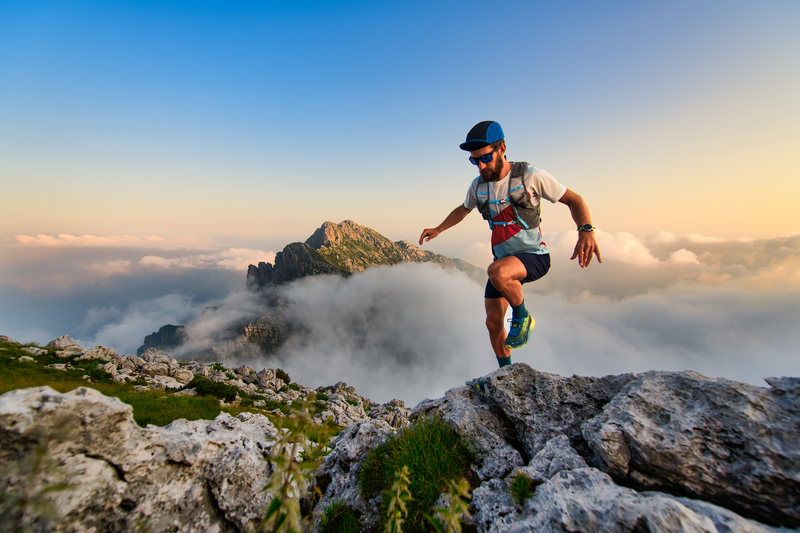
95% of researchers rate our articles as excellent or good
Learn more about the work of our research integrity team to safeguard the quality of each article we publish.
Find out more
ORIGINAL RESEARCH article
Front. Educ. , 30 April 2024
Sec. Educational Psychology
Volume 9 - 2024 | https://doi.org/10.3389/feduc.2024.1310449
This article is part of the Research Topic Systematic Screening to Support Well-being with PreK-12 Students View all 6 articles
Introduction: The Strengths and Difficulties Questionnaire (SDQ) is one the most widely used behavior screening tools for public schools due to its strong psychometric properties, low cost, and brief (25-question) format. However, this screening tool has several limitations including being primarily developed for the purposes of identifying clinical diagnostic conditions and primarily in a European population. To date, there has been minimal comparative research on measurement invariance in relationship to important U.S. socio-demographic metrics such as race and gender.
Method: This study utilized both structural equation modeling (i.e., confirmatory factor analysis) and item response theory (IRT) methods to investigate the measurement invariance of the SDQ across gender (male, female) and race (Black, White). CFA analyses were first conducted for each of the SDQ subscales to identify potential misfit in loadings, thresholds, and residuals. IRT-graded response models were then conducted to identify and quantify the between-group differences at the item and factor levels in terms of Cohen's d styled metrics (d > 0.2 = small, d > 0.5 = medium, d > 8 = large).
Results: There were 2,821 high school participants (52% Male, 48% Female; 88% Black, 12% White) included in these analyses. CFA analyses suggested that the item-factor relationship for most subscales were invariant, but the Conduct Problems and Hyperactivity subscales were non-invariant for strict measurement invariance. IRT analyses identified several invariant items ranging from small to large. Despite moderate to large effects for item scores on several scales, the test-level effects on scale scores were negligible.
Discussion: These analyses suggest that the SDQ subscale scores display reasonable comparable item-factor relationships across groups. Several subscale item scores displayed substantive item-level misfit, but the test level effects were minimal. Implications for the field are discussed.
School-based mental health services in the United States (U.S.) are of increasing interest over the last few decades as data have suggested that student's mental health has declined (Aldridge and McChesney, 2018; Arakelyan et al., 2023). To proactively identify students with behavioral concerns, many schools are engaging in universal behavior screening wherein students are screened with brief screening tools with scoring algorithms that allow administrators to flag students at potential risk of concerns (Glover and Albers, 2007; Chin et al., 2013; Dowdy et al., 2015). Universal screening is a shared aspect of most multi-tiered systems of support (MTSS) wherein students are triaged at varying levels of behavioral and educational risk [e.g., Response to Intervention (RTI), Positive Behavioral Interventions and Supports (PBIS), Comprehensive, Integrated, three-tiered model of prevention (Ci3T)]. Universal behavior screening is typically conducted at the school level, and additional information is collected on students flagged at risk to confirm risk and determine the need for low-level preventive interventions. Universal behavior screening augments traditional administrative systems that typically identify students once their behaviors have exceeded a critical behavioral threshold and resulted in negative consequences (e.g., office disciplinary referrals).
A notable benefit of universal behavior screening is the inclusion of internalizing behaviors thought to be predictive of educational outcomes (Melkevik et al., 2016; Finning, 2019; Wickersham et al., 2021). Historically, school system records prioritize the identification of students displaying externalizing behaviors (e.g., aggression, lying) that are easier to measure and, in the extreme, have clear negative impacts on educational outcomes for individual students, classrooms, and teacher wellbeing and retention. However, a growing body of evidence shows that internalizing symptoms and behaviors, including signs of anxiety or depression, are less likely to be captured via traditional administrative systems yet associated with meaningful educational outcomes (Melkevik et al., 2016; Finning, 2019; Wickersham et al., 2021).
Successful universal behavior screening is premised on the availability of brief, low-cost, and high-quality screening tools for use in educational settings (Oakes et al., 2014). Brevity is usually operationalized as a tool with items that may be filled out within 5–10 min and may be easily scored by hand or some automatized algorithm. Low-cost is a preferred term (rather than free) as few screening tools are truly open access, and all require some degree of time and resources in terms of teachers and administrators to plan for the implementation, scoring, and interpretation of findings. High quality refers to the design, reliability, and validity of screening tools. Design refers to screening tools shown to have utility in school settings, even when initially designed for clinical use in non-educational settings. Reliability and validity are standard psychometric concepts, respectively, referring to the consistency and accuracy of measurement (Furr, 2021).
There are a number of universal behavior screening tools that have either been designed or adapted for use in schools; however, Oakes et al. (2014) reported that only two screening tools meet the criteria for being brief, low-cost, and of high-quality: the Student Risk Screening Scale (SRSS; Drummond, 1994) and the Strengths and Difficulties Questionnaire (SDQ; Goodman, 1997). The SRSS and its more recent revised version, the Student Risk Screening Scale—Internalizing and Externalizing (SRSS-IE; Drummond, 1994; Lane and Menzies, 2009), are universal behavior screening tools designed specifically for use in U.S. school systems and under active psychometric development by the Ci3T research team network (https://www.ci3t.org/). The SDQ is a clinical scale that has been adapted for use in school-based settings and has a large body of psychometric research supporting different (e.g., caregiver, teacher, self-report) versions across multiple ages. Thus, evidence indicates that these tools are useful for universal screening as the scales have good validity, are reliable at identifying students at risk of negative educational outcomes, and are low cost.
The SDQ is a low-cost screener designed to identify externalizing and internalizing symptoms in students (Goodman, 1997). The SDQ is comprised of 25 items that combine into five subscales, respectively, designed to measure conduct problems, hyperactivity, peer problems, withdrawal, and social skills. The conduct problems and hyperactivity subscales may be combined to create an Externalizing scale, and the peer problems and withdrawal scales may be combined to create an Internalizing scale. The four externalizing and internalizing scales, together and in combination, are most frequently employed in literature. Each five-item scale is scored on a 0 (i.e., not true), 1 (i.e., somewhat true), and 2 (i.e., certainly true) Likert scale with summative measures ranging from 0, least severe, to 10, most severe. Parent, teacher, and child versions of the SDQ have been developed, as have cut points for each subscale. Historically, on the student SDQ, for example, a score of 5 or greater would flag students as abnormal on the emotion problems subscale, 4 or greater would flag students on the conduct problems or peer problems subscale, and 7 or greater would flag students on the hyperactivity subscale.
Despite many years of substantial psychometric investigation, the research on the SDQ is limited in several different ways. First, most of the research to date focuses on traditional psychometric analyses, including global factor analyses outlining the latent structure and justifying the utility of the proposed scales (exploratory and confirmatory), predictive validity studies, and traditional reliability studies (Caci et al., 2015; Graybill et al., 2021). Second, most of the research has been conducted on non-school-based clinical populations in the U.K., where the SDQ was originally developed, or in other non-U.S. countries (Stone et al., 2010; Croft et al., 2015; Hoosen et al., 2018; Ferreira et al., 2021). Psychometric studies in the U.S. are substantially more rarely conducted, particularly in relationship to the utility of the SDQ in schools (He et al., 2013; Jones et al., 2020; Graybill et al., 2021). Third, comparative research establishing that the SDQ measures traits similarly across socio-demographic groups is rarely conducted, with most available research also conducted on UK populations. Finally, various versions of the SDQ have been developed for different language groups (e.g., U.S. English, U.K. English versions), different ages, and for different raters, with most of the comparative research to date conducted on gender and ethnic groups in non-U.S. settings (Kersten et al., 2016).
A small body of research generally supports the applied use of the SDQ in U.S.-based school settings. For example, recent research shows that the SDQ scale scores are valid for predicting relevant school-based outcomes (Jones et al., 2020; Graybill et al., 2022). For example, in a validity study of interest to school administrators, Jones et al. (2020) recently reported that the SDQ predicted office disciplinary referrals (ODRs). Furthermore, factor analytic work with optimal analyses accounting for the SDQ ordinal design suggests that the originally proposed 5-factor model generally captures the externalizing and internalizing traits of U.S. school-age populations for the self-rating forms frequently used in universal screening studies, allowing for some items cross-loading (Ruchkin et al., 2008; Graybill et al., 2021). Research on parent-report forms has been more variable, with some studies suggesting 3-, and others 5-, factors (Dickey and Blumberg, 2004; Palmieri and Smith, 2007; He et al., 2013).
Measurement invariance refers to the fact that groups of people may systematically differ on either the underlying traits measured by items andto scales or how they engage or interpret items (Mindt et al., 2010; Warne et al., 2014). When engagement with, understanding of, or interpretationto of items differ systematically across groups of people, or traits are fundamentally different across groups, the interpretation and use of scale scores are undermined due to meaningful group variance (i.e., referred to as non-invariance in the methodological literature). In other words, for scales to be valid for comparisons across different groups, it should be established that the same trait is measured similarly across groups (Mindt et al., 2010; Warne et al., 2014). Further, the negative effects of inadequately vetted instruments are known to diminish interpretations of mean comparisons and may relate to well-established problems with higher false positive rates seen in some marginalized populations, resulting in unnecessary concerns and costs for individuals and family members and wasted service provision for systems (Guthrie et al., 2019; Gonzalez and Pelham, 2021).
Few U.S. measurement invariance studies have been conducted to determine if the SDQ scales display equitable scoring across gender and Black and White race groupings. Considering the widespread use of the SDQ, the need for comparative psychometric research across major sociodemographic groups in the U.S. is vitally important. Furthermore, for school systems using the SDQ for behavioral surveillance, between subscale non-invariance could result in students incorrectly identified (i.e., false positives) or inappropriate interpretations of mean group comparisons. To date, gender and race invariance has been investigated in two studies of U.S. students with the SDQ (He et al., 2013; Graybill et al., 2021). He et al. (2013) and Graybill et al. (2021) reported non-invariance for race and gender, respectively, on the caregiver and self-report SDQ. Graybill et al. reported on U.S. middle school students in the Southeastern U.S. and He et al. adolescents aged 13–18. Both studies reported that the SDQ has five distinct factors conforming to the hypothesized structure by Goodman (1997).
The primary purpose of this study is to investigate the measurement invariance of gender (male/female) and race (Black/White) on the self-rated SDQ subscales in a racially diverse group of high school students from the Southeastern U.S. Gender and race were selected as these subgroups are commonly tracked at the school and district level in behavioral surveillance studies. As such, non-invariance can result in differential missed (e.g., false negative) cases or misrepresent student behavioral health at the aggregate mean level (Meade, 2010; Gonzalez and Pelham, 2021). Specifically, we will use two of the most used statistical approaches for identifying group differences in traits: multi-group Confirmatory Factor Analysis (MG-CFA) and Differential Item and Differential Test Functioning (DIF/DTF) (Teresi, 2006). Though these developed from different methodological traditions, MG-CFA and DIF/DTF largely share the same scientific goal: falsifying hypotheses regarding equivalence between groups (Putnick and Bornstein, 2016). Historically, MG-CFA was developed with parametric data in mind and has developed adapted approaches to deal with non-parametric (e.g., ordinal Likert items) data typical of social sciences, including scales used in universal screening. Item response theory (IRT)/DIF approaches, on the other hand, were initially developed with non-parametric categorical data. Notably, despite some unique differences for both approaches, “the principal concern is the same: determining whether item parameters are equal across groups” (Bauer, 2017, p. 7). This will be the first SDQ study co-considering IRT and MG-CFA approaches to answer the following research question: Is there measurement invariance of the SDQ across gender (male, female) and race (Black, White) within a US sample of high school students?
Participants included 2,821 unique respondents, the majority of whom identified as Black (n = 2,473, ~87.7%), with a minority as White (n = 348, 12.3%). Gender was forced choice and evenly split among the participants (Female n = 1,464, 51.9%; Male n = 1,302; 46.2%; Non-binary n = 0.5%; Prefer not to say n = 41; ~1.5%). Due to small samples, non-binary and prefer not to say gender identification was low, these were changed to missing and not included in analyses. Grade was evenly split (9th n = 88, 31.3%; 10th n = 766, 27.2%; 11th n = 618, 21.9%, 19.4%; 12th n = %; missing n = 6, 0.2%). The participation rate was 49.6%, which is comparable to other studies reporting high school screening data (e.g., Siceloff et al., 2017). See Table 1 for details.
Table 1. Descriptive statistics of 2,821 high school students in a large state in the Southeastern U.S. who the Strengths and Difficulties Questionnaire.
Data collected here were from six high schools in the Southeastern U.S. All schools had a free and reduced lunch rate of 95%. See Table 2 for demographic variation across the six schools.
For this study, four SDQ scales from the SDQ were used: Emotional Symptoms, Peer Problems, Hyperactivity, and Conduct Problems. Emotional Symptoms and Peer Problems are considered internalizing scales and are frequently summed to create a scale to that effect; Hyperactivity and Conduct Problems are externalizing scales, also summed to create an externalizing scale (Caci et al., 2015; Margherio et al., 2019). Scoring for all scales developed from an ordinal ranking of 0 (i.e., not true), 1 (i.e., somewhat true), and 2 (i.e., certainly true) on Likert selections. This study used the student self-report U.S. English version for students ages 4–17, though there are parent, teacher, and student self-report versions available (Goodman, 1997).
Item interpretations are available in Table 3.
This study was approved by the author's home university (IRB # H15404). Data for all analyses were collected as part of the first wave of a universal behavior screening initiative for high school students in a large state in the Southeastern U.S. The first author met monthly with school administrators across the course of the project to ensure that the project was conducted in accordance with district approvals and with project fidelity. During a planning year, all schools were trained by one of the authors on a consistent process for collecting screening data. In tandem with trainings school personnel ensured that study consent was procured from participating students. Screening data were collected in all schools 30 days after the start of the school year. Students with parent consent from participating schools completed the SDQ online using a secure server. SDQ data were used to inform data-based decision -making within the schools' multi-tiered system of support.
To address invariance across groups, both MC-CFA and IRT DIF/DTF were conducted. Within MG-CFA, factor, item intercepts, factor loadings, and item residuals will be tested to determine if constraining groups to strict (intercepts, factor loadings, and residuals), strong (intercepts and factor loadings) or weak (factor loadings only) assumptions impact the interpretations of SDQ scales across groups (Meredith, 1993). IRT approaches do not address residuals (i.e., strict invariance) but do focus on intercepts and factor loadings (respectively difficulty and discrimination parameters in IRT parlance), and historically consider both uniform (i.e., differences in intercepts only) and non-uniform (differences in factor loadings, independent of intercept differences) DIF. Efforts to understand the common and unique strengths and challenges of MC-CFA and IRT approach is an area of active research (e.g., Bauer, 2017). Currently, research suggests that Type 1 errors are inflated in IRT compared to MG-CFA approaches (i.e., more items identified as discrepant than truly are), but that IRT is more powerful for identifying non-uniform DIF than MG-CFA (Elosua and Wells, 2013).
For the MG-CFA, robust diagonal weighted least squares (DWLS) confirmatory factor analyses (CFA) were conducted to determine global differences across race and gender (Rosseel, 2012; Li, 2015). Initial models loaded items on a single univariate factor. Items were allowed to covary in the event of poor fit. Differences between models were determined by considering the chi-square test of model fit, the root mean square error of approximation (RMSEA), the standardized root mean squared (SRMS), the Bentler comparative fit index (CFI), and the Tucker–Lewis fit index (TLI). Nonsignificant chi-square tests indicated good fit but are rare due to large sample sizes such as those used here. Values <0.08 for RMSEA/SRMS values are considered acceptable, with <0.05 considered as good. Further, values >0.90 are considered acceptable, and >0.95, for CFI and TLI (Schreiber et al., 2006). For group comparisons, the following were used to determine meaningful changes: ΔCFI > 0.01; ΔRMSEA > 0.15; ΔSRMSR > 0.03 (Chen, 2007).
IRT Differential Item Functioning was conducted using Samejima's (1969) graded response model (GRM) approach. Discrimination parameters (i.e., how well scores discriminate between individuals at different trait levels) were conducted for each item at the overall and threshold (i.e., for each Likert level) and interpreted using Baker's (2001) framework: 0 = minimal; 0.01 to 0.34 = very low, 0.35 to 0.64 = low; 0.65 to 1.34 = moderate; 1.35 to 1.69 = high; >1.7 = very high (Baker, 2001). The Likelihood Ratio Test (LRT) approach was used to determine DIF between race and ethnic groups, which employs a constrained model wherein group parameters are forced to be equal; this is then compared to a model with means/variances varying across groups; chi-square is then used to develop p-values (Kim and Cohen, 1998). Items with p-values < 0.01 are considered to display non-DIF and are used as anchor items against which items displaying DIF are compared (Meade and Wright, 2012). LRT is robust to sample size inequalities, like those seen in the race comparisons (Clark and LaHuis, 2012). The R mirt package was used to conduct these analyses (Chalmers, 2012). Finally, Expected Score Standardized Difference (ESSD) effects sizes were developed to help interpret the meaningfulness of item and group differences (Meade, 2010). ESSD is considered interpretable as Cohen's d (Cohen, 1988) where d < 0.2 is negligible, d > 0.2 and < 0.5 is small, d > 0.5 and < 0.8 is moderate, and d > 0.8 large.
To establish that the SDQ subscales reflect reasonably well-fitting item factor relationships for our sample, univariate CFAs were fit for the Conduct Problems, Emotion Problems, Hyperactivity, and Peer Problems subscales for the entire sample. The SDQ Conduct Problems scale indicated adequate item to factor fit (CFI = 0.96, TLI = 0.92, RMSEA = 0.05, SRMS = 0.04) and with good fit when allowing items 12 and 18 to correlate (CFI = 0.99, TLI = 0.98, RMSEA = 0.03, SRMS = 0.02). The Emotion Problems scale indicated good item to factor fit (CFI = 0.99, TLI = 0.99, RMSEA = 0.04, SRMS = 0.03). The Hyperactivity scale indicated adequate item to factor fit (CFI = 0.96, TLI = 0.94, RMSEA = 0.09, SRMS = 0.05) and was good fitting when items 21 and 25 were allowed to covary (CFI = 0.99, TLI = 0.98, RMSEA = 0.05, SRMS = 0.03). The Peer Problems scale was poorly fitting initially (CFI = 0.73, TLI = 0.46, RMSEA = 0.15, SRMS = 0.05) and require allowing item 23 to covary with items 6 and 14 to achieve adequate to good fit (CFI = 0.98, TLI = 0.94, RMSEA = 0.05, SRMS = 0.02; Table 4).
Table 4. CFA global factor structure and configural, weak, strong, and strict measurement invariance for race and gender on Strengths and Difficulties Questionnaire.
Configural, weak, strong, and strict invariance was maintained for the Conduct Problems, Emotional Symptoms, and Hyperactivity scales (ΔCFIs < 0.01; ΔRMSEAs < 0.15; ΔSRMSRs < 0.03; Table 4). The Peer Problems scale displayed differences for the strict invariance only for the CFI metric (ΔCFI > 0.01; Table 4).
The were no substantive differences for configural, weak, or strong invariance for the Conduct Problems, Emotional Symptoms, Hyperactivity, or Peer Problems scales (ΔCFIs < 0.01; ΔRMSEAs < 0.15; ΔSRMSRs < 0.03; Table 4). Changes in CFI and SRMR from strong to strict was seen for Conduct Problems (ΔCFI > 0.01; ΔSRMSR > 0.03). Changes in CFI, RMSEA, and SRMSR were also seen for the Emotional Symptoms subscales (ΔCFI > 0.01; ΔRMSEA > 0.15; ΔSRMSR > 0.03). This suggests between group differences in residuals for Conduct and Emotional Problems (Table 4).
For distinguishing between individuals moving from not true to somewhat true, item 22 was very high, item 12 strong, and items 5, 7, and 18 low; for distinguishing between individuals moving from somewhat true to certainly true, all items were very high. Black and White students displayed negligible DIF on items 5 (–LL = −9,234.622, χ2 = 13.07, p = 0.004, d = 0.09) and 7 (–LL = −9,233.171, χ2 = 15.97, p = 0.001, d = 0.10), but none on 22 (–LL = −9,239.172, χ2 = 3.97, p = 0.265, d = 0.19), 12 (–LL = −9,239.399, χ2 = 3.51, p = 0.319) or 18 (–LL = −9,240.217, χ2 = 1.88, p = 0.597). Male and female students displayed moderate DIF on item 5 (–LL = −9,020.228, χ2 = 48.92, p < 0.001, d = 0.62), small on item 7 (–LL = −9,044.481, χ2 = 0.41, p = 0.003, d = 0.28), negligible on item 22 (–LL = −9,035.759, χ2 = 17.86, p < 0.001, d = 0.19), and none on items 12 (–LL = −9,044.481, χ2 = 0.41, p = 0.937) or 18 (–LL = −9,042.108, χ2 = 5.16, p = 0.160). There were negligible test level differences for race (d = 0.007) or gender (d = 0.12).
For distinguishing between individuals moving from not true to somewhat true, item 2 was moderate, items 15, 21, and 25 low, and item 10 low; for distinguishing between individuals moving from somewhat true to certainly true, items 10, 21, and 25 were very strong and items 2 and 15 were moderate. Black and White students displayed small DIF levels on item 10 (–LL = −12,671.80, χ2 = 19.75, p < 0.001, d = 0.40), negligible on item 2 (–LL = −12,674.73, χ2 = 13.89, p = 0.003, d = 0.05), and none on items 15 (–LL = −12,679.52, χ2 = 4.31, p = 0.230), 21 (–LL = −12,677.53, χ2 = 8.30, p = 0.040), or 25 (–LL = −12,679.17, χ2 = 5.01, p = 0.171), which were set to anchor. Male and female students displayed small levels of DIF on items 10 (–LL = −12,443.60, χ2 = 28.21, p < 0.001, d = 0.27) and 25 (–LL = −12,449.92, χ2 = 15.58, p = 0.001, d = 0.27), negligible on item 2 (–LL = −12,450.19, χ2 = 15.02, p = 0.002, d = 0.16), and none on items 15 (–LL = −12,455.37, χ2 = 4.67, p = 0.197) and 21 (–LL = −12,454.17, χ2 = 7.08, p = 0.069), which were set to anchor. There were negligible test level differences for race (d = 0.09) and gender (d = 0.01).
For distinguishing between individuals moving from not true to somewhat true, items 3 and 13 were very low, items 8, 16, and 24 were low, and item 10 was minimal; for distinguishing between individuals moving from somewhat true to certainly true, items 3, 13, and 24 were very high, and items 8 and 16 were moderate. Black and White students displayed small levels of DIF on item 24 (–LL = −12,499.28, χ2 = 16.25, p = 0.001, d = 0.41), but none on 3 (–LL = −12,503.07, χ2 = 8.67, p = 0.034), 8 (–LL = −12,503.49, χ2 = 7.84, p = 0.049), 13 (–LL = −12,504.36, χ2 = 6.09, p = 0.107), or 16 (–LL = −12,505.74, χ2 = 3.34, p = 0.314), which were set to anchor. Male and female students displayed small levels of DIF on item 3 (–LL = −12,129.32, χ2 = 10.48, p = 0.015, d = 0.28), negligible on 8 (–LL = −12,127.98, χ2 = 13.15, p = 0.004, d = 0.10), and none on items 13 (–LL = −12,130.77, χ2 = 7.57, p = 0.056), 16 (–LL = −12,133.93, χ2 = 1.27, p = 0.736), and 24 (–LL = −12,130.72, χ2 = 7.69, p = 0.053), which were set to anchor. There were negligible test level differences for race (d = 0.008) and gender (d = 0.02).
For distinguishing between individuals moving from not true to somewhat true, items 19 and 23 were very high, item 6 was high, item 11 moderate, and item 14 low; for distinguishing between individuals moving from somewhat true to certainly true, all items were very high. Black and White students displayed moderate levels of DIF on items 6 (–LL = −11,663.05, χ2 = 10.55, p = 0.014, d = 0.54) and 11 (–LL = −11,653.14, χ2 = 30.373, p < 0.001, d = 0.71), small levels on item 14 (–LL = 11,648.69, χ2 = 39.28, p = < 0.001, d = 0.46), and negligible on item 19 (–LL = −11,662.24, χ2 = 12.17, p = 0.007, d = 0.17). No DIF was present on item 23 (–LL = −11,666.32, χ2 = 4.01, p = 0.260), which was set to anchor. Male and female students displayed moderate DIF on item 6 (–LL = −11,345.38, χ2 = 35.82, p = < 0.001, d = 0.54) and small levels on item14 (–LL = −11,347.48, χ2 = 31.61, p = < 0.001, d = 0.46), but none on items 11 (–LL = −11,362.97, χ2 = 0.63, p = 0.89), 19 (–LL = −11,360.92, χ2 = 4.74, p = 0.191), and 23 (–LL = −11,362.42, χ2 = 1.73, p = 0.629). There were negligible test level differences for race (d = 0.15) and gender (d = 0.07).
The SDQ is one of the most widely used mental health screening tools in the world; however, despite a substantive body of psychometric work on this instrument internationally, there are relatively few U.S.-based factor analytic studies and only one focused on the Southeastern U.S. Thus, this study breaks ground in a few ways. First, this is the first U.S.-based SDQ study to conduct an in-depth analysis of measurement invariance across Black and White students in high school using the self-report SDQ. Second, this study adds to a smaller body of work outlining gender invariance on the SDQ. Ultimately, the data reported here suggest that, despite some item-level differences, SDQ scores do not vary substantively between Black and White and male and female adolescents in the U.S. in a manner that schools should be concerned about systematically misinterpreting individual cut-off or mean-level scores.
This study resonates with a growing body of work suggesting that the SDQ scores are psychometrically valid and useful for school-based screening studies in schools with racially diverse student bodies (He et al., 2013; Graybill et al., 2021). Most recently, Graybill et al. (2021) reported minimal differences in residuals across several models between socio-demographics for middle school students in the Southeastern U.S. There are several notable differences between the current study and that of Graybill et al. (2021). First, Graybill et al. investigated the multi-variate global structure of the SDQ, whereas the current analysis considers the univariate scales. Second, the current analysis co-considers CFA alongside IRT to leverage the IRT method for establishing Cohen's d like metrics of item and scale differences between groups. And third, Graybill et al., focused on a slightly different age group compared to the current analysis (i.e., middle school students). Despite these differences in focus, both sets of analysis indicate that the SDQ scales display some measurement invariance but that the impact from a CFA perspective is that residuals are the only substantive impact indicating that SDQ subscale scores are largely comparable across groups.
Alongside this research are other U.S.-based studies with secondary students indicating that the SDQ subscale scores are useful for predicting which students are at greater odds of office disciplinary referrals (ODRs). For example, Jones et al. (2020) found that the high school students' SDQ scores predicted both ODRs and absences and identified overlapping and similar students at risk for these ODRs and absences compared to other screening tools. Furthermore, Graybill et al. (2022) recently reported that fall SDQ scores predicted the number of ODRs across the year for middle school students, even when controlling for important demographic co-variates and the total number ODRs received in the fall. That the SDQ scores seem to be non-invariant suggests that these subscales are measuring the same trait across race and gender. Thus, the current study fits with a line of research indicating that the SDQ may be useful for universal screening purposes.
These results contribute to the research investigating student self-report behavior screening in secondary schools. Our understanding of the value of screening in secondary schools is emerging and there are many behavioral screening tools currently on the market available for school leaders to consider for behavioral surveillance projects; however, as more school leaders grapple with youth mental health concerns and the pressing demand to identify students in need of supports early, the availability of tools vetted for equitably use with racially diverse audiences is necessary. Collectively, research on the SDQ suggests that this tool's scores are likely equitably useful across genders and with Black and White students in that it appears to tap similar constructs. The current analyses indicate that mean differences between groups are safely inferred to carry the same meaning, and this tool is useful for administrators to consider for behavioral surveillance projects as scores do not appear systematically biased in one group over another.
Now that a lower threshold has been set regarding the invariance of the two top low-cost tools used for school-based universal screening, there is a need to refine our understanding across several areas. First, comparisons between gender and Black and White race groupings are necessary but insufficient for determining the use of these tool's scores in racially diverse school systems. Thus, more equity work is needed regarding both instruments with other socio-demographic groups (e.g., Native American populations) (Gone, 2021). Second, there is still the pressing need to determine the utility of the internalizing symptoms scales in relation to outcomes relevant to school administrators. Currently, most studies focus on ODRs, absences, and other negative outcomes that existing data systems address (Jones et al., 2020; Graybill et al., 2022). The data regarding internalizing symptoms for these scale scores has not yet been firmly established.
Despite several strengths, this study has some important limitations that should be underscored. First, there is the fact that our White sample (n = 348, 12%) was relatively small in comparison to the Black sample (n = 2,473, 88%). Thus, although we have a relatively large sample in comparison to commonplace rules of thumb (e.g., samples >200), it is possible that larger samples would result in substantively different findings. However, we anticipate that this is not likely as many factor analyses have been conducted on primarily Eurocentric populations, including from the U.S.; while none have compared race groups, the fact that the item-factor relations largely corresponded to hypothesized relationships in the CFAs (with mild deviations at the item levels revealed by the DIF analyses) indicates a largely similar structure. Additionally, while this study extends research on the SDQ in the Southeastern U.S., there are currently no invariance studies from other regions of the U.S. Furthermore, this study does not investigate whether the mild levels of invariance identified has pragmatic impacts in relation to differences in relevant outcomes (e.g., ODRs, grades). Recently, researchers have begun to outline ways to show the applied impact of invariance on relevant outcomes, in particular diagnostic accuracy (Gonzalez and Pelham, 2021). Specifically, Gonzalez and Pelham (2021) developed a procedure to estimate the practical impact of DIF on sensitivity and sensitivity in typical clinical research settings. The conceptual framework for understanding the impact of DIF on downstream systemic outcomes relevant to educational systems is currently not developed. However, one could theoretically frame school outcomes within a diagnostic accuracy framework and seek to establish unique inequity measures displaying how ODRs, for example, are (in)equitably administered according to latent trait metrics. Thus, while these findings provide an initial test indicating that the scales are psychometrically similar across groups, caution regarding the general strength of this instrument for universal use is warranted pending replications and extensions to other regions and with other demographic groups.
The datasets presented in this article are not readily available because these data are not publicly available due to restrictions required by collaborating educational institutions. Requests to access the datasets should be directed to ZWdyYXliaWxsQGdzdS5lZHU=.
The studies involving humans were approved by Federal Wide Assurance (FWA) of Compliance (Number 00000129). The studies were conducted in accordance with the local legislation and institutional requirements. Written informed consent for participation in this study was provided by the participants' legal guardians/next of kin.
EG: Conceptualization, Data curation, Funding acquisition, Investigation, Project administration, Resources, Supervision, Writing – review & editing. BB: Formal analysis, Methodology, Software, Writing – original draft. AS: Data curation, Project administration, Writing – review & editing. SL: Formal analysis, Writing – original draft.
The author(s) declare financial support was received for the research, authorship, and/or publication of this article. This paper was developed in part under grant number SM061877 from the Substance Abuse and Mental Health Services Administration (SAMHSA), U.S. Department of Health and Human Services (HHS). The views, policies, and opinions expressed are those of the authors and do not necessarily reflect those of SAMHSA or HHS.
The authors declare that the research was conducted in the absence of any commercial or financial relationships that could be construed as a potential conflict of interest.
All claims expressed in this article are solely those of the authors and do not necessarily represent those of their affiliated organizations, or those of the publisher, the editors and the reviewers. Any product that may be evaluated in this article, or claim that may be made by its manufacturer, is not guaranteed or endorsed by the publisher.
Aldridge, J. M., and McChesney, K. (2018). The relationships between school climate and adolescent mental health and wellbeing: a systematic literature review. Int. J. Educ. Res. 88, 121–145. doi: 10.1016/j.ijer.2018.01.012
Arakelyan, M., Freyeue, S., Avula, D., McLaren, J. L., O'Malley, J., Leyenaar, J. K., et al. (2023). Pediatric mental health hospitalizations at acute care hospitals in the US, 2009-2029. JAMA 329, 1000–1011. doi: 10.1001/jama.2023.1992
Baker, F. (2001). The Basics of Item Response Theory. College Park, MD: ERIC Clearinghouse on Assessment and Evaluation, University of Maryland.
Bauer, D. J. (2017). A more general model for testing measurement invariance and differential item functioning. Psychol. Methods 22:507. doi: 10.1037/met0000077
Caci, H., Morin, A. J., and Tran, A. (2015). Investigation of a bifactor model of the strengths and difficulties questionnaire. Eur. Child Adolesc. Psychiatry 24, 1291–1301. doi: 10.1007/s00787-015-0679-3
Chalmers, R. P. (2012). mirt: a multidimensional item response theory package for the R environment. J. Stat. Softw. 48, 1–29. doi: 10.18637/jss.v048.i06
Chen, F. F. (2007). Sensitivity of goodness of fit indexes to lack of measurement invariance. Struct. Equ. Model. 14, 464–504. doi: 10.1080/10705510701301834
Chin, J. K., Dowdy, E., and Quirk, M. P. (2013). Universal screening in middle school: examining the behavioral and emotional screening system. J. Psychoeduc. Assess. 31, 53–60. doi: 10.1177/0734282912448137
Clark, P. C., and LaHuis, D. M. (2012). An examination of power and type I errors for two differential item functioning indices using the graded response model. Organ. Res. Methods 15, 229–246. doi: 10.1177/1094428111403815
Cohen, J. (1988). Effect Sizes: Power Analysis for the Behavioural Sciences, 2nd ed. Mahwah, NJ: Lawrence Erlbaum.
Croft, S., Stride, C., Maughan, B., and Rowe, R. (2015). Validity of the strengths and difficulties questionnaire in preschool-aged students. Pediatrics 135, e1210–e1219. doi: 10.1542/peds.2014-2920
Dickey, W. C., and Blumberg, S. J. (2004). Revisiting the factor structure of the strengths and difficulties questionnaire: United States, 2001. J. Am. Acad. Child Adolesc. Psychiatry 43, 1159–1167. doi: 10.1097/01.chi.0000132808.36708.a9
Dowdy, E., Furlong, M., Raines, T. C., Bovery, B., Kauffman, B., Kamphaus, R. W., et al. (2015). Enhancing school-based mental health services with a preventive and promotive approach to universal screening for complete mental health. J. Educ. Psychol. Consult. 25, 178–197. doi: 10.1080/10474412.2014.929951
Drummond, T. (1994). The Student Risk Screening Scale (SRSS). Grants Pass, OR: Josephine County Mental Health Program. doi: 10.1037/t27737-000
Elosua, P., and Wells, C. S. (2013). Detecting DIF in polytomous items using MACS, IRT and ordinal logistic regression. Psicológica 34, 327–342. Available online at: https://www.redalyc.org/articulo.oa?id=16929535011
Ferreira, T., Geiser, C., Cadima, J., Matias, M., Leal, T., Mena Matos, P., et al. (2021). The strengths and difficulties questionnaire: an examination of factorial, convergent, and discriminant validity using multitrait-multirater data. Psychol. Assess. 33, 45–59. doi: 10.1037/pas0000961
Finning, K. (2019). The Association between Emotional Disorder and Absence from School in Students and Young People. Exeter: University of Exeter.
Glover, T. A., and Albers, C. A. (2007). Considerations for evaluating universal screening assessment. J. Sch. Psychol. 45, 117–135. doi: 10.1016/j.jsp.2006.05.005
Gone, J. P. (2021). The (post) colonial predicament in community mental health services for American Indians: explorations in alter-Native psy-ence. Am. Psychol. 76:1514. doi: 10.1037/amp0000906
Gonzalez, O., and Pelham III, W. E. (2021). When does differential item functioning matter for screening? A method for empirical evaluation. Assessment 28, 446–456. doi: 10.1177/1073191120913618
Goodman, R. (1997). The strengths and difficulties questionnaire: a research note. J. Child Psychol. Psychiatry 38, 581–586. doi: 10.1111/j.1469-7610.1997.tb01545.x
Graybill, E., Roach, A., and Barger, B. (2021). Factor structure of the self-report strength and difficulties questionnaire in a diverse US sample. J. Psychopathol. Behav. Assess. 43, 388–398. doi: 10.1007/s10862-020-09833-4
Graybill, E., Salmon, A., Barger, B., and Roach, A. T. (2022). Examining the predictive utility of the self-report Strengths and Difficulties Questionnaire with middle school students. Int. J. Ment. Health 1–13. doi: 10.1080/00207411.2022.2038983
Guthrie, W., Wallis, K., Bennett, A., Brooks, E., Dudley, J., Gerdes, M., et al. (2019). Accuracy of autism screening in a large pediatric network. Pediatrics 144:e20183963. doi: 10.1542/peds.2018-3963
He, J. P., Burstein, M., Schmitz, A., and Merikangas, K. R. (2013). The Strengths and Difficulties Questionnaire (SDQ): the factor structure and scale validation in US adolescents. J. Abnorm. Child Psychol. 41, 583–595. doi: 10.1007/s10802-012-9696-6
Hoosen, N., Davids, E. L., de Vries, P. J., and Shung-King, M. (2018). The Strengths and Difficulties Questionnaire (SDQ) in Africa: a scoping review of its application and validation. Child Adolesc. Psychiatry Ment. Health 12, 1–39. doi: 10.1186/s13034-017-0212-1
Jones, C., Graybill, E., Barger, B., and Roach, A. T. (2020). Examining the predictive validity of behavior screeners across measures and respondents. Psychol. Sch. 57, 923–936. doi: 10.1002/pits.22371
Kersten, P., Czuba, K., McPherson, K., Dudley, M., Elder, H., Tauroa, R., et al. (2016). A systematic review of evidence for the psychometric properties of the Strengths and Difficulties Questionnaire. Int. J. Behav. Dev. 40, 64–75. doi: 10.1177/0165025415570647
Kim, S. H., and Cohen, A. S. (1998). Detection of differential item functioning under the graded response model with the likelihood ratio test. Appl. Psychol. Meas. 22, 345–355. doi: 10.1177/014662169802200403
Lane, K. L., and Menzies, H. M. (2009). Student Risk Screening Scale for early internalizing and externalizing behavior (SRSS-IE) (Screening scale). Available online at: Ci3t.org/screening (accessed March 12, 2024).
Li, C. (2015). Confirmatory factor analysis with ordinal data: comparing robust maximum likelihood and diagonally weighted least squares. Behav. Res. Methods 48, 936–949. doi: 10.3758/s13428-015-0619-7
Margherio, S. M., Evans, S. W., and Owens, J. S. (2019). Universal screening in middle and high schools: Who falls through the cracks? Sch. Psychol. 34, 591–602. doi: 10.1037/spq0000337
Meade, A. W. (2010). A taxonomy of effect size measures for the differential functioning of items and scales. J. Appl. Psychol. 94, 728–743. doi: 10.1037/a0018966
Meade, A. W., and Wright, N. A. (2012). Solving the measurement invariance anchor item problem in item response theory. J. Appl. Psychol. 97, 1016–1031. doi: 10.1037/a0027934
Melkevik, O., Nilsen, W., Evensen, M., Reneflot, A., and Mykletun, A. (2016). Internalizing disorders as risk factors for early school leaving: a systematic review. Adolesc. Res. Rev. 1, 245–255. doi: 10.1007/s40894-016-0024-1
Meredith, W. (1993). Measurement invariance, factor analysis and factorial invariance. Psychometrika 58, 525–543. doi: 10.1007/BF02294825
Mindt, M. R., Byrd, D., Saez, P., and Manly, J. (2010). Increasing culturally competent neuropsychological services for ethnic minority populations: a call to action. Clin. Neuropsychol. 24, 429–453. doi: 10.1080/13854040903058960
Oakes, W. P., Lane, K. L., Cox, M. L., and Messenger, M. (2014). Logistics of behavior screenings: How and why do we conduct behavior screenings at our school? Prev. Sch. Fail. 58, 159–170. doi: 10.1080/1045988X.2014.895572
Palmieri, P. A., and Smith, G. C. (2007). Examining the structural validity of the Strengths and Difficulties Questionnaire (SDQ) in a US sample of custodial grandmothers. Psychol. Assess. 19:189. doi: 10.1037/1040-3590.19.2.189
Putnick, D. L., and Bornstein, M. H. (2016). Measurement invariance conventions and reporting: The state of the art and future directions for psychological research. Dev. Rev. 41, 71–90. doi: 10.1016/j.dr.2016.06.004
Rosseel, Y. (2012). Lavaan: an R package for structural equation modeling and more. Version 0.5-12 (BETA). J. Stat. Softw. 48, 1–36. doi: 10.18637/jss.v048.i02
Ruchkin, V., Jones, S., Vermeiren, R., and Schwab-Stone, M. (2008). The Strengths and Difficulties Questionnaire: the self-report version in American urban and suburban youth. Psychol. Assess. 20:175. doi: 10.1037/1040-3590.20.2.175
Samejima, F. (1969). Estimation of latent ability using a response pattern of graded scores. Psychometrika 34, 1–97. doi: 10.1007/BF03372160
Schreiber, J. B., Nora, A., Stage, F. K., Barlow, E. A., and King, J. (2006). Reporting structural equation modeling and confirmatory factor analysis results: a review. J. Educ. Res. 99, 323–338. doi: 10.3200/JOER.99.6.323-338
Siceloff, E. R., Bradley, W. J., and Flory, K. (2017). Universal behavioral/emotional health screening in schools: overview and feasibility. Rep. Emot. Behav. Disord. Youth 17, 32–38.
Stone, L. L., Otten, R., Engels, R. C., Vermulst, A. A., and Janssens, J. M. (2010). Psychometric properties of the parent and teacher versions of the strengths and difficulties questionnaire for 4-to 12-year-olds: a review. Clin. Child Fam. Psychol. Rev. 13, 254–274. doi: 10.1007/s10567-010-0071-2
Teresi, J. A. (2006). Different approaches to differential item functioning in health applications: advantages, disadvantages and some neglected topics. Med. Care 44, S152–S170. doi: 10.1097/01.mlr.0000245142.74628.ab
Warne, R. T., Yoon, M., and Price, C. J. (2014). Exploring the various interpretations of “test bias”. Cult. Divers. Ethn. Minor. Psychol. 20:570. doi: 10.1037/a0036503
Wickersham, A., Sugg, H. V., Epstein, S., Stewart, R., Ford, T., Downs, J., et al. (2021). Systematic review and meta-analysis: the association between child and adolescent depression and later educational attainment. J. Am. Acad. Child Adolesc. Psychiatry 60, 105–118. doi: 10.1016/j.jaac.2020.10.008
Keywords: universal behavior screening, Strengths and Difficulties Questionnaire, measurement invariance, structural equation modeling, confirmatory factor analysis, item response theory
Citation: Graybill E, Barger B, Salmon A and Lewis S (2024) Gender and race measurement invariance of the Strengths and Difficulties Questionnaire in a U.S. base sample. Front. Educ. 9:1310449. doi: 10.3389/feduc.2024.1310449
Received: 12 October 2023; Accepted: 02 April 2024;
Published: 30 April 2024.
Edited by:
Kathleen Lane, University of Kansas, United StatesReviewed by:
Jeffrey M. DeVries, University of California, Irvine, United StatesCopyright © 2024 Graybill, Barger, Salmon and Lewis. This is an open-access article distributed under the terms of the Creative Commons Attribution License (CC BY). The use, distribution or reproduction in other forums is permitted, provided the original author(s) and the copyright owner(s) are credited and that the original publication in this journal is cited, in accordance with accepted academic practice. No use, distribution or reproduction is permitted which does not comply with these terms.
*Correspondence: Brian Barger, YmJhcmdlcjFAZ3N1LmVkdQ==
Disclaimer: All claims expressed in this article are solely those of the authors and do not necessarily represent those of their affiliated organizations, or those of the publisher, the editors and the reviewers. Any product that may be evaluated in this article or claim that may be made by its manufacturer is not guaranteed or endorsed by the publisher.
Research integrity at Frontiers
Learn more about the work of our research integrity team to safeguard the quality of each article we publish.