon through the concept of AI literacy in sustainable development.
Since there is no widely accepted consensus or guidelines for defining learning goals of AI literacy, we adapted the relevant learning goals of technological literacy to our conceptualization. Whilst defining the taxonomy of AI literacy for sustainable development, we adapted the ITEA’s (2007) standards that were widely accepted taxonomies across western countries. ITEA (2007) defined the standards of technological literacy for K12 education in the United States, which implemented them across the states in the USA. They defined 20 standards for technological literacy that are the main learning objectives that students will know or master to fulfill their main goal. The standards are crystalized to five generic learning goals: (1) understanding of the nature of technology, (2) understanding of technology and society, (3) understanding of design, (4) abilities for technological world, and (5) understanding of designed world in selecting and using various technologies.
We adapted those learning goals to our AI literacy model (Table 4). Interestingly, ITEAs (2007) standards fit well to our model but they did not contribute much to the higher level of goals and objectives in our model. Unlike ITEA’s (2007) standards that are for K12 education, our model is primarily designed for higher education. In addition to ITEA’s (2007) conceptualization of technological literacy, we adapted the revised Bloom’s taxonomy (Krathwohl, 2002) in our AI literacy in education and sustainable development taxonomy. The Bloom’s taxonomy is a widely used taxonomy that provides levels to conceptualize the learning objects into the cumulative hierarchy where students first need to learn basic knowledge and understanding in order, they can learn higher level of capabilities such as analyzing, synthetizing, creation, and evaluation. The revised Bloom’s taxonomy emphasizes the ability to metacognition that is the highest-level capability that ensures continuous learning and self-directed critical thinking and reflection.
5.2 Pedagogical model for teaching AI literacy for sustainable development
We adopted the problem-based learning approach in the experiment. Successful problem solving requires basic knowledge and understanding of the phenomenon to be solved. The results of this study showed that the students had difficulties solving the actual problem because they lacked knowledge and understanding of the basics of artificial intelligence and sustainable development. As the revised Bloom’s taxonomy (Krathwohl, 2002) shows, a basic knowledge and understanding of the topic are needed before a solution can be found. Those basic elements of AI literacy help students to further analyze and evaluate the phenomenon, and question its current situation critically. This is also a prerequisite for creating a new solution which was also a learning goal in the workshop. In fact, the students were able to create new use cases and thus solve problems, but they still felt that they should have had better basic technological capabilities regarding the opportunities of artificial intelligence.
Our findings also pointed out to the students’ need of more time to solve similar learning assignments, which would make it possible to find out needed knowledge base through self-directed learning and instructor-led teaching. The difference between the groups was noticeable when the understanding of artificial intelligence was clearer. This was reflected in the ability to answer meta-level questions and in finding practical examples. When the basic concepts were unclear, the groups could not move forward and became frustrated. This was reflected in the answers. Instead, in groups where one person had a deeper understanding of the concepts, the whole group began to understand the task and work on both meta-level ethical questions and practical examples.
In higher education pedagogy, it is essential to teach students higher-level thinking. The findings show that students can evaluate their competences and shortcomings in relation to the workshop’s assignment. Such metacognitive skills are important learning objectives. The assignment in the workshop made the students reflect on their own knowledge in relation to a very current social and environmental topic, namely AI-related problem solving in the sustainable development context. This study revealed that similar problem-solving-oriented methods could work elsewhere. The taxonomy of AI literacy in sustainable development brings a new reference framework for learning task and course planning in higher education, which also helps to prepare scaffolding-type learning methods where students solve problems in this context.
AI literacy is a fascinating concept in the context of sustainable development. For example, the relationship of technology to, for example, the themes of climate change and social justice is contradictory. On the one hand, it has been the accelerator of many unfavorable developments, but also a savior in the field of medicine, for example. The possibilities and dangers of artificial intelligence are an emerging field, and therefore a particularly important and fascinating subject of research and pedagogical development.
An essential starting point of our study was to get an overview on the capabilities university students have regarding the operation and role of AI in society, as well as AI’s possibilities to solve the great challenges of our time. Complex social-ecological challenges such as climate change, biodiversity loss and global social inequality require in-depth sustainability transformations, across all sectors, scales and actors. It was expected that the skills would vary, but the uncertainty about the concepts and the variation in the skills were surprising. This is a strong signal that there is an urgent need for further research and new pedagogical ideas. More research is needed on how to develop learning to learn and critical thinking skills for life-long learning that is needed in rapidly developing AI-based environment. We also encourage educational researchers to study how students could develop their metacognitive skills to be able to manage AI-enabled world in the future.
Data availability statement
The raw data supporting the conclusions of this article will be made available by the authors, without undue reservation.
Ethics statement
Ethical approval was not required for the studies involving humans because the questionnaires were delivered anonymously. The studies were conducted in accordance with the local legislation and institutional requirements. The participants provided their written informed consent to participate in this study. Written informed consent was obtained from the individual(s) for the publication of any potentially identifiable images or data included in this article.
Author contributions
AA: Conceptualization, Data curation, Formal analysis, Investigation, Methodology, Writing – original draft. CN: Conceptualization, Data curation, Formal analysis, Investigation, Methodology, Project administration, Writing – original draft. AK: Methodology, Analysis, Writing – review & editing. AS: Formal analysis, Methodology, Writing – review & editing.
Funding
The author(s) declare financial support was received for the research, authorship, and/or publication of this article. The research was funded by two grants from Ministry of Education and Culture, Finland: Grant No. OKM/117/523/2020 (Ethical Competencies for Sustainability in Higher Education and Working Life) and Grant No. OKM/108/523/2021 (AI Driver Project).
Conflict of interest
The authors declare that the research was conducted in the absence of any commercial or financial relationships that could be construed as a potential conflict of interest.
Publisher’s note
All claims expressed in this article are solely those of the authors and do not necessarily represent those of their affiliated organizations, or those of the publisher, the editors and the reviewers. Any product that may be evaluated in this article, or claim that may be made by its manufacturer, is not guaranteed or endorsed by the publisher.
References
Alamäki, A. (2018). A conceptual model for knowledge dimensions and processes in design and technology projects. Int. J. Technol. Design Educ. 28, 667–683.
Alamäki, A., Aunimo, L., Ketamo, H., and Parvinen, L. (2019). “Interactive machine learning: Managing information richness in highly anonymized conversation data,” in Proceedings of the Collaborative Networks and Digital Transformation, PRO-VE 2019, (Cham: Springer International Publishing), 173–184.
Alamäki, A., Mäki, M., and Kauttonen, J. (2023). How students’ information sensitivity, privacy trade-offs, and stages of customer journey affect consent to utilize personal data. Interdiscip. J. Inform. Knowl. Manag. 18, 127–147.
Alvesson, M., and Sköldberg, K. (2009). Reflexive methodology: New vistas for qualitative research, 2nd Edn. London: Sage.
Archibugi, D., and Coco, A. (2005). Measuring technological capabilities at the country level: A survey and a menu for choice. Res. Policy 34, 175–194.
Bustinza, O. F., Vendrell-Herrero, F., Perez-Arostegui, M., and Parry, G. (2019). Technological capabilities, resilience capabilities and organizational effectiveness. Int. J. Hum. Resour. Manag. 30, 1370–1392.
Copeland, B. J. (2023). Artificial intelligence. Encyclopedia britannica. Available online at: https://www.britannica.com/technology/artificial-intelligence
Dubois, A., and Gadde, L. E. (2002). Systematic combining: An abductive approach to case research. J. Bus. Res. 55, 553–560.
Elo, S., and Kyngäs, H. (2008). The qualitative content analysis process. J. Adv. Nurs. 62, 107–115.
Figueres, C., Schellnhuber, H., Whiteman, G., Rockström, J., Hobley, A., and Rahmstorf, S. (2017). Three years to safeguard our climate. Nature 546, 593–595.
Gailhofer, P., Herold, A., Schemmel, J., Scherf, C., Urrutia, C., Köhler, A., et al. (2021). The role of artificial intelligence in the european green deal. Luxembourg: European Parliament.
Gašević, D., Siemens, G., and Sadiq, S. (2023). Empowering learners for the age of artificial intelligence. Comput. Educ. Artific. Intellig. 4:100130.
Gioia, D. A., Corley, K. G., and Hamilton, A. L. (2013). Seeking qualitative rigor in inductive research: Notes on the gioia methodology. Organ. Res. Methods 16, 15–31. doi: 10.1177/1094428112452151
Goralski, M. A., and Tan, T. K. (2020). Artificial intelligence and sustainable development. Int. J. Manag. Educ. 18:100330.
Hornberger, M., Bewersdorff, A., and Nerdel, C. (2023). What do university students know about Artificial Intelligence? Development and validation of an AI literacy test. Comput. Educ. Artific. Intellig. 5:100165. doi: 10.1016/j.caeai.2023.100165
ITEA (2007). Standards for technological literacy: Content for the study of technology, Third Edn. Reston, VA: ITEA International Technology Education Association.
Kimbell, R., Stables, K., and Green, R. (1996). Understanding practice in design and technology. Buckingham: Open University Press.
Laupichler, M. C., Aster, A., and Schirch, J. (2022). Artificial intelligence literacy in higher and adult education: A scoping. Comput. Educ. 3:100101.
Leal Filho, W., Yang, P., Eustachio, J. H. P. P., Azul, A. M., Gellers, J. C., Gielczyk, A., et al. (2023). Deploying digitalisation and artificial intelligence in sustainable development research. Environ. Dev. Sustainabil. 25, 4957–4988.
Long, D., and Magerko, B. (2020). “What is AI literacy? Competencies and design considerations,” in Proceedings of the 2020 CHI conference on human factors in computing systems, (New York, NY: Association for Computing Machinery), 1–16.
Mononen, A., Alamäki, A., Kauttonen, J., Klemetti, A., Passi-Rauste, A., and Ketamo, H. (2023). Forecasted self: AI-based careerbot-service helping students with job market dynamics. Eng. Proc. 39:99. doi: 10.3390/engproc2023039099
Ng, D. T. K., Leung, J. K. L., Chu, K. W. S., and Qiao, M. S. (2021a). AI literacy: Definition, teaching, evaluation, and ethical issues. Proc. Assoc. Inform. Sci. Technol. 58, 504–509.
Ng, D. T. K., Leung, J. K. L., Chu, S. K. W., and Qiao, M. S. (2021b). Conceptualizing AI literacy: An exploratory review. Comput. Educ. Artific. Intellig. 2:100041.
Nguyen, A., Ngo, H. N., Hong, Y., Dang, B., and Nguyen, B. P. T. (2022). Ethical principles for artificial intelligence in education. Educ. Inform. Technol. 28, 4221–4241.
Petrina, S. (1998). “The politics of technological literacy: Toward critical technological literacy,” in Paper Presented at the International Working Seminar of Scholars for Technology Education, (Washington DC: George Washington University).
Redecker, C., Leis, M., Leendertse, M., Punie, Y., Gijsbers, G., Kirschner, P., et al. (2011). The future of learning: Preparing for change. Luxembourg: Publications Office of the European Union.
Reichertz, J. (2004). “Abduction, deduction, and induction in qualitative research,” in Companion to qualitative research, eds U. Flick, E. von Kardorff, and I. Steinke (London: Sage), 159–164.
Ripple, W. J., Wolf, C., Newsome, T. M., Galetti, M., Alamgir, M., Crist, E., et al. (2017) World scientists’ warning to humanity: A second notice. BioScience 67, 1026–1028. doi: 10.1093/biosci/bix125
Saldana, J. (2009). The coding manual for qualitative researchers. Los Angeles, CA: Sage Publications.
Silverman, D. (1993). Interpreting qualitative data. methods for analysing talk, text and interaction. London: SAGE.
Steffen, W., Richardson, K., Rockström, J., Cornell, S. E., Fetzer, I., Bennett, E. M., et al. (2015). Planetary boundaries: Guiding human development on a changing planet. Science 347, 1259855.
Strauss, A., and Corbin, J. M. (1998). Basics of qualitative research: Techniques and procedures for developing grounded theory, 2nd Edn. Thousand Oaks, CA: Sage Publications.
Su, J., and Ng, D. T. K. (2023). Artificial intelligence (AI) literacy in early childhood education: The challenges and opportunities. Comput. Educ. Artific. Intellig. 4:100124.
Van den Branden, K. (2015). Sustainable education: Exploiting students’ energy for learning as a renewable resource. Sustainability 7, 5471–5487. doi: 10.3390/su7055471
Vinuesa, R., Azizpour, H., Leite, I., Balaam, M., Dignum, V., Domisch, S., et al. (2020). The role of artificial intelligence in achieving the sustainable development goals. Nat. Commun. 11, 1–10.
Keywords: artificial intelligence literacy, sustainable development, higher education, pedagogics, artificial intelligence
Citation: Alamäki A, Nyberg C, Kimberley A and Salonen AO (2024) Artificial intelligence literacy in sustainable development: A learning experiment in higher education. Front. Educ. 9:1343406. doi: 10.3389/feduc.2024.1343406
Received: 23 November 2023; Accepted: 31 January 2024;
Published: 06 March 2024.
Edited by:
James Dyke, University of Exeter, United KingdomReviewed by:
Vanessa Camilleri, University of Malta, MaltaOlalekan Akintande, University of Ibadan, Nigeria
Copyright © 2024 Alamäki, Nyberg, Kimberley and Salonen. This is an open-access article distributed under the terms of the Creative Commons Attribution License (CC BY). The use, distribution or reproduction in other forums is permitted, provided the original author(s) and the copyright owner(s) are credited and that the original publication in this journal is cited, in accordance with accepted academic practice. No use, distribution or reproduction is permitted which does not comply with these terms.
*Correspondence: Crister Nyberg, crister.nyberg@haaga-helia.fi