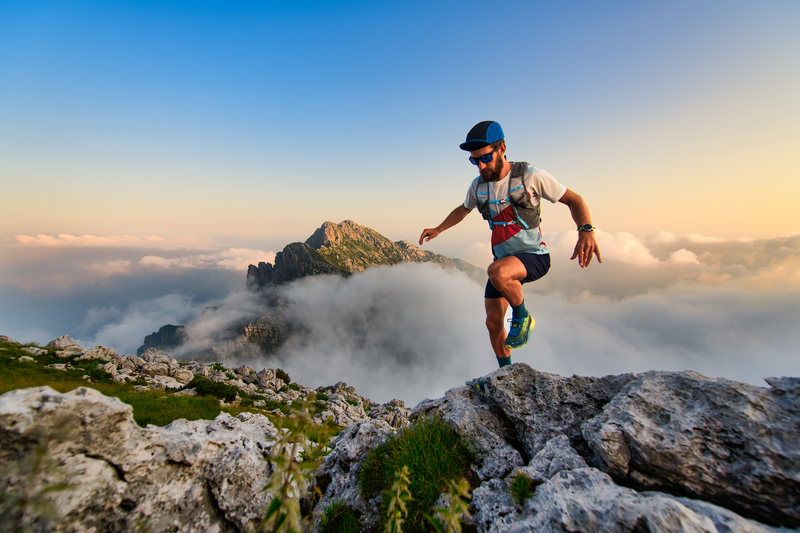
95% of researchers rate our articles as excellent or good
Learn more about the work of our research integrity team to safeguard the quality of each article we publish.
Find out more
ORIGINAL RESEARCH article
Front. Educ. , 12 April 2024
Sec. Educational Psychology
Volume 9 - 2024 | https://doi.org/10.3389/feduc.2024.1334034
Extra-mathematical knowledge is often overlooked when investigating mathematical skills. This study explores profiles of mathematical skills and associations with extra-mathematical knowledge and the understanding of complex sentences. The study involved 1,288 sixth-grade students (52.1% male) from 95 classes in 58 schools in Estonia. Students completed a math test as part of their regular lessons. The profiles of mathematical skills included students’ calculation skills, standard problems, and complex problems. Three distinct profiles of students emerged: students with high skill levels, students with average skill levels, and students with low skill levels. Students with high mathematical skills also had high extra-mathematical knowledge showing the crucial role of understanding the context of the math tasks in addition to having good mathematical skills.
Having good mathematical skills can help people to solve day-to-day problems, succeed in their careers, and contribute to modern society. The importance of mathematics has been recognized by many organizations [for a review, see Haara et al. (2017)], and countries have developed their own agendas about the best way to teach mathematics in school. Policymakers emphasize that approaches to teaching mathematics have to change according to the needs of the 21st century (The Council of European Union, 2018; OECD, 2019). Meanwhile, PISA 2022 assessments showed significant declines across OECD countries: Mathematics performance decreased by nearly 15 points compared to 2018—three times more than any prior decline (OECD, 2023a). Despite different countries wanting to offer the best teaching and learning methods, teachers find it difficult to follow the guidelines while students become frustrated with mathematics (Haara et al., 2017). Students are often taught how to solve typical mathematical problems in school, but when real-life situations are incorporated into word problems, students tend to ignore them (Reusser and Stebler, 1997). Although focusing too much on applying procedures to solve the mathematical textbook problem leaves students less time to acquire a mathematical skill students could use in everyday life, many teachers still follow this approach (Boesen et al., 2014). Difficulties in mathematics can lead to a loss of interest in studying (Gottfried et al., 2007). However, students with good mathematical skills tend to acquire a better economic status as adults (Ritchie and Bates, 2013); moreover, improving people’s skills can lead to enormous economic gains (OECD, 2010).
Thus far, the skills and knowledge needed to solve word problems have often been described as mathematics specific (e.g., knowing mathematical concepts, understanding how to reason with these concepts; Lindquist et al., 2017; Niss and Højgaard, 2019). In the current study we apply latent profile analysis (LPA) to investigate students’ skill profiles. LPA is a person-oriented classification technique that classifies people into homogeneous latent profiles based on their similarities in observed variables (Woo et al., 2018). In contrast to variable-oriented techniques that examine relationships among observed variables, LPA looks for patterns in responses across multiple variables to identify unobserved subgroups or profiles within a population (Bergman and Andersson, 2010). As a result, the sample is not analyzed as a single group, but as distinct subgroups with unique responses that may exhibit different patterns of association with a variety of covariates that can then be analyzed (Lanza et al., 2013; Howard and Hoffman, 2018). A further strength of a person-oriented approach is the ability to examine potential differences among groups without using arbitrary cut-offs (Psyridou et al., 2023). Thus, LPA can provide more precise cut-off points for the division of samples into groups than variable-oriented methods. The person-oriented approach has recently gained popularity and has been used to explore patterns of students’ motivation in mathematics (Pongsakdi et al., 2019) or explore the developmental profiles of mathematical skills from grades 1 to 9 (Psyridou et al., 2023). Several studies have found that students can have distinct mathematical skill profiles (e.g., Mädamürk et al., 2016, 2018; Kainulainen et al., 2017).
In addition, mathematical tasks (e.g., mathematical word problems) often include other topics or contexts that are not exclusively mathematics-related (e.g., map reading); to solve such problems, students need to understand these contexts as well. The extra-mathematical knowledge does not have to be mathematics-specific knowledge, but is rather a type of knowledge that benefits students by enabling them to use mathematics in a real-life situation (see Niss, 1994). In the PISA mathematics assessment, students are not only expected to demonstrate mathematical knowledge, but also tackle real-world problems (OECD, 2023a). Beginning at level 2, students should be able to use mathematics in simple real-life situations, while students from level 4 onwards should be able to demonstrate the ability to integrate equations and formulas directly into aspects of real life (OECD, 2023b). In Estonia, 85% of students achieved at least level 2 and 34% achieved level 4 or above on the PISA 2022 test (OECD, 2023a; Tire et al., 2023). In addition to students’ extra-mathematical knowledge, we measure understanding of complex sentences because a rather complicated representational process lies behind mathematical components, which allows the solver to interpret the text of the problem (Kintsch and Greeno, 1985). We aim to carry out preliminary research to investigate whether student math-specific skill profiles will differ regarding the extra-mathematical knowledge and understanding of complex sentences.
Mathematics provides a set of tools for describing, analyzing, and predicting systems in the real world (Verschaffel and De Corte, 1997). Mathematical skills cannot be limited to use in a specific subject matter area students learn at school; it also has to include elements to help individuals cope outside school in modern society (Haara et al., 2017; Niss and Højgaard, 2019; OECD, 2019). The type of mathematical skills students develop is often related to particular types of problems they practice (Reusser and Stebler, 1997). Using mathematics in everyday life requires students to be active problem solvers who, first, get information and formulate the problem; second, employ different strategies to solve questions; and third, interpret, reason, and communicate the results (Lindquist et al., 2017; Niss and Højgaard, 2019; OECD, 2019). In addition, it is not possible to formulate, employ, or interpret mathematically if a student does not possess the necessary knowledge and skills underlying the problem-solving process (OECD, 2019). Conceptual understanding and procedural skills are the two key domains of the knowledge underpinning mathematical problem-solving processes (Rittle-Johnson et al., 2001). Both mathematical concepts and procedures are closely related and usually develop together (Rittle-Johnson, 2017). In order to attain better mathematical skills, one must possess both knowledge of mathematical concepts as well as knowledge and skills of mathematical procedures (Niss and Højgaard, 2019).
In light of the fact that a person’s psyche is shaped by their environment (Vygotsky, 1978; Bronfenbrenner, 1979; Bronfenbrenner and Morris, 2006; Toomela, 2020), mathematical concepts and procedures can only serve as a part of an individual’s problem-solving arsenal, and it is imperative that individuals possess real-world knowledge of the situation at hand (Verschaffel and De Corte, 1997). By understanding mathematical concepts and procedures, as well as real-world knowledge outside of mathematics, an individual is better equipped to achieve their goals and develop their abilities to participate in society (Toomela et al., 2020). Accordingly, in the current study students’ mathematical skills as well as their extra-mathematical knowledge are investigated to better understand the interplay between subject-specific skills and grasping the real-life context.
In order to develop calculation skills, one must be aware of calculation rules and strategies and be able to retrieve arithmetic facts (i.e., procedural skills; Rittle-Johnson et al., 2001) and understand number concepts (i.e., conceptual knowledge; Rittle-Johnson et al., 2001). In elementary school, strong calculation skills include the ability to add, subtract, multiply, and divide natural numbers, which primarily requires procedural knowledge and skills (i.e., counting strategy and fact retrieval; Koponen et al., 2018). Calculation skills form the bedrock for more challenging mathematical skills like solving word problems (Fuchs et al., 2012).
Mathematical word problems have been developed to serve as models for everyday life, enabling students to learn to use mathematics in real-life situations (Verschaffel et al., 2020), and are often used to measure mathematical skills (Crooks and Alibali, 2014). Mathematical word problem-solving can be viewed as a process. First, students need to read and understand the problem situation described (Kintsch and Greeno, 1985). Based on their understanding, they have to construct a mathematical model that describes the essence of those situational elements and relationships of the elements. Third, they have to solve the model to identify its unknown element(s) as well as interpret and evaluate the outcome (and if it meets the terms of the practical situation that was the basis of the mathematical model). Finally, they must communicate the results (Verschaffel and De Corte, 1997).
School textbooks often include word problems in which students learn to solve tasks mechanically, without understanding the essence behind them, or word problems constructed in a way that guides students toward the correct procedure (Reusser, 1988; Verschaffel et al., 2010). As a result, students might develop proficiency in procedural knowledge, but not necessarily substantial understanding of the mathematical problems (e.g., Mädamürk et al., 2016, 2018). For example, students can learn a step-by-step procedure for solving standard arithmetic computations, but when faced with a new type of problem, they might not be able to apply a previously learned procedure or they might extrapolate it inappropriately (Rittle-Johnson et al., 2001; Niss and Højgaard, 2019). The solution to standard word problems generally requires both conceptual and procedural knowledge—in other words, a representational side based on conceptual knowledge and an algorithmic side based on procedural knowledge (Scheibling-Sève et al., 2020). Still, students who have mastered the ability to solve standard mathematical tasks are not necessarily able to correctly solve the more complex word problems that include real-word components (Verschaffel et al., 2020). For example, Verschaffel et al. (1994) presented 10- to 11-year-old students with standard word problems they were able to solve using learned procedures (S-problems) and more realistic complex word-problems (P-problems) that required them to consider the real-life context. Many students who solved the standard problems correctly ignored real-life logic when solving the complex problems.
The complex word problems that simulate real-life situations are often the most difficult to solve (Verschaffel et al., 2020). When creating a mathematical model of the problem situation, in addition to requiring a substantive understanding of the concepts and procedures as well as relationships to other concepts and procedures in a domain (i.e., learners can recognize, recall, group, derive information from text or tables, and use it to compute and measure; Rittle-Johnson et al., 2001; Crooks and Alibali, 2014; Lindquist et al., 2017; Niss and Højgaard, 2019), there is also a need to take the realistic problem modeling perspective into account (Verschaffel et al., 1994; Verschaffel and De Corte, 1997).
The current study includes calculation tasks, standard problems, and complex problems to better understand the interplay among different mathematical skills. As previously shown (Mädamürk et al., 2016), students can have quite good calculation skills but lower word problem-solving skills. Accordingly, a person-oriented approach is employed to investigate whether students can have contrasting levels of distinct mathematical skills.
The mathematical concepts and procedures are just one piece in problem solvers’ repertoire as they should also possess real-world knowledge about the problem situation (Reusser and Stebler, 1997). Verschaffel and De Corte (1997) explained that studies should (a) not simply assume that students possess the relevant knowledge of real-world situations, but also explicitly measure their mastery of these concepts, and (b) analyze the extent to which students are able to apply their mathematical and real-world knowledge in an integrated manner when modeling and solving word problems. According to Bronfenbrenner (1979), the environment has several parts, including physical space and things as well as people in different roles and activities through which to interact with the environment. Thus, when students want to buy something from a shop (physical space and things), they are in a specific role (shopper) and need to interact with the environment (activities), which requires knowledge about the environment. For example, students can have knowledge about how to calculate money but, when they do not know how the shop operates (i.e., extra-mathematical knowledge), they can encounter difficulties despite their good mathematical skills. Alternatively, when they do not know the rules of the board games (Siegler and Ramani, 2008), how to use a ruler (MacDonald and Lowrie, 2011), or how to use cell phones (McMullen et al., 2019; McMullen and Siegler, 2020), they can have difficulties learning number concepts or spontaneously focusing on mathematical relationships. Although this understanding is in line with many mathematical competence definitions that state that a person possesses proficiency in mathematical competence when able to use mathematics in everyday life (Haara et al., 2017; Niss and Højgaard, 2019; OECD, 2019; Toomela et al., 2020) and many researchers have directed our attention to how using mathematics outside the classroom is a valuable skill (Gainsburg, 2008; MacDonald and Lowrie, 2011; Reinke and Casto, 2020), in reality many students (Reusser and Stebler, 1997; Verschaffel and De Corte, 1997; Altay et al., 2017; Krawitz et al., 2018) and teachers (Lee, 2012) make superficial connections between mathematics and real life and tend to neglect the real-world knowledge when solving mathematical problems. Accordingly, in the current study we aim to pilot tasks assessing extra-mathematical knowledge and how it is related to math-specific skills.
The difficulty of word problems is heavily influenced by factors related to text comprehension (Cummins et al., 1988; Björn et al., 2016) and, accordingly, text comprehension and mathematical skills have shown positive correlations from kindergarten to elementary school (Vilenius-Tuohimaa et al., 2008; Watts et al., 2014), middle school, and college (Singer and Strasser, 2017; Powell et al., 2019). Text comprehension skills are more important when solving complex word problems than when doing calculations (Kikas et al., 2019).
In general, text comprehension can be divided into two categories. First, lower-level processes translate the written code into meaningful language units (Kendeou et al., 2014). During this step, the text must first be stored in working memory in order to be processed; the concepts are then constructed and organized according to prior knowledge, structure, and importance (micro structures; Kintsch and Mangalath, 2011). Second, higher-level processes combine these units into a coherent mental representation (macro structures; Kendeou et al., 2014). During this step, a given meaning, order, macro structures, and links to the context and situation model are created (Sulak and Güneş, 2017). The successful solution of reality-based word problems can be specifically challenging as building a suitable situation model is particularly relevant for the comprehension of such tasks [see Verschaffel et al. (2020)]. Situation complexity (open questions, with a lot of overdetermination, and no hints to structure the situation versus clear questions with clear situations and relevant information) as well as linguistic complexity can have an impact on word problem-solving (Leiss et al., 2019). Other studies have shown how rewriting problems can have a significant impact on student performance and sense-making as well as engagement with the problems (Palm, 2008; Kirkland and McNeil, 2021).
Connections between different mathematical skills have been extensively studied (Rittle-Johnson et al., 2001; Rittle-Johnson, 2017; Niss and Højgaard, 2019; Hurst and Hurrell, 2020), and the importance of extra-mathematical context has been emphasized in a number of studies (Niss, 1994; Verschaffel and De Corte, 1997; Palm, 2008; Leiss et al., 2019; Verschaffel et al., 2020; Kirkland and McNeil, 2021). Still, studies on how extra-mathematical knowledge is associated with different mathematical skills are scarce. The current study aimed to conduct preliminary research to determine if mathematical skills profiles of sixth-grade students are related to different levels of extra-mathematical knowledge and the understanding of complex sentences.
Specifically, we first investigated different profiles emerging from sixth-grade students’ calculation skills, standard problem-solving skills, and complex problem-solving skills. As different mathematical skills develop together (Rittle-Johnson et al., 2001; Rittle-Johnson, 2017; Niss and Højgaard, 2019) while students tend to ignore the real-life context when building a mental situation model of the problem (Verschaffel et al., 1994; Verschaffel and De Corte, 1997), we expected to find (H1a) a low skill group that exhibits limited understanding of calculation problems and standard problems and, thus, is only very poorly able to solve complex problems and (H1b) a high skill group that exhibits high mathematical skills and, thus, is better able to solve the calculation problems and standard problems correctly.
Second, we investigated whether these profiles show different levels of extra-mathematical knowledge and the understanding of complex sentences. Concerning extra-mathematical knowledge, we hypothesized (H2a) that the high skill group will exhibit higher levels of extra-mathematical knowledge than the low skill group because the low skills group might ignore the real-life context of the presented problem more (Verschaffel et al., 1994; Verschaffel and De Corte, 1997). Furthermore, as text comprehension plays an important part in solving word problems (Cummins et al., 1988), we expect (H2b) the high skill group to possess the highest skills in understanding complex sentences compared to other profile groups.
The data were collected as part of a larger project of the Estonian Ministry of Education and Research aiming to develop a mathematical competence assessment tool for students in Grades 3 and 6. The current study used part of the Grade 6 test and sample. All schools throughout Estonia were informed about the project; those schools that agreed to participate received instructions regarding the testing procedure and access to the tests. In total, 1,288 children in Grade 6 (mean age = 154.46 months, SD = 4.36; 52.1% male) from 95 classes in 58 schools participated in the study. Students were tested during regular lessons in the 2019 fall semester. It took one academic lesson for students to complete the mathematical skills test and another lesson to complete the language test. Students completed the online tests under the guidance of their teachers.
In Estonia, where the study took place, all comprehensive schools have to follow the National Curriculum for Basic Schools (Eesti Vabariigi Valitsus, 2011/2022), which also presents the requirements for mathematics education. Thus, we considered the National Curriculum requirements while composing the mathematics tasks. Accordingly, by the end of sixth grade, students should be able to perform calculations involving natural numbers (up to the billions), integers, positive rational numbers, and ordinary fractions; be familiar with the general scheme of problem solving and be able to justify its reason as well as verify its correctness; know the concept of percentage and be able to find part of the whole; know and be able to convert units of length, area, volume, and time; and be able to calculate the area and volume of basic geometric shapes (Eesti Vabariigi Valitsus, 2011/2022).
Calculation skills were assessed using calculation tasks and unit comparison tasks. In the calculation tasks, students had to perform simple calculations of adding, subtracting, multiplying, and dividing up to 1,000. Most calculation tasks were similar to the calculations students were required to perform in order to solve the standard on complex problems tasks. In the unit comparison tasks, students had to convert and compare units of (a) length and mass and (b) time. The units were in different scales (gram, kilogram, millimeter, centimeter, decimeter, meter, minute, hour). Students had to choose among three options—smaller than, greater than, or equal to—to make the comparison correct. Students received one point for each correct calculation and comparison, up to a maximum of 20 points. The CFA model had a good fit [χ2 (168, N = 1,288) = 326.51, p < 0.01; CFI = 0.97; TLI = 0.96; RMSEA = 0.027; SRMR = 0.08]. The internal reliability was good (Cronbach’s α = 0.87).
Word problem-solving skills were assessed with standard and complex word problems. Standard problems were assessed using three tasks. In two standard problems, which were word problems, the questions guided the solution process. As part of the task, after reading the word problem (e.g., “Children have to read a 120-page book. One third of the pages are covered with images. The deadline for reading the book is in 4 days. Martha has not read any pages. She can read 25 pages of text each day”), students were required to answer three consecutive questions to solve it: (1) How many pages of text are in the book? (2) How many pages can Martha read in 4 days? (3) Will she finish the book on time? As these questions hinted at the creation of the correct situational model, students should have been able to solve these problems once they gained a solid understanding of the mathematical concepts, as the calculation task for the solution was relatively straightforward (120/3 = 40, 120–40 = 80; 4 × 25 = 100; 100 > 80). In the third task, students had to choose the smallest perimeter rectangle from four rectangles and then the biggest perimeter rectangle from another four rectangles. The measurements of the rectangles were in meters, centimeters, and millimeters. Students received one point for each question answered correctly, up to a maximum of 7 points for the standard problem-solving tasks. The CFA model had a good fit [χ2 (13, N = 1,288) = 45.61, p < 0.01; CFI = 0.99; TLI = 0.98; RMSEA = 0.044; SRMR = 0.05]. The internal reliability was good (Cronbach’s α = 0.77).
Complex word problems were assessed using six word problem-solving tasks that represent everyday situations students might experience. Students had to either choose the correct answers from among predefined options or submit the correct answer themselves. To solve the tasks, students needed to add, subtract, multiply, and divide using positive whole numbers up to 100 and have knowledge about units of length, mass, and time (e.g., “Dad bought 5 wooden bars to repair the fence, each 1.2 m long. How many one-meter tall wooden bars did he get from the purchased bars?”; we counted only five as the correct answer). For some tasks, more than one question was asked; for others, only one question was asked. The score was calculated by adding the points awarded for each correct answer. Students could receive a maximum 15 points for the complex word problem-solving tasks. The CFA model had a good fit [χ2 (88, N = 1,288) = 408.23, p < 0.01; CFI = 0.97; TLI = 0.96; RMSEA = 0.053; SRMR = 0.09]. The internal reliability was good (Cronbach’s α = 0.80).
Extra-mathematical knowledge was measured using four questions, where students could answer how much they knew about the field to which the mathematical tasks were related (e.g., one of the complex word problems included buying amusement park tickets; thus, the question was: “What kind of tickets can be bought at the amusement park?” Answer options included: men’s tickets, tickets with a fixed time limit, children’s tickets, family tickets, I do not know). We counted tickets with a fixed time limit, children’s tickets, and family tickets as the correct answers. One point was given for each correct answer; another point was given if they did not choose the wrong answer. Students could get a maximum of 16 points.
The understanding of complex sentences was assessed with a task that contained long sentences describing space, time, and amount relationships. The task consisted of one example exercise and 10 tasks. The student was shown a sentence, followed by four to six pictures on the screen. Students had to select one picture that matched the corresponding sentence (e.g., in the medium-height vase there are three more flowers than in the tallest vase, but two times less flowers than in the shortest vase). Students received one point for each correct answer, up to a maximum 10 points for the task. The CFA model had a good fit [χ2 (35, N = 1,265) = 39.38, p = 0.28; CFI = 0.99; TLI = 0.99; RMSEA = 0.010; SRMR = 0.04]. The internal reliability of the understanding complex sentences task was good (Cronbach’s α = 0.75).
Data were analyzed using the statistical package SPSS 29 and Mplus 8.3 (Muthén and Muthén, 2012–2017). To confirm the calculations, standard problems, complex problems, and how the understanding of complex sentences forms one-dimensional factors, confirmatory factor analyses (CFA) were conducted. In addition to the model test statistic (chi-square with degrees of freedom and p-value), multiple fit indices of χ2, CFI, TLI, RMSEA, and SRMR were used as indicators of the model fitting the data (Hu and Bentler, 1999). A non-significant chi-square indicated good fit. The cut-off criteria for accepting a model for CFI and TLI were equal to or greater than 0.95, for RMSEA they were equal to or less than 0.06, and for SRMR they were less than 0.08 (Hu and Bentler, 1999).
To identify the profiles of students based on the responses of multiple continuous indicators (calculation skills; standard and complex problem-solving skills), a latent profile analysis (LPA) was used. An LPA is a person-oriented method; in contrast to variable-centered approaches, it classifies individuals in a given group in accordance with some construct that cannot be directly measured (Lanza et al., 2013; Howard and Hoffman, 2018; Woo et al., 2018). This identification of latent profiles can serve as a useful tool for characterizing qualitative differences among learners (Hickendorff et al., 2018). A maximum likelihood with robust standard errors (MLR) estimation was used to classify students with similar performances according to the measures. Mixture complex analyses were used to adjust the standard errors and fit statistics for clustering (students were clustered into classrooms). Standardized scores of mathematical skills tasks were used in the LPA. To choose the optimal number of profiles, we combined the statistical evidence provided by the Akaike information criterion (AIC), Bayesian information criterion (BIC), adjusted BIC (aBIC), entropy, and Vuong–Lo–Mendell–Rubin likelihood ratio test (VLMR; Lanza et al., 2013). In terms of AIC, BIC, and aBIC, a lower value suggests a more favorable fit. Using the VLMR, the estimated model is compared to a model with one profiles less than the estimated model. The non-significant p-value supports the one profiles less model (Woo et al., 2018). An entropy is a measure of classification accuracy that ranges from 0 to 1, with a higher value (i.e., above 0.8 and closer to 1) indicating greater accuracy. In addition, it may not be feasible to conduct the study in profiles with less than 5% of cases (Marsh et al., 2009; Lanza et al., 2013). To find the best fitting model, we started testing with two profiles; when the fit statistics were not acceptable, additional profiles were added, as it was assumed that additional subprofiles existed in the population (Nylund et al., 2007). In this way, we were able to determine and describe the optimal number of subpopulations in the sample necessary to produce an accurate picture of the sample’s people (Lanza et al., 2013). For the additional fit criteria, we analyzed the classification probabilities for the most likely latent profile membership in LPA. The classification probabilities indicated the likelihood that each individual in the sample belonged to a particular latent profile based on their observed responses to the measured variables. In addition to these fit indicators, we looked at the theoretical background to select the optimal number of profiles. In order to conduct an additional statistical validation of our choice of three profile models, the statistical package SPSS 29 was used to randomly divide the sample in half. The LPA was repeated for half of the sample (668 students) using Mplus 8.3 (Muthén and Muthén, 2012–2017).
To test whether the students with distinct profiles have different levels of extra-mathematical knowledge and the understanding of complex sentences, the Bolck, Croon, and Hagenaars (BCH) method (Asparouhov and Muthén, 2021) was used.
There was minor missingness in the data: four cases due to technical problems during the testing in the variables “Calculation” and “Extra-mathematical knowledge” (mathematical skills test) as well as 29 cases in the variable “Understanding complex sentences” (language test that was taken separately from mathematical skills and some students did not take it). The overall data coverage was 97.8–100%. Schafer (1999) recommended that, with minor missingness (5%), data imputations provide marginal benefit. Still, we used maximum likelihood with a robust standard error (MLR) estimator in the LPA models to handle missingness with non-normal data (Muthén and Asparouhov, 2002).
The descriptive statistics and bivariate correlations for all the measures used in the study are presented in Table 1. Calculation problems, standard and complex problems, extra-mathematical knowledge, and understanding of complex sentences were all correlated with each other at the p < 0.01 level.
The results of the LPA fit indicators are presented in Table 2. LPA was conducted with three variables (i.e., calculation problems, standard problems, and complex problems). According to the statistical evidence provided by fit indicators and the theoretical background, we chose the three latent profiles as the best fitting model because, (a) although the AIC, BIC, and aBIC indicators continued to decline with four and five profiles, this decrease was lower than the drop from two to three profiles; (b) with three profiles, the smallest profile accounted for 11.2%, with four profiles, the smallest profile accounted for only 4% and, with five profiles, only 2% of the total sample size; and although the entropy values were greater than 0.70 for all models, the VLMR was significant with three profiles but not with four or five profiles; for a more complex model to be preferred, the VLMR likelihood ratio test should be significant (Woo et al., 2018); (c) the classification probabilities in the three-profile model (Table 3) showed strong fit (0.84–0.99) compared to 0.66–0.91 with the four-profile model; according to Nylund et al. (2007), an item probability above 0.85 corresponds to a high probability of fit; and (d) the three profiles that emerged from the LPA are in line with the theoretical underpinnings—namely, different mathematical skills develop in parallel (Rittle-Johnson et al., 2001; Rittle-Johnson, 2017; Niss and Højgaard, 2019), with calculation and word problem-solving skills being closely related (Rittle-Johnson et al., 2001; Rittle-Johnson and Star, 2007; Rittle-Johnson, 2017; Powell et al., 2019). Students in low skill profiles are characterized by weak calculation and word problem-solving skills, which makes them less capable of solving complex word problems. The average skill profile possesses average calculation skills and word problem-solving skills, meaning they can solve some of the complex word problems. The high profile possesses good calculation and word problem-solving skills; in addition, they are able to comprehend complex word problems, which requires a combination of mathematical skills as well as real-life knowledge (Verschaffel et al., 1994; Verschaffel and De Corte, 1997). With the four-profile model, there were still low and high skill profiles, but the two middle profiles were very similar and did not provide additional theoretical explanations.
In order to conduct an additional statistical validation of our choice of profiles, the statistical package SPSS 29 was used to randomly divide the sample into half. The LPA was repeated for half of the sample (668 students) using Mplus 8.3 (Muthén and Muthén, 2012–2017). The results of the LPA fit indicators for the random sample are presented in Table 4. The statistical evidence provided by fit indicators and the theoretical background supported the three-profile model once more. Although the AIC, BIC, and aBIC indicators continued to decline with four and five profiles, with the four-profile model, the smallest profile accounted for only 1.2% of the total sample size. Once again, the VLMR was not significant with four or five profiles.
The means of the three profiles are presented in Table 5. The standardized results are presented in Figure 1. The results presented in Table 5 show that the mean difference among the three profiles was significant in terms of complex problems [F (2, 1,285) = 365.82, p < 0.001, η2 = 0.36], standard problems [F (2, 1,285) = 1607.00, p < 0.001, η2 = 0.71], and calculation problems [F (2, 1,281) = 792.10, p < 0.001, η2 = 0.55]. The three latent profiles were labeled “low skill” (accounting for 11% of the sample and characterized by low skills in calculation, standard, and complex problems), “average skill” (accounting for 38% of the sample and characterized by average levels for all assessed skills), and “high skill” (accounting for 51% of the sample and characterized by high levels for all assessed skills).
We tested the equality of means of different auxiliary variables expected to be associated across latent profiles (Table 6). We used the BCH method (Asparouhov and Muthén, 2021), which reduces the shift in latent profiles when auxiliary variables are included. The BCH results show that the profiles were associated with different means across extra-mathematical knowledge: (χ2 = 43.46, p < 0.001). The low skill profile had lower-than-average means in extra-mathematical knowledge, compared to average means in the average skill profile and higher-than-average means in the high skill profile. Regarding complex sentences, the high skill profile tended to have a statistically significantly higher understanding of complex sentences compared to both average and low skill profiles (χ2 = 311.94, p < 0.001).
The current study aimed to understand how the extra-mathematical knowledge and understanding of complex sentences are associated with different skill profiles among sixth-grade students. The profiles were based on calculation skills, standard problem-solving, and complex word problem-solving skills. Three distinguished profiles of students emerged: high skill, average skill, and low skill profiles. Students in the high skill profile tended to have better extra-mathematical knowledge and understanding of complex sentences compared to those in other profiles. The results provided further evidence regarding the importance of knowledge about real-life situations and text comprehension in gaining mathematical skills.
Three profiles of mathematical skills emerged. The high skill profile constituted the largest segment of the sample, encompassing 51% of participants. In concordance with the hypothesis (H1b) the high skill group exhibited exceptional proficiency in calculation skills and high standard and complex problem-solving skills. The average skill profile (38%) showed average calculation and, accordingly, average standard and complex problem-solving skills. The low skill profile (H1a; 11%) included students who tended to have poorer performance in all skills. The high, average, and low skill profiles show the strong connection among distinct mathematical skills (Rittle-Johnson et al., 2001; Rittle-Johnson and Star, 2007; Rittle-Johnson, 2017; Powell et al., 2019). Although previous person-oriented studies have also indicated that at least some students can have, for example, high calculation skills but low word problem-solving skills (e.g., Mädamürk et al., 2016, 2018), this was not the case in the current study. One reason for similar findings in the high, average, and low skill groups could be because of the strong connections between the tasks: Calculation tasks included the same calculations necessary for solving both standard and complex problems. Accordingly, students struggled to solve simple calculations also had problems solving standard and complex problems.
Concerning extra-mathematical knowledge, differences emerged in the levels across all profiles: The low skill group tended to have less extra-mathematical knowledge than the other groups, the average skill group tended to have average extra-mathematical knowledge, and the high skill group (H2a) tended to have the highest extra-mathematical knowledge. This result indicates that there can be considerable variability among students in these everyday experiences (e.g., shopping and cooking), and we cannot assume that all or most students have good knowledge in even quite simple everyday contexts that are often present in mathematical tasks. On the other hand, the correlation between extra-mathematical knowledge and problem-solving skills was quite low. Thus, there were also students who had good extra-mathematical knowledge but low skills. We can assume that at least some students solve word problems by performing arithmetic operations on the given numbers without considering the context (Verschaffel et al., 2010), even if they are familiar with the context. In addition, this type of connection between extra-mathematical knowledge and mathematical skills might not occur without specific directed interventions (e.g., Verschaffel and De Corte, 1997; Siegler and Ramani, 2008; McMullen et al., 2019). Moreover, the extra-mathematical knowledge with which students need to be familiar in order to solve the task in real life can be very different. Students can use their bank cards instead of cash to pay at the store, and they might not need to calculate whether they can afford a product or which product is more cost-effective. In the future, when they have to make buying decisions with many options available and the stakes are high, this could become a more pressing issue. Students’ ability to make purchase decisions in real life is enhanced when they know that different stores sell similar products for different prices. There are also online retailers. Furthermore, a different color on the product’s price sticker can indicate that the product is on sale at a discount, although not always. Stores offer special discounts when products are sold cheaper, and many products are also available on the second-hand market. All these components can be incorporated into mathematics tasks to enhance students’ real-life word problem-solving skills.
On the other hand, real-life problems presented in textbooks still include abstract or ambiguous language, and students need help to better understand these complex sentences. As shown in the current study, students in the high skill level group (H2b) also tended to have a high level of understanding of complex sentences. When students lack conceptual knowledge and have difficulties understanding the text, it can be very difficult for them to even form the correct visual and mathematical representations of the task (Rittle-Johnson et al., 2001).
The results of the current study are in line with several previous studies that show strong connections among different mathematical skills, such as calculation and word problem-solving skills (Rittle-Johnson et al., 2001; Rittle-Johnson and Star, 2007; Rittle-Johnson, 2017; Powell et al., 2019). In addition, in order to solve complex word problems, students need not only good mathematical skills but also extra-mathematical knowledge (Niss, 1994; Verschaffel et al., 1994; Verschaffel and De Corte, 1997) as well as good reading comprehension (Jõgi et al., 2015; Björn et al., 2016).
The current study also has important limitations to consider when interpreting the results. The results are correlative and cannot explain the causal relationships, meaning other reasons may explain why a student is not able to solve mathematical tasks. The components that could inhibit the acquisition of better mathematical skills include, for example, a lack of attention, working memory problems (Fuchs et al., 2019), a lack of motivation (Gottfried et al., 2007; Mädamürk et al., 2018; Verschaffel et al., 2020), poor reading ability (Watts et al., 2014; Singer and Strasser, 2017), slow processing speed, and mathematical anxiety (Powell et al., 2019). Thus, such variables should be considered in future studies investigating the role of extra-mathematical knowledge in solving mathematical tasks. In addition, the concepts and measures of extra-mathematical knowledge used in this study are preliminary and do not involve all the possible aspects of the fields used in mathematical tasks. Thus, these concepts and measures should be developed further.
The consensus is that mathematics is an important skill in everyday life, so the teaching of mathematics has to change according to 21st-century needs (Ritchie and Bates, 2013; The Council of European Union, 2018; OECD, 2019). The purpose of the present study is to provide insights into the significance of possessing extra-mathematical knowledge when solving mathematical problems.
Based on the results, more effective teaching methods and more accurate interventions can be developed. The results indicated that students can be divided into at least three profile groups; thus, interventions can be developed based on the assumption that there are students with distinct skill profiles in classroom. Furthermore, in order to learn how to solve complex word problems, students should be provided with mathematical problems that encourage them to utilize their everyday knowledge and experience in order to solve them. It would be possible, for example, to solve a problem related to shopping in conjunction with a discussion about a topic outside of mathematics, such as how students typically shop in stores, what they pay attention to, and what their options are.
Thus far, most studies that have examined the use of mathematical knowledge outside the classroom have targeted younger children (Daucourt et al., 2021), but the knowledge about the field considered in the mathematical tasks might become more important at higher education levels or in workplaces (Novak et al., 2007). Therefore, more research on using mathematics in a specific field and general out-of-school contexts should include higher education students and adults.
The raw data supporting the conclusions of this article will be made available by the authors, without undue reservation.
Ethical approval was not required for the study involving human samples in accordance with the local legislation and institutional requirements. Written informed consent for participation in this study was provided by the participants’ legal guardians/next of kin.
HS: Conceptualization, Data curation, Formal analysis, Investigation, Methodology, Validation, Writing – original draft. KM: Conceptualization, Data curation, Formal analysis, Funding acquisition, Investigation, Methodology, Project administration, Validation, Writing – original draft, Writing – review & editing.
The author(s) declare financial support was received for the research, authorship, and/or publication of this article. This study was supported by the research funding TRU17155A and TRU19115A of the Estonian Ministry of Education and Research, and by the Estonian Research Council grant PSG741.
The authors declare that the research was conducted in the absence of any commercial or financial relationships that could be construed as a potential conflict of interest.
All claims expressed in this article are solely those of the authors and do not necessarily represent those of their affiliated organizations, or those of the publisher, the editors and the reviewers. Any product that may be evaluated in this article, or claim that may be made by its manufacturer, is not guaranteed or endorsed by the publisher.
Altay, M. K., Yalvac, B., and Yeltekin, E. (2017). 8th grade Student’s skill of connecting mathematics to real life. J. Educ. Train. Stud. 5, 158–166. doi: 10.11114/jets.v5i10.2614
Asparouhov, T., and Muthén, B. (2021). Auxiliary variables in mixture Modeling: Using the BCH method in Mplus to estimate a distal outcome model and an arbitrary secondary model. Struct. Equ. Model. 21. Available at: https://www.statmodel.com/examples/webnotes/webnote21.pdf
Bergman, L. R., and Andersson, H. (2010). The person and the variable in developmental psychology. J. Psychol. 218, 155–165. doi: 10.1027/0044-3409/a000025
Björn, P. M., Aunola, K., and Nurmi, J. E. (2016). Primary school text comprehension predicts mathematical word problem-solving skills in secondary school. Educ. Psychol. 36, 362–377. doi: 10.1080/01443410.2014.992392
Boesen, J., Helenius, O., Bergqvist, E., Bergqvist, T., Lithner, J., Palm, T., et al. (2014). Developing mathematical competence: from the intended to the enacted curriculum. J. Math. Behav. 33, 72–87. doi: 10.1016/j.jmathb.2013.10.001
Bronfenbrenner, U. (1979). The ecology of human development: Experiments in nature and design. Cambridge, MA: Harvard University Press.
Bronfenbrenner, U., and Morris, P. A. (2006). “The bioecological model of human development” in Handbook of child psychology: theoretical models of human development. eds. R. M. Lerner and W. Damon (New York, NY: John Wiley & Sons Inc), 793–828.
Crooks, N. M., and Alibali, M. W. (2014). Defining and measuring conceptual knowledge in mathematics. Dev. Rev. 34, 344–377. doi: 10.1016/j.dr.2014.10.001
Cummins, D. D., Kintsch, W., Reusser, K., and Weimer, R. (1988). The role of understanding in solving word problems. Cogn. Psychol. 20, 405–438. doi: 10.1016/0010-0285(88)90011-4
Daucourt, M. C., Napoli, A. R., Quinn, J. M., Wood, S. G., and Hart, S. A. (2021). The home math environment and math achievement: a meta-analysis. Psychol. Bull. 147, 565–596. doi: 10.1037/bul0000330
Eesti Vabariigi Valitsus . (2011/2022). Põhikooli riiklik õppekava. [National curriculum for Basic schools], Riigi teataja RT I, 2022, 10. Available at: https://www.riigiteataja.ee/akt/112042022010
Fuchs, L. S., Compton, D. L., Fuchs, D., Powell, S. R., Schumacher, R. F., Hamlett, C. L., et al. (2012). Contributions of domain-general cognitive resources and different forms of arithmetic development to pre-algebraic knowledge. Dev. Psychol. 48, 1315–1326. doi: 10.1037/a0027475
Fuchs, L., Fuchs, D., Seethaler, P. M., and Barnes, M. A. (2019). Addressing the role of working memory in mathematical word-problem solving when designing intervention for struggling learners. ZDM Math. Educ. 52, 87–96. doi: 10.1007/s11858-019-01070-8
Gainsburg, J. (2008). Real-world connections in secondary mathematics teaching. J. Math. Teach. Educ. 11, 199–219. doi: 10.1007/s10857-007-9070-8
Gottfried, A. E., Marcoulides, G. A., Gottfried, A. W., Oliver, P. H., and Guerin, D. W. (2007). Multivariate latent change modeling of developmental decline in academic intrinsic math motivation and achievement: childhood through adolescence. Int. J. Behav. Dev. 31, 317–327. doi: 10.1177/0165025407077752
Haara, F. O., Bolstad, O. H., and Jenssen, E. S. (2017). Research on mathematical literacy in schools - aim, approach and attention. Eur. J. Sci. Math. Educ. 5, 285–313. doi: 10.30935/scimath/9512
Hickendorff, M., Edelsbrunner, P. A., McMullen, J., Schneider, M., and Trezise, K. (2018). Informative tools for characterizing individual differences in learning: latent class, latent profile, and latent transition analysis. Learn. Individ. Differ. 66, 4–15. doi: 10.1016/j.lindif.2017.11.001
Howard, M. C., and Hoffman, M. E. (2018). Variable-centered, person-centered, and person-specific approaches: where theory meets the method. Organ. Res. Methods 21, 846–876. doi: 10.1177/%2F1094428117744021
Hu, L., and Bentler, P. M. (1999). Cutoff criteria for fit indexes in covariance structure analysis: Conventional criteria versus new alternatives. Structural Equation Modeling: J. Multidiscip. Res. 6, 1–55. doi: 10.1080/10705519909540118
Hurst, C., and Hurrell, D. (2020). Multiplicative thinking: ‘pseudo-procedures’ are enemies of conceptual understanding. Int. Electron. J. Math. Educ. 15:em0611. doi: 10.29333/iejme/8567
Jõgi, A.-L., Kikas, E., Lerkkanen, M.-K., and Mägi, K. (2015). Cross-lagged relations between math-related interest, performance goals, and skills in groups of children with different general abilities. Learn. Individ. Differ. 39, 105–113. doi: 10.1016/j.lindif.2015.03.018
Kainulainen, M., McMullen, J., and Lehtinen, E. (2017). Early developmental trajectories toward concepts of rational numbers. Cogn. Instr. 35, 4–19. doi: 10.1080/07370008.2016.1251287
Kendeou, P., van den Broek, P., Helder, A., and Karlsson, J. (2014). A cognitive view of reading comprehension: implications for reading difficulties. Learn. Disabil. Res. Pract. 29, 10–16. doi: 10.1111/ldrp.12025
Kikas, E., Mädamürk, K., and Palu, A. (2019). What role do comprehension-oriented learning strategies have in solving math calculation and word problems at the end of middle school? Br. J. Educ. Psychol. 90, 105–123. doi: 10.1111/bjep.12308
Kintsch, W., and Greeno, J. G. (1985). Understanding and solving word arithmetic problems. Psychol. Rev. 92, 109–129. doi: 10.1037/0033-295X.92.1.109
Kintsch, W., and Mangalath, P. (2011). The construction of meaning. Top. Cogn. Sci. 3, 346–370. doi: 10.1111/j.1756-8765.2010.01107.x
Kirkland, P. K., and McNeil, N. M. (2021). Question design affects students’ sense-making on mathematics word problems. Cogn. Sci. 45:e12960. doi: 10.1111/cogs.12960
Koponen, T. K., Sorvo, R., Dowker, A., Räikkönen, E., Viholainen, H., Aro, M., et al. (2018). Does multi-component strategy training improve calculation fluency among poor performing elementary school children? Front. Psychol. 9:1187. doi: 10.3389/fpsyg.2018.01187
Krawitz, J., Schukajlow, S., and Van Dooren, W. (2018). Unrealistic responses to realistic problems with missing information: what are important barriers? Educ. Psychol. 38, 1221–1238. doi: 10.1080/01443410.2018.1502413
Lanza, S. T., Bray, B. C., and Collins, L. M. (2013). “An introduction to latent class and latent transition analysis” in Handbook of psychology. eds. J. A. Schinka, W. F. Velicer, and I. B. Weinger, Vol. 2. 2nd ed. (Hoboken, NJ: John Wiley), 691–716.
Lee, J. E. (2012). Prospective elementary teachers’ perceptions of real-life connections reflected in posing and evaluating story problems. J. Math. Teach. Educ. 15, 429–452. doi: 10.1007/s10857-012-9220-5
Leiss, D., Plath, J., and Schwippert, K. (2019). Language and mathematics—key factors influencing the comprehension process in reality-based tasks. Math. Think. Learn. 21, 131–153. doi: 10.1080/10986065.2019.1570835
Lindquist, M., Philpot, R., Mullis, I. V. S., and Cotter, K. E. (2017). “TIMSS 2019 mathematics framework” in TIMSS 2019 assessment frameworks. eds. I. V. S. Mullis and M. O. Martin (Chestnut Hill, MA: TIMSS & PIRLS International Study Center, Boston College), 11–25.
MacDonald, A., and Lowrie, T. (2011). Developing measurement concepts within context: Children’s representations of length. Math. Educ. Res. J. 23, 27–42. doi: 10.1007/s13394-011-0002-7
Mädamürk, K., Kikas, E., and Palu, A. (2016). Developmental trajectories of calculation and word problem solving from third to fifth grade. Learn. Individ. Differ. 49, 151–161. doi: 10.1016/j.lindif.2016.06.007
Mädamürk, K., Kikas, E., and Palu, A. (2018). Calculation and word problem-solving skill profiles: relationship to previous skills and interest. Educ. Psychol. 38, 1239–1254. doi: 10.1080/01443410.2018.1495830
Marsh, H. W., Lüdtke, O., Trautwein, U., and Morin, J. S. A. (2009). Classical latent profile analysis of academic self-concept dimensions: synergy of person- and variable-Centered approaches to theoretical models of self-concept. Struct. Equ. Model. 16, 191–225. doi: 10.1080/10705510902751010
McMullen, J., Hannula-Sormunen, M. M., Kainulainen, M., Kiili, K., and Lehtinen, E. (2019). Moving mathematics out of the classroom: using mobile technology to enhance spontaneous focusing on quantitative relations. Br. J. Educ. Technol. 50, 562–573. doi: 10.1111/bjet.12601
McMullen, J., and Siegler, R. S. (2020). Spontaneous focusing on multiplicative relations and fraction magnitude knowledge. Math. Think. Learn. 22, 351–359. doi: 10.1080/10986065.2020.1816284
Muthén, B., and Asparouhov, T. (2002). Using Mplus Monte Carlo simulations in practice: a note on non-normal missing data in latent variable models. Mplus Web notes: No. 2. Version 2, March 22, 2002.
Muthén, L. K., and Muthén, B. O. (2012–2017). Mplus User’s Guide. Eighth. Los Angeles, CA: Muthén & Muthén
Niss, M. (1994). “Mathematics in society” in Didactics of mathematics as a scientific discipline. eds. R. Biehler, R. W. Scholz, R. Strässer, and B. Winkelmann (Dordrecht, The Netherlands: Kluwer Academic Publishers), 367–378.
Niss, M., and Højgaard, T. (2019). Mathematical competencies revisited. Educ. Stud. Math. 102, 9–28. doi: 10.1007/s10649-019-09903-9
Novak, J., Markey, V., and Allen, M. (2007). Evaluating cognitive outcomes of service learning in higher education: a meta-analysis. Commun. Res. Rep. 24, 149–157. doi: 10.1080/08824090701304881
Nylund, K. L., Asparouhov, T., and Muthén, B. O. (2007). Deciding on the number of classes in latent class analysis and growth mixture modeling: a Monte Carlo simulation study. Struct. Equ. Model. Multidiscip. J. 14, 535–569. doi: 10.1080/10705510701575396
OECD (2010). The high cost of low educational performance. The long-run economic impact of improving PISA outcomes. Available at: https://www.oecd.org/pisa/44417824.pdf
OECD (2023a). PISA 2022 results (volume I): the state of learning and equity in education, PISA, OECD Publishing, Paris.
OECD (2023b). PISA 2022 Technical Report, Chapter 17: Proficiency Scale Construction for the PISA Core Domains. Available at: https://www.oecd.org/pisa/data/pisa2022technicalreport/PISA-2022-Technical-Report-Ch-17-PISA-Proficiency-Scale-Constructions-Core-Domains.pdf
Palm, T. (2008). Impact of authenticity on sense making in word problem solving. Educ. Stud. Math. 67, 37–58. doi: 10.1007/s10649-007-9083-3
Pongsakdi, N., Veermans, K., Lehtinen, E., Hannula-Sormunen, M. M., Laakkonen, E., and Laine, T. (2019). The role of beliefs and motivational variables in enhancing word problem solving. Scand. J. Educ. Res. 63, 179–197. doi: 10.1080/00313831.2017.1336475
Powell, S. R., Fuchs, L. S., and Gilbert, J. K. (2019). Variables influencing algebra performance: understanding rational numbers is essential. Learn. Individ. Differ. 74:101758. doi: 10.1016/j.lindif.2019.101758
Psyridou, M., Torppa, M., Tolvanen, A., Poikkeus, A.-M., Lerkkanen, M.-K., and Koponen, T. (2023). Developmental profiles of arithmetic fluency skills from grades 1 to 9 and their early identification. Dev. Psychol. 59, 2379–2396. doi: 10.1037/dev0001622
Reinke, L. T., and Casto, A. R. (2020). Motivators or conceptual foundation? Investigating the development of teachers’ conceptions of contextual problems. Math. Educ. Res. J. 34, 113–137. doi: 10.1007/s13394-020-00329-8
Reusser, K. (1988). Problem solving beyond the logic of things: contextual effects on understanding and solving word problems. Instr. Sci. 17, 309–338. doi: 10.1007/BF00056219
Reusser, K., and Stebler, R. (1997). Every word problem has a solution—the social rationality of mathematical modeling in schools. Learn. Instr. 7, 309–327. doi: 10.1016/S0959-4752(97)00014-5
Ritchie, S. J., and Bates, T. C. (2013). Enduring links from childhood mathematics and Reading achievement to adult socioeconomic status. Psychol. Sci. 24, 1301–1308. doi: 10.1177/0956797612466268
Rittle-Johnson, B. (2017). Developing mathematics knowledge. Child Dev. Perspect. 11, 184–190. doi: 10.1111/cdep.12229
Rittle-Johnson, B., Siegler, R. S., and Alibali, M. W. (2001). Developing conceptual understanding and procedural skill in mathematics: an iterative process. J. Educ. Psychol. 93, 346–362. doi: 10.1037/0022-0663.93.2.346
Rittle-Johnson, B., and Star, J. R. (2007). Does comparing solution methods facilitate conceptual and procedural knowledge? An experimental study on learning to solve equations. J. Educ. Psychol. 99, 561–574. doi: 10.1037/0022-0663.99.3.561
Schafer, J. L. (1999). Multiple imputation: a primer. Stat. Methods Med. Res. 8, 3–15. doi: 10.1177/096228029900800102
Scheibling-Sève, C., Pasquinelli, E., and Sander, E. (2020). Assessing conceptual knowledge through solving arithmetic word problems. Educ. Stud. Math. 103, 293–311. doi: 10.1007/s10649-020-09938-3
Siegler, R. S., and Ramani, G. B. (2008). Playing linear numerical board games promotes low-income children's numerical development. Dev. Sci. 11, 655–661. doi: 10.1111/j.1467-7687.2008.00714.x
Singer, V., and Strasser, K. (2017). The association between arithmetic and Reading performance in school: a meta-analytic study. Sch. Psychol. Q. 32, 435–448. doi: 10.1037/spq0000197
Sulak, S. E., and Güneş, F. (2017). The effects of teaching informative text through processual model on Reading comprehension skills. Int. Electron. J. Elem. Educ. 10, 265–271. doi: 10.26822/iejee.2017236121
The Council of European Union , (2018). Council Recommendationon of 22 May 2018 on key competences for lifelong learning (Text with EEA relevance.) ST/9009/2018/INIT. Available at: https://eur-lex.europa.eu/legal-content/EN/TXT/?uri=uriserv%3AOJ.C_.2018.189.01.0001.01.ENG&toc=OJ%3AC%3A2018%3A189%3ATOC
Tire, G., Puksand, H., Kraav, T., Jukk, H., Henno, I., Lindemann, K., et al. (2023). PISA 2022 Eesti tulemused Eesti 15-aastaste õpilaste teadmised ja oskused matemaatikas, funktsionaalses lugemises ja loodusteadustes. Tallinn, Estonia: Auratrükk.
Toomela, A., Mädamürk, K., Soodla, P., and Härma, E. (2020). Arvutipõhised hindamisvahendid lugemis- ja matemaatikapädevuse hindamiseks põhikooli I ja II kooliastmes. Juhendid testide läbiviimiseks ja tulemuste interpreteerimiseks. Available at: www.innove.ee/eksamid-ja-testid/uldpadevustestid/lugemis-ja-matemaatikapadevuse-testid/
Verschaffel, L., and De Corte, E. (1997). Teaching realistic mathematical modeling in the elementary school- a teaching experiment with fifth graders. J. Res. Math. Educ. 28, 577–601. doi: 10.2307/749692
Verschaffel, L., de Corte, E., and Lasure, S. (1994). Realistic considerations in mathematical modeling of school arithmetic word problems. Learn. Instr. 4, 273–294. doi: 10.1016/0959-4752(94)90002-7
Verschaffel, L., Schukajlow, S., Star, J., and Van Dooren, W. (2020). Word problems in mathematics education: a survey. ZDM 52, 1–16. doi: 10.1007/s11858-020-01130-4
Verschaffel, L., Van Dooren, W., Greer, B., and Mukhopadhyay, S. (2010). Reconceptualising word problems as exercises in mathematical modelling. J. Math.-Didakt. 31, 9–29. doi: 10.1007/s13138-010-0007-x
Vilenius-Tuohimaa, P. M., Aunola, K., and Nurmi, J. E. (2008). The association between mathematical word problems and reading comprehension. Educ. Psychol. 28, 409–426. doi: 10.1080/01443410701708228
Vygotsky, L. S. (1978). Mind in society: the development of higher psychological processes. Massachusetts: Harvard University Press.
Watts, T. W., Duncan, G. J., Siegler, R. S., and Davis-Kean, P. E. (2014). What's past is prologue: relations between early mathematics knowledge and high school achievement. Educ. Res. 43, 352–360. doi: 10.3102/0013189X14553660
Keywords: word problems, calculation, extra-mathematical knowledge, complex sentences, person-oriented methods
Citation: Sigus H and Mädamürk K (2024) Context matters: the importance of extra-mathematical knowledge in solving mathematical problems. Front. Educ. 9:1334034. doi: 10.3389/feduc.2024.1334034
Received: 06 November 2023; Accepted: 02 April 2024;
Published: 12 April 2024.
Edited by:
Jin Su Jeong, University of Extremadura, SpainReviewed by:
Nicolas Hübner, University of Tübingen, GermanyCopyright © 2024 Sigus and Mädamürk. This is an open-access article distributed under the terms of the Creative Commons Attribution License (CC BY). The use, distribution or reproduction in other forums is permitted, provided the original author(s) and the copyright owner(s) are credited and that the original publication in this journal is cited, in accordance with accepted academic practice. No use, distribution or reproduction is permitted which does not comply with these terms.
*Correspondence: Hardi Sigus, aGFyZGkuc2lndXNAdGx1LmVl
Disclaimer: All claims expressed in this article are solely those of the authors and do not necessarily represent those of their affiliated organizations, or those of the publisher, the editors and the reviewers. Any product that may be evaluated in this article or claim that may be made by its manufacturer is not guaranteed or endorsed by the publisher.
Research integrity at Frontiers
Learn more about the work of our research integrity team to safeguard the quality of each article we publish.