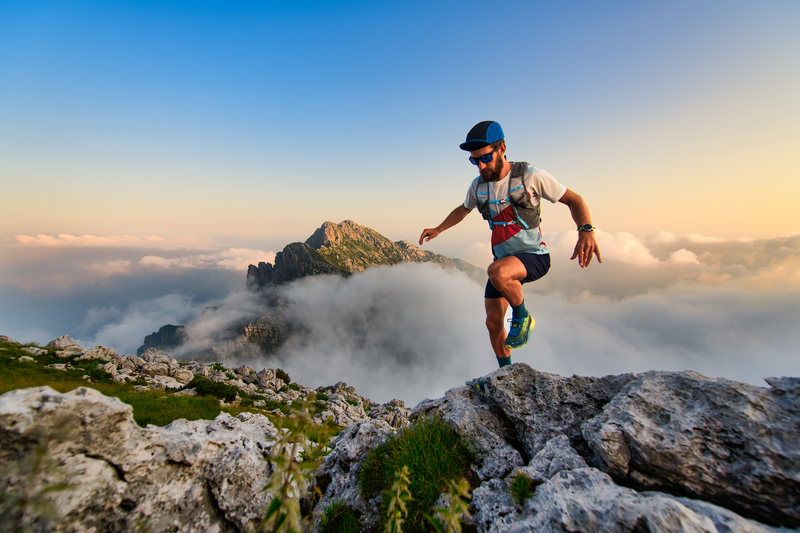
94% of researchers rate our articles as excellent or good
Learn more about the work of our research integrity team to safeguard the quality of each article we publish.
Find out more
BRIEF RESEARCH REPORT article
Front. Educ. , 10 July 2024
Sec. Digital Education
Volume 9 - 2024 | https://doi.org/10.3389/feduc.2024.1339623
This article is part of the Research Topic Cognitive Benefits of Technologies Applied to Learning in Education View all 19 articles
Exploring deep learning and its influencing factors is receiving increasing attention. This study examines influencing factors (i.e. self-efficacy, learning engagement, and teacher-student interaction) of high school students' English deep learning in blending courses. Research hypotheses are proposed, and a structural equation model (SEM) of influencing factors of deep learning is built. A sample size of 225 participants was recruited on a voluntary and anonymous basis from a high school in Southwestern China for this study. We find: (1) self-efficacy, learning engagement and teacher-student interaction all predict and have positive impacts on English deep learning; (2) self-efficacy has a positive impact on learning engagement and teacher-student interaction; (3) teacher-student interaction has a positive impact on learning engagement. The findings underscore the importance of self-efficacy, learning engagement, and teacher-student interaction for EFL high school learners to achieve deep learning in a blending context. Drawn from these findings, pedagogical implications for promoting these learners' deep learning are provided.
As a symbol of high-level learning and a method that is conducive to improving learners' learning ability, deep learning has attracted more and more attention from researchers (Fullan et al., 2018). Horizon Report issued by New Media Consortium (NMC) in 2017 and Chinese higher education vision of Horizon Report aiming at the application status of higher education technology in China delivered by Smart Learning Institute of Beijing Normal University both mention that key tendency of the application of technology is turning from the tangible learning forms and learning spaces to intangible innovation and deep learning (Gao and Huang, 2017).
How can traditional education model further promote students' deep learning? In accordance with Action Plan of Educational informatization 2.0, issued by Ministry of Education of the People's Republic of China in 2018, educational informatization is the core and symbol of education modernization—We should wield the advantage of technology and transform the traditional model to accelerate the deep integration between new technology and education. The action plan aims to promote the implementation of “Internet + Education.” Tech-enhanced blending courses then have emerged. With the support of hardware and information technology such as smart devices and mobile learning apps, the blending courses, characterized by “Internet-accessible smart devices + traditional classroom teaching,” can realize the sharing of high-quality teaching resources and improve the effectiveness of teaching and learning. According to Action Plan of Educational informatization 2.0, smart education development and innovation action is one of its most important contents and integrated technology education that aims to promote deep learning is the core pillar of smart education.
The blending courses teaching environment based on the smart education pays more attention on the integration of physical environment of smart classroom and innovative smart teaching. The cramming strategy, for the traditional high-school English teaching in China, makes students likely to choose a shallow learning method, that is, one that does not require too much effort, and does not require too much understanding to easily obtain kind of “success,” which is the so-called high score. Blending courses aim to provide students more humanistic learning environment in which teachers, armed with technology, are able to design a variety of classroom activities and adopt multiple teaching strategies through smart devices to encourage students to participate more in the process of teaching and learning. So, can high school students in these blending courses truly achieve English deep learning? What factors affect students' deep learning?
So far, a great number of studies have been done on favorable effects of the use of new Internet technology in a learning process in terms of facilitation and enhancement of learning (Allen, 2011). In previous studies, some researchers tested the relationship between only a single influencing factor and deep learning, or explored the reasons why students' deep learning did not happen from the perspective of teachers by focusing on post-secondary setting, or analyzed and summarized the influencing factors of deep learning, but there is either a lack of data-based analysis or a theoretical framework for analysis. We need to conduct in-depth discussions on the influencing factors and mechanism of English deep learning in blending courses. To this end, this research draws on the existing research results, analyzes and discusses the factors (learning engagement, self-efficacy, and teacher-student interaction) that affect the English deep learning of high school students in the blending courses, and proposes a theoretical hypothesis model. The model is validated using measurement data, which reveals the impacts of influencing factors on English deep learning and their interrelationships.
Blending teaching assisted by smart devices mixes online learning with traditional face-to-face classroom instruction, offering a novel approach for achieving the deep learning of students. The research questions are as follows:
(1) How do those influencing factors (i.e., learning engagement, self-efficacy, and teacher-student interaction) affect the English deep learning in blending course?
(2) What are the relationships between the various influencing factors?
As a future education trend and learning idea, deep learning is an in-depth progression of the blending courses model. Blending learning (B-learning) is a teaching model that combines the benefits of traditional classroom teaching (Face-to-Face) and network teaching (e-Learning) (Delialioglu and Yildirim, 2007). Its starting point is to increase students' in-depth understanding and the fundamental goal is to allow students to go from shallow to deep learning, develop their low-level cognition to a higher one (Zhu, 2016), with the goal of assisting students in achieving optimal learning and promoting students to reach the level of deep learning (Zhang and Wang, 2014). Furthermore, the blending courses teaching model and deep learning processes adapt to and complement one another. Deep learning consisted of three processes: “acquiring information (understanding of shallow learning level)—developing skills (ability to analyze and reflect)—deep learning (problem solving, application of inventive capacity).” This basically matched the process of blending courses teaching model. In the pre-class stage of blending courses, students obtained the basic information of knowledge points online using smart devices, and then in the face-to-face teaching stage in the classroom, students reflected on knowledge and established their own knowledge framework through interaction with teachers. Finally, students addressed practical issues based on the knowledge they have gained in order to achieve internalization and consolidation of knowledge. The blending courses teaching model combined the benefits of online and offline teaching, and according to cognitive load theory, the overall burden in teaching is fairly divided in three stages: pre-class, in-class, and after-class stage, making it easier for students to achieve deep learning (Zhang and Wang, 2014). As a result, we can see that blending courses teaching and deep learning are tightly linked, and the two are interdependent, making it possible for us to increase the effectiveness of English instruction. Scholars have been studying the actual teaching effects in the blending courses of deep learning, and they have proven, through theoretical and practical studies, that it can actually promote deep learning (e.g., He et al., 2019; Tan, 2019).
When Akyol and Garrison (2011) investigated the issues of cognitive presence in pure online and blending inquiry-based communities, they discovered that in blending inquiry-based communities, cognitive presence and perceptual and factual learning outcomes were higher than in pure online learning, and learners were more likely to reach deep learning. According to Nazarenko (2015), a blending format promoted the development of students' professional and informational capabilities in a case study research based on the experience of applying a blending learning approach. Wang et al. (2017) analyzed the behavior of deep and shallow learners in a blending learning environment using the learning behavior analysis approach and discovered that deep learners were more likely to actively participate in classroom activities. In terms of the further effects of blending learning practice, Du and Fu (2016) investigated the learning effects of MOOC blending teaching and found that the design of MOOC blending teaching can increase teaching quality and meet the intended in-depth learning goals. To deepen the learning depth of blending learning, Peng and Yang (2017) proposed that it was necessary to start from the construction of blending learning field, improvement of teachers' teaching design capabilities, and training of blending learning control capabilities to fully leverage the power of big data analysis skills in order to form synergies. As a result, we may assume that the blending courses teaching model seeks deep learning to some level, which is a potential prerequisite of blending teaching.
Learning engagement refers to the level of behavioral participation and emotional experience students have when they begin and complete learning activities. Learning engagement is a pleasant and continuous emotional state demonstrated by learners during the course of learning activities, with the key characteristics of activity, attentiveness, and devotion (Gao et al., 2015). Activity referred to the level of effort and persistence learners put into learning; attentiveness referred to the degree to which the learner is attentive in the learning process and devotion primarily referred to learners' pride and excitement for learning activities, as well as their willingness to overcome the problems faced in learning (Schaufeli et al., 2002). Engagement consists of behavioral, emotional, and cognitive components; behavioral engagement refers to actively participating in professional and social activities; emotional engagement refers to academic aspirations and a sense of belonging to the school; and cognitive engagement refers to the methods and strategies used to improve learning efficiency (Fredricks et al., 2004).
“Engagement” can be embodied through behaviors such as beginning, participating, working hard, persistence, continuing to try in the face of difficulties or failures, and positive emotional experiences (such as positivity, optimism, enthusiasm, happiness, curiosity, and interest), and can be demonstrated through reflection of behavior toward the goal of struggle. Learning engagement had been proven in studies to be an essential indication of students' learning in school and to have a direct and stable association with the learning successes that students may attain (Bresó et al., 2011). A higher degree of learning engagement was beneficial for learners in achieving their learning objectives and obtaining desirable learning outcomes (Ronimus et al., 2014). Besides, when students were studying, behavioral engagement was an important observation index of their learning engagement, and it was an explicit feature of learning engagement. That is to say, they would have a serious and committed attitude, actively communicated with teachers and classmates, paid attention to information comprehension and problem solving, and built creativity and cooperation abilities. As a result, behavioral engagement was a prerequisite for deep learning, a key element influencing academic performance and results (Johnson and Sinatra, 2013), and an essential indicator of educational progress (Kim et al., 2016). According to Liu and Wang (2017), behavioral engagement can lessen the negative effects of cognitive load on students, prevent shallow learning, enhance the development of students' collaborative and metacognitive abilities, and complete deep learning capacity training in a virtual reality context. Zhao et al. (2013) also discovered that learning engagement encouraged critical thinking and cooperative communication, as well as deep learning. In other words, the level of students' learning engagement determined the quality of CALL to a large extent. Thus, in the novel blending courses teaching model, students' learning engagement can be assumed to have a big influence on deep learning promotion and specifies the quality of teaching that can be accomplished by employing the model.
An individual's self-efficacy is defined as his/her belief, judgment, or subjective self-experience about his/her ability to complete a task. It's a crucial cognitive factor that influences self-regulated learning (Bandura, 1998).
Researchers discovered that self-efficacy predicted and explained academic accomplishment (Vayre and Vonthron, 2017). Self-efficacy could improve learning effectiveness and was an essential component influencing learning motivation and predicting learning outcomes (Yin and Xu, 2011; Tsai, 2012). People with high self-efficacy, according to the findings of the aforementioned studies, had a stronger potential to improve their own abilities, so as to achieve higher level learning. Deep learning was linked to self-efficacy, according to Papinczak et al. (2008), and students with strong self-efficacy can attain higher levels of deep learning. In an intelligent device-assisted teaching environment, Lee and Choi (2017) investigated the influencing elements of deep learning and discovered that students' self-efficacy and evaluation methods influenced the level of deep learning. Furthermore, studies have shown that self-efficacy can predict learning engagement (Bresó et al., 2011). When faced with a difficult or challenging task, individuals with higher self-efficacy were able to persevere on the task for longer periods of time and are less likely to give up in the middle (Chouinard et al., 2007). According to Bandura's theory of social cognition, if learners wished to maintain a high level of learning engagement, they have a strong sense of self-efficacy in order to retain a decent level of motivation to commit themselves to learning activities. We can deduce that self-efficacy will have a positive impact on high school students' learning engagement based on this. The relevant findings from the study on self-efficacy and teacher-student interaction revealed that increasing students' self-efficacy had a significant impact on the frequency and degree of teacher-student interaction (Huang et al., 2015). The research of Dong (2015) further demonstrated that high school students' chemistry classroom participation was significantly influenced by their chemistry learning self-efficacy. The better the students' chemistry learning self-efficacy, the greater their classroom participation and academic success in chemistry. Self-efficacy determines the content and nature of the learner's imaginal realization of future learning scenarios or processes, which affects the individual's motivational psychology in the implementation of learning activities, and thus has an impact on actual learning activities, directly or indirectly. Thus, this study infers that self-efficacy has a beneficial influence on teacher-student interaction in a blending English course.
The different forms, qualities, and degrees of interaction and their impacts between teachers and students in the classroom teaching environment are referred to as “teacher-student interaction”.
In the specific learning environment of the blending courses, smart devices and information technology have substantially enhanced the opportunities and means of interactions between teachers and students. Compared to that in the conventional classroom, the interaction between teachers and students in the blending courses teaching model includes the following features: first, the teacher-student interaction forms exhibit a tendency of variety and flexibility with the use of smart technologies. The advancement of information technology lessens the sensation of distance in online virtual interactions while simultaneously increasing the flexibility of real-world interactions in classrooms. Second, the content of teacher-student interaction is more open, and is no longer limited to specific textbook knowledge and problem solving in classrooms. In reform-based blending courses teaching, students have a variety of possibilities to communicate with teachers and share their thoughts and learning experiences (Rimmkaufman et al., 2015). On the research of college students' learning engagement, Zhu (2010) discovered that the interaction between teachers and students in the school had an important impact on learning engagement. Among them, teacher-student interaction in classroom was the most significant factor. After performing an investigation in a university classroom in China, Ma et al. (2011) developed a model of the influence of teacher-student interaction on teaching quality. According to the findings of the study, the influence of teacher-student interaction on students' learning behavior is primarily achieved through learning motivation, and teachers' teaching concept, teaching level, and teaching strategy all have significant impacts on students' learning motivation; teachers can stimulate students by increasing the degree of learning participation, thereby transforming students from passive to active learners. Thus, this study assumes that in the blending courses, teacher-student interaction will have a positive influence on students, encouraging them to have more active learning beliefs and behaviors, deepen their learning engagement, and finally achieve the standards of deep learning.
There were 225 second-year high school students from a key middle school in Southwestern China participated in the questionnaire survey, with 107 males and 118 females, and the ages of them were from 16 to 18.
This middle school began to explore the reform of a blending courses teaching model in 2018, first conducting pilot teaching in the three subjects of Chinese, Mathematics and English. The school combines advanced and mature educational informatization research results and information technology methods with the actual situation and needs of the school's teaching and learning, and draws on the experience of building smart classrooms in universities and colleges, and proposes a design for blending courses construction from eight aspects to build a three-in-one construction model of “the integration of resource space, physical space, interactive space, and multi-dimensional data collection and application” (He and Huang, 2018). Through 3 years of construction and development, with the support of smart devices and information technology, the school's blending courses teaching model has achieved significant results. It can be seen that the students in this middle school already have the experience of learning in a blended classroom, and they are quite appropriate to be selected as the research participants of this study.
The questionnaire consists of four subscales, including the deep learning scale, the learning engagement scale, the teacher-student interaction scale and the self-efficacy scale. It is a five-point Likert scale (1–5 respectively indicate “totally disagree,” “basically disagree,” “neural,” “basically agree” and “totally agree”).
(1) deep learning scale
This scale is adapted from the deep learning subscale constructed by the NSSE-China research group of the Institute of Education of Tsinghua University (Shi et al., 2011).
(2) learning engagement scale
It was formed on the basis of the Utrecht Work Engagement Scale-Student scale (UWES-S) (Zhang and Gan, 2005).
(3) self-efficacy scale
The scale uses the Chinese version of the General Self-Efficacy Scale (GSES) compiled by Schwarzer et al. (Wang et al., 2001).
(4) teacher-student interaction scale
The scale is the Chinese version of the teacher-student interactive questionnaire developed by Kang (Zhang, 2019).
Before the questionnaires were formally distributed, the researcher attended several classes accompanied by the English teachers of the tested classes. In order to comply with the ethical requirements of the university being studied and maintain anonymity, detailed information of all participants has been omitted. Before seeking their consent, participants were informed of the research objectives and confidentiality of the study before the administration of questionnaires and then ensured that the responses to the questionnaires would be treated confidentially and could be withdrawn at any time. All participants consented to be involved in the study voluntarily. A total of 225 questionnaires were sent to all classes. The 225 surveys were all gathered. The questionnaire recovery rate was 100%. The researchers excluded questionnaires with questions forgotten to answer and all questions of the same answers. A total of 216 valid surveys were gathered, and the questionnaire's valid rate was 96%. Throughout the data collection process, the researchers strictly followed ethical standards.
Based on the previous research on the influencing factors of English deep learning of high school students in blending courses, the following research hypotheses are made:
H1: Learning engagement, self-efficacy and teacher-student interaction all predict and have positive effects on deep learning.
H2: Teacher-student interaction has a positive effect on learning engagement and self-efficacy has a positive effect on both learning engagement and teacher-student interaction.
Based on the above hypotheses, this research constructs a theoretical research model of influencing factors of English deep learning of high school students in blending courses, as shown in Figure 1.
SPSS26.0 was used to test the reliability and validity of the model. The reliability test is generally based on the Cronbach's alpha, and the validity test is generally based on the Value of KMO and Bartlett's. As shown in Table 1, the coefficients of each scale are >0.8, indicating that the internal consistency of the questionnaire is high. The questionnaire's overall reliability coefficient is 0.924, suggesting that the consistency and stability of the questionnaire's items are quite good. As a result, all of the data obtained in this study's questionnaire have extremely high reliability.
As shown in Table 2, The entire scale's KMO value is greater than 0.8, indicating that there is no significant difference between items in terms of correlation, and the Bartlett's Test of Sphericity result p = 0.000, which reaches a significant level, suggesting that the items of the questionnaire are not independent, and the questionnaire is valid.
In summary, both reliability and validity of the entire scale are generally high in this study, and the test findings fulfill the relevant particular requirements, suggesting that the data are acceptable for factor analysis.
The fitness index is used to verify the structural equation model. Various indicators use certain values as the foundation for their assessment as the primary measure of the degree of fit between the model and the data. Table 3 shows the acceptable standard for the commonly used fitness index values in structural equation models and the fitness index summary of the original model after using AMOS25.0
In terms of the influencing factor model's fitness index, when compared to the reference standard for the value of the fitness index, the original model has to be altered and modified to some amount. AGFI = 0.791, which was less than the acceptable standard value of 0.8; CF1 = 0.883, which was smaller than the acceptable standard value of 0.9; and RMSEA = 0.088, which was greater than the standard value of 0.08. All of these values did not satisfy the level of fitness. The remaining indicators have all attained the standard value.
The fitness index in Table 3 shows that the fitness effect of the structural equation model is not ideal, indicating that the original model has to be modified to some extent. After modification for 4 times, the influencing factor model of English deep learning for high school students in the blending courses finally meets the standard of fitness index. All data of the modified model's fitness are shown in Table 4 and the modified model diagram is obtained as shown in Figure 2.
The path coefficient map of the modified model is shown in Figure 3.
The model of influencing factors of high school students' English deep learning in blending courses, as shown in Figure 3, contains four latent variables: self-efficacy, learning engagement, teacher-student interaction, and deep learning. The relationship between the four latent variables can be analyzed and explained through this structural equation model: self-efficacy, learning engagement and teacher-student interaction all predict and have direct positive impacts on deep learning. Among them, the influence effect of self-efficacy is 0.37, the influence coefficient of learning input factor is 0.38, and the influence coefficient of teacher-student interaction factor is relatively small, 0.19; Learning engagement is directly influenced by self-efficacy and teacher-student interaction, of which the influence coefficient of self-efficacy is 0.22, and the influence coefficient of teacher-student interaction is 0.45; Finally, teacher-student interaction is influenced by self-efficacy, with an influence coefficient of 0.47.
The researcher validates the hypotheses proposed in this study using the analysis described above. Theory-based research hypotheses are confirmed, by using AMOS25.0, through confirmatory factor analysis between the theoretical model and the questionnaire data, the fitness test of the model fitness index and the model modification. To some extent, the model is scientific, and it serves as a reference for high school to carry out blending courses teaching and to increase high school students' English deep learning.
In this study, students' self-efficacy has a significant positive effect on their English deep learning in the blending courses, which is consistent with the conclusion drawn by the previous studies (Papinczak et al., 2008; Yin and Xu, 2011; Tsai, 2012; Lee and Choi, 2017). It indicates that increasing students' self-efficacy will result in an increase in students' English deep learning.
In the blending courses, students' positive feelings or beliefs in the face of various English learning activities and learning scenarios have a major influence on English deep learning. The more confident students are in English learning under a variety of learning activities and circumstances in blending courses, the deeper their English learning may be. If students believe they can have gains in the blending courses, they will be more active in studying relevant course content; if students believe they will not learn much in blending courses, they will devote less energy to learning assignments. Nilsen's action research points out that students' self-efficacy significantly affects students' motivation to learn deeply. The more self-confidence students have in their ability to learn, the more time they will dedicate to learning assignments, and vice versa (Nilsen, 2009). Zhang's research on the relationship between college students' self-efficacy and deep learning in a digital environment discovers that online self-efficacy may predict deep learning significantly. Her research also suggests that increasing online self-efficacy encourage learners to employ more diverse learning techniques (Zhang, 2015), which is consistent with the fact that in this study, self-efficacy has a substantial impact on students' English deep learning, and students with a higher level of self-efficacy are more likely to have greater self-confidence, gain a stronger potential to improve their own abilities, be able to control over their behaviors to get desired results, and persevere on the task for longer periods of time, so as to achieve higher level learning.
Students' self-efficacy positively affects their learning engagement in the blending course. As we have mentioned previously, in this blending context the overall burden in teaching and learning is fairly divided in three stages: pre-class, in-class, and after-class stage, making it easier for students to learn. Students in this context are more likely to have a lower level of learning load, and they are also provided with more humanistic learning environment, and teachers are able to design a variety of classroom activities and adopt multiple teaching strategies through smart devices and information technology, thus students tend to get a higher level of self-efficacy, to be motivated and encouraged to participate more in the process of teaching and learning. According to Bandura's theory of social cognition, if learners wished to maintain a high level of learning engagement, they have a strong sense of self-efficacy in order to retain a decent level of motivation to commit themselves to learning activities, which was also confirmed by Dong's (2015) research. Students will gradually develop more committed attitude, actively communicate with teachers and classmates, pay attention to information comprehension and problem solving, and build creativity and cooperation abilities, resulting in more and more intense learning engagement, and will also further improve their own learning efficacy so as to create a virtuous circle. For high school students, full and active learning enthusiasm enables them to generate learning motivation, hone their perseverance, and improve their learning ability; the improvement of learning ability and the spirit of continuing to pay for learning enable students to obtain a good learning self-experience, and then go all out to devote themselves to learning.
In the blending course, students' self-efficacy positively affects teacher-student interaction, demonstrating that increasing students' self-efficacy can increase the frequency and degree of interaction between students and teacher. This finding is in line with findings of previous studies of Huang et al. (2015) and Dong (2015). According to self-efficacy theory, students with varying skill levels choose to perform the same learning activity in various ways and to varying degrees. The greater the student's sense of self-efficacy, the more likely they are to engage in a certain action. In the blending context, with various online and offline activities, students get more access to communicate with teachers, and when they feel they have abilities to complete a task, they will be eager to undertake difficulties and more willing to seek help from teachers. When they fail to reach their learning goals, they will increase their efforts and attribute their failures in favor of success. Thus, more teacher-student interaction will be seen under this circumstance, and with more and more communications between teachers and students, students could be more confident or less anxious to achieve success.
In the blending course, teacher-student interaction has a positive impact on the deep learning of English. It can be shown that increasing the frequency and degree of interaction between students and teachers will result in an improvement in students' English deep learning level, which are also found by Ma et al. (2011)'s investigation in a university classroom. In the blending courses, the traditional teacher-centered interaction is replaced by student- and knowledge-centered interaction—students are at the center of teaching and students interact with teacher on an equal basis thus forming a special partnership in order to achieve common goals.
The forms of interaction between students and teachers are varied through the blending courses teaching model which combines online learning with traditional classroom teaching. During the pre-class preparation stage, teachers use the intelligent software platform to assign a series of specific shallow learning tasks and upload pre-class learning materials, such as micro-videos of learning courses and exercises on relevant knowledge. The goal is to guide students in understanding and applying some basic English core knowledge, and then teachers use the teaching software platform to create personalized written reports for students and conduct diagnostic evaluations for students, while also realizing real-time learning supervision and management of each student via the smart learning platform. When they face difficulties, students may also communicate with teachers via the software learning platform and ask for teachers' feedback and clarification. The software learning platform captures each student's frequent difficulties. After summarizing, it is shown on the teacher's smart device terminal, allowing teachers to provide professional and thorough responses to students in the classroom. Teachers' professional expertise, as well as their support and responsiveness to students in various situational tasks, might enable students to receive timely assistance. Students can promote their own deep learning strategies and abilities to use rules, methods, skills, and so on to solve problems in the learning process, and also to master core knowledge content, develop critical thinking, problem-solving, cooperative communication, and other skills in the interaction with teachers. All these can contribute to students' deep learning.
Teacher-student interaction has a positive influence on students' learning engagement in the blending course. It was confirmed by Zhu (2010) who discovered that the interaction between teachers and students had an important impact on learning engagement. The interaction between teachers and students is to enhance the development of students' cognition and abilities. It may nurture students' abilities to recall, interpret, apply, analyze, synthesize, and evaluate through their interaction with teachers, and realize the shift from ignorance to knowledge so as to create a distinct cognition. Moreover, teacher-student interaction may influence the relationship between the two, as well as students' emotions in English learning, which in turn has an impact on students' dedication to learning. With more interactions between teachers and students, and when they get more feedback from teachers, students will be more willing to communicate, develop more interest in learning, and become more actively involved in learning activities.
Students' learning engagement has a positive influence on their English deep learning in the blending course. The behavior and cognition of students actively engaged in learning provide the foundation for English deep learning in the blending courses. As Johnson and Sinatra (2013) found, behavioral engagement was a prerequisite for deep learning, a key element influencing academic performance and results. Students who were highly engaged behaviorally and cognitively tended to gain support for meaningful learning outcomes. The finding of the present study also echoes the findings of Liu and Wang (2017) and Zhao et al. (2013), who claimed that behavioral engagement could lessen the negative effects of cognitive load on students, prevent shallow learning, enhance the development of students' critical thinking, collaborative and metacognitive abilities, and complete deep learning capacity training in a virtual reality context. Previous research has demonstrated that the learning engagement theory is congruent with the key principles of deep learning, and that learning behavior and cognitive engagement match the requirements of the deep learning mechanism.
This study investigated the factors that influence high school students' English deep learning in the blending course. We found that students' self-efficacy and engagement predicted and had strong positive influences on English deep learning. Self-efficacy had considerable beneficial influences on students' learning engagement and teacher-student interaction. Teacher-student interaction positively impacted English deep learning and students' learning engagement.
Drawn from these findings, we get some pedagogical implications for promoting EFL learners' deep learning in the blending courses. To increase students' English deep learning level in the blending courses, it is vital to strengthen students' self-efficacy and improve students' learning engagement. When design instructional activities, teachers could thoroughly analyze students' need and match the level of classroom tasks to students' existing learning capacity. The online platform can provide accurate data to analyze the needs and current level of students. Teachers can follow the change of students' learning process and better satisfy students' needs by using online sources. During the online learning phase, students can continue to accrue “successful experience” by performing acceptable minor tasks, and with constant repetition, steadily enhance their English learning, and eventually accomplish the aim of tackling greater learning tasks. The goal of blending teaching is not only to give information and resources, but also to develop students' abilities to learn and update knowledge and integrate resources on a continual basis. In the face-to-face teaching stage, teachers could engage students actively by carrying out various classroom tasks and providing care and support to students through encouragement and verbal persuasion. Students' perceptions of their own abilities are heavily impacted by the opinions of those around them. Teachers could assist students in developing a right vision of learning and personal values, as well as promote students' concentration on knowledge and ability development rather than just completing academic requirements.
Increasing the frequency and degree of interaction between students and teachers can promote the growth of students' English deep learning. It is suggested that teachers coordinate “dual-line” teaching activities (online learning and face-to-face classroom teaching) to enhance the interaction between teachers and students. Teachers could thoroughly examine the benefits of “dual-line” teaching activities in order to maximize interaction between teachers and students. Although different strategies are used to increase the occurrence of deep learning online and offline in blending courses, online and offline cannot be independent of one another, but should complement and coordinate with each other to form synergy in terms of learning content, learning tasks, learning process and evaluation.
The English curriculum layout of blending courses teaching model should take the dual channels of “shallow learning + deep learning” as the teaching purpose, which should run through all phases of English teaching. As for the pre-class stage, teachers could fairly plan a succession of specialized shallow learning activities, and establish a common learning community for teachers and students, where teachers and students can discuss and exchange learning experiences. Teachers can also initiate challenging learning tasks in the learning community to motivate students to participate, and students can complete learning tasks through cooperation and interaction with teachers. Then, through the student's individualized report and the diagnostic assessment provided by the learning platform, teachers could design the following tasks. During the face-to-face teaching stage, due to the individual counseling that cannot be completed by an online learning platform, teachers can complete it using face-to-face ways. Teachers could change teaching arrangements as needed in response to students' previews and reviews, create a variety of learning settings and possibilities, and provide prompt feedback. After class, teachers assign personalized learning courseware and activities to students, track the accomplishment of students' work in real time, give timely feedback to students, and encourage students to actively interact with teachers so that their deep learning can be improved.
Besides, it is suggested that we enrich evaluation methods and promote students' learning reflection. The assessment should evaluate students not just on their academic success, but also on their growth of diverse talents and emotional input. The inter-group assessment, peer assessment and self-assessment can be used integratively online and offline. Finally, improving IT support could benefit students' deep learning. The effective use of technology can transform teaching and learning practices, making them more important and interesting, while being able to connect more closely with students, which in turn can fundamentally change the quality of the student learning experience and enhance their deep learning (Malliarakis et al., 2014). The fast growth of information technology and smart devices has enabled the advent of the blending courses. Online learning platforms, which created a multitude of learning resources for students, can be tailored to meet the learning characteristics of students. The use of smart touch devices, virtual reality, intelligent technology, etc., provides students with a variety of learning avenues that allow them to interact with teachers and learning content at any time. Thus, frequent updating of online learning resources, maintenance of the learning platform, development of the campus network, and a variety of additional information technology support all play a significant part in students' deep learning.
This study focused on a single group English learners in a single school. Future studies could explore other influencing variables of deep learning of students with varied learning capacities in schools at different levels of education. Simultaneously, this study focused on quantitative data without qualitative insights and relied solely on self-reported data from a single source. Future researchers can employ interviews, classroom observations, and journals, to make more in-depth and complete research, and combine students' and teachers' perspective to have a holistic view of deep learning and underlying mechanisms of student interactions and learning processes.
The original contributions presented in the study are included in the article/supplementary material, further inquiries can be directed to the corresponding author.
The studies involving humans were approved by the China University of Petroleum. The studies were conducted in accordance with the local legislation and institutional requirements. The participants provided their written informed consent to participate in this study. Informed consent was obtained from the individual(s) for the publication of any potentially identifiable images or data included in this article.
HS: Conceptualization, Methodology, Project administration, Supervision, Writing—review & editing. PL: Conceptualization, Data curation, Investigation, Writing—original draft, Writing—review & editing.
The author(s) declare financial support was received for the research, authorship, and/or publication of this article. This work was supported by Science Foundation of China University of Petroleum, Beijing [grant number ZX20230108].
The authors declare that the research was conducted in the absence of any commercial or financial relationships that could be construed as a potential conflict of interest.
All claims expressed in this article are solely those of the authors and do not necessarily represent those of their affiliated organizations, or those of the publisher, the editors and the reviewers. Any product that may be evaluated in this article, or claim that may be made by its manufacturer, is not guaranteed or endorsed by the publisher.
Akyol, Z., and Garrison, D. R. (2011). Understanding cognitive presence in an online and blended community of inquiry: assessing outcomes and processes for deep approaches to learning. Br. J. Educ. Technol. 42, 233–250. doi: 10.1111/j.1467-8535.2009.01029.x
Allen, I. (2011). Going the distance: online education in the United States. Sloan Consortium 18:44. doi: 10.1111/1467-8659.1450275
Bandura, A. (1998). Perceived self-efficacy in cognitive development and functioning. Educ. Psychol. 28, 117–148. doi: 10.1207/s15326985ep2802_3
Bresó, E., Schaufeli, W. B., and Salanova, M. (2011). Can a self-efficacy-based intervention decrease burnout, increase engagement, and enhance performance? A quasi-experimental study. Higher Educ. 61, 339–355. doi: 10.1007/s10734-010-9334-6
Chouinard, R., Karsenti, T., and Roy, N. (2007). Relations among competence beliefs, utility value, achievement goals, and effort in mathematics. Br. J. Educ. Psychol. 77, 501–517. doi: 10.1348/000709906X133589
Delialioglu, O., and Yildirim, Z. (2007). Students' perceptions on effective dimensions of interactive learning in a blended learning environment. J. Educ. Technol. Soc. 10, 133–146.
Dong, X. (2015). A survey and research on the current status of self-efficacy in chemistry learning among high school seniors. Chem. Educ. 55–58.
Du, S., and Fu, Z. (2016). Blended learning and its empirical research based on MOOC. China Educ. Technol. 12, 129–133.
Fredricks, J. A., Blumenfeld, P. C., and Paris, A. H. (2004). School engagement: potential of the concept, state of the evidence. Rev. Educ. Res. 74, 59–109. doi: 10.3102/00346543074001059
Fullan, M., Quinn, J., and McEachen, J. (2018). Deep Learning: Engage the World, Change the World. Thousand Oaks, CA: Corwin Press.
Gao, J., Li, M. J., and Zhang, W. L. (2015). The relationship between proactive personality and online learning engagement: the perspective of self-determination motivation theory. e-Educ. Res. 36, 18–29.
Gao, Y., and Huang, R. H. (2017). Interpretation and implications of 2017 NMC technology outlook of higher education in China: A horizon project regional report. China Educ. Technol. 38, 15–22.
He, L., Tang, L., and Liu, J. (2019). Design and practice of MOOC blended teaching based on deep learning. Comput. Educ. 1, 150–157.
He, Z., and Huang, T. (2018). The construction concept, model, and application prospects of smart classrooms in universities: taking Central China Normal University as an example. Mod. Educ. Technol. 28, 54–60.
Huang, F., Wang, Q. H. X., and Peng, H. (2015). A survey of high school students' classroom participation and its influencing factors—Taking high school chemistry class as an example. Theor. Prac. Educ. 35, 55–57.
Johnson, M. L., and Sinatra, G. M. (2013). Use of task-value instructional inductions for facilitating engagement and conceptual change. Contemp. Educ. Psychol. 38, 51–63. doi: 10.1016/j.cedpsych.2012.09.003
Kim, D., Park, Y., Yoon, M., and Jo, I. H. (2016). Toward evidence-based learning analytics: using proxy variables to improve asynchronous online discussion environments. The Int. Higher Educ. 30, 30–43. doi: 10.1016/j.iheduc.2016.03.002
Lee, J., and Choi, H. (2017). What affects learner's higher-order thinking in technology-enhanced learning environments? the effects of learner factors. Comput. Educ. 115, 143–152. doi: 10.1016/j.compedu.2017.06.015
Liu, Z. Y., and Wang, Z. J. (2017). The empirical study of behavior engagement influence on deep learning: exemplified with video learning in virtual reality (VR) environment. J. Dist. Educ. 35, 72–81.
Ma, Z. Y., Meng, J. Z., and Yan, J. P. (2011). An empirical study on effects of classroom interaction on teaching quality: a case of a local university in Shanghai. Fudan Educ. Forum 2, 51–56.
Malliarakis, C., Satratzemi, M., and Xinogalos, S. (2014). Technology Integration in the Most Favorable Conditions: Findings From a Professional Development Training Program. New York, NY: Springer Publishing.
Nazarenko, A. L. (2015). Blended learning vs traditional learning: What works? (a case study research). Proc.- Soc. Behav. Sci. 200, 77–82. doi: 10.1016/j.sbspro.2015.08.018
Nilsen, H. (2009). Influence on student academic behavior through motivation, self-efficacy and value expectation: An action research project to improve learning. Issues Inf. Sci. Inf. Technol. 6, 545–556. doi: 10.28945/1080
Papinczak, T., Young, L., Groves, M., and Haynes, M. (2008). Effects of a metacognitive intervention on students' approaches to learning and self-efficacy in a first year medical course. Adv. Health Sci. Educ. Theor. Pract. 13, 213–232. doi: 10.1007/s10459-006-9036-0
Peng, F. X., and Yang, W. (2017). How to deepen the depth of learning in blended learning—How to supported learning analysis with education Big Data. Modern Dist. Educ. 2, 31−39.
Rimmkaufman, S. E., Baroody, A. E., Larsen, R., Curby, T. W., and Abry, T. (2015). To what extent do teacher-student interaction quality and student gender contribute to fifth graders' engagement in mathematics learning?. J. Educ. Psychol. 107, 170–185. doi: 10.1037/a0037252
Ronimus, M., Kujala, J., Tolvanen, A., and Lyytinen, H. (2014). Children's engagement during digital game-based learning of reading: the effects of time, rewards, and challenge. Comput. Educ. 71, 237–246. doi: 10.1016/j.compedu.2013.10.008
Schaufeli, W. B., Salanova, M., Gonzálezromá, V., and Bakker, A. B. (2002). The measurement of engagement and burnout: a two sample confirmatory factor analytic approach. J. Happ. Stu. 3, 71–92. doi: 10.1023/A:1015630930326
Shi, J., Tu, D., Wang, S., Lv, Z., and Zhao, L. (2011). Annual report of national college education survey 2009. Tsinghua J. Educ. 32, 9–23.
Tan, S. (2019). Construction of “blending teaching” model in colleges pointing to Deep Learning. China Higher Educ. 6, 51−53.
Tsai, C. C. (2012). The development of epistemic relativism versus social relativism vi a online peer assessment, and their relations with epistemological beliefs and internet self-efficacy. Educ. Technol. Soc. 15, 309–316.
Vayre, E., and Vonthron, A. M. (2017). Psychological engagement of students in distance and online learning: effects of self-efficacy and psychosocial processes. J. Educ. Comput. Res. 55, 197–218. doi: 10.1177/0735633116656849
Wang, C. K., Hu, Z. F., and Liu, Y. (2001). Evidences for reliability and validity of the Chinese version of general self-efficacy scale. Chin. J. Appl. Psychol. 7, 37–40.
Wang, H. B., Li, J. H., and Yang, X. M. (2017). Research on differences of learning behaviors between deep learners and surface learners in blend learning in higher Education. e-Educ. Res. 12, 44−50.
Yin, R., and Xu, D. (2011). Relationship between online learning environment and undergraduates' self-efficacy. Distance Educ. China 31:53.
Zhang, J. H. (2019). The current situation and effect of teacher-student interaction in college teaching. Res. Educ. Dev. 23, 10−17.
Zhang, Q. (2015). A study on the correlation between self-efficacy and deep learning among college students in the e-learning environment. E Educ. Res. 55–61.
Zhang, Q. L., and Wang, A. C. (2014). The design of new blended learning model based on flipped classroom. Modern Educ. Technol. 24, 27–32.
Zhang, Y. W., and Gan, Y. Q. (2005). The Chinese version of Utrecht work engagement scale: an examination of reliability and validity. Chin. J. Clin. Psychol. 3, 268–281.
Zhao, Z., Wang, X., and Song, W. (2013). A study on the level of deep learning among college students and related factors: analysis based on the survey of academic situation at Ocean University of China. Educ. Res. Exp. 73–77.
Zhu, H. (2010). The relationship between student engagement and college student achievement—the analysis of 2010 annual data set of Beijing college student survey. Tsinghua J. Educ. 31, 35–43.
Keywords: blending courses, deep learning, influencing factors, SEM, high school students
Citation: Shi H and Lan P (2024) Exploring the factors influencing high school students' deep learning of English in blended learning environments. Front. Educ. 9:1339623. doi: 10.3389/feduc.2024.1339623
Received: 16 November 2023; Accepted: 29 May 2024;
Published: 10 July 2024.
Edited by:
Beatriz Peña-Acuña, University of Huelva, SpainReviewed by:
Jamal Kaid Mohammad Ali, University of Bisha, Saudi ArabiaCopyright © 2024 Shi and Lan. This is an open-access article distributed under the terms of the Creative Commons Attribution License (CC BY). The use, distribution or reproduction in other forums is permitted, provided the original author(s) and the copyright owner(s) are credited and that the original publication in this journal is cited, in accordance with accepted academic practice. No use, distribution or reproduction is permitted which does not comply with these terms.
*Correspondence: Hong Shi, c2hpaG9uZzIwMDVzZEAxNjMuY29t
Disclaimer: All claims expressed in this article are solely those of the authors and do not necessarily represent those of their affiliated organizations, or those of the publisher, the editors and the reviewers. Any product that may be evaluated in this article or claim that may be made by its manufacturer is not guaranteed or endorsed by the publisher.
Research integrity at Frontiers
Learn more about the work of our research integrity team to safeguard the quality of each article we publish.