- 1Department of Educational Sciences, Open University, Heerlen, Netherlands
- 2Department Educational Development and Research and the School of Health Professions Education (SHE), Maastricht University, Maastricht, Netherlands
Teachers’ instructions on learning strategies play a key role on how students study. However, how the effectiveness of learning strategies can be matched to different types of knowledge in a diversity of subject content has remained unexplored. In the present study, we related the effectiveness of learning strategies to different types of knowledge through an umbrella review. Furthermore, using focus-groups interviews, we explored the relation between school subjects and these knowledge types. We concluded that the effects of particular learning strategies vary across different learning objectives of the subjects and we offered suggestions for the instruction of effective learning strategies.
Highlights
• The impacts of effective learning strategies differ for different knowledge types;
• Teachers should consider the obtained type of knowledge in their subject before instructing learning strategies;
• School subjects differ in dominant learning objectives and recommended learning strategies;
• Distributed practice is most effective throughout knowledge types, closely followed by retrieval practice.
Introduction
In secondary education, students are tested on a regular basis. Students can use different approaches to prepare for these tests, such as re-reading material, writing summaries, or explaining the learning content to themselves. These preparatory techniques are referred to as learning strategies—some of which are more effective than others (Dunlosky et al., 2013). However, students often choose less effective learning strategies, such as highlighting or re-reading (Blasiman et al., 2017; Dirkx et al., 2019). Teachers can influence students’ learning strategy choice by guiding and instructing them on how to use learning strategies for particular tasks within a subject (Van den Hurk et al., 2016; Coffman et al., 2019). However, the effectiveness of learning strategies can vary across school subjects with different learning objectives. To provide the most suitable advice or instruction on the use of particular learning strategies, teachers require information about which learning strategies are most effective for their subject. To the best of our knowledge, this subject-specific perspective has not yet been represented in current literature. This study aims to fill this gap by connecting effective learning strategies to different learning objectives in school subjects so as to better inform teachers on how to advise students in the use of effective learning strategies. In the following section, we elaborate on different forms of effective learning strategies and introduce the often used knowledge dimension of Bloom’s taxonomy (Anderson et al., 2001), which we applied for analyzing the learning objectives of specific school subjects.
Effective learning strategies
Learning is a challenging skill every student has to face. Students use all sorts of techniques to remember learning content. We define these techniques as learning strategies: “techniques that students could use to improve student learning and achievement” (Dunlosky et al., 2013). These strategies can be either on a cognitive and an metacognitive level (Chamot and O’Malley, 1987). In their review, Dunlosky et al. (2013) assessed the effectiveness of 10 cognitive and metacognitive learning strategies and emphasized the low utility of several popular learning strategies. The current study reviews five learning strategies that were considered to have moderate or high utility by Dunlosky et al. (2013): practice testing, distributed practice, interleaved practice, self-explanation, and elaborative interrogation. There are four reasons to review these strategies in the current study. First, these strategies are easy to use for every student without assistance present during learning. Second, these strategies can be applied without special equipment, thus suitable for every learner. Third, these strategies are potentially applicable for all sorts of learning tasks, in a various domains of learning, in contrast to, for example, strategies that were designed for second language learning. Fourth, empirical evidence for these strategies is available in several educational domains.
The first strategy, practice testing, or retrieval practice, involves students taking tests to retrieve learning content from memory. Practice testing facilitates a better recall of learning content compared to other strategies, such as re-reading (e.g., Agarwal et al., 2012; Rowland, 2014; Adesope et al., 2017). These practice tests can take several forms, including guided or free recall tests (Zaromb and Roediger, 2010), or multiple choice tests (Roediger and Marsh, 2005). Compared to re-study, the positive results of practice testing—a phenomenon known as the testing effect—appear across learning content (Roediger and Karpicke, 2006), students’ age (Meyer and Logan, 2013), school level (Adesope et al., 2017), and even in conditions where practice testing was used as a learning strategy with poor testing quality and no feedback (Roediger and Karpicke, 2006). In the context of a psychology course, Golding et al. (2012) found that students reported their use of flashcards (a common example of retrieval practice in self-study situations) of the study material (i.e., textbook information, notes). Those that reported a heavier use of flashcards scored significantly higher on the final tests (Golding et al., 2012). Moreover, retrieving students’ existing knowledge facilitates the learning of new content. This is often referred to as the forward testing effect (Chan et al., 2006; Yang et al., 2018).
Second, when using distributed practice as a learning strategy, the practice of learning content is spaced out over multiple study sessions, with no learning occurring in between said sessions (Chen et al., 2021). This is known as the spacing gap, which can range from seconds to weeks (Carpenter et al., 2012). Distributed practice is often compared to massed practice, where practice with particular learning content is massed into one single session. Spacing learning sessions over time has been proven to be more effective than massed practice, a phenomenon termed the spacing effect (e.g., Pashler et al., 2007; Carpenter et al., 2012). Bloom and Shuell (1981) provided an example of distributed practice in its application in a regular second language (French) class in high school. Students learned French vocabulary using distributed practice (three 10-min sessions on successive days). A control group learned the same content using massed practice (one 30-min session). The results were similar for both groups in an initial test. However, on a delayed test (four days later), the students who used distributed practice outperformed the control group by 35%.
Third, when using interleaved practice, students alternate between different, but related, types of practice problems. The interleaving effect refers to the benefits of interleaving examples from different types of practice problems (ABCABC) over a blocked presentation (AABBCC) (Brunmair and Richter, 2019). In a typical study on interleaved practice, participants learned to discriminate between the different styles of painters (Kornell and Bjork, 2008). The participants studied landscape paintings from 12 artists, either in a blocked or interleaved presentation. The participants were then asked to determine the artist of non-studied landscaped paintings. Interleaved practice facilitated a better recognition of painters’ styles. Interestingly, the participants themselves preferred the blocked learning strategy, despite achieving a better performance under the interleaved strategy.
Fourth, when using self-explanation as a study strategy, students explain the meaning and relevance of learning content to themselves. In doing so, students connect to-be-learned material to earlier learned content (Bisra et al., 2018). This makes self-explanation a more effective strategy than re-reading (e.g., Griffin et al., 2008). Self-explanation can be either prompted (Crippen and Earl, 2007) or initiated by the student (Moreno et al., 2005). The content of the explanations generated by students varies. For example, they can refer to how a concept works (Siegler and Chen, 2008) or explain tangible examples (Berthold et al., 2009). Chi et al. (1994) asked students to use self-explanation after reading each line of an expository text. A control group used re-reading as a learning strategy. A post-test showed that the extent to which students used self-explanation was a predictor for text recall and understanding.
Fifth, when using elaborative interrogation, students process to-be-learned material by asking themselves why a specific stated fact is true (Wood et al., 1990). On one hand, elaborative interrogation helps to link new information to prior knowledge. On the other, elaborative interrogation facilitates discrimination between knowledge. Both processes not only enable recall, but also the correct use of knowledge given the circumstances (Dunlosky et al., 2013). For example, Woloshyn et al. (1994) tested elaborative interrogation in learning from written facts. The participants studied 32 statements using elaborative interrogation (experimental group) or by only reading the statements (control group). Half of the statements were consistent with the participants’ prior knowledge, while the remainder were inconsistent in this regard. After studying, recognition (immediate and delayed) and recall (free and cued) was tested. On all tests, the experimental group outperformed the control group.
Dunlosky et al. (2013) found extensive empirical evidence to show that these five learning strategies can be used effectively, and have a high-to-moderate utility. However, despite this, students in both higher and secondary education continue to use ineffective strategies during self-study (e.g., Bartoszewski and Gurung, 2015; Morehead et al., 2015; Blasiman et al., 2017). For instance, Dirkx et al. (2019) found that most of their student participants in secondary education reported using re-reading and highlighting as their (often primary) learning strategies. Moreover, they found retrieval practice to be used by 60.1% of the respondents, although only 8.1% reported this as their primary learning strategy. Distributed practice was used by 3.8% of the respondents, self-explaining by 2.9%, interleaved practice by 0.3%, and elaborative interrogation by 1.9%.
Student characteristics, like effortful control: the efficiency of executive attention or reading comprehension, can affects students’ learning strategy choice, students with high levels of reading comprehension, for example, are more likely to relate the content of the text to existing knowledge (Trassi et al., 2019). For example, Medina and Nagamine (2019) found a causal correlation between reading comprehension and learning strategy use, more specific, metacognitive learning strategies. On the other hand, reading comprehension is also affected by learning strategy instruction. In the study by Nejabati (2015), students that received learning strategy instruction prior to a reading comprehension training, outperformed the control group on a reading comprehension test.
Since students tend to use rather ineffective learning strategies, it is vital for teachers to consider students’ learning strategy choices during instruction. Teachers play an important role in students’ learning strategy choices when studying on their own (e.g., Van den Hurk et al., 2016; Coffman et al., 2019). The attitude that teachers have towards learning strategies is a predictor of the students’ choice of strategies (Mevarech and Kramarski, 2003; Ornstein et al., 2010; Grammer et al., 2013; Hudson et al., 2018; Ornstein and Coffman, 2020; Kikas et al., 2021). When students are trained to use different strategies, the attitudes and opinions that teachers hold can affect the use of these newly-taught strategies (De Boer et al., 2014; Kistner et al., 2015). In a longitudinal study (15 teachers, 104 students), Coffman et al. (2019) showed that the extent to which educators’ first-grade teaching containing instructions on strategy use or metacognitive questioning affected students’ study skills and strategy choices. They found this instruction to impact students’ strategy choices both within and beyond the first grade. Several studies have emphasized the importance of training pre-service teachers in learning strategies as it can positively affect their strategy choices. Moreover, once they become teachers, they will pass on these learning strategies to their students (Arcoverde et al., 2020; Cazan, 2020).
To increase the impact of teachers’ instructions on learning strategies, effective learning strategies need to be applied to learning objectives in school subjects. Since learning objectives and pedagogical approaches vary depending on the subject in question (for instance, learning a language requires a different approach than studying mathematics), it is possible that the effectiveness of the learning strategies may also differ per school subject.
Indeed, it can be expected that secondary school subjects not linger in one type of knowledge, moreover, all subjects offer a variety in types of learning objectives, action levels in learning objectives and, related to these learning objectives, types of knowledge. For example, in geography education, Lane et al. (2019) explained that both factual, conceptual and procedural knowledge from Bloom’s knowledge dimension (Anderson et al., 2001) are present in geography education. Despite the presence of the three knowledge types, conceptual knowledge is considered to be dominant in geography learning (Lane et al., 2019). On the other hand, mathematic teachers find it challenging to encourage students into a more conceptual understanding of their subject. Many teachers focus on problem-solving strategies or calculation procedures (Kazemi and Stipek, 2009). Students can apply mathematical procedures, rather than mathematical reasoning (Richland et al., 2012). Similar, chemistry education often focusses on declarative knowledge (Freire et al., 2019). Furthermore, as another example, in history education the learning of important historical facts is an often chosen pedagogical approach (Havekes et al., 2012). Language learning contains a variety of learning objectives, such as vocabulary, grammar, writing etc. For example, vocabulary learning is the acquisition of factual knowledge (Hew et al., 2014) and grammar is often taught in terms of lower-order thinking: memorize grammar rules and apply those rules on isolated sentences (Van Rijt et al., 2019). Final terms for the exams in secondary education in The Netherlands reflect differences in learning objectives and pedagogy (College voor Toetsen en Examens, 2024). Considering mathematics, most final terms focus on tasks where students need to follow procedures. With respect to geography, final terms are on a more conceptual level, with a focus on analyzing and explaining phenomena, and relations between these phenomena.
Because learning objectives vary over school subjects, it would be reasonable to assume that knowledge of effective learning strategies would be more effective if tailored to subject-specific learning tasks. Thus, teachers need to obtain specific knowledge about which learning strategy to use based on students’ tasks or learning objective. The current literature lacks an overview of the subject-specific application of effective learning strategies. Furthermore, previous review studies on effective learning strategies have tended to use different ways to categorize learning content—primarily due to the available literature on particular learning strategies. For example, some categorizations are based on how learning content is presented to the student (stimuli, e.g., text), whereas other categorizations are more grounded on the result of learning (e.g., retention). The following examples emphasize the inconsistencies with which learning content has been analyzed by several review studies. While Dunlosky et al. (2013) discussed the effectiveness of learning strategies for specific learning content, e.g., problem solving tasks, paired associates or foreign-language translations, they did not present a structured taxonomy of these materials. This is similar in Sperling et al. (2016), where, among other strategies, self-explanation and elaborative interrogation were reviewed. Firth et al. (2021) also compared “different types of tasks” in their analysis on interleaved practice. They listed stimuli, such as “bird images” or “statistics examples,” but mentioned that interleaved practice cannot be generalized to all learning tasks. Roediger and Karpicke (2006) mentioned learning content in their discussion of several examples of experimental studies of retrieval practice. They distinguished between paired-associates, prose materials, and classroom materials (e.g., lectures, teachers instruction). In their review on retrieval practice, Adesope et al. (2017) foregrounded the differences in outcome constructs: learning resulting in retention (mere recall of to-be-learned material), transfer (applying learning content in new situations), or a combination of both. However, neither categorization sufficiently takes the types of knowledge into consideration. Rowland (2014) discussed several explanatory theories about retrieval practice, notably making a distinction between “single words,” “paired-associates,” and “prose.” These were used as a moderator in the analysis, complemented by “other”. Pashler et al. (2007) discussed the effects of distributed practice in the categories of math problem solving and perceptual categorization learning. Latimier et al. (2021) emphasized the importance of types of learning tasks by using “type of material” as a moderator. However, given the small amount of comparisons to be drawn if using more categories, they only used “pairs” or “other.” Brunmair and Richter (2019) used “learning material” as a moderator in their review of interleaved practice. They distinguished between paintings, naturalistic photographs, artificial pictures, mathematical tasks, expository texts, words, and tastes as types of “learning materials.” Bisra et al. (2018) used “type of learning” as a moderator in their analysis, distinguishing between problem solving, text studying, study worked example, study case, study simulation, or other. They also used types of knowledge as a moderator, differentiating between factual and conceptual knowledge. The basic problem with the categorization of learning content or learning objectives is that the categories to be used are based on what is available in the literature on a particular learning strategy, with little-to-no regard on the objective of a learning task (i.e., if students study a text, it is unclear what the learning objective is, such as rote learning, understanding key content, or analyzing the text structure). Therefore, no complete set of learning content or learning objective types covering all school subjects has hitherto been provided.
Since previous review studies have examined learning objectives somewhat inconsistently, it is difficult to compare the results of review studies based only on learning objectives. Therefore, a classification of learning objectives is a necessary step to providing more specific instructions on learning strategy choices. The knowledge dimension from the revisited Bloom’s taxonomy (Anderson et al., 2001) can provide this classification.
A taxonomy of learning objectives
A basic taxonomy would allow for a consistent analysis of the available literature, thereby resulting in an overview of the effectiveness of learning objectives in similar learning situations. The knowledge dimension in Bloom’s Taxonomy, revised by Anderson et al. (2001), clarifies what students should learn from learning tasks. For example, textual material can be used to memorize, learn key content, analyze text structure, or function as an example in text writing. The knowledge dimension from Bloom’s Taxonomy, revised by Anderson et al. (2001), describes the following types of knowledge.
Factual knowledge
Factual knowledge consists of “the basic elements students must know to be acquainted with a discipline or solve problems in it” (Anderson et al., 2001). In this type of knowledge, facts stand alone. Connections to other knowledge do exist, but the aim of learning is the recall of the fact—not connections to other facts. Both knowledge of terminology and knowledge of specific details and elements are subcategories in the taxonomy. Examples of factual knowledge are the meaning of symbols, definition of key-concepts, and vocabulary learning.
Conceptual knowledge
Conceptual knowledge is defined as “interrelationships among the basic elements within a larger structure that enable them to function together” by Anderson et al. (2001). Connections to other knowledge become important, thus increasing the significance of understanding the principles and relations of knowledge. Subcategories of conceptual knowledge in the taxonomy are knowledge of classifications and categories, knowledge of principles and generalizations, and knowledge of theories, models and structures. This type of knowledge contains, for example, categories (e.g., of different vertebrates), theoretical models (e.g., political systems), or basic principles (e.g., the law of communicating vessels).
Procedural knowledge
Procedural knowledge is the ability to follow a certain method to obtain desired results (i.e., knowing how to do something). Far from being simply abstract, procedural knowledge is concrete through the learner’s practical application. It is not limited to the insight of the relationships of cohesive facts (i.e., conceptual knowledge), but requires the ability to choose a strategy and follow the steps necessary for achieving the required result. The taxonomy subcategorizes procedural knowledge into knowledge of subject-specific skills and algorithms, knowledge of subject-specific techniques and methods, and knowledge of criteria for determining when to use the appropriate procedure. This type of knowledge includes all kinds of problem-solving procedures and skills, such as how to perform CPR, how to determine a company’s economic stability, or the ability to choose and perform the correct mathematical procedure.
Metacognitive knowledge
Metacognitive knowledge consists of knowledge about cognition, both personal and general. It refers to knowing one’s cognitive strengths and weaknesses, the ability to choose appropriate strategies, and determining how best to study. This type of knowledge does not focus on what is to be learned. Accordingly, it is less suitable for classifying learning objectives and will thus not be used to do so. Moreover, the current study contributes to metacognitive knowledge, both for teachers and students.
Current study
This study aims to provide recommendations for teachers’ instructions on how to study, with regard to learning objectives in their school subject. While Dunlosky et al. (2013) considered five learning strategies to have moderate or high utility, students have a tendency to prefer ineffective strategies (e.g., Dirkx et al., 2019). Teachers can help students use effective learning strategies through their instructions. We believe these instructions can be enhanced by knowledge about subject-specific effectiveness of learning strategies. Deriving subject-specific guidelines for the use of learning strategies from review studies is challenging due to the inconsistent manner in which the learning content used to assess learning strategies has been described. The knowledge dimension from Bloom’s Taxonomy (Anderson et al., 2001) offers the possibility to categorize learning content according to the objective of learning. Using this categorization, it can be investigated what the evidence is for the effectiveness of particular learning strategies for different types of knowledge. Finally, investigating how types of knowledge appear in school subjects is a necessary step to be able to pair effective learning strategies with learning objectives in respective specific school subjects. Thus, our main research question was: What evidence-based recommendations for the use of effective learning strategies can be derived for learning objectives of specific school subjects? This question can be subdivided into the following sub-questions:
1. How effective are respective learning strategies for knowledge types in the knowledge dimension of Bloom’s Taxonomy?
2. How do different types of knowledge appear in learning objectives from different school subjects?
The synthesis of these two research questions forms the answer to the main question. From the perspective of subjects and the extent to which learning types are present, effectiveness of learning strategies regarding to subjects’ learning objectives can be stated.
Method
To answer the research questions, we employed a mixed methods design. An umbrella review was conducted to determine the effectiveness of respective effective learning strategies in either factual, conceptual, or procedural knowledge. Additionally, to gain additional insights into how Bloom’s knowledge dimensions (Anderson et al., 2001) are represented in learning objectives from different school subjects, we conducted three focus group interviews with secondary school teachers. Both the literature review and the focus group interviews together yielded recommendations about the use of particular learning strategies in relation to different learning objectives within school subjects.
Umbrella review
Selection
Since existing review studies about effective learning strategies have already provided a solid basis, the method of an umbrella review was chosen. Both meta-analyses and narrative review studies were considered. Regarding inclusion criteria, review studies needed to be (a) published in peer-reviewed journals, and (b) in the English language. Review studies with (c) an explicit focus outside regular secondary education were excluded (e.g., Schwieren et al., 2017 which focused on the psychology classroom). Studies that focused on special education or on students with disorders were excluded (e.g., Leonard and Deevy, 2020). Moreover, the studies needed to (d) focus on one or more of the following learning strategies: distributed practice, retrieval practice, interleaved practice, self-explanation, elaborative interrogation; and (e) contain information that can be related to the types of knowledge defined by Anderson et al. (2001). Accordingly, we searched the EBSCO Host and Web of Science databases in June 2022, using the following search terms: [one of the effective learning strategies or common synonyms] present in the title, AND “review of literature” OR “literature review” OR “meta-analysis” OR “systematic review” present in the abstract (see Appendix A for the search terms for the respective learning strategies). We also used a snowballing approach to complete the list of review studies.
The selection was conducted in several steps. In each step, any study that clearly failed to meet one of the inclusion criteria was excluded. When in doubt about a study, the reference was retained until the next step. Peer-debriefing sessions with all of the authors were held to discuss the appropriateness of the use of the inclusion criteria to confirm or reject the inclusion of studies. After conducting these steps, the final selection consisted of 22 primary studies (see Figure 1).
Procedure and analysis
From all of the review studies, all relevant information pertaining to the effectiveness of learning strategies for specific knowledge types was listed. For narrative reviews, full texts were analyzed to collect information. In the meta-analyses, only the relevant overall effects were listed. The analysis of the narrative review studies and meta-analyses resulted in sub-divisions for each type of knowledge (e.g., for factual knowledge learning: paired-associates, singe-items, and individual facts). These subdivisions were made based upon the terms and descriptions used in the review studies to describe the learning content that served as examples, or on the terms and descriptions used to describe moderators in meta-analyses. The corresponding author conducted all of the analyses, randomly verified by the other authors.
Focus group interviews
To gain further insights into how different learning objectives and types of knowledge, according to Bloom’s taxonomy (Anderson et al., 2001), appear in different school subjects, we held focus group interviews. We chose these interviews as they allow for interactions between participants, which can serve to strengthen the results of the data collection (Stalmeijer et al., 2014).
Participants
Three homogeneous focus group interviews were held, with each group comprised of teachers from the same Dutch secondary school. Homogeneity was based on the subject the teachers taught. The participants were teachers in either mathematics (four participating teachers), geography (three participating teachers) or native (Dutch) language (three participating teachers).We selected a subject in each of the domains (alpha, beta and gamma). Furthermore, we selected subjects where differences in dominant types of knowledge could be expected, as hypothesized in the introduction. Due to the explorative character of this study, we limited the subjects to three. We selected the participants through convenience sampling (Ritchie et al., 2014).
Procedure
An interview guide was newly developed based on Stalmeijer et al. (2014), in three iterations: The corresponding author wrote the initial interview guide, the co-authors provided feedback, the corresponding author processed the feedback (e.g., rephrasing questions) into a final version, which was then approved by the co-authors. A pilot was conducted to assess the quality of the questions: Two teachers were asked to spontaneously react to the questions so as to assess their comprehensibility, which led to rephrasing two questions to improve the distinction between knowledge types that these questions aimed for. The interviews were held online using Microsoft Teams. The interview guide is provided in Appendix B and contains three groups of questions: (1) an opening question that had to be answered by all participants in turn; (2) after an introduction of the types of knowledge according to Bloom’s Taxonomy, key questions were asked on the types of knowledge in particular subjects, students’ test preparation and homework, examples of types of knowledge in a subject, the relation between the types of knowledge, and the connection with other subjects in terms of knowledge type. The interview ended with (3) an open question that invited participants to share more information concerning knowledge types that had not previously been discussed in the interview. During the entire interview, a brief overview of the knowledge dimension (provided in Appendix B) was visible to all participants. The focus group interviews were recorded and moderated by the first author. The records were transcribed using Amberscript software (Amberscript, 2022), of which transcripts were double-checked and corrected on a verbatim level before the analysis was conducted.
Analysis
The focus group interviews were analyzed using three steps for inductive coding. In the first step, open coding was used to gather all explanations and examples of how a certain type of knowledge appeared in a school subject. The second step involved using axial coding to combine codes from the first step into larger themes. These themes were based on how a type of knowledge appeared in a school subject as long as the conditions for a knowledge type and the relationship between types of knowledge in a school subject had been mentioned. The third step involved selective coding where, for each of the discussed knowledge types, the core of the participants’ answers was recapitulated. The coding of the focus group interviews is included in Appendix C.
Results
Below, the content-related results of the umbrella review regarding the effectiveness of the learning strategies for different types of knowledge is presented. After that, the interview results for the appearance of types of knowledge in particular school subjects’ learning objectives are given.
Effects of learning strategies for types of knowledge
The systematic search resulted in 119 studies related to distributed practice. After deleting the duplicates and inspecting the titles, abstracts, and full texts, seven studies were included that met the inclusion criteria. One more study was added after snowball sampling, resulting in a total of eight studies related to distributed practice. The same procedure was used for the other learning strategies. For retrieval practice, the search in both databases resulted in 128 studies, 6 of which met the inclusion criteria. Using the snowballing approach, a further two studies were found, resulting in a total of eight included studies. For the strategy of self-explanation, 12 studies were found, of which 2 met the inclusion criteria. The strategy of interleaved practice was represented by 88 studies. Three of these studies met the inclusion criteria. For elaborative interrogation, three results were found, but none of these studies met the inclusion criteria. Using the snowballing approach, one study that met the inclusion criteria was found. Table 1 provides an overview of the included review studies and Table 2 shows an overview of the number of included review studies per learning strategy.
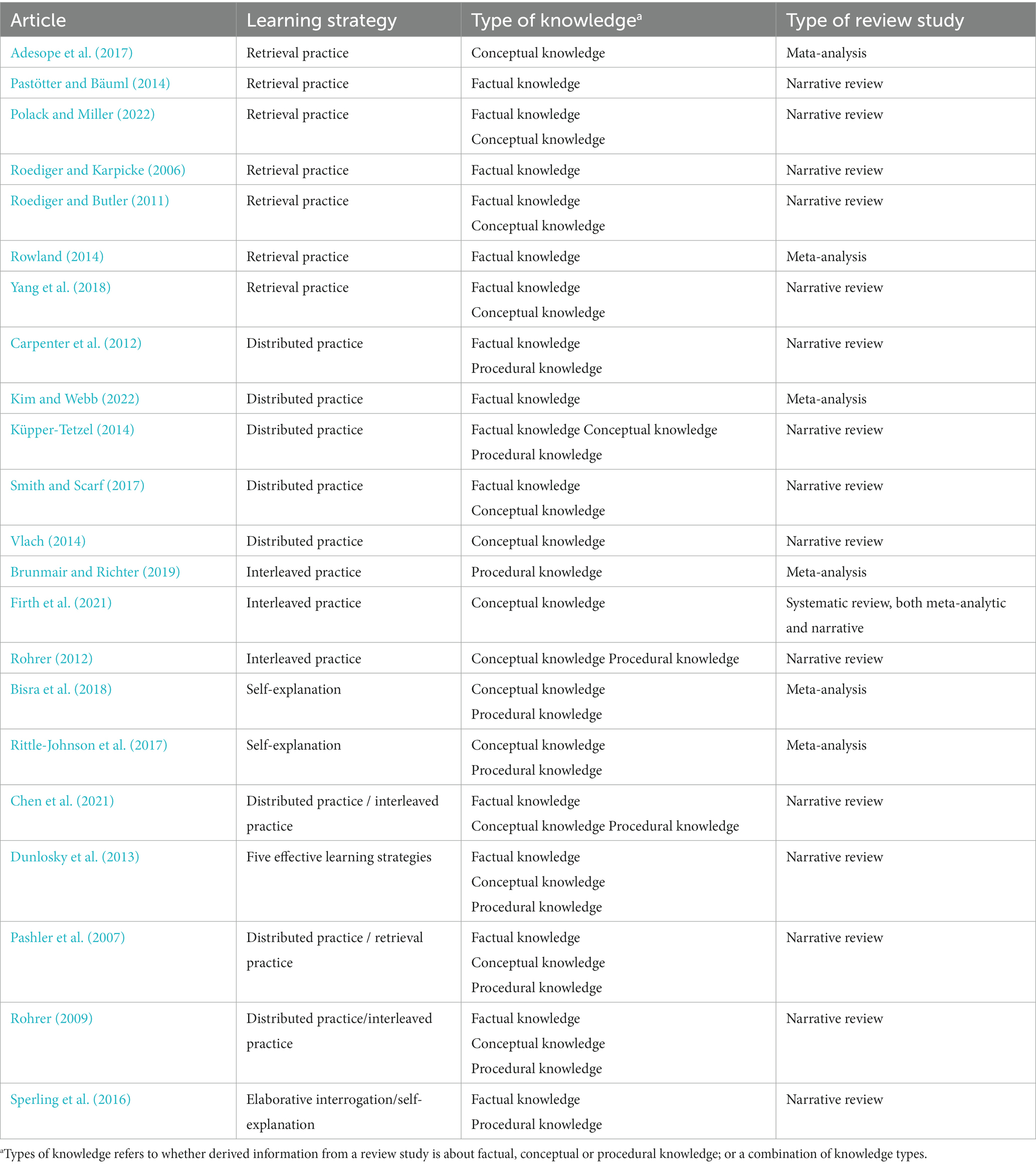
Table 1. Overview of review studies, included learning strategy, type of knowledge, and type of review study (N = 22).
Factual knowledge
Regarding factual knowledge learning, we distinguished four sub-categories: (a) paired-associate learning, i.e., after presenting a cue term, an associated response can be produced by the learner (Campbell et al., 2014); (b) vocabulary learning or foreign vocabulary learning, e.g., the learning of definitions, synonyms or translations of foreign words. Although (foreign) vocabulary learning is part of paired-associate learning, we consider this a separate sub-category of factual knowledge learning due to its relevance in secondary education. (c) Single-item learning, i.e., rote learning of a list of items (e.g., a shopping list); and (d) learning of individual facts, i.e., the learning of stand-alone facts (e.g., definitions).
Retrieval practice
Eight review studies reported the positive effects of retrieval practice on factual knowledge learning. In terms of paired-associate learning, five review studies cited the benefits of retrieval practice, using such examples as face-name pairs, word pairs, or synonyms (Roediger and Karpicke, 2006; Pashler et al., 2007; Roediger and Butler, 2011; Dunlosky et al., 2013; Rowland, 2014). In his meta-analysis, Rowland (2014) found an overall effect for retrieval practice on the learning of “paired-associates”: g = 0.59, with a 95% confidence interval ranging from −0.49 to 0.70. The positive effects of retrieval practice on foreign vocabulary learning, such as in English–Swahili, were reported by five review studies (Roediger and Karpicke, 2006; Pashler et al., 2007; Roediger and Butler, 2011; Dunlosky et al., 2013; Polack and Miller, 2022). Four review studies discussed the positive effects for forms of single-item learning, such as learning word lists or nonsense syllables (Roediger and Karpicke, 2006; Dunlosky et al., 2013; Rowland, 2014; Polack and Miller, 2022). Rowland (2014) analyzed the effect of retrieval practice on “single words” learning in his meta-analysis, resulting in an overall positive effect of g = 0.39, with a 95% confidence interval ranging from −0.24 to 0.53. Four studies reported the effectiveness of retrieval practice for individual fact learning, such as facts from texts, features and locations on maps, general knowledge facts, multiplication facts, obscure facts, spelling and trivia facts, and definitions (Roediger and Karpicke, 2006; Pashler et al., 2007; Dunlosky et al., 2013; Polack and Miller, 2022). In the specific context of the forward testing effect (i.e., that the retrieval of previously-studied content facilitates the learning of new material), two review studies reviewed this phenomenon within factual knowledge learning (Pastötter and Bäuml, 2014; Yang et al., 2018). A forward testing effect was found for factual learning content in paired-associate learning (e.g., face-name pairs), single-item learning (e.g., word lists), foreign vocabulary, and individual facts (history facts).
Distributed practice
Eight review studies discussed the positive effects of distributed practice for factual knowledge learning. Four review studies did so for paired-associate learning, using various examples (e.g., face-name pairs, question-answer pairs, and word pairs) (Dunlosky et al., 2013; Küpper-Tetzel, 2014; Smith and Scarf, 2017; Kim and Webb, 2022). Furthermore, Kim and Webb (2022) found an overall positive effect of distributed practice in paired-associate learning in their meta-analysis of distributed practice in second language learning: g = 0.67–1.36 on immediate and delayed post-tests, with a 95% confidence interval ranging from −0.29 to 1.92. Additionally, Kim and Webb (2022) also found an overall positive effect for distributed practice in second language vocabulary learning: g = 0.76–1.15 on immediate and delayed post-tests, with a 95% confidence interval ranging from 0.26 to 1.49. Vocabulary learning was also discussed by five narrative review studies (Pashler et al., 2007; Carpenter et al., 2012; Dunlosky et al., 2013; Küpper-Tetzel, 2014; Smith and Scarf, 2017), all of which reported the positive effects of distributed practice on vocabulary learning. Beneficial impacts were also reported for the learning of native vocabulary. Four review studies found distributed practice’s positive effects on single-item learning, using word lists or spelling lists (Carpenter et al., 2012; Küpper-Tetzel, 2014; Smith and Scarf, 2017; Chen et al., 2021). Six review studies found distributed practice to be an effective learning strategy for individual facts, using such examples as trivia facts, definitions, and historical facts (Rohrer, 2009; Carpenter et al., 2012; Dunlosky et al., 2013; Küpper-Tetzel, 2014; Smith and Scarf, 2017; Chen et al., 2021).
Interleaved practice
The effect of interleaved practice in factual knowledge learning was discussed in two review studies (Dunlosky et al., 2013; Chen et al., 2021). Dunlosky et al. (2013) concluded that interleaved practice does not facilitate vocabulary learning. Chen et al. (2021) cited examples of studies in which interleaved practice was used to learn factual knowledge (paired-associates: word pairs). They concluded that no interleaving effect was found because the participants had no need of discriminating between items while learning. Thus, interleaved practice does not facilitate learning when contrasting and discriminating between items is not part of the learning task.
Elaborative interrogation
Sperling et al. (2016) identified positive effects of elaborative interrogation on the learning of individual facts (facts about animals, the solar system, and plants).
Conceptual knowledge
We identified three subcategories of conceptual knowledge learning in the review studies: (a) Category learning, which involves learning to discriminate within or between categories; (b) the learning of complex material, when relations between factual knowledge are part of the learning task; and (c) the transfer of learning (or the generalization of knowledge), where learners apply learning content to new situations.
Retrieval practice
Five review studies reported the effects of retrieval practice on conceptual knowledge learning. Three discussed the learning of complex material (Roediger and Butler, 2011; Dunlosky et al., 2013; Polack and Miller, 2022). These three review studies all identified the benefits of retrieval practice for text comprehension. However, across the review studies, only two examples of empirical studies were mentioned (i.e., Butler, 2010; Karpicke and Blunt, 2011). Four review studies reported on the benefits of retrieval practice for the transfer of learning (Roediger and Butler, 2011; Dunlosky et al., 2013; Polack and Miller, 2022). In their meta-analysis, Adesope et al. (2017) found an overall positive effect for retrieval practice on transfer of learning: g = 0.53, with a 95% confidence interval ranging from 0.38 to 0.68. However, Polack and Miller (2022) stated that, if the transfer of learning content is the learning objective, retrieval practice activities in the learning phase must be aligned to this goal. For example, while learning about lightning, participants used either a practice test focused on the recall of facts or a test involving analytical questions. The testing effect was only found when the practice test corresponded with the final test (Johnson and Mayer, 2009). In the context of the forward testing effect, Yang et al. (2018) found retrieval practice to have positive effects on both category learning (e.g., paintings were categorized according to the artists’ style), the learning of complex material from texts, and the generalization of knowledge.
Distributed practice
Five review studies discussed the effects of distributed practice on conceptual knowledge learning. Category learning was only reported by Pashler et al. (2007), and was limited to perceptual categorization learning (i.e., categorizing checkboard patterns), which was not facilitated by distributed practice. In contrast, the learning of complex material was found to benefit from distributed practice, as reported by three review studies (Dunlosky et al., 2013; Küpper-Tetzel, 2014; Vlach, 2014). The examples presented in these studies included educational learning content, namely the conceptual learning of scientific concepts, as well as the conceptual learning of science, mathematics, and statistics. Concerning the transfer of learning, distributed practice was found to be an effective learning strategy by three review studies (Küpper-Tetzel, 2014; Vlach, 2014; Smith and Scarf, 2017). In most of the examples used in the review studies, key concepts formed the transferred learning content. Furthermore, Vlach (2014) presented an example whereby distributed practice facilitated learning on the “novel noun generalization task”: a learning task where young children transferred new words to novel objects.
Interleaved practice
Five review studies highlighted the effect of interleaved practice on conceptual knowledge learning (Rohrer, 2009, 2012; Dunlosky et al., 2013; Chen et al., 2021; Firth et al., 2021), with a specific focus on category learning. The empirical studies that were presented to discuss the effect of interleaved practice on category learning included such materials as artists’ painting style, species of trees, insects or birds, psychopathological disorders, lists of pictures, and chest radiographic patterns. In their meta-analysis, Firth et al. (2021) analyzed effect of interleaving examples in the learning of concepts. They reported an overall positive effect of g = 0.56, with a 95% confidence interval ranging from 0.41 to 0.71. No effect of interleaved practice was found on the pronunciation of French words (Chen et al., 2021). For this task, Chen et al. (2021) reasoned that interleaved practice was not an effective learning strategy because learners did not need to discriminate between rules while learning. From their analysis, Chen et al. (2021) concluded interleaved practice to be more effective for discriminating between and within highly similar categories.
Self-explanation
The effects of self-explanation on conceptual knowledge learning were reported in three review studies. The learning of complex material was discussed by Dunlosky et al. (2013). They found self-explanation to benefit learning theorems from texts or principles. Bisra et al. (2018) used conceptual knowledge as a moderator in their meta-analysis. They found an overall effect of g = 0.60, with a 95% confidence interval ranging from 0.45 to 0.75. In their meta-analysis, Rittle-Johnson et al. (2017) analyzed self-explanation in mathematics learning, and found an effect size on conceptual knowledge of 0.33, with a 95% confidence interval ranging from 0.09 to 0.57 when immediately tested after intervention. However, when tested with a delay, the effect size on conceptual knowledge was −0.05, with a 95% confidence interval ranging from −0.29 to 0.19.
Elaborative interrogation
The effect of elaborative interrogation on conceptual knowledge learning was only reported in the review by Dunlosky et al. (2013). Elaborative interrogation was found to be effective for the learning of complex material in a learning task where relations were made between facts.
Procedural knowledge
In the review studies, procedural knowledge learning was conceptualized as learning how to solve problems. The majority of problem-solving tasks were mathematical in nature, although grammar, physics, and logical reasoning tasks were also included to a lesser extent. In most reported studies on procedural knowledge learning, selecting and executing the appropriate procedure was part of the problem-solving task.
Distributed practice
Five review studies found distributed practice to have positive effects on procedural knowledge learning. Positive effects were reported for mathematical tasks (e.g., adding fractions, permutation problems) and applying grammar rules (Pashler et al., 2007; Rohrer, 2009; Carpenter et al., 2012; Küpper-Tetzel, 2014; Chen et al., 2021).
Interleaved practice
Five review studies reported on the effect of interleaved practice on procedural knowledge learning. While the majority of this research focused on mathematical tasks, verb conjugation and physics problems were also discussed (Rohrer, 2009, 2012; Dunlosky et al., 2013; Brunmair and Richter, 2019; Chen et al., 2021). Brunmair and Richter (2019) found an overall effect of interleaved practice on mathematical tasks: g = 0.34, with a 95% confidence interval ranging from 0.11 to 0.57. Three review studies indicated that the similarity between and within categories was an important factor in determining the effectiveness of interleaved practice. Rohrer (2012) stated that interleaved practice is effective in procedural knowledge learning in mathematics as it encourages students to learn to discriminate between different procedures. This enables students to choose the correct procedure to solve a mathematical problem. In an example mentioned by Chen et al. (2021), participants did not need to discriminate between similar items (rules) in a category because mathematical tasks from different domains were alternated. Equivalent to factual and conceptual knowledge learning, contrasting or discriminating between or within similar categories as part of the learning task is conditional for interleaved practice to be effective.
Self-explanation
Four review studies identified self-explanation as an effective strategy for procedural knowledge learning, of which two were meta-analyses. Bisra et al. (2018) found self-explanation to have an overall positive effect on the learning of procedural knowledge: g = 0.47, with a 95% confidence interval ranging from 0.14 to 0.80. The meta-analysis by Rittle-Johnson et al. (2017) examined the effects of self-explanation on procedural knowledge learning in mathematics and found an overall positive effect of self-explanation using both immediate and delayed testing formats. Immediately after the intervention, the effect size was 0.28, with a 95% confidence interval ranging from 0.07 to 0.49. Tested with a delay, the effect size was 0.13, with a 95% confidence interval ranging from −0.13 to 0.39. The positive effects of self-explanation on logical reasoning and mathematics tasks were also discussed in narrative reviews (Dunlosky et al., 2013; Sperling et al., 2016).
Elaborative interrogation
Only Dunlosky et al. (2013) discussed the effect of elaborative interrogation on procedural knowledge learning. They presented one example in which participants used elaborative interrogation while studying texts about retail, merchandising, and accounting. A final problem-solving test revealed no effect on procedural knowledge learning.
Types of knowledge in school subjects
To answer the second research question how different types of knowledge appear in different school-subjects, focus group interviews were held. In the analysis, open coding resulted in 167 explanations or examples of how knowledge types appear in school subjects’ learning objectives. Axial coding resulted in 52 larger themes. Selective coding yielded 12 quotes or recapitulations representing the core of the participants’ answers. Below, we report the findings that were specific for each of the focus group interviews with teachers in mathematics, native (Dutch) language, and geography. The coding scheme of the focus group interviews can be seen in Appendix C.
Mathematics
The participants indicated that factual knowledge forms a necessary base from which to enable the learning of more complex aspects of mathematics learning. They mentioned several examples of factual knowledge learning: Ordering mathematical operations (e.g., the BODMAS acronym: first calculations between brackets, then powers or square roots etc.), definitions (e.g., types of shapes and angles, perpendicular line, π ≈ 3.14, types of parabolas), and standard calculation rules or methods (e.g., recalling the Pythagorean theorem or formulas for calculating volumes).
The participants referred to conceptual knowledge infrequently. Indeed, they cited it as limited to higher educational levels and to high-achieving mathematics students. One participant mentioned that “Conceptual knowledge is exceptional; it only appears at the highest levels.” The teachers argued that, at the highest levels, students need to be able to explain why a particular answer is true. One example of conceptual knowledge learning in mathematics is scaling sizes (i.e., calculating real distances from scales on a map). Although many students memorize the scales and the formulas necessary for converting them (merely on a factual level), the objective is to understand scaling more conceptually. The second example mentioned was students’ ability to apply skills of diagrams and flowcharts in novel situations.
Procedural knowledge was indicated as the dominant knowledge type in mathematics learning. To the agreement of the other teachers, one participant stated that “We can conclude that procedural knowledge is most important for us.” In the mathematics curriculum, students develop a “basket filled with tools” from which students can choose to solve a variety of mathematical problems. In other words, students learn to choose and execute the correct procedure when solving mathematics problems. The participants provided many examples of procedural learning throughout the mathematics curriculum (e.g., solving equations, algebraic, or goniometric problems, such as calculating missing angles of triangles), and distinguished between theoretical procedures (e.g., solving equations without context) and real-life problem-solving tasks (e.g., how much paint would you need to coat every side of a barn [visualized in a picture]).
Student characteristics were consistently mentioned when discussing the three types of knowledge in mathematics learning. In the experience of the participants, the appearance of the types of knowledge in mathematics learning depends on the students’ own achievements in the subject. One participant explained that low-achieving students need to rely mainly on factual knowledge and use a close step-by-step approach toward problem solving. The participants described factual knowledge learning as less important for high-achieving students.
Native (Dutch) language
As with mathematics, factual knowledge was described as necessary to forming a basis for native language learning. According to one participant: “It starts with factual knowledge: you need a starting point.” Many examples of factual knowledge learning were mentioned (e.g., facts about literary history, rote learning of grammar and spelling rules, vocabulary, types of texts and purposes of the author, word classes). However, all of the participants agreed that factual knowledge learning is not a learning objective in itself, but rather a prerequisite for the learning of conceptual and procedural knowledge.
Conceptual knowledge was indicated to appear in native language learning as part of larger sets of grammar and spelling rules. During the application of these rules, the participants emphasized the necessity of using several grammar rules to form correct sentences. One participant stated that “You need conceptual grammar knowledge to conjugate a verb correctly. In order to conjugate correctly, you need to know how to find the noun phrase.” Moreover, a knowledge of text structures was described as a form of conceptual knowledge in native language learning. This knowledge is needed for reading comprehension, writing skills, and verbal expression.
The participants indicated that procedural knowledge appears in grammar and spelling tasks. In these tasks, students follow a step-by-step approach to obtain the correct result. Procedural knowledge was also said to be used to perform more complex tasks (e.g., writing a plea or conducting a lecture). These assignments are prepared and conducted using a procedure.
The participants further claimed that objectives in native language learning include procedural or conceptual knowledge, but not factual knowledge—despite its being a necessary basis for both.
Geography
Regarding geographical knowledge, teachers reported that factual knowledge is a necessary basis from which to engage in more complex forms of geography learning. One participant described factual knowledge “an essential condition.” This factual knowledge was defined as consisting of both definitions of geographical terms (e.g., demographic terms: population density, population growth, migration, surplus population etc.) and common knowledge (e.g., political situations or current events). Despite their being outside of the geographical domain, language skills (particularly vocabulary) were cited as important. All of the participants agreed that factual learning is not a learning objective in itself in geography learning. For example, in learning topography, the learning objective is to “create a mental map from the world, in which the relation between, for example, cities and their relative position to mountains and rivers, becomes clear”.
Conceptual knowledge was described as the dominant knowledge type within geography, due primarily to its clear presence in all domains of geography learning. For example, in the demographic domain, the whole of individual concepts and demographical models (e.g., demographic transition model) result in a theoretical structure about demography. Moreover, students learn to combine information from several domains. For example, students need to apply information from the Köppen climate classification to vegetation or landscape zones. Consequently, conceptual knowledge enables students to analyze regions or continents using geography domains.
Several examples of procedural knowledge learning were mentioned in geography learning (e.g., finding and combining information in atlases, using coordinates, calculating population growth, river stream gradients, reading graphics or tables, and following schemes to determine climates). The participants emphasized that the learned procedures contribute to conceptual knowledge as they result in information which contributes to a conceptual view of a region. However, some participants explained that procedural tasks do not necessarily always lead to a conceptual understanding. Furthermore, practical and research assignments were reported as procedural because teachers offer a step-by-step procedure for conducting practical or research assignments.
Discussion
General findings
Teachers’ strategy instruction affects students’ choice of learning strategy (e.g., Coffman et al., 2019). To be able to give appropriate instructions on which strategy to use for learning tasks in their secondary school subject, teachers must be aware of which strategies are most effective for specific learning tasks. Although much research has been conducted on the effectiveness of particular learning strategies (e.g., Dunlosky et al., 2013), an overview of how learning strategies’ effectiveness is related to the content of secondary school subjects is absent from the literature. The main goal of this study was to specify, based on the existing literature, how the effectiveness of particular learning strategies relates to different types of knowledge. Then, by mapping types of knowledge on to the objectives of different school subjects, we sought to specify which learning strategies would be most suitable for particular subjects so as to enable teachers to instruct appropriate learning strategies. We used the types of knowledge (factual, conceptual, and procedural) from Bloom’s Taxonomy (Anderson et al., 2001) to create an overview of the effectiveness of learning strategies to attain the learning objectives of different school subjects. This overview was created to answer two questions: (1) how effective are respective learning strategies for types of knowledge from the knowledge dimension of Bloom’s Taxonomy? and (2) how do different types of knowledge appear in learning objectives from different school-subjects? We performed an umbrella review to answer the first question and held focus group interviews with secondary school teachers to answer the second.
In the umbrella review, we analyzed 22 review studies to assess the effectiveness of 5 effective learning strategies for factual, conceptual, and procedural knowledge. The effectiveness of the learning strategies varied depending on the type of knowledge. For factual knowledge learning, we found most evidence for the effectiveness of retrieval practice and distributed practice (e.g., Pashler et al., 2007; Roediger and Butler, 2011). While elaborative interrogation was also found to be effective, existing research appears to be limited to learning individual facts (Sperling et al., 2016). In conceptual knowledge learning, self-explanation was identified as effective (e.g., Bisra et al., 2018). Retrieval practice was also found to be effective for conceptual knowledge learning so long as retrieved information during learning was focused on conceptual knowledge (Polack and Miller, 2022). Distributed practice has been reported as effective for the learning of complex material (e.g., Küpper-Tetzel et al., 2014) and the transfer of learning (i.e., applying learned material to novel situations) (e.g., Smith and Scarf, 2017). Chen et al. (2021) identified interleaved practice as fruitful to category learning, while Dunlosky et al. (2013) found elaborative interrogation to be beneficial to the learning of complex material. For procedural knowledge learning, distributed practice and self-explanation were found to be effective strategies (e.g., Rohrer, 2009; Carpenter et al., 2012). Interleaved practice has been deemed efficacious when students are required to learn how to discriminate between items (e.g., Brunmair and Richter, 2019). See Table 2 for an overview of the results from the umbrella review.
In the focus-group interviews, we interviewed secondary school teachers in mathematics, Dutch, and geography about the appearance of the investigated types of knowledge in their subjects. All of the participants indicated that factual knowledge was a necessary basis for further learning in their subjects, which is consistent with previous research (Anderson et al., 2001; Roediger and Pyc, 2012; Küpper-Tetzel, 2014). Math teachers cited learning objectives aiming for procedural knowledge as the dominant type of knowledge in their subject. In contrast to previous research (e.g., Rohrer, 2009; Rittle-Johnson et al., 2017), they indicated that learning of conceptual knowledge is scarce. Dutch language teachers claimed conceptual and procedural knowledge to be of equal importance. For example, the rules within a language system were described as being part of conceptual learning objectives. The language teachers also spoke of procedural knowledge (e.g., the application of language rules are taught in a procedural way). Moreover, for completing large assignments (i.e., writing or speaking assignments), students must follow a set of procedures. Geography teachers cited conceptual knowledge as the dominant type of knowledge in their subject. Conceptual knowledge was indicated to be the learning objective of (almost) all geography education. Procedural knowledge learning was also described as present within geography learning objectives, although not as central as its conceptual counterpart. The outcome of procedural tasks often offered input for conceptual knowledge learning (i.e., calculating river gradients offers inputs for landscape analyses).
As a synthesis to our two research question, we have formulated several suggestions for learning strategy instruction. Specifically, we propose four suggestions, followed by subject-specific examples. These suggestions and examples are based on the results from the focus group interviews and the umbrella review. First, results from the umbrella review indicate that there are differences in the effectiveness of a learning strategy depending on the knowledge type from the learning objective. Therefore, teachers need to pay attention to the alignment of these two. For example, if teachers instruct students to study a text, they should consider the specific type of knowledge that is learned: Are students supposed to learn definitions offered in the text, relate the information contained within to prior studied material, or analyze the text in a certain way? Second, if factual knowledge learning is the objective of a learning objective, we would propose retrieval and distributed practice as learning strategies, followed by elaborative interrogation. Third, if conceptual knowledge is the objective of a learning objective, retrieval practice (with attention to cues that focus on conceptual knowledge), distributed practice, self-explanation, and elaborative interrogation are advised. Fourth, if procedural knowledge is the objective, we would encourage the use of distributed practice, self-explanation, and interleaved practice as the optimal strategies. When using interleaved practice as a learning strategy in procedural knowledge learning, teachers should bear in mind that its effectiveness increases in line with the similarity of the content.
Further to these suggestions, we formulated specific examples for instructing learning strategies for mathematics, native language, and geography. Based on the learning objectives reported by the teachers in the focus group interviews, we formed examples on how the suggestions above can be applied to these school subjects. For mathematics, objectives aiming for factual and procedural knowledge were reported. If, for example, students need to recall formulas to calculate volumes, teachers can instruct the learning of these formulas to be spaced out over a longer period of time and stimulate the retrieval of formulas in both class and self-study. If students must solve mathematical problems requiring the use or calculation of formulas, teachers could recommend solving a number of problems a day rather than instructing blocked practice sessions. Furthermore, teachers can offer problems in an interleaved presentation and instruct students to explain to themselves how they found the correct solutions to the presented problems. For native language learning, objectives aimed at factual, conceptual, and procedural knowledge were reported. Learning strategies for factual knowledge can be applied in, for example, rote learning of different past tense conjugations. Teachers can apply distributed retrieval practice by making students answer questions on how different past tenses should be conjugated in an online learning environment. If students need to learn how these past tenses relate to the meaning of a sentence, teachers can, for example, instruct students to explain to themselves how a change in tense can alter the meaning of a particular sentence. If students make exercises in which they need to apply the correct past tense, teachers can offer exercises in an interleaved way and instruct students on how best to space out these exercises over time. For geography learning, the teachers cited factual, conceptual, and procedural knowledge. When learning demographic definitions, teachers can provide guidance on the use of flashcards and spacing study sessions over time. If students need to apply their knowledge about demography to explain changes in population size and economic development, teachers can offer sets of practice questions which students can answer during studying. Students can, during studying, explain why their answers are true. If students need to learn how to calculate population development, teachers can offer similar demographic calculations in an interleaved order and space exercises about demographic calculations out over a longer period of time.
The findings as presented above, are of high importance for teaching practice. Not only can teachers foster more effective learning by instructing effective strategies, these findings help teachers to instruct appropriate learning strategies in relation to the learning task in their subject. By instructing adequate learning strategy choice educates students in strategy choice, ultimately enabling them to choose appropriate learning strategies on their own. This self-regulated strategy choice is a vital skill throughout their academic career. Moreover, meta-cognitive skills, in this case the choice of appropriate learning strategies, facilitate effective learning over students’ entire life.
Limitations and directions for future research
In this study, we employed the methods of an umbrella review and focus group interviews, both of which have their limitations. Although we included only peer-reviewed review studies, an umbrella review depends on the quality of the analyses of other researchers. Only a limited number of the review studies (e.g., Bisra et al., 2018; Polack and Miller, 2022) used types of knowledge related to Bloom’s Taxonomy (Anderson et al., 2001) to analyze the learning content used in included studies. Bisra et al. (2018) stated that analyses based on knowledge types are often challenging, primarily because experimental studies typically report the learning content used to assess a learning strategy, not the objective. According to Anderson et al. (2001), “How this content is structured by teachers in terms of their objectives and instructional activities results in different types of knowledge.” For example, it is difficult to assess a learning strategy’s effectiveness for studying texts as this activity can refer to rote learning, memorizing the main content, understanding the content related to prior knowledge, or analyzing the text relative to the author’s objective, among others. The objective type of knowledge in experimental studies is occasionally either left undescribed or unclear. Thus, it is important for future experimental studies to offer a clear description of the used learning task and its objected type of knowledge. Similarly, if future review studies use Bloom’s knowledge dimension (Anderson et al., 2001) to describe the effects and limitations of learning strategies, teachers would be able to more effectively apply learning strategies to their subjects. Due to the impossibility of relating conducted analyses in review studies to Bloom’s knowledge dimension (Anderson et al., 2001), 10 review studies were excluded. These studies could have contributed to the analysis in the present study, if the knowledge types were taken into account. The analysis in the present study relies on a consistent use of the types of knowledge from Bloom’s Taxonomy (Anderson et al., 2001). Variations in used definitions of types of knowledge between the authors of the included review studies and ourselves could have impacted the results. For example, Sperling et al. (2016) discussed examples studies in which the effects of elaborative interrogation on factual knowledge learning was investigated. However, the “why” questions used in elaborate interrogation connect factual information to existing knowledge. Thus, the learning objective could be more conceptual in nature: Connections of the facts become part of the learning task. Another critical point regarding the use of an umbrella review is that the included empirical studies could well overlap. This potentially influences the weight of the results. For example, the research by Rohrer and Taylor (2007) was used by several review studies to analyze interleaved practice in procedural knowledge learning (Rohrer, 2009; Carpenter et al., 2012; Rohrer, 2012; Dunlosky et al., 2013; Chen et al., 2021). Moreover, in the umbrella review, only review studies were analyzed. Therefore, we did not conduct an analysis of all available experimental studies. As a result, we might have missed experimental studies relevant to the research question in the present study, because these studies were not included in the review studies included in our umbrella review. For example, none of the included review studies analyzed the effects of retrieval practice on procedural knowledge learning. Therefore, this umbrella review could not suggest this learning strategy for this knowledge type, despite the fact that there is at least some empirical evidence to support its use (e.g., Wong et al., 2019). The exploration in the umbrella review formed the base of suggestions and examples regarding the instruction of learning strategies. However, the evidence from the umbrella review to support these suggestions was found in effects of learning strategies regarding a certain knowledge type, such as distributed practice in conceptual knowledge learning. However, the umbrella review did not yield results that compared one effective learning strategy to another in a certain learning type. For example, no comparisons were made between distributed and retrieval practice in conceptual knowledge learning. Evidence showed that both are effective, but not which one is most effective. In short, we can only present the evidence of one learning strategy being effective in a certain knowledge type, not the evidence of which effective learning strategy is most effective regarding the knowledge type. Therefore, further research is needed to asses our suggestions.
The scope of the focus group interviews was limited to three school subjects and three or four teachers from said subjects in a Dutch secondary education context. However, there are cross-cultural differences in how school subjects are offered to students (e.g., Aikenhead and Jegede, 1999; Miller et al., 2005), especially for native language: For instance, the complexity of the linguistic structure might influence the extent of conceptual knowledge. Moreover, our findings for the three school subjects do not cover all secondary education subjects. The variation in included school subjects in this study and the focus on learning objectives rather than learning content offers teachers the opportunity to relate learning objectives from their subject to those which have been included. However, more research on the relationship between effective learning strategies and school subjects is needed to validate and generalize our findings. Along this line, further research is also needed to the appearance of the knowledge dimension in other secondary school subjects and educational practices, and the effects of effective learning strategies on respective types of knowledge to generalize our findings. Indeed, further research could provide teachers with more detailed guidelines for instructing learning strategies to their students.
Self-explanation and elaborative interrogation are two similar learning strategies. Although often reviewed separately (e.g., Dunlosky et al., 2013; Sperling et al., 2016), the similarities between them often cause both to coincide. Using elaborative interrogation, “why…?” questions are asked about learning content. Interestingly, these questions are also used as a cue in self-explanation. For example, in the meta-analysis by Rittle-Johnson et al. (2017), self-explanation was introduced with two examples of cue-questions: “How does this work? Why is this true?” This is similar to the review by Sperling et al. (2016), which discussed elaborative interrogation and self-explanation separately. In this review study, the study by Renkl et al. (1998) was used as an example of self-explanation [also included in the meta-analysis by Rittle-Johnson et al. (2017)]. In the study by Renkl et al. (1998), students explained steps in a mathematics worked example, explained by Sperling et al. (2016) as answering the question “why different steps or operations were appropriate.” The overlap in these learning strategies suggests that our results defined for self-explanation might, at least partially, apply to elaborative interrogation (and vice versa).
As stated in the introduction, teachers’ instructions have the ability to affect students’ learning behavior (e.g., Coffman et al., 2019). Through our findings, teachers in secondary education can be better informed on which learning strategy to instruct in their subjects. Indeed, subjects do differ in learning types and learning objectives. All teachers confirmed that factual knowledge is a necessary base for learning in their subjects, according to Anderson et al. (2001). Specifically, teachers in mathematics struggled with conceptual learning in their subject, stating that it was almost absent at all. This is in line with Kazemi and Stipek (2009). However, the review studies by Rohrer (2009) and Rittle-Johnson et al. (2017) did report conceptual learning objectives in mathematics learning. Moreover, it has been emphasized that conceptual understanding of mathematics is important for learning in this subject (Rittle-Johnson et al., 2001; Kazemi and Stipek, 2009). Native language teachers associated grammar with conceptual knowledge. This is remarkable, because most pedagogical approaches to grammar are rather factual and procedural: rote learning of grammar rules and apply these on isolated sentences. However, a more conceptual approach to grammar teaching has been emphasized by Van Rijt et al. (2019). The focus on conceptual learning in geography is in line with earlier findings (Lane et al., 2019).
Despite the potential of teachers’ subject-specific instruction on learning strategies, research has shown that changing learning behavior is challenging. Indeed, students tend to stick with their learning habits (Biwer et al., 2020; McDaniel and Einstein, 2020). Teachers’ instruction to students on which learning strategy is applicable for a particular learning task might be insufficient for encouraging them to choose the most effective learning strategies for self-study. To facilitate students’ self-regulated effective learning strategy choice, teachers might need to address the six principles formulated by Biwer and De Bruin (2023), such as “students need to set specific goals” or “students need guided practice and context-embedded support.” Similarly, McDaniel and Einstein (2020) designed the Knowledge, Belief, Commitment and Planning framework. Further research is needed to discover how to extend these training programs to improve teachers’ instructions.
The suggestions and examples for instructing on learning strategies formulated above, focus on complementing teachers’ instructions on both what and how to learn. However, the results from this study—as well as the research upon which it has been based—also contains implications on how school subjects or within-subject themes are programmed. For example, most mathematics textbooks do not provide an interleaved presentation of (similar) problems to solve, but rather present problems in a blocked fashion (Rohrer, 2009). While this problem could be addressed by publishers, teachers also need to interleave textbook assignments by themselves. Similarly, the planning of school subjects’ programs (in classroom activities or embedded in textbooks) should consider distributed practice. Long-term learning can be facilitated by spacing out learning content over time. Despite the knowledge about the impacts of effective learning strategies, changing learning behavior is not simply a matter of paying more attention during classroom instruction to how learning occurs. As described above, changing learning behavior is a more complex issue. Therefore, instructing on learning strategies might also affect class preparations and pedagogical choices. Not only do teachers need to consider effective learning strategies when designing education programs, they must also embed learning strategies in their pedagogy. For example, teachers could encourage retrieval practice through demonstrating its benefits in the classroom. This also implies that pre-service teachers need to familiarize themselves with effective learning strategies—which is currently not evident in teacher education (Surma et al., 2018).
This study is—to the best of our knowledge—the first to explore the relation between learning strategies, knowledge types, and learning objectives in school subjects. It offers suggestions to help teachers select which learning strategies to instruct for the task at hand, with the aim of increasing the effectiveness of their students’ study behavior. Moreover, with appropriate instructions, teachers do have the ability to make students’ learning more effective, thus enabling students to reap greater rewards from their self-study activities.
Author contributions
SL: Conceptualization, Data curation, Formal analysis, Investigation, Methodology, Project administration, Validation, Writing – original draft. DD: Conceptualization, Methodology, Writing – review & editing. DJ: Conceptualization, Supervision, Writing – review & editing. GC: Conceptualization, Methodology, Supervision, Writing – review & editing.
Funding
The author(s) declare that no financial support was received for the research, authorship, and/or publication of this article.
Conflict of interest
The authors declare that the research was conducted in the absence of any commercial or financial relationships that could be construed as a potential conflict of interest.
Publisher’s note
All claims expressed in this article are solely those of the authors and do not necessarily represent those of their affiliated organizations, or those of the publisher, the editors and the reviewers. Any product that may be evaluated in this article, or claim that may be made by its manufacturer, is not guaranteed or endorsed by the publisher.
Supplementary material
The Supplementary material for this article can be found online at: https://www.frontiersin.org/articles/10.3389/feduc.2024.1340120/full#supplementary-material
References
Adesope, O. O., Trevisan, D. A., and Sundararajan, N. (2017). Rethinking the use of tests: a meta-analysis of practice testing. Rev. Educ. Res. 87, 659–701. doi: 10.3102/0034654316689306
Agarwal, P. K., Bain, P. M., and Chamberlain, R. W. (2012). The value of applied research: retrieval practice improves classroom learning and recommendations from a teacher, a principal, and a scientist. Educ. Psychol. Rev. 24, 437–448. doi: 10.1007/s10648-012-9210-2
Aikenhead, G. S., and Jegede, O. J. (1999). Cross-cultural science education: a cognitive explanation of a cultural phenomenon. J. Res. Sci. Teach. 36, 269–287. doi: 10.1002/(sici)1098-2736(199903)36:3<269::aid-tea3>3.0.co;2-t
Amberscript (2022). [Transcription software]. Available at: https://www.amberscript.com/en/.
Anderson, L. W., Krathwohl, D. R., Airasian, P. W., Cruikshank, K. A., Mayer, R. E., Pintrich, P. R., et al. (2001). A taxonomy for learning, teaching, and assessing: a revision of Bloom’s taxonomy of educational objectives, Abridged edition. White Plains, NY: Longman 5.
Arcoverde, Â. R. d. R., Boruchovitch, E., Acee, T. W., and Góes, N. M. (2020). Self-regulated learning of Brazilian students in a teacher education program in Piaui: the impact of a self-regulation intervention. Front. Educ. 5:571150. doi: 10.3389/feduc.2020.571150
Bartoszewski, B. L., and Gurung, R. A. (2015). Comparing the relationship of learning techniques and exam score. Scholarsh. Teach. Learn. Psychol. 1, 219–228. doi: 10.1037/stl0000036
Berthold, K., Eysink, T. H., and Renkl, A. (2009). Assisting self-explanation prompts are more effective than open prompts when learning with multiple representations. Instr. Sci. 37, 345–363. doi: 10.1007/s11251-008-9051-z
Bisra, K., Liu, Q., Nesbit, J. C., Salimi, F., and Winne, P. H. (2018). Inducing self-explanation: A meta-analysis. Educ. Psychol. Rev. 30, 703–725. doi: 10.1007/s10648-018-9434-x
Biwer, F., and De Bruin, A. B. H. (2023). “Teaching students to ‘study smart’ – a training program based on the science of learning” in In their own words: What scholars want you to know about why and how to apply the science of learning in your academic setting. eds. C. E. Overson, C. M. Hakala, L. L. Kordonowy, and V. A. Benass (Society for the Teaching of Psychology), 411–425. Available at: https://teachpsych.org/ebooks/itow
Biwer, F., Oude Egbrink, M. G., Aalten, P., and De Bruin, A. B. (2020). Fostering effective learning strategies in higher education-a mixed-methods study. J. Appl. Res. Mem. Cogn. 9, 186–203. doi: 10.1016/j.jarmac.2020.03.004
Blasiman, R. N., Dunlosky, J., and Rawson, K. A. (2017). The what, how much, and when of study strategies: comparing intended versus actual study behaviour. Memory 25, 784–792. doi: 10.1080/09658211.2016.1221974
Bloom, K. C., and Shuell, T. J. (1981). Effects of massed and distributed practice on the learning and retention of second-language vocabulary. J. Educ. Res. 74, 245–248. doi: 10.1080/00220671.1981.10885317
Brunmair, M., and Richter, T. (2019). Similarity matters: a meta-analysis of interleaved learning and its moderators. Psychol. Bull. 145, 1029–1052. doi: 10.1037/bul0000209
Butler, A. C. (2010). Repeated testing produces superior transfer of learning relative to repeated studying. J. Exp. Psychol. Learn. Mem. Cogn. 36, 1118–1133. doi: 10.1037/a0019902
Campbell, K. L., Trelle, A., and Hasher, L. (2014). Hyper-binding across time: age differences in the effect of temporal proximity on paired-associate learning. J. Exp. Psychol. Learn. Mem. Cogn. 40, 293–299. doi: 10.1037/a0034109
Carpenter, S. K., Cepeda, N. J., Rohrer, D., Kang, S. H., and Pashler, H. (2012). Using spacing to enhance diverse forms of learning: review of recent research and implications for instruction. Educ. Psychol. Rev. 24, 369–378. doi: 10.1007/s10648-012-9205-z
Cazan, A.-M. (2020). An intervention study for the development of self-regulated learning skills. Curr. Psychol. 41, 6406–6423. doi: 10.1007/s12144-020-01136-x
Chamot, A. U., and O'Malley, J. M. (1987). The cognitive academic language learning approach: a bridge to the mainstream. TESOL Q. 21, 227–249. doi: 10.2307/3586733
Chan, J. C., McDermott, K. B., and Roediger, H. L. (2006). Retrieval-induced facilitation: initially nontested material can benefit from prior testing of related material. J. Exp. Psychol. Gen. 135, 553–571. doi: 10.1037/0096-3445.135.4.553
Chen, O., Paas, F., and Sweller, J. (2021). Spacing and interleaving effects require distinct theoretical bases: a systematic review testing the cognitive load and discriminative-contrast hypotheses. Educ. Psychol. Rev. 33, 1499–1522. doi: 10.1007/s10648-021-09613-w
Chi, M. T., De Leeuw, N., Chiu, M.-H., and LaVancher, C. (1994). Eliciting self-explanations improves understanding. Cogn. Sci. 18, 439–477. doi: 10.1016/0364-0213(94)90016-7
Coffman, J. L., Grammer, J. K., Hudson, K. N., Thomas, T. E., Villwock, D., and Ornstein, P. A. (2019). Relating children’s early elementary classroom experiences to later skilled remembering and study skills. J. Cogn. Dev. 20, 203–221. doi: 10.1080/15248372.2018.1470976
College voor Toetsen en Examens (2024). Examenblad [Online]. Available at: https://www.examenblad.nl [Accessed 01-13-2024].
Crippen, K. J., and Earl, B. L. (2007). The impact of web-based worked examples and self-explanation on performance, problem solving, and self-efficacy. Comput. Educ. 49, 809–821. doi: 10.1016/j.compedu.2005.11.018
De Boer, H., Donker, A. S., and Van der Werf, M. P. (2014). Effects of the attributes of educational interventions on students’ academic performance: a meta-analysis. Rev. Educ. Res. 84, 509–545. doi: 10.3102/0034654314540006
Dirkx, K. J. H., Camp, G., and Kester, L. (2019). Do secondary school students make use of effective study strategies when they study on their own? Appl. Cognit. Psychol. 33, 952–957. doi: 10.1002/acp.3584
Dunlosky, J., Rawson, K. A., Marsh, E. J., Nathan, M. J., and Willingham, D. T. (2013). Improving students’ learning with eff ective learning techniques: promising directions from cognitive and educational psychology. Psychol. Sci. Public 14, 4–58. doi: 10.1177/1529100612453266
Firth, J., Rivers, I., and Boyle, J. (2021). A systematic review of interleaving as a concept learning strategy. Rev. Educ. 9, 642–684. doi: 10.7565/ssp.2019.2650
Freire, M., Talanquer, V., and Amaral, E. (2019). Conceptual profile of chemistry: a framework for enriching thinking and action in chemistry education. Int. J. Sci. Educ. 41, 674–692. doi: 10.1080/09500693.2019.1578001
Golding, J. M., Wasarhaley, N. E., and Fletcher, B. (2012). The use of flashcards in an introduction to psychology class. Teach. Psychol. 39, 199–202. doi: 10.1177/0098628312450436
Grammer, J., Coffman, J. L., and Ornstein, P. (2013). The effect of teachers' memory-relevant language on children's strategy use and knowledge. Child Dev. 84, 1989–2002. doi: 10.1111/cdev.12100
Griffin, T. D., Wiley, J., and Thiede, K. W. (2008). Individual differences, rereading, and self-explanation: concurrent processing and cue validity as constraints on metacomprehension accuracy. Mem. Cogn. 36, 93–103. doi: 10.3758/mc.36.1.93
Havekes, H., Arno-Coppen, P., and Luttenberg, J. (2012). Knowing and doing history: a conceptual framework and pedagogy for teaching historical contextualisation. History Educ. Res. J. 11, 72–93. doi: 10.18546/HERJ.11.1.06
Hew, K. F., and Cheung, W. S. (2014). “Enhancing students’ learning of factual knowledge” in Using blended learning. Springerbriefs in education. Singapore: Springer.
Hudson, K. N., Coffman, J. L., and Ornstein, P. A. (2018). Addition in kindergarten: the role of mothers’ and teachers’ language. J. Cogn. Dev. 19, 65–86. doi: 10.1080/15248372.2017.1415900
Johnson, C. I., and Mayer, R. E. (2009). A testing effect with multimedia learning. J. Educ. Psychol. 101, 621–629. doi: 10.1037/e527342012-537
Karpicke, J. D., and Blunt, J. R. (2011). Retrieval practice produces more learning than elaborative studying with concept mapping. Science 331, 772–775. doi: 10.1126/science.1199327
Kazemi, E., and Stipek, D. (2009). Promoting conceptual thinking in four upper-elementary mathematics classrooms. J. Educ. 189, 123–137. doi: 10.1177/0022057409189001-209
Kikas, E., Mädamürk, K., Hennok, L., Sigus, H., Talpsep, T., Luptova, O., et al. (2021). Evaluating the efficacy of a teacher-guided comprehension-oriented learning strategy intervention among students in grade 4. Eur. J. Psychol. Educ. 37, 509–530. doi: 10.1007/s10212-021-00538-0
Kim, S. K., and Webb, S. (2022). The effects of spaced practice on second language learning: a meta-analysis. Lang. Learn. 72, 269–319. doi: 10.1111/lang.12479
Kistner, S., Rakoczy, K., Otto, B., Klieme, E., and Büttner, G. (2015). Teaching learning strategies. The role of instructional context and teacher beliefs. J. Educ. Res. Online 7, 176–197. doi: 10.25656/01:11052
Kornell, N., and Bjork, R. A. (2008). Learning concepts and categories: is spacing the “enemy of induction”? Psychol. Sci. 19, 585–592. doi: 10.1111/j.1467-9280.2008.02127.x
Küpper-Tetzel, C. E. (2014). Understanding the distributed practice effect. Z. Psychol. 222, 71–81. doi: 10.1027/2151-2604/a000168
Küpper-Tetzel, C. E., Erdfelder, E., and Dickhäuser, O. (2014). The lag effect in secondary school classrooms: enhancing students’ memory for vocabulary. Instr. Sci. 42, 373–388. doi: 10.1007/s11251-013-9285-2
Lane, R., Carter, J., and Bourke, T. (2019). Concepts, conceptualization, and conceptions in geography. J. Geogr. 118, 11–20. doi: 10.1080/00221341.2018.1490804
Latimier, A., Peyre, H., and Ramus, F. (2021). A meta-analytic review of the benefit of spacing out retrieval practice episodes on retention. Educ. Psychol. Rev. 33, 959–987. doi: 10.1007/s10648-020-09572-8
Leonard, L. B., and Deevy, P. (2020). Retrieval practice and word learning in children with specific language impairment and their typically developing peers. J. Speech Lang. Hear. Res. 63, 3252–3262. doi: 10.1044/2020_jslhr-20-00006
McDaniel, M. A., and Einstein, G. O. (2020). Training learning strategies to promote self-regulation and transfer: the knowledge, belief, commitment and planning framework. Perspect. Psychol. Sci. 15, 1363–1381. doi: 10.1177/1745691620920723
Medina, D., and Nagamine, M. (2019). Autonomous learning strategies in the Reading comprehension of high school students. J. Educ. Psychol. 7, 147–159. doi: 10.20511/pyr2019.v7n2.276
Mevarech, Z. R., and Kramarski, B. (2003). The effects of metacognitive training versus worked-out examples on students' mathematical reasoning. Br. J. Educ. Psychol. 73, 449–471. doi: 10.1348/000709903322591181
Meyer, A. N., and Logan, J. M. (2013). Taking the testing effect beyond the college freshman: benefits for lifelong learning. Psychol. Aging 28, 142–147. doi: 10.1037/a0030890
Miller, K. F., Kelly, M., and Zhou, X. (2005). “Learning mathematics in China and the United States: cross-cultural insights into the nature and course of preschool mathematical development” in The handbook of mathematical cognition. ed. I. D. Campbell (New York: Psychology Press), 163–178.
Morehead, K., Rhodes, M. G., and DeLozier, S. (2015). Instructor and student knowledge of study strategies. Memory 24, 257–271. doi: 10.1080/09658211.2014.1001992
Moreno, K., Bhattacharjee, S., and Brandon, D. M. (2005). The effectiveness of alternative training techniques on analytical procedures performance. SSRN Electron. J. doi: 10.2139/ssrn.739785
Nejabati, N. (2015). The effects of teaching self-regulated learning strategies on EFL students' reading comprehension. J. Lang. Teach. Res. 6:1343. doi: 10.17507/jltr.0606.23
Ornstein, P. A., and Coffman, J. L. (2020). Toward an understanding of the development of skilled remembering: the role of teachers’ instructional language. Curr. Dir. Psychol. Sci. 29, 445–452. doi: 10.1177/0963721420925543
Ornstein, P., Coffman, J., Grammer, J., San Souci, P., and McCall, L. (2010). “Linking the classroom context and the development of children’s memory skills” in Handbook of research on schools, schooling and human development (Routledge), 60–77.
Pashler, H., Rohrer, D., Cepeda, N. J., and Carpenter, S. K. (2007). Enhancing learning and retarding forgetting: choices and consequences. Psychon. Bull. Rev. 14, 187–193. doi: 10.3758/bf03194050
Pastötter, B., and Bäuml, K.-H. T. (2014). Retrieval practice enhances new learning: the forward effect of testing. Front. Psychol. 5:286. doi: 10.3389/fpsyg.2014.00286
Polack, C. W., and Miller, R. R. (2022). Testing improves performance as well as assesses learning: a review of the testing effect with implications for models of learning. J. Exp. Psychol. 48, 222–241. doi: 10.1037/xan0000323
Renkl, A., Stark, R., Gruber, H., and Mandl, H. (1998). Learning from worked-out examples: the effects of example variability and elicited self-explanations. Contemp. Educ. Psychol. 23, 90–108. doi: 10.1006/ceps.1997.0959
Richland, L. E., Stigler, J. W., and Holyoak, K. J. (2012). Teaching the conceptual structure of mathematics. Educ. Psychol. 47, 189–203. doi: 10.1080/00461520.2012.667065
Ritchie, J., Lewis, J., Nicholls, C. M., and Ormston, R. (2014). Qualitative research practice: A guide for social science students and researchers. second Edn Sage.
Rittle-Johnson, B., Loehr, A. M., and Durkin, K. (2017). Promoting self-explanation to improve mathematics learning: a meta-analysis and instructional design principles. ZDM 49, 599–611. doi: 10.1007/s11858-017-0834-z
Rittle-Johnson, B., Siegler, R. S., and Alibali, M. W. (2001). Developing conceptual understanding and procedural skill in mathematics: an iterative process. J. Educ. Psychol. 93, 346–362. doi: 10.1037/0022-0663.93.2.346
Roediger, H. L., and Butler, A. C. (2011). The critical role of retrieval practice in long-term retention. Trends Cogn. Sci. 15, 20–27. doi: 10.1016/j.tics.2010.09.003
Roediger, H. L., and Karpicke, J. D. (2006). The power of testing memory: basic research and implications for educational practice. Perspect. Psychol. Sci. 1, 181–210. doi: 10.1111/j.1745-6916.2006.00012.x
Roediger, H. L., and Marsh, E. J. (2005). The positive and negative consequences of multiple-choice testing. J. Exp. Psychol. Learn. Mem. Cogn. 31, 1155–1159. doi: 10.1037/0278-7393.31.5.1155
Roediger, H. L., and Pyc, M. A. (2012). Inexpensive techniques to improve education: applying cognitive psychology to enhance educational practice. J. Appl. Res. Mem. Cogn. 1, 242–248. doi: 10.1016/j.jarmac.2012.09.002
Rohrer, D. (2009). Research commentary: the effects of spacing and mixing practice problems. J. Res. Math. Educ. 40, 4–17. doi: 10.5951/jresematheduc.40.1.0004
Rohrer, D. (2012). Interleaving helps students distinguish among similar concepts. Educ. Psychol. Rev. 24, 355–367. doi: 10.1007/s10648-012-9201-3
Rohrer, D., and Taylor, K. (2007). The shuffling of mathematics problems improves learning. Instr. Sci. 35, 481–498. doi: 10.1007/s11251-007-9015-8
Rowland, C. A. (2014). The effect of testing versus restudy on retention: a meta-analytic review of the testing effect. Psychol. Bull. 140, 1432–1463. doi: 10.1037/a0037559
Schwieren, J., Barenberg, J., and Dutke, S. (2017). The testing effect in the psychology classroom: a meta-analytic perspective. Psychol. Learn. Teach. 16, 179–196. doi: 10.1177/1475725717695149
Siegler, R. S., and Chen, Z. (2008). Differentiation and integration: guiding principles for analyzing cognitive change. Dev. Sci. 11, 433–448. doi: 10.1111/j.1467-7687.2008.00689.x
Smith, C. D., and Scarf, D. (2017). Spacing repetitions over long timescales: a review and a reconsolidation explanation. Front. Psychol. 8:962. doi: 10.3389/fpsyg.2017.00962
Sperling, R. A., Ramsay, C. M., Reeves, P. M., Follmer, D. J., and Richmond, A. S. (2016). Supporting students’ knowledge construction and self-regulation through the use of elaborative processing strategies. Middle Sch. J. 47, 25–32. doi: 10.1080/00940771.2015.1135099
Stalmeijer, R. E., McNaughton, N., and Van Mook, W. N. (2014). Using focus groups in medical education research: AMEE guide no. 91. Med. Teach. 36, 923–939. doi: 10.3109/0142159x.2014.917165
Surma, T., Vanhoyweghen, K., Camp, G., and Kirschner, P. A. (2018). The coverage of distributed practice and retrieval practice in Flemish and Dutch teacher education textbooks. Teach. Teach. Educ. 74, 229–237. doi: 10.1016/j.tate.2018.05.007
Trassi, A. P., Oliveira, K. L., and Inácio, A. L. M. (2019). Reading comprehension, learning strategies and verbal reasoning: possible relationships. Psico-USF 24, 615–624. doi: 10.1590/1413-82712019240401
Van den Hurk, H., Houtveen, A., and Van de Grift, W. (2016). Fostering effective teaching behavior through the use of data-feedback. Teach. Teach. Educ. 60, 444–451. doi: 10.1016/j.tate.2016.07.003
Van Rijt, J. H., De Swart, P. J., Wijnands, A., and Coppen, P.-A. J. (2019). When students tackle grammatical problems: exploring linguistic reasoning with linguistic metaconcepts in L1 grammar education. Linguist. Educ. 52, 78–88. doi: 10.1016/j.linged.2019.06.004
Vlach, H. A. (2014). The spacing effect in children's generalization of knowledge: allowing children time to forget promotes their ability to learn. Child Dev. Perspect. 8, 163–168. doi: 10.1111/cdep.12079
Woloshyn, V. E., Paivio, A., and Pressley, M. (1994). Use of elaborative interrogation to help students acquire information consistent with prior knowledge and information inconsistent with prior knowledge. J. Educ. Psychol. 86, 79–89. doi: 10.1037/0022-0663.86.1.79
Wong, S. S. H., Ng, G. J. P., Tempel, T., and Lim, S. W. H. (2019). Retrieval practice enhances analogical problem solving. J. Exp. Educ. 87, 128–138. doi: 10.1080/00220973.2017.1409185
Wood, E., Pressley, M., and Winne, P. H. (1990). Elaborative interrogation effects on children's learning of factual content. J. Educ. Psychol. 82, 741–748. doi: 10.1037/0022-0663.82.4.741
Yang, C., Potts, R., and Shanks, D. R. (2018). Enhancing learning and retrieval of new information: a review of the forward testing effect. NPJ Sci. Learn. 3, 1–9. doi: 10.1038/s41539-018-0024-y
Keywords: effective learning strategies, knowledge dimensions, learning strategy instruction, study strategies, subject specific effectiveness of effective learning strategies
Citation: Langbroek S, Duchatelet D, Joosten-ten Brinke D and Camp G (2024) Enhancing teachers’ instruction on how to study: an exploration of the effectiveness of learning strategies for particular secondary school subjects. Front. Educ. 9:1340120. doi: 10.3389/feduc.2024.1340120
Edited by:
Dominik E. Froehlich, University of Vienna, AustriaReviewed by:
Maria Sofologi, University of Ioannina, GreeceHanneke Theelen, Zuyd University of Applied Sciences, Netherlands
Copyright © 2024 Langbroek, Duchatelet, Joosten-ten Brinke and Camp. This is an open-access article distributed under the terms of the Creative Commons Attribution License (CC BY). The use, distribution or reproduction in other forums is permitted, provided the original author(s) and the copyright owner(s) are credited and that the original publication in this journal is cited, in accordance with accepted academic practice. No use, distribution or reproduction is permitted which does not comply with these terms.
*Correspondence: Sander Langbroek, sander.langbroek@maastrichtuniversity.nl