- Faculty of Education, Loei Rajabhat University, Loei, Thailand
Introduction: Self-regulated learning is an active process in which learners employ self-directed behaviors, thoughts, and actions to attain learning objectives. It is essential for students as it can result in improved academic achievement, task completion, and the acquisition of life skills. The objectives of this research were to examine the congruence between a causal model of self-regulated learning and empirical data and to investigate the direct and indirect effects of the model on a sample of 660 students from Loei Rajabhat University.
Methods: The data were analyzed using structural equation modeling. The results indicate that the causal model of self-regulated learning aligns with the empirical data.
Results: The study showed that self-efficacy, achievement motive, and learning by imitation variables had a favorable impact on self-regulated learning. The achievement motive and learning by imitation have indirect impacts on self-regulated learning through the self-efficacy variable. The findings suggest an increase in self-efficacy, achievement motive, and learning by imitation. Increasing self-efficacy, achievement motive, and learning by imitation among students may be an effective strategy for enhancing the efficiency of self-regulated learning.
Discussion: This research suggests that teachers should organize teaching and learning activities that promote achievement motivation and develop self-efficacy, and they should be good role models for students. All parties, including administrators, should implement policies that promote and develop activities to create incentives for achievement. Self-efficacy and learning by imitation are necessary for students to practice self-regulated learning in the future.
1 Introduction
In the Twelfth National Economic and Social Development Plan B.E. 2,560–2,564 (2017–2021), Strategy 1 on the potential promotion and development of human capital in Thailand sets the goal of people of all ages possessing skills, knowledge, and ability as a basis for national development through lifelong learning. This is because education is a mechanism for developing people’s quality of life so that they can live happily in society under the prevailing and rapidly advancing economic, environmental, social, and technological conditions. Moreover, most behavioral psychologists view the act of learning as being different from learning according to social cognitive theory, which focuses on the internal behavior of a person. For instance, the learning theory of Bandura (1986) assumes that people learn through observation, modeling, and reinforcement; this theory is rooted in social perception theory, focusing on social factors in human behavioral development.
The theory of self-regulated learning (SRL) focuses on the involvement of an individual learner in three processes: (i) Cognition is the learner’s mental process of perceiving, processing, and storing new data, involving aspects such as memory and problem-solving; (ii) metacognition is the learner’s perception and control of their strengths and weaknesses, ability to plan and monitor their learning, and ability to reflect on their own learning experience, involving aspects such as planning, examination, and evaluation; (iii) motivation is an internal and external factor affecting the learner’s involvement, effort expenditure, and persistence in learning, involving aspects such as goal-setting, self-efficacy, and self-determination (Zimmerman, 1990). SRL is not a static process but is instead constantly changing and dynamic and can be improved by training or instruction (Zimmerman, 2002). SRL is behavior that is based on the concepts of self-regulation and learning in social cognitive theory. Self-regulation is the process of a person using various strategies to regulate thoughts, behaviors, and emotions to learn different skills through their own motivation and actions (Schunk and Zimmerman, 1994). Self-regulation has three causes: person, environment, and behavior, all of which have interdependent effects that change with each factor (Zimmerman, 1989). The process of self-regulation involves three steps. (i) Self-observation helps a person to perceive their actions; this is important because successful self-regulation partly results from clear, regular, and accurate observation. Therefore, self-regulation enables a person to accurately diagnose conditions and express or adjust their behaviors. (ii) Self-judgment uses information obtained from self-observation, where comparisons are made with certain criteria before making a judgment to change one’s behavior. It affects behavioral change when the outcome is valuable enough and concerns one’s ability and actions. (iii) Self-reaction is a process that depends on each person’s decision benchmark and obtained outcome; for example, if a person achieves a specified standard, then they will reward themselves; in contrast, if they do something below a specified standard, then they usually react by punishing themselves. In addition, self-reaction can be either observable (e.g., working harder) or unobservable (e.g., a sense of pride). Therefore, SRL is an important behavior for learners in the 21st century because it can regulate and guide learners to continue studying outside of class to develop their knowledge, understanding, and skills. In an age with quick access to information without time and place limitations, learners can study using technological media (Jongjaisurathum et al., 2015). Learners without SRL usually face both academic and behavioral problems in school (Chianchana et al., 2010).
It is essential to study the background or causes of self-regulation; thus, the present study focuses on SRL by reviewing previous research that included variables that affect self-regulation. Many researchers have studied the relationship between different variables and SRL. For example, Aldridge and Rowntree (2022), Koshkouei et al. (2016), Milyavskaya and Werner (2018), and Zimmerman (2002) studied the relationship between achievement motive (MOT) and SRL, finding that MOT had a significant effect on SRL. Meanwhile, Azari Noughabi and Amirian (2021), Chung (2000), and Ha (2021) studied self-efficacy (SEE) and SRL, finding that SEE had a significant effect on SRL. Also, Bandura et al. (1961), Schunk and Hanson (1985), and Choeysuwan (2013) studied learning by imitation (LIM) and SRL, finding that LIM had a significant effect on SRL. Thus, these studies demonstrate that students who have a high level of MOT, SEE, and LIM will have a high level of self-regulation in their learning.
Against the above background, the present authors were interested in developing a causal model of SRL by students at Loei Rajabhat University (LRU) to obtain data to guide the development of student potential. It is hoped that teachers and administrators will use the model as a guideline for developing students’ SRL, leading to students’ professional development in their future occupations, as well as benefiting society and the country more broadly. The research objectives were as follows: (i) to examine the congruence between the model and empirical data; (ii) to investigate the direct and indirect impacts of achievement incentive, SEE, and LIM on SRL according to the model. We put forward the following research hypotheses:
H1: SEE has a significant effect on SRL.
H2: MOT has a significant effect on SRL.
H3: LIM has a significant effect on SRL.
H4: MOT has a significant indirect effect on SRL through the mediating variable of SEE.
H5: LIM has a significant indirect effect on SRL through the mediating variable of SEE.
2 Literature review
2.1 Self-regulated learning
The foundation of self-regulation is social cognitive theory, which offers multiple interpretations of self-regulation based on core beliefs. Bandura (1994) defined self-regulation as the influence an individual has over their own motivation, cognitive processes, emotional states, and behavioral patterns. Various scholars have extensively studied SRL during the past three decades. The consensus among most writers is that the process is cyclical, consisting of many stages and areas that partially overlap (Panadero, 2017). Examining previous research on SRL models, Panadero (Ibid.) highlighted a three-phase framework established by Puustinen and Pulkkinen (2001). The three fundamental stages found in nearly all models, however occasionally referred to by other names, are preparation, performance, and appraisal. Similar to the stages, various models can differ in the domains of SRL, typically identifying three or four phases. While certain aspects of SRL may occasionally need more focus, it is crucial to address all of them since they are significant and should not be overlooked. The OECD (2013) has recognized self-regulation as a crucial skill for the twenty-first century and a significant factor in achieving success. Developing self-regulation abilities in learning is essential since undergraduate students are required to have the ability to autonomously manage their academic objectives. Several studies have confirmed that to succeed and improve their performance in learning, students must develop self-regulation skills (Kizilcec et al., 2017; Greene et al., 2018). The current study builds upon previous research on self-regulated learning by developing a specific causal model for students at Loei Rajabhat University. This model offers new insights and practical implications for promoting self-regulated learning in this particular setting. Researchers have concluded that SRL refers to students’ self-regulation in class and extracurricular activities. It involves choosing problem-solving learning methods, planning studies to achieve good learning outcomes, and succeeding according to expectations or objectives. Social cognitive theory identifies three subprocesses of SRL (Bandura, 1986): (i) Self-Observation (SEO): If individuals do not observe their behavior, they have no control over their actions. Self-regulation requires awareness of actions. This process requires clarity, consistency, self-observation, precision, and self-recording. Bandura stressed the importance of self-observation factors such as actions, consistency, similarity, and precision. (ii) Self-Judgment (SEJ): If individuals cannot assess whether self-observation data meets their needs, it will have little impact on their behavior. Personal standards learned through direct instruction must be applied. Evaluating social reactions to behavior and observing model behavior help in the transmission of process standards. Another important factor in judgment is social, self, and group comparisons with the social reference group. (iii) Self-Reaction (SER): Setting assessment and decision-making standards in the previous step causes self-reaction.
2.2 Self-efficacy
Self-efficacy (SEE), as a psychological concept, relies heavily on Bandura’s (1977) social-cognitive theory as a crucial framework for comprehending SEE. SEE refers to an individual’s self-assessment of their capacity to complete a task or their confidence in their skills to do a task (Pintrich et al., 1991). Personal beliefs, judgments, and convictions influence SEE, making a single comprehensive exam an inaccurate measure (Bandura, 1977). The measurement of SEE depends on the specific environment and activities involved. Instead, it defers to SRL. Bandura (1997) and Schunk (1995) concur that SEE beliefs have an impact on effort, task selection, perseverance, resilience, and accomplishment. Contemporary comprehension of SEE encompasses metacognition and motivational processes (Zimmerman and Campillo, 2003; Zimmerman and Moylan, 2009). In addition, Winne (2011) explores the cognitive and metacognitive elements of SRL based on the Information Processing Theory. These elements include (i) task definition (comprehension of the task), (ii) goal setting and planning (establishing objectives and determining how to achieve them), (iii) implementing study tactics and strategies (executing a set of actions), and (iv) metacognitive adaptation of studying (making long-term changes in motivation, strategies, and beliefs) (Panadero, 2017). The models highlight SEE as a vital SRL process (Panadero, 2017). Eroglu and Ozbek (2018) regard SRL as one of the most significant individual talents of our time. Researchers have synthesized and categorized the components of SEE based on theories and research on SEE, designating them as follows: (i) Choice Behavior (CHO): decision-making about behavior and learning based on one’s abilities in specific situations; (ii) Effort Expenditure and Persistence (EEP): the determination and perseverance in learning to achieve success; and (iii) Thought and Emotion Reaction (TER): the cognitive processes and emotional responses that influence learning.
2.3 Achievement motive
The achievement motive refers to the desire to excel and to experience a sense of accomplishment when pursuing specific objectives, as well as experiencing anxiety in the face of failure (McClelland, 1961). Weiner (1972) explained how high-and low achievers differ. People with high achievement motives work harder, handle failures better, and enjoy challenges. They take the initiative, start many activities, and take more pride in their achievements than those with weak achievement motives. Achievement motive, sometimes referred to as achievement goals, have an important influence on SRL (Nurudin et al., 2023). Students who have mastery-and performance-approach objectives demonstrate more regulation effort in all aspects of SRL (Paz-Baruch and Hazema, 2023). This highlights the significance of these goals in influencing learning behaviors. Moreover, there is a notable impact of learning motivation on SRL, as evidenced by a positive connection between these two characteristics (Greisel et al., 2023). Essentially, SRL closely connects accomplishment goals and learning motivation, influencing students’ learning strategies and outcomes. Based on these findings, researchers have synthesized and streamlined the components of achievement motivation, identifying the following three important elements: (i) Energetic (ENE): works hard, whether mentally or physically, to feel important and have a sense of accomplishment. (ii) Individual Responsibility (IND): strives for personal fulfillment and success without praise; prefers intellectual and behavioral autonomy. (iii) Ambition (AMB): exhibits a strong desire to learn, exerts great effort to accomplish objectives regardless of how simple or difficult the tasks are, and strives for success. Individuals with strong achievement motives are intrinsically motivated, overcoming obstacles to accomplish their goals and achieve success.
2.4 Learning by imitation
Bandura (1986) defined learning by imitation as the process of observing, imitating, or emulating the behavior of others as a guide for one’s actions. Bandura (1977) suggested that environmental interactions cause behavior. In an ongoing process, learners imitate models by observing response patterns, diverse reactions, and environmental indicators. When observation is a factor, students can demonstrate novel behaviors or replicate model behaviors without making mistakes. As social development progresses, learners imitate those closest to them and grow beyond them. Imitators observe and remember the behaviors of esteemed models, but their motivation determines whether they emulate them. Imitation by learning entails instructing an agent to exhibit behavior by replicating expert demonstrations (Masaki, 2023; Parveen et al., 2023). SRL is a cognitive process in which learners actively manage and control their learning activities (Teng, 2023). While imitation through learning involves replicating actions, SRL stresses learners assuming responsibility for their learning process. Both approaches entail acquiring knowledge through the act of seeing and engaging in practical activities, although they vary in terms of the degree of independence and self-guidance. Imitation by learning relies on external supervision, whereas SRL enables individuals to autonomously control their learning. Gaining a comprehension of the relationship between imitation by learning and SRL can offer valuable insight into how individuals gain new abilities by integrating the process of learning through observation with tactics for self-regulation, ultimately leading to improved learning outcomes. Based on the theories and research on learning by imitation, researchers have synthesized, defined, and appropriately named the components of learning by imitation. There are two factors: peer imitation (PIM) and teacher imitation (TIM). Peer imitation refers to the process of observing, imitating, or emulating peers in various aspects, such as studying, playing, reviewing lessons, managing study time, pursuing additional knowledge, and engaging in activities. Teacher imitation is the process of observing, imitating, or emulating instructors in areas such as punctuality, work planning, effort, pursuing additional information, and participation in activities.
3 Methodology
3.1 Population and sample
This study investigates the students of Loei Rajabhat University in particular, given the substantial influence that the COVID-19 pandemic has had on the learning behaviors of students in virtual learning environments. Despite efforts to create conducive learning environments through online systems that facilitate efficient learning, online learning still impacts students. For instance, diminished academic performance and increased procrastination are consequences of perceived deficiencies in self-regulatory skills. We, as educators and researchers at Loei Rajabhat University, acknowledge the significance of identifying the variables that impact the self-regulation of learning among university students. Therefore, we conducted this study using students currently enrolled at Loei Rajabhat University. This survey research was a descriptive investigation of a causal model of SRL by LRU students. The study population comprised 12,279 undergraduate students from five faculties at LRU in the 2022 academic year, and the sample size was estimated using the LISREL software package. Schumacker and Lomax (2015) suggested the rule of thumb of using a sample size of 10–20 per estimated parameter; in the present study, the estimation involved 29 parameters, so the authors determined the sample size using the ratio of 20 participants per parameter and obtained a sample size of no fewer than 580 participants. Proportional stratified random sampling was then used to select undergraduate students at LRU in the 2022 academic year. Based on the sample size and the population in each stratum, the number of participants in each group was calculated proportionally. An extra 20% of participants were chosen from each faculty to make up for non-respondents who did not fill out the survey and to ensure sufficient data coverage for the analysis. Consequently, the final sample comprised 660 undergraduate students: 219 were male (33.20%) and 441 were female (66.80%). Within the sample, 173 respondents were from the Faculty of Education (26.20%), 142 were from the Faculty of Science and Technology (21.50%), 151 were from the Faculty of Humanities and Social Sciences (22.90%), 171 were from the Faculty of Management Science (25.90%), and 23 were from the Faculty of Industrial Technology (3.50%).
3.2 Research framework
Based on the SRL literature and a review of prior research that incorporated variables that influence self-regulation, the current study concentrates on SRL. Numerous researchers have investigated the correlation between SRL and various variables. They were then put together in a framework based on Bandura’s social cognitive learning to create the current causal model of SRL by LRU students. In the causal model, SEE, MOT, and LIM have direct effects on SRL, while MOT and LIM have indirect effects on SRL by transmission through SEE. The conceptual framework is shown schematically in Figure 1.
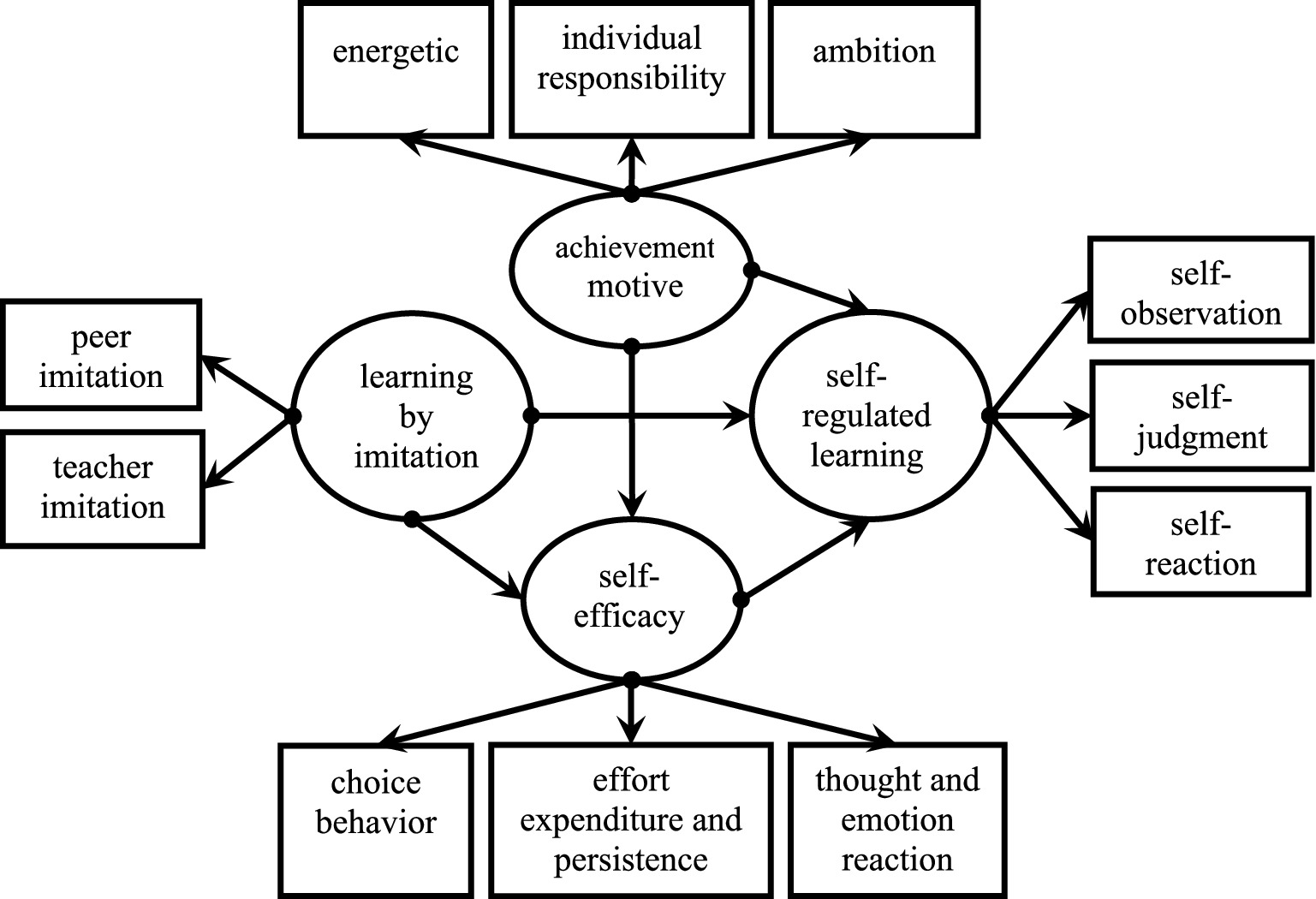
Figure 1. Conceptual framework of a causal model of self-regulated learning (SRL) by students at Loei Rajabhat University (LRU).
3.3 Instrument for data collection
The instrument for data collection was a three-part questionnaire about the SRL of students at LRU: Part 1 covered basic data on the respondents in terms of gender, educational level, and faculty; Part 2 covered SRL; and Part 3 covered the variables affecting SRL, i.e., SEE, MOT, and LIM. Five experts tested the questionnaire’s content validity using a five-level rating scale (1 = minimum, 5 = maximum). The results showed that all items had an item-level content validity index (CVI) higher than 0.78, and the scale-level CVI was 0.91, higher than the specified criterion of no less than 0.90 (Polit and Beck, 2012) The questionnaire contained 58 items in total: three on basic respondent data, such as gender, education level, and faculty; 15 on SRL in three aspects; 15 on SEE in three aspects; 15 on MOT in three aspects; and 10 on LIM in two aspects. After validation, the questionnaire was revised and improved before being tested on another group of 100 students similar to the sample, i.e., 20 students from each faculty. The results were used to examine the quality of the instrument by calculating the corrected item-total correlation (CITC) with Pearson’s correlation coefficient; the criterion for selection was a discrimination power of 0.20–1.00. The reliability was then calculated to find the Cronbach’s alpha coefficient; according to George and Mallery (2021), the reliability should be moderate (0.70) or higher. The questionnaire was classified according to each variable as follows:
3.3.1 SRL
This part of the questionnaire included 15 items with a discrimination power of 0.623–0.789 and a reliability value of 0.946. It contained three factors, each of which comprised five items: (i) SEO (e.g., A student always plans to study all subjects in advance. A student sets learning goals) with a discrimination power of 0.617–0.717 and a reliability value of 0.864; (ii) SEJ (e.g., A student can make his or her own study decisions. A student self-checks his or her study) with a discrimination power of 0.609–0.819 and a reliability value of 0.874; (iii) SER (e.g., A student is satisfied with academic results that meet his or her goals. A student can correct his or her learning deficiencies to achieve the goals) with a discrimination power of 0.790–0.844 and a reliability value of 0.913.
3.3.2 SEE
This part of the questionnaire included 15 items with a discrimination power of 0.530–0.751 and a reliability value of 0.925. It contained three factors, each of which comprised five items: (i) CHO (e.g., A student can choose learning activities on his or her own. A student believes that the learning activities chosen are suitable for his or her abilities) with a discrimination power of 0.580–0.809 and a reliability value of 0.884; (ii) EEP (e.g., A student can solve any problem that occurs while studying. If a student sees that learning is too complicated and confusing, he or she will never try to do it again) with a discrimination power of 0.429–0.750 and a reliability value of 0.799; (iii) TER (e.g., A student enjoys learning in class. A student feels proud when he or she achieves academic goals) with a discrimination power of 0.523–0.773 and a reliability value of 0.859.
3.3.3 MOT
This part of the questionnaire included 15 items with a discrimination power of 0.655–0.790 and a reliability value of 0.949. It contained three factors, each of which comprised five items: (i) ENE (e.g., A student will do their best on his or her assignments. A student will never abandon assignments and will do the best he or she can) with a discrimination power of 0.685–0.830 and a reliability value of 0.908; (ii) IND (e.g., When a student is given any assignments, he or she will complete them before the due date. A student is determined to work to achieve their goals) with a discrimination power of 0.850–0.891 and a reliability value of 0.951; (iii) AMB (e.g., A student wants to be a leader among his or her classmates. A student is likely to have higher academic expectations than his or her classmates, which will push him or her to compete with themselves and others) with a discrimination power of 0.566–0.933 and a reliability value of 0.924.
3.3.4 LIM
This part of the questionnaire included 10 items with a discrimination power of 0.528–0.787 and a reliability value of 0.911. It contained two factors, each of which comprised five items: (i) PIM (e.g., A student follows the learning practices of classmates who study hard. A student follows classmates who review lessons in their free time) with a discrimination power of 0.658–0.777 and a reliability value of 0.883; (ii) TIM (e.g., A student follows teachers in that he or she will not give up if not successful. A student follows teachers in researching knowledge from the library and the internet) with a discrimination power of 0.732–0.887 and a reliability value of 0.938.
3.4 Data analysis
The scores of the scale were examined against the criteria, with the analysis using the basic statistics of mean, standard error, standard deviation, minimum and maximum, normal distribution considering skewness and kurtosis, and the multicollinearity test. Pearson’s correlation coefficient was then used to test the congruence between the hypothesized model and empirical data, and the fitness index was used in the LISREL software package for the data analysis. The criterion values for the model fit coefficients were as follows: 0.05 < p ≤ 1.00; 0 < χ 2 /df ≤ 2; 0.95 ≤ GFI ≤ 1.00; 0.95 ≤ AGFI ≤1.00; 0 < RMSEA ≤0.05; 0 < SRMR ≤0.05; 0.95 ≤ NFI ≤ 1.00; 0.97 ≤ CFI ≤ 1.00; CN ≥ 200 (Diamantopoulos and Siguaw, 2000). In addition, the path coefficient was analyzed in the causal model to examine the direct effect (DE), indirect effect (IE), and total effect (TE) of different variables in the model to study the direct and indirect effects on SRL by LRU students.
4 Results
4.1 Descriptive statistical analysis of variables
The means (M) of the latent variables were between 3.484 and 3.795. The highest mean was for SEE (M = 3.795), followed by MOT, SRL, and LIM (M = 3.746, 3.672, and 3.484). The standard deviation (S) ranged from 0.647 to 0.733. Examining the coefficient of variation (CV) of the variables, it was determined that LIM variables had the greatest CV. The MOT variable had the greatest degree of data dispersion (CV = 21.032), the SRL variable was ranked second (CV = 17.904), and the SEE variable had the lowest distribution coefficient (CV = 17.058). Considering the skewness (Sk) of the variables, all variables were left-skewed. Since there was a statistically significant negative value at the 0.01 level, most students had a higher opinion level of the variable than the average. In terms of kurtosis (Ku), all variables had positive kurtosis with statistical significance at the 0.05 and 0.01 levels. The distribution characteristics of the variables were curved higher than normal (leptokurtic), showing that the students had a high degree of agglutination behavior. Pearson product–moment correlations between the SRL, SEE, MOT, and LIM variables ranged from small to moderate (0.276–0.653). These values (see Table 1) were lower than the criterion for concerns about multicollinearity (correlations between the independent variables < 0.80; Preacher and Hayes, 2004).
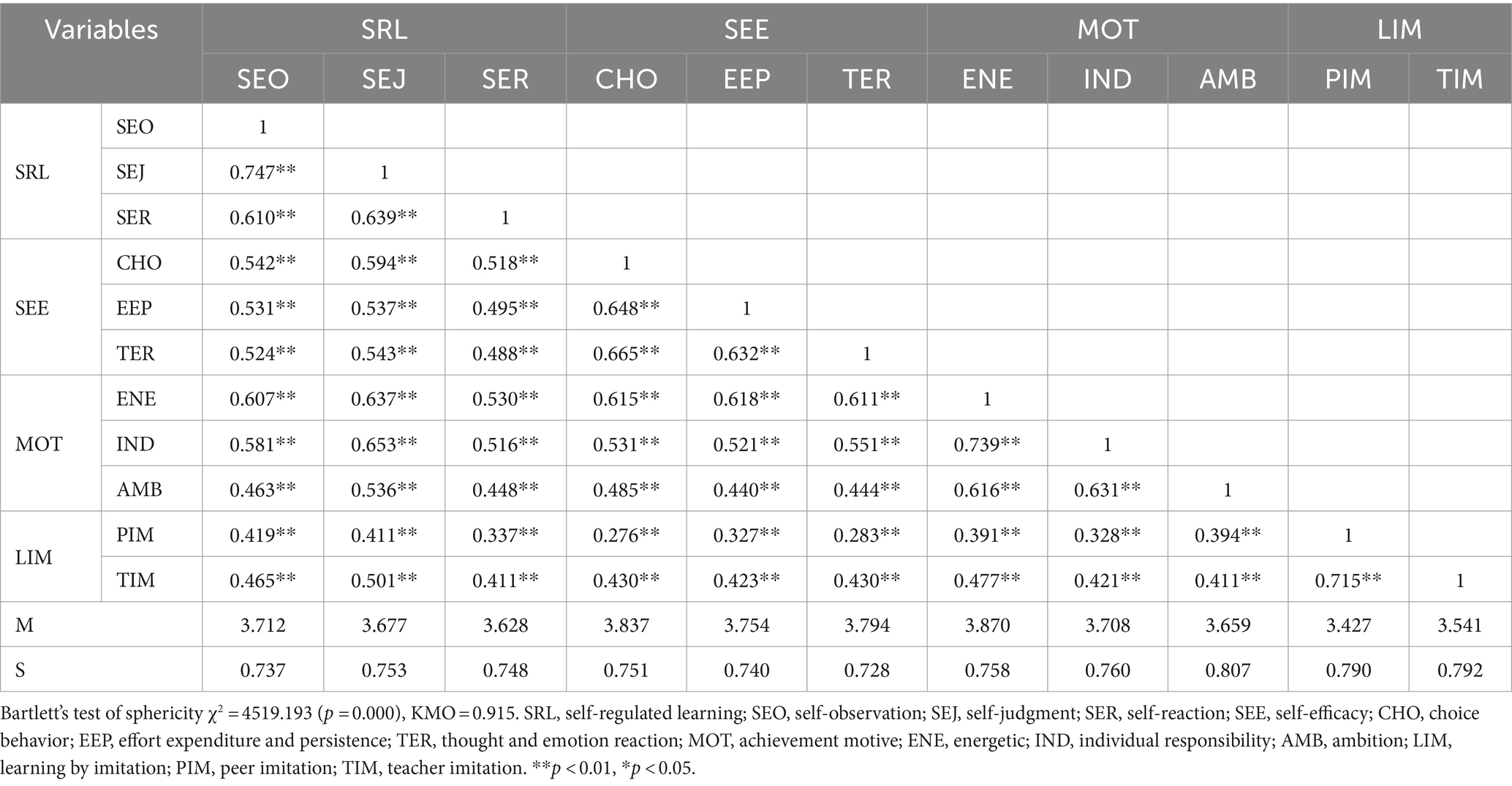
Table 1. Pearson correlation-coefficient matrix, mean, and standard deviation between variables observed in the research model.
4.2 Correlation coefficients between variables observed in the model
Pearson’s correlation coefficient analysis between all 52 pairs of observed variables found that none of the correlation coefficients was greater than 0.800. All variables were significantly correlated at the 0.01 level, with correlation coefficients ranging from 0.276 to 0.747. These results are given in Table 1.
4.3 Data analysis according to research objectives
Examination of the causal model of SRL by LRU students showed that the model was not congruent with the empirical data (χ2 = 107.615, df = 38, p = 0.000, GFI = 0.971, AGFI = 0.950, RMSEA = 0.053, SRMR = 0.024, NFI = 0.989, CFI = 0.993, CN = 359.801). The model was then adjusted by the researchers to be compatible with the empirical facts, taking into account its applicability and theoretical feasibility. The researchers changed the values in the THETA-DELTA (TD) and THETA-DELTA-EPS (TH) matrices as follows: TD (3,2) TD (4,3) TH (2,5) TH (4,1) TH (4,3) TH (2,1) TH (3,4). This was done one by one according to the program’s instructions until the model agreed with the empirical data and showed that the model was congruent with the empirical data (χ2 = 38.404, df = 31, p = 0.169, GFI = 0.990, AGFI = 0.978, RMSEA = 0.019, SRMR = 0.015, NFI = 0.996, CFI = 0.999, CN = 882.315). The reliability of the 11 observed variables ranged from 0.484 to 0.859, with TIM having the highest reliability (R2 = 0.859), ENE ranked second (R2 = 0.796), and AMB having the lowest reliability (R2 = 0.484). The values for SRL and SEE for the forecasting coefficient (R2) in the structural equation of the latent variables were 0.736 and 0.721, respectively. This means that LIM and MOT could explain 73.60 and 72.10% of the variance in SRL and SEE, as shown in Figure 2. The results for the sizes of the direct and indirect effects among the studied variables are described below.
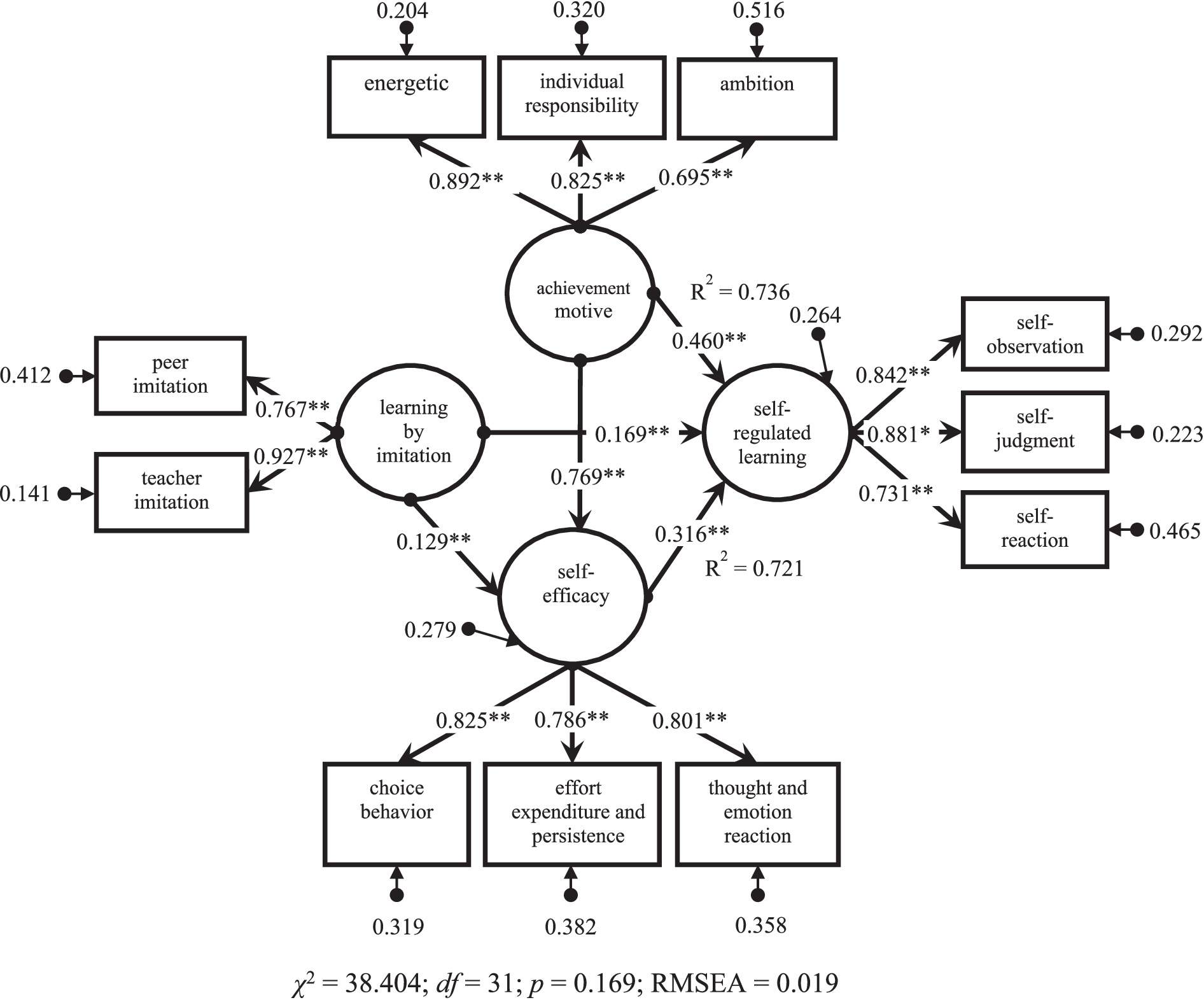
Figure 2. Parameter values in the SRL model with causal effects of self-efficacy (SEE), achievement motive (MOT), and learning by imitation (LIM). *p <0.05, **p < 0.01.
4.3.1 Self-efficacy (SEE)
Regarding the direct effects on SEE, the study found that MOT and LIM had direct effects on SEE at a statistical significance of 0.01. MOT had a positive effect on SEE with an effect value of 0.769, higher than the direct effect of LIM with a positive effect of 0.129. This indicates that students with high MOT and LIM also had high SEE.
4.3.2 Self-regulated learning (SRL)
Regarding the direct and indirect effects on SRL, the study found that SEE, MOT, and LIM had direct effects on SRL at a statistical significance of 0.01 with effect sizes of 0.316, 0.460, and 0.169, respectively, indicating that students with high SEE, MOT, and LIM had high SRL in terms of SEO, SEJ, and SER. Moreover, MOT also had an indirect effect on SRL at a statistical significance of 0.01 with an effect size of 0.243 by transmission through SEE, and LIM had an indirect effect on SRL at a statistical significance of 0.01 with an effect size of 0.041 by transmission through SEE. Comparing the direct and indirect effects on SRL, the direct effect of MOT (0.460) was higher than its indirect effect (0.243), and the direct effect of LIM (0.169) was higher than its indirect effect (0.041). These results are given in Table 2.
5 Discussion
The study results are discussed separately according to the study objectives below; the following two issues are of relevance.
5.1 Issue 1
Without adjustment, the causal model of self-regulated learning by LRU students was not congruent with the empirical data. Therefore, the authors modified the model by adjusting the error terms of the observed variables to partly correlate with one another so that the adjusted model was congruent with the empirical data. Furthermore, it aligns with the conceptual framework of this study. Researchers have also investigated the correlation between self-regulated learning and various variables. According to these studies, students with a high achievement motive, self-efficacy, and learning by imitation will demonstrate high self-regulation in their learning.
5.2 Issue 2
Apart from the congruence of the causal model of self-regulated learning by LRU students with the empirical data, some other interesting issues are discussed below.
(1) The research findings concerning the constituents of self-regulated learning indicated that the standardized component score weights for the observed variables of self-regulation learning varied between 0.731 and 0.881. Self-judgment (0.881) had the highest weighted standard deviation of any component, signifying that it assessed problem-solving in learning, decisive decision-making, and reasoned decision-making. This was followed closely by self-observation (0.842). This component encompasses the use of organized learning tools, effective time management for assignments and homework, strict adherence to school regulations, thorough task verification, and punctuality. Finally, self-reaction (0.731) comprises activities such as self-reward, self-reflection on performance, and error-related adjustments and corrections. These findings are consistent with those of Chianchana et al. (2010), and Jongjaisurathum et al. (2015). This indicates that educators should encourage self-judgment, self-reaction, and self-observation to foster self-regulated learning.
(2) According to energetic, individual responsibility, and ambition measurements, achievement motive (0.460) had the greatest direct impact on self-regulated learning for LRU students, and energetic had the highest weight of importance. Individual responsibility and ambition were the next two most important factors. These findings indicate that to achieve good achievement motive, energetic is the main factor that stimulates students to study diligently, work, and pay full attention to assignments. Despite dealing with hard work and demanding mental and physical effort, students attempt to accomplish tasks and obtain a sense of achievement. Compared to students with low achievement motive, those with high achievement motive pay better attention to studying and assignments, are more patient when faced with failures, prefer complex tasks, initiate different things from their ideas, and are proud to deal with difficult work, leading to an effect on self-regulated learning by LRU students. These findings are consistent with those of Aldridge and Rowntree (2022), Koshkouei et al. (2016), and Milyavskaya and Werner (2018), who found that achievement motive affected students’ self-regulated learning. Therefore, helping students possess achievement motive helps them perform self-regulated learning.
In addition, achievement motive had a positive direct effect on self-efficacy (0.769), which reflects the fact that students’ achievement motive affects their self-efficacy. This accords with Bjørnebekk et al. (2013), González Fernández et al. (2020), and Turner et al. (2021), who found that achievement motive affects self-efficacy.
Regarding indirect effects, achievement motive also had an indirect effect on self-regulated learning for LRU students through self-efficacy. This finding reflects the fact that to enhance students’ self-regulated learning, achievement motive alone is not enough, and self-efficacy is also needed.
(3) The next variable with a direct effect on self-regulated learning by LRU students was self-efficacy (0.316) as measured by choice behavior, effort expenditure, persistence, and thought and emotion reaction; the factor with the most weight of importance was choice behavior, followed by thought and emotion reaction, and then effort expenditure and persistence. These findings indicate that if students have a choice behavior, then they will decide to perform some behaviors and do some learning activities according to their ability in particular situations, demonstrating effort and persistence to learn successfully through the process of thought and emotion reaction. Consequently, these students obtain good self-efficacy, which has an effect on self-regulated learning by LRU students. These findings are consistent with those of An et al. (2021), Azari Noughabi and Amirian (2021), Ha (2021), Aldridge and Rowntree (2022), Lu et al. (2022), and Truong (2022), who noted that self-efficacy affects students’ self-regulated learning. Therefore, helping students to acquire self-efficacy helps them to apply self-regulated learning.
(4) The last variable with a direct effect on self-regulated learning by LRU students was learning by imitation (0.169), as measured by peer imitation and teacher imitation; the factor with the most weighted importance was teacher imitation, followed by peer imitation. Teacher imitation involves imitating teachers’ good practices such as punctuality, work planning, persistence, additional research, and activity participation, while peer imitation involves imitating friends in terms of study, play, lesson review, time management for study, knowledge-seeking, and activity participation. Such imitation enables students to achieve learning by imitation, resulting in self-regulated learning by LRU students. These findings are consistent with those of Bandura et al. (1961), Schunk and Hanson (1985), and Choeysuwan (2013), who noted that learning by imitation affects students’ self-regulated learning. Therefore, helping students learn through good imitation helps them apply self-regulated learning.
Moreover, learning by imitation also had a positive direct effect on self-efficacy (0.129), which reflects the fact that if students learn by good imitation, then their self-efficacy will also be positively affected.
In terms of indirect effects, learning by imitation had an indirect effect on self-regulated learning for LRU students through self-efficacy. This finding reflects the fact that to enhance students’ self-regulated learning, learning by imitation alone is not enough, and self-efficacy is also needed.
5.3 Research suggestions for implementation
There are two areas in which research recommendations can be made: (i) suggestions for using the research results, and (ii) suggestions for future research.
(i) Suggestions for using the research results.
According to the results on the causal model of self-regulated learning by LRU students, self-efficacy, achievement motive, and learning by imitation had direct effects on students’ self-regulated learning. The following are recommendations for teachers and administrators on how to use the research results:
Teachers should arrange learning activities and activities for developing desirable student characteristics both inside and outside classes to promote and develop students’ self-regulated learning. They should arrange activities for students to practice goal-setting, determining desirable behaviors, methods for behavior modification, and self-monitoring. Teachers should record the students’ performance to assess their ability to meet targets, improve weaknesses, and achieve goals. They should train students to use decision-making processes and self-assessment to assess if their behaviors align with the target; reward themselves if changes are in line with the target; or review outcomes to adjust their behavior if not. These activities would help train students to develop self-regulated learning according to its factors.
The researchers found that self-efficacy, achievement motive, and learning by imitation significantly influenced students’ self-regulated learning. Therefore, teachers should use teaching methods that enhance self-efficacy, such as activities to promote mastery experience, modeling, verbal persuasion, and emotional arousal. Moreover, teachers should promote students’ achievement motive, such as by showing admiration or giving a reward when students succeed in learning activities or other activities that require full effort to accomplish. Furthermore, the teaching methods used should promote students’ self-efficacy, resulting in better self-regulated learning.
Administrators should set policies for promoting the arrangement of activities and the development of self-regulated learning by using teaching methods that enhance students’ self-efficacy and achievement motive. Administrators should also train teachers to be good role models for students by participating in courses or projects.
(ii) Suggestions for future research.
Further investigation is warranted in the form of longitudinal studies that scrutinize the enduring impacts of self-regulated learning, achievement motive, self-efficacy, and learning by imitation. Studies should also examine the ramifications of cultural differences and the efficacy of technology-facilitated interventions. Researchers should investigate how peers and instructors impact achievement motivation and learning through imitation, as well as how interpersonal relationships and classroom dynamics influence these abilities. In addition, to promote achievement motive and self-efficacy among at-risk student populations, interventions should be customized. The purpose of these suggestions is to augment students’ self-regulated learning.
6 Conclusion
The causal model of self-regulated learning by LRU students matched actual evidence and self-efficacy achievement motive, and learning by imitation directly affected self-regulated learning. Transmission through self-efficacy, achievement motive, and learning by imitation indirectly affected self-regulated learning. The research guides teachers and administrators on how to support self-regulated learning. To encourage self-regulated learning, teachers should plan learning and character development activities within and outside the class. Students should practice goal-setting, identifying desired behaviors, behavior adjustment, and self-monitoring. Administrators should use educational strategies to improve students’ self-efficacy and achievement motive and promote self-regulated learning and activity organization. Administrators should also train teachers to be role models and integrate activities into courses, activities, and projects.
7 Limitations of the study
This study used a sample comprising only LRU students. Future studies should investigate developing the self-regulated learning of students at other universities to test whether the results of the present causal model of self-regulated learning are confirmed or confounded. Also, the present results should be examined further in future research by studying a learning activity package for developing students’ self-regulated learning in self-development and the development of knowledge and necessary skills, as well as students’ potential and learning achievement.
Data availability statement
The raw data supporting the conclusions of this article will be made available by the authors, without undue reservation.
Ethics statement
This study was approved by the Loei Rajabhat University Ethics Committee for Human Research based on the Declaration of Helsinki and the ICH Good Clinical Practice Guidelines (Approval No.386 HE 016/2563, certificated on August 28, 2020). Written informed consent for participation in the study was not required from the participants in accordance with the national legislation and institutional requirements.
Author contributions
AK: Conceptualization, Data curation, Formal analysis, Funding acquisition, Investigation, Methodology, Project administration, Resources, Software, Supervision, Validation, Visualization, Writing – original draft, Writing – review & editing. PK: Writing – review & editing. CP: Writing – review & editing. JS: Writing – review & editing. CI: Writing – review & editing.
Funding
The author(s) declare financial support was received for the research, authorship, and/or publication of this article. This article is supported by the Loei Rajabhat University Personnel Research Support Fund, administered by the Faculty of Education in 2019 (076/2562).
Acknowledgments
Thank you to Loei Rajabhat University for providing funding and grants in support of the publication of this research article.
Conflict of interest
The authors declare that the research was conducted in the absence of any commercial or financial relationships that could be construed as a potential conflict of interest.
Publisher’s note
All claims expressed in this article are solely those of the authors and do not necessarily represent those of their affiliated organizations, or those of the publisher, the editors and the reviewers. Any product that may be evaluated in this article, or claim that may be made by its manufacturer, is not guaranteed or endorsed by the publisher.
References
Aldridge, J. M., and Rowntree, K. (2022). Investigating relationships between learning environment perceptions, motivation and self-regulation for female science students in Abu Dhabi, United Arab Emirates. Res. Sci. Educ. 52, 1545–1564. doi: 10.1007/s11165-021-09998-2
An, Z., Wang, C., Li, S., Gan, Z., and Li, H. (2021). Technology-assisted self-regulated English language learning: associations with English language self-efficacy, English enjoyment, and learning outcomes. Front. Psychol. 11:558466. doi: 10.3389/fpsyg.2020.558466
Azari Noughabi, M., and Amirian, S. M. R. (2021). Assessing the contribution of autonomy and self-efficacy to EFL teachers’ self-regulation. Eng. Teach. Learn. 45, 71–88. doi: 10.1007/s42321-020-00060-4
Bandura, A. (1977). Self-efficacy: toward a unifying theory of behavioral change. Psychol. Rev. 84, 191–215. doi: 10.1037/0033-295X.84.2.191
Bandura, A. (1986). Social foundations of thought and action: A social cognitive theory. Ed. D. F. F Marks. The Health Psychology Reader. Englewood Cliffs, New Jersey: Prentice-Hall, Inc.
Bandura, A. (1994). “Social cognitive theory of mass communication” in Media effects: Advances in theory and research. Eds. J. Bryant and D. Zillmann (Hillsdale, New Jersey: Lawrence Erlbaum Associates Inc.), 61–90.
Bandura, A., Ross, D., and Ross, S. A. (1961). Transmission of aggression through imitation of aggressive models. J. Abnorm. Soc. Psychol. 63, 575–582. doi: 10.1037/h0045925
Bjørnebekk, G., Diseth, Å., and Ulriksen, R. (2013). Achievement motives, self-efficacy, achievement goals, and academic achievement at multiple stages of education: a longitudinal analysis. Psychol. Rep. 112, 771–787. doi: 10.2466/14.09.PR0.112.3.771-787
Chianchana, C., Wattananonsakul, S., and Aieamyeesoon, P. (2010). Development of indicators of student’s self-regulation: an application of the second-order confirmatory factor analysis model. Tech. Educ. J. King Mongkut’s Univ. Technol. North Bangkok 1, 1–10. Available at: http://ojs.kmutnb.ac.th/index.php/jote/article/view/2836/2166
Choeysuwan, C. (2013). Factors affecting the self-regulated learning of mathematics by students at schools in Aphiromruedi consortium under secondary educational service area office 9. Eds. S. Thirakanan and B. Phunthai [Master’s thesis] Bangkok, Thailand: Ramkhamhaeng University. Available at: https://digital.lib.ru.ac.th/m/B11851570/ChatchavalChoeysuwan.pdf.
Chung, M. K. (2000). The development of self-regulated learning. Asia Pac. Educ. Rev. 1, 55–66. doi: 10.1007/BF03026146
Diamantopoulos, A., and Siguaw, J. A. (2000). Introducing LISREL: a guide for the uninitiated. London: Sage. doi: 10.4135/9781849209359
Eroglu, M., and Ozbek, R. (2018). The investigation of the relationship between attitudes towards e-learning and self-directed learning with technology of secondary school students. Int. Online J. Educ. Sci. 10, 297–314. doi: 10.15345/iojes.2018.05.019
Evans, R. I. (1989). Albert Bandura: the man and his ideas—A dialogue. New York: Praeger Publishers. Available at: https://psycnet.apa.org/record/1989-98643-000.
George, D., and Mallery, P. (2021). IBM SPSS statistics 27 step by step: a simple guide and reference (17th). New York: Routledge. doi: 10.4324/9781003205333
González Fernández, F. T., Baena-Morales, S., Ramos-Pérez, D., and Morente-Oria, H. (2020). Motivation and self-efficacy influence in the academic performance of secondary students-athletes. J. Hum. Sport Exerc. 15, S1059–S 1070. doi: 10.14198/jhse.2020.15.proc4.08
Greene, J. A., Copeland, D. Z., Deekens, V. M., and Yu, S. B. (2018). Beyond knowledge: examining digital literacy’s role in the acquisition of understanding in science. Comput. Educ. 117, 141–159. doi: 10.1016/j.compedu.2017.10.003
Greisel, M., Melzner, N., Kollar, I., and Dresel, M. (2023). How are achievement goals associated with self-, co-, and socially shared regulation in collaborative learning? Educ. Psychol. 43, 384–402. doi: 10.1080/01443410.2023.2211751
Ha, N. T. D. (2021). An attempt to investigate the correlation between online self-regulation and self-efficacy in English learning. MEXTESOL J. 45, 1–10. Available at: https://www.mextesol.net/journal/public/files/06d6059abde93dc0d7ceb830f5f2368c.pdf
Jongjaisurathum, S., Supparerkchaisakul, N., and Damsuwan, W. (2015). Self-regulated learning strategies of 21st century. J. Behav. Sci. Dev. 7, 15–26. Available at: https://so02.tci-thaijo.org/index.php/JBSD/article/view/29915/25757
Kizilcec, R. F., Pérez-Sanagustín, M., and Maldonado, J. J. (2017). Self-regulated learning strategies predict learner behavior and goal attainment in massive open online courses. Comput. Educ. 104, 18–33. doi: 10.1016/j.compedu.2016.10.001
Koshkouei, H. J., Shahvarani, A., Behzadi, M. H., and Rostamy-Malkhalifeh, M. (2016). Structural modeling for influence of mathematics self-concept, motivation to learn mathematics and self-regulation learning on mathematics academic achievement. Math. Educ. Trends Res. 2016, 1–12. doi: 10.5899/2016/metr-00083
Lu, S., Cheng, L., and Chahine, S. (2022). Chinese university students’ conceptions of feedback and the relationships with self-regulated learning, self-efficacy, and English language achievement. Front. Psychol. 13:1047323. doi: 10.3389/fpsyg.2022.1047323
Masaki, F. (2023). Self-regulated learning from a cultural psychology perspective: shifting from strategy to process with the trajectory Equifinality approach. Hum. Arenas, 1–19. doi: 10.1007/s42087-023-00326-w
McClelland, D. C. (1961). “The achieving society” in University of Illinois at Urbana-Champaign's academy for entrepreneurial leadership historical research reference in entrepreneurship. Achieving society. New York: Free Press. Available at: https://books.google.co.th/books?hl=en&lr=&id=Rl2wZw9AFE4C&oi=fnd&pg=PA1&ots=NJKd-fwAI3&sig=E2YlzuKtXVyVV3H7oSRn7hVblpY&redir_esc=y#v=onepage&q&f=false
Milyavskaya, M., and Werner, K. M. (2018). Goal pursuit: current state of affairs and directions for future research. Can. Psychol. 59, 163–175. doi: 10.1037/cap0000147
Nurudin, S. M., Zain, Z. M., Yaacob, N. A., Kamarudin, N. A., Hassan, F., and Rahmat, N. H. (2023). Motivational belief, cognitive strategy use & self-regulation Alliance pertaining students learning achievement. Int. J. Acad. Res. Bus. Soc. Sci. 13, 172–189. doi: 10.6007/IJARBSS/v13-i7/17184
OECD. (2013) Skilled for life? Key findings from the survey of adult skills. ed. A. Gurría Paris: OECD. Available at: https://www.oecd.org/skills/piaac/SkillsOutlook_2013_ebook.pdf.
Panadero, E. (2017). A review of self-regulated learning: six models and four directions for research [review]. Front. Psychol. 8:422. doi: 10.3389/fpsyg.2017.00422
Parveen, A., Jan, S., Rasool, I., Waseem, R., and Bhat, R. A. (2023). “Self-regulated learning” in Handbook of research on redesigning teaching, learning, and assessment in the digital era. Ed. E. Meletiadou (Hershey, PA: IGI Global), 388–414. doi: 10.4018/978-1-6684-8292-6.ch020
Paz-Baruch, N., and Hazema, H. (2023). Self-regulated learning and motivation among gifted and high-achieving students in science, technology, engineering, and mathematics disciplines: examining differences between students from diverse socioeconomic levels. J. Educ. Gift. 46, 34–76. doi: 10.1177/01623532221143825
Pintrich, P. R., Smith, D. A. F., Garcia, T., and McKeachie, W. J. (1991). A manual for the use of the motivated strategies for learning questionnaire (MSLQ). Ed. J. S. Stark. Ann Arbor: National Center for Research to Improve Postsecondary Teaching and Learning, University of Michigan. Available at: https://files.eric.ed.gov/fulltext/ED338122.pdf.
Polit, D., and Beck, C. (2012). Data Collection in Quantitative Research. Nursing Research, Generating and Assessing Evidence for Nursing Practice, 9th Edition. Eds. D. Polit and C. Beck. Philadelphia: Wolters Kluwer Health/Lippincott Williams & Wilkins. 293–327.
Preacher, K. J., and Hayes, A. F. (2004). SPSS and SAS procedures for estimating indirect effects in simple mediation models. Behav. Res. Methods Instrum. Comput. 36, 717–731. doi: 10.3758/BF03206553
Puustinen, M., and Pulkkinen, L. (2001). Models of self-regulated learning: a review. Scand. J. Educ. Res. 45, 269–286. doi: 10.1080/00313830120074206
Schumacker, R. E., and Lomax, R. G. (2015). A Beginner’s guide to structural equation modeling. 4th ed. New York: Routledge. doi: 10.4324/9781315749105
Schunk, D. H. (1995). Self-efficacy, motivation, and performance. J. Appl. Sport Psychol. 7, 112–137. doi: 10.1080/10413209508406961
Schunk, D. H., and Hanson, A. R. (1985). Peer models: influence on children’s self-efficacy and achievement. J. Educ. Psychol. 77, 313–322. doi: 10.1037/0022-0663.77.3.313
Schunk, D. H., and Zimmerman, B. J. (1994). Self-Regulation in Education: Retrospect and Prospect. Self-Regulation of Learning and Performance. Issues and Educational Applications. Eds. D. H. Schunk and B. J. Zimmerman. Hillsdale: New Jersey, Erlbaum.
Teng, M. F. (2023). “10 self-regulation” in Cognitive individual differences in second language acquisition: Theories, Assessment and Pedagogy. (Berlin, Boston: De Gruyter Mouton), 201–222. doi: 10.1515/9781614514749-010
Truong, T. N. N. (2022). Psychometric properties of self-regulated learning strategies in learning English grammar and English grammar self-efficacy scales. Front. Educ. 7:801570. doi: 10.3389/feduc.2022.801570
Turner, J. E., Li, B., and Wei, M. (2021). Exploring effects of culture on students’ achievement motives and goals, self-efficacy, and willingness for public performances: the case of Chinese students’ speaking English in class. Learn. Individ. Differ. 85:101943. doi: 10.1016/j.lindif.2020.101943
Weiner, B. (1972). Attribution theory, achievement motivation, and the educational process. Rev. Educ. Res. 42, 203–215. doi: 10.3102/00346543042002203
Winne, P. H. (2011). “A cognitive and metacognitive analysis of self-regulated learning” in Handbook of self-regulation of learning and performance. Eds. B. J. Zimmerman and D. H. Schunk (New York, NY: Routledge), 15–32.
Zimmerman, B. J. (1989). A social cognitive view of self-regulated academic learning. J. Educ. Psychol. 81, 329–339. doi: 10.1037/0022-0663.81.3.329
Zimmerman, B. J. (1990). Self-regulated learning and academic achievement: An overview. Educ. Psychol. 25, 3–17. doi: 10.1207/s15326985ep2501_2
Zimmerman, B. J. (2002). Becoming a self-regulated learner: An overview. Theory Pract. 41, 64–70. doi: 10.1207/s15430421tip4102_2
Zimmerman, B. J., and Campillo, M. (2003). “Motivating self-regulated problem solvers” in The psychology of problem solving. Eds. J. E. Davidson and J. Sternberg (Cambridge: Cambridge University Press), 233–262.
Keywords: self-regulated learning, causal model, self-efficacy, achievement motive, learning by imitation
Citation: Kumyoung A, Kessung P, Pinasa C, Srijumnong J and Inyai C (2024) Development of a causal model of self-regulated learning by students at Loei Rajabhat University. Front. Educ. 9:1334995. doi: 10.3389/feduc.2024.1334995
Edited by:
Orna Heaysman, Achva Academic College, IsraelReviewed by:
Chaiwichit Chianchana, King Mongkut's University of Technology North Bangkok, ThailandAnela Nikcevic-Milkovic, University of Zadar, Croatia
Copyright © 2024 Kumyoung, Kessung, Pinasa, Srijumnong and Inyai. This is an open-access article distributed under the terms of the Creative Commons Attribution License (CC BY). The use, distribution or reproduction in other forums is permitted, provided the original author(s) and the copyright owner(s) are credited and that the original publication in this journal is cited, in accordance with accepted academic practice. No use, distribution or reproduction is permitted which does not comply with these terms.
*Correspondence: Anuphum Kumyoung, YW51cGh1bS5rdW1AbHJ1LmFjLnRo
†ORCID: Anuphum Kumyoung, https://orcid.org/0000-0003-2770-3737