- 1School of Mathematics and Statistics, UNSW Sydney, Kensington, NSW, Australia
- 2School of Life and Environmental Sciences, University of Sydney, Sydney, NSW, Australia
- 3Data61, CSIRO, Eveleigh, NSW, Australia
Student evaluations of teaching (SET) have been examined over the years to better understand the student experience, with an increasing portion of literature exploring the presence of implicit bias in SET surveys against minority gender and ethnic lecturers. This study explores free-text comments made by students from a large public university in Australia over the period 2010–2016, using a semi-supervised statistical approach. Data were collected via surveys administered online at the end of each course to every student officially enrolled in that course via the learning management system, and completion of the surveys was voluntary. We build a probabilistic topic model which incorporates student and lecturer characteristics into the topic formation process. We make statistical inference on the effects of gender and cultural or language backgrounds based on the topic and word prevalence probabilities. The results showed clear separation of topics discussed between male and female lecturers. From a gendered perspective, our topic analyses have found that students are significantly more likely to critique female lecturers to improve on structural aspects of the course, as well as aspects of time management and control of the lecturing environment. In comparison, male lecturers were significantly more likely to be critiqued on specific aspects related to lecture delivery. Lecturers from non-English speaking backgrounds were more likely to be both critiqued and praised for the clarity of their delivery.
1 Introduction
Student evaluations of teaching (SET) surveys commonly include a free-text field for students to offer commentary on the teaching they have received in addition to numerical scores used to provide a rating for specific questions. Research has found that students provide valuable insights in the free-text fields that are consistent with their responses to the questionnaire and further elaborates on some areas of importance to the student (Brockx et al., 2012). These text responses provide us with a rich source of data and insight into the mindset of the students when they are completing these questionnaires. Increasingly, university administration is placing higher weight on these comments, with an expectation that lecturers formally address issues raised by students.
A number of studies analyzing numerical ratings from SET surveys have found evidence of gender bias where female lecturers receive lower ratings (Boring, 2017; Mengel et al., 2019; Fan et al., 2019). While others found gender bias in both directions (Aragón et al., 2023) depending on the students' expectations of gender roles, though Binderkrantz and Bisgaard (2022) found no overall gender bias, but a gender affinity effect, where students evaluate a teacher of their own gender best. These studies also find differences in the way female and male students evaluate teaching. Huang and Cai (2024) considered how student and instructor gender and under-represented minority status impact students' perception of teaching as related to diversity, and they found that students perceive more diversity-related materials taught by instructors with under-represented minority status.
Sprague and Massoni (2005) and Gelber et al. (2022) have argued that gender bias may not be easily detectable by quantitative data, and even when numerical responses do not show gender bias, text responses can show interesting gendered differences. In a study involving 288 college students, using a word frequency approach, Sprague and Massoni (2005) examined common words used to describe the best and worst teachers that students have had and found some revealing differences between male and female teachers. The findings for the best teachers had six of the top eight words in common across both genders—caring, understanding, intelligent, helpful, interesting, and fair. Men were more likely to be described as caring, understanding, and funny, while women were more often described as caring, helpful, and kind. This leads to the hypothesis that men are assessed on how they behave while women are judged on their actions, and more often than not need to prove themselves while men can be judged on their potential alone. Both men and women were criticized but to overcome these expectations requires more effort on the female's behalf. For example, male lecturers can clean up their content and with practice will improve their delivery and ability to engage, allowing them to recycle the content semester after semester; however, for women they would need to develop the relationship with each student and be as responsive to one student as another and hence there is no shortcut within or between semesters. This is also supported by Sigudardottir et al. (2022), whose qualitative study showed that male teachers received comments on subject knowledge while female teachers received comments more in terms of service to students.
Using qualitative methods involving a team of researchers to define a set of topics, Adams et al. (2021) found that male and female lecturers may be assessed differently and these surveys appear to measure conformity with gendered expectations. The authors found that men are being judged on their delivery of content and their ability to entertain while women are judged on their nurturing characteristics and relationship with students.
Gelber et al. (2022) used the Leximancer (https://mcrc.journalism.wisc.edu/files/2018/04/Manual_Leximancer.pdf) software to automatically extract concepts and themes from SET text data available from political science and international relations students at an Australian University. The authors focused only on students' responses to the best features of teaching and found it difficult to analyze responses to the question regarding how teaching can be improved, due to a lack of coherence within the text. Their analyses on best features found both male and female students evaluate female lecturers in similar ways but differ when they evaluate male lecturers. They also found when students discuss “help”, comments are related to gendered stereotypes. They argue that gender operated by producing subtle, but unequal, expectations on male and female lecturers.
An examination of student nominations of teaching excellence awards discovered that students were more likely to nominate lecturers who were the same gender as themselves, though male students were disproportionately unlikely to nominate female teachers (Kwok and Potter, 2021). According to Shifting Standards Theory (SST) (Biernat, 1995), this disproportionate male student-female lecturer nomination can be explained by male students holding female teachers to a higher standard of excellence than men and hence making it harder to recognize excellence in female teachers. Female students nominating female teachers were more likely to discuss themes related to “available” and “supportive”, while male students mentioned these terms less frequently for male teachers.
These earlier attempts to analyze SET comments are qualitative, often relying on a thematic approach, where the researchers define several themes, then rely heavily on manual analysis. Even when themes were obtained objectively, as in the case of Gelber et al. (2022), these approaches still heavily rely on human interpretation of gendered differences, and they cannot detect any statistical differences between genders, which cast doubt on whether these findings are reproducible and able to be generalized. For further discussions on generalization, see, for example, Valsiner (2019) and Gastaldi et al. (2015). Rigorous statistical analysis of the comments is challenging, both in terms of the large quantity of the available data, and the open nature of the comments. The need to incorporate metadata, such as gender and cultural backgrounds of lecturers and students, course, and program information adds an additional complexity to the statistical analysis.
In this study, we use the probabilistic topic model framework to test the hypotheses that (a) students evaluate male and female lecturers on different themes and (b) the languages/words used by male and female students are different for a given topic. The unsupervised topic model approach allows us to pool all the data from the underlying populations (of lecturer and student characteristics), leading to more accurate inference, while at the same time, the results will be less reliant on the subjective interpretation of the individual researcher. We analyzed the data on how to improve teaching separately to the best features of teaching, as topics found in the improvement data will likely lead the lecturer to address the issues and make changes, and such changes will in turn impact on the quality of the teaching as well as additional time spent by the lecturer on the course. Similarly, topics in the best features data will likely lead the lecturer to keep existing practice. In the rest of this study, we first give a brief description of the data used in the analysis, followed by the statistical modeling approach, and further details will be in Supplementary material. We then present our results, interpretations, and conclusions.
1.1 Data and method
We use data collected electronically at a large Australian university over a 7-year period from 2010 to 2016. Students were prompted in the SET survey to discuss the best features and areas for improvements required by their lecturer. The surveys were then linked with the student and lecturer information so as to produce covariate information for individual survey responses; we therefore have information on student's gender and program details, as well as lecturer gender and cultural backgrounds (an indicator combining both language and cultural background of the lecturer, see Fan et al., 2019 for details). The surveys are then de-identified and made available for this analysis. Five large faculties within the university were considered; these were Arts and Social Sciences (ART), Commerce (COM), Engineering (ENG), Medicine (MED), and Science (SCI).
We first consider the response students provided when prompted by the question “The lecturer's teaching could be improved by”. Linguistically, the responses to this question is different to the other free-text question “what was the best feature of the lecturer's teaching”, as the improvement question can lead to the use of negative verbiage when it in fact translates to a positive sentiment. For example, when students comment “nothing”, this suggests no improvements are required; however, when “nothing” is used in response to the best features question, it has a completely opposite meaning. For this reason, we will restrict our analysis to topic modeling (as opposed to sentiment analysis) and consider separate analyses for the improvement and best feature data. We expect that there will be overlapping topics between the two datasets, but the improvement topics are more likely to lead to lecturers making changes to the course, hence substantially impacting on both the time invested by the lecturer and the resulting quality of the course.
As mentioned earlier, the issue with the lecturer improvement comments is that students may not necessarily place comments of improvements, they might also state that there is “no improvements” or “the lecturer is great”, and hence the first step is to filter out those genuine suggestions of improvements from those students who were satisfied with the teaching they were provided. See Supplementary material (Methods I) for further details on data collection and how the comments were filtered. After filtering, the 68,020 comments (19,272 female lecturers and 21,170 from a non-English speaking background) classified as genuine improvements were then used in the subsequent analysis. For the best feature data, we did not need to perform any further cleaning other than removing all those without comments, converting text to lowercase, and removing all punctuation marks. This resulted in 119,665 comments (39,042 female lecturers and 35,452 from a non-English speaking background) for analysis. Finally, a breakdown of the staff and student demographic distributions in these two datasets can be found in Table 1.
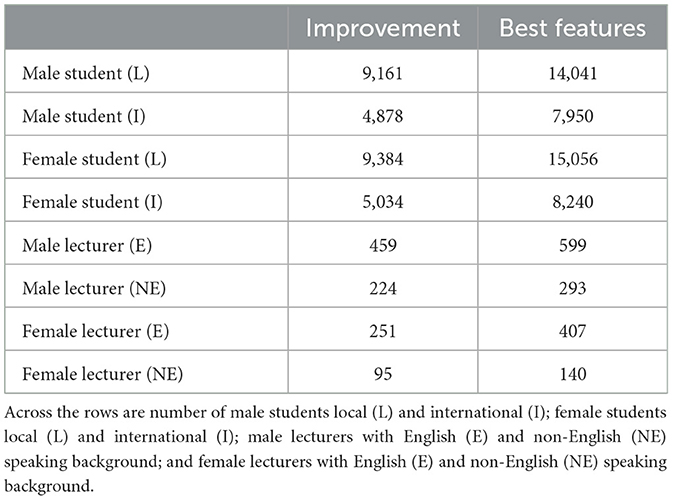
Table 1. Breakdown of unique number of staff and student by demographic for the improvement and best features comments datasets.
We then use the topic model, Latent Dirichlet Allocation (LDA) (Blei et al., 2003) as a basis for finding separate topics in the texts. This is an unsupervised mixed membership model that allows each document to take on multiple topics, unlike other clustering techniques. LDA is a three level hierarchical Bayesian model, where each document is modeled as a finite mixture over a latent set of topics and each topic is modeled as a mixture of topic words. Variational approximation and an expectation-maximization (EM) algorithm formed the basis for empirical Bayes parameter estimation. The topic probabilities assigned are a numerical representation of the original qualitative data indicating the likelihood of the corresponding topic. LDA preserves the necessary statistical relationships by identifying short descriptions of the members of a collection that enable efficient processing of large collections that can then be used for basic tasks involving detection, classification, and summarization of text.
In many instances, text data are also available with certain metadata, such as in our SET dataset where we have information on gender and cultural backgrounds of lecturers and students, this information could provide further valuable insight. In addition, naive fitting of the LDA model in an unbalanced dataset where, for example, there are far more male lecturers than females, would lead to topics dominated by comments for the male lecturer. One approach is to separately fit topic models for each demographic category, as is often the approach in the SET literature. However, there are several short-comings with such an approach. First, separately obtained topics can be difficult to analyze and compare; thus, statistical inferences are not possible. Second, topic modeling might return poor results when sample sizes are reduced due to the splitting of the data (this would be the case when there are multiple groups). We therefore advocate incorporating covariates directly into the topic model to allow us to borrow information across the documents. Structural topic model (STM) (Roberts et al., 2014, 2016) incorporates the covariates to LDA at both the topic and word level, by modeling the topic proportions (or topical prevalence as it is referred to in STM) parameters θ and word frequency (or topical content) parameters β as functions of covariates. Additional details of the LDA and STM framework are given in Supplementary material (Methods II and III).
To build the topic model which takes into account the metadata of the document, the STM package in R was utilized (Roberts et al., 2019). The topic covariates we considered were lecturer gender (flag for female), lecturer culture (flag for non-English speaking background), and a flag for the faculties, and this allows us to specify how these covariates influence the different topics. The content covariates contain four levels of the student characteristics—male local, male international, female local, and female international—as the student characteristics would influence the word choice for each particular topic. Further details on the implementation of STM, including a permutation test, can be found in Supplementary material (Methods III).
2 Results and discussion
2.1 What are the students critiquing?
After examining the output from STM, and reviewing the top terms and documents corresponding to each topic, we label each topic as follows: Topic 1 Communication is related to communication with students in the approach taken to encourage participation, speaking/asking questions, and providing explanations. Topic 2 Lecture Structure & Environment covers the structure of the lecture and the learning environment created for the students. Topic 3 Lecture Slides is related to the formatting of slides and the physical presentation of information. Topic 4 Time Management covers all aspects related to the lecturer's ability to arrive on time and manage the timing of the contents within the lecture. Topic 5 Lecture Delivery is quite broad covering all aspects of the lecture delivery, particularly related to the ability to explain concepts clearly in terms of pace and detail/information of supporting notes. Topic 6 Control is related to the lecturer's ability to control disruptions during class (i.e., other student's talking). Topic 7 Examples covers the examples covered in the tutorial and practice examples for assessments and exams. To validate the labeling, we randomly sample 100 documents, and these labels produced an accuracy of 82%, which corresponds to the dominant topic falling appropriately under the topic labels assigned. Table 2 presents the most prominent topics identified by STM, together with the corresponding most frequently occurring topic words. Topic labeling is done manually based on the topic words and their context in the data.
The discussion to follow only addresses covariates that were found to be significant at the 95% level of significance, see Supplementary Table S1 where entries with an asterisk indicate the variables which performed well under the permutation test; hence, we have a higher level of confidence that these results are not just due to chance. Students were more likely to comment on Topics 2, 4, and 6 (lecture structure/environment, time management, and control of the class) for female lecturers, which included comments on the structure and content of the course, management of time that includes allocation of time during discussions and group work, and crowd control. The comments are more on supporting functions that facilitate the teaching. In contrast, students were more likely to discuss Topics 1, 5, and 7 (communication, lecture delivery, and examples) for male lecturers. These topics cover the mode and manner of communication, specifics of lecture delivery, and comments related to examples and assessments. These topics tend to be more directly associated with teaching and as such easier to action upon. Finally, students were equally likely to comment on topic 3, concerning lecture slides and presentation of notes.
These results demonstrate that students focus on different topics when discussing male and female lecturers, and their gendered expectations of lecturers may be a factor, for example, time management skills (Topic 4), which is a typical female associated characteristic (Boring, 2017), and classroom control (Topic 6), where students request the lecturer to be “tougher on students who consistently talk during the lecture”, “kicking rude people out of lectures”, and having “more control of loud and distracting students in the lecture”. This can be both a reflection of an actual unruly classroom, potentially caused by students not recognizing the female lecturers authority, or a perceived lack of authority in females even when the class was in fact well-organized. There were some comments that suggested that tougher female lecturers were not too well-received with comments such as “she was too intimidating and needs to be more approachable” and “needs a more personal interaction and connection to students”. Thus, it is a fine line between lacking control of the classroom and being too strict and cold, suggesting efforts to improve these perceived lack of control situations may not be easily achievable, and depends also on the students behavior. However, improvement comments for male lecturers are more within their direct control and thus actionable, and some of those comments included writing “words more clearly”, “the way he wrote draft and notes on computer-aided worksheet is not satisfactory”, and “more student involvement would aid learning”.
Non-English speaking lecturers were more likely to receive comments on Topic 5 (lecture delivery) and less likely to be critiqued on Topic 4 (time management) and Topic 6 (control) of the class compared to their English native speaking colleagues. The lecture delivery topic incorporates the pace and clarity with which a lecturer speaks and some comments made by students also make mention of the accent of the lecturer. For example, a student complained there was a “bit of a communication barrier sometimes hard to understand her accent but that can't be fixed”, while other students saw the accent as something that can and ought to be changed with suggestions such as “less accent” and “improvement in accent required”. Thus, all things being equal between two lecturers, and it appears that an accent may hinder their perceived teaching effectiveness. Furthermore, even if students do not make mention of an accent explicitly, they still critique if they “can not understand his english” and might also avoid making note of the accent by instead emphasizing the need to improve “clarity and depth” and “explain concepts more clearly”. As accents are normally drawn to attention if they differ from the accent of the nation in which the teaching occurs, this may be a factor contributing to lower numerical ratings awarded to lecturers from a non-English speaking background, as was found in Fan et al. (2019). Again, changing one's accent is not easily achievable.
The student characteristics were set as the topical content variable to examine the different word usage by the different student groups; however, none of these student characteristics were significant for any of the topics. We also found some small variations between the different faculties, although only the ART faculty was significantly more likely to talk about Topic 6 (control). This may be more of an importance in ART as there tends to be more group discussions and sharing of ideas, for example, as student commented “i think a control on the group discussion, sometimes i felt the group discussions were really just individuals talking and i don't know if it was just my tut group but i felt like anything i had to say was shot down as not important by the group which in effect made me not want to contribute”.
2.2 Comparison with best feature comments
A similar approach was carried out for the analysis of the best features comments to explore whether there are also gendered and/or cultural differences in the topics students praise their lecturer on. The most frequently appearing topic words and representative documents for each topic are displayed in Table 3. After analysis of the top terms and documents corresponding to each topic, the labels provided to each topic are as follows: Topic 1 Engagement & Preparedness is related to the lecturer's ability to engage the students and deliver material in a well-structured manner, clearly demonstrating to students they have prepared for the class. Topic 2 Explanation covers the ability of the lecturer to explain the topics, covering clear explanation in delivery and in lecture notes and materials provided to students. Topic 3 Availability is related to the availability of the lecturer to answer student questions and the willingness to do so (e.g., dedicated office hours). Topic 4 Entertainment covers all aspects related to the design of the delivery and material that students were able to positively respond to and be excited by. Topic 5 Relevance captures the ability of the lecturer to translate the theory to the real world and allow the students to grasp the relevance of what they are learning with appropriate examples. Topic 6 Assessment is related to the clear communication of assessment tasks, prompt feedback, and the overall support provided to students in relation to the assessable contents of a course. Topic 7 Interest covers the ability of their lecturer to spark interest in the students to participate in the discussion and share in their passion for the subject matter. Topic 7 also covers how much the students like their lecturer in general, for example, describing their lecturer as good, great, awesome etc. Again, based on a random sample of 100 documents, these labels produced an accuracy of 77%. These topics show much overlap from the literature where such text data were analyzed, Sprague and Massoni (2005), Gelber et al. (2022), and Adams et al. (2021), further validating that the topic modeling approach produced sensible results.
The discussion to follow only addresses covariates that were found to be significant at the 95% level of significance, see Supplementary Table S2. The output shows that students are more likely to praise female lecturers on Topics 1, 2, and 6, which relate to their engagement and preparation, explanations, and the quality of assessments. For example, students provided the following praises for their female lecturer ability to explain concepts by “going through the lecture slides and concepts in depth, and having class demonstrations for clarity”, “effective teaching, willing to demonstrate practically, good personality”, “she explained everything manually”. Students also praised the female lecturers for their willingness to help, with comments like “her willingness to listen and accommodate for whatever was thrown at her!”, “always willing to help, goes through content thoroughly for those who need extra help understanding”, and “excellent responsiveness and willingness to help out students. I particularly recall an incident where an assignment submission was due at midnight and was present on open learning answering questions and helping students with submissions at times on wards of 11 p.m. Excellent dedication to the student body of the course”.
Meanwhile, male lecturers are more likely to receive praise on their entertainment factor and their ability to connect the material with the real world. Students praised the engagement with comments like “he made the content particularly engaging by providing personal and/or historic examples”, “tried to make lectures interesting through a sense of humor and perspectives and was very interested in the material himself, which reflected in the quality of teaching”, “is an engaging storyteller and is clearly knowledgeable and passionate about the topic area; he is a master at imparting complex concepts by embedding them within memorable anecdotes. Always approachable and quick to respond to student queries”, and “he was engaging and was passionate about the subject material. His use of real-world examples was helpful in generating interest about some of the less interesting subject material”.
Lecturers from a non-English speaking background were more likely to be praised for their explanation, availability, and assessment guidance. For example, “he explained very clear and can make people (me for example) interested to this course as he really into this course :) the way he teach is very comfortable” and “he can answer all questions quickly and clearly, more over, he is patient to answer online”. Interestingly, the students praised lecturers with non-English speaking backgrounds for their explanation and delivery, while simultaneously asked for improvement in the lecture delivery topic (the two topics broadly overlap), a potential explanation is that perhaps non-native speakers may have put additional effort in trying to be clearer in their explanations, while at the same time perceived difficulty in the usage of English for a non-native speaker brings attention to the perceived quality of the lecture delivery.
Lecturers from an English speaking background were more likely to be commented on their entertainment, relevance, and being interesting, with comments such as “Fun, Interesting, Informative, Enthusiastic”, “her notes were really helpful and quite in depth. She explained things clearly and precisely. Her lectures were really interactive and interesting as she made students interact by coming up to demonstrate certain examples”, and “very interactive with the students and attempted to make less stimulating concepts really interesting”.
There were some significant student characteristics, between female international students (baseline) and male local students, within the best feature STM output, see Supplementary Table S3. Plots of the common words used by the various student groups for the significant Topics 1 (engagement/preparedness), 2 (explanation), 5 (relevance), and 7 (interest) were examined. For Topic 1, where female lecturers are more affected, female international students were more focused on the lectures and how prepared the lecturers were while male local students were more concerned with the engagement and content, see Supplementary Figure S2A. For Topic 2, where the comments are more likely related to female lecturers and lecturers with non-English speaking backgrounds, female international students comment on clarity (“clear”) in general while male local students comment on the materials, notes, and explanations. For the students discussing Topic 5, where the topics are more likely for male and lecturers with English speaking background, male local students discussed “examples” while female international students use the word “us” perhaps suggesting the relevance needed to be tied directly to them in some manner as opposed to broader examples, see Supplementary Figure S2C. For Topic 7, primarily concerning lecturers with English language background, female international students comment on the patience of the lecturer while on the other end male local students are focused more on the knowledge possessed by the lecturer, see Supplementary Figure S2D.
3 Limitations
We note that our study is based on observational data, and statistical analyses have been based only on the sample of students who responded to the SET survey and left comments. Different students will have different probabilities of doing so, depending on various factors. As such our findings should not be generalized to the whole student population, which includes those students who do not respond to survey or those who respond but do not leave comments. Interpretation of our results is conditional on the student having left a text comment. See, for example, Imbens and Rubin (2015) and Pearl (2009), for detailed discussions and potential solutions. Future study will attempt to generalize findings to the entire student population, and it will be interesting to find differences between the self-selected population who contribute text comments, to the non-response population.
While we have limited our study to understanding the association between gender characteristics with the topics, additional covariates could be added to the model, at some increased computational cost. Adding additional covariates could provide further insight and would be of interest in the future studies.
Finally, analysis of large corpus of text data is a difficult task. We acknowledge that the STM model is not perfect, and in particular, topic labeling was carried out manually. The procedure can be influenced by the authors' own interpretation of the words and context.
4 Conclusion
Student comments on how lecturers can improve their teaching is particularly important because lecturers are increasingly being asked by their employer to address student concerns. Previous studies have either combined the best features with improvement, or only focused on best features. The pooling of the two datasets likely lead to the best features dominating improvement comments as there are typically fewer comments in the improvement dataset. As far as we are aware, this is the first study that analyses improvement comments in detail. Our approach relies on the structural topic modeling framework that allows us to make inferential statements about gender or cultural effects on the prevalence of a topic. We have identified clearly distinctive topics driven by gender and culture of lecturers.
Overall, we have found that female lecturers have been asked to improve on topics which may not be entirely within their control, such as lecture environment and crowd control (i.e., time management and controlling classroom disruptions) which may relate to the perceived lack of authority in female lectures. While male lecturers were asked to improve on specific, and often, more actionable items, such as the pace, explanations, and quality of notes of the lecture delivery.
Furthermore, it seems that lecturers from a non-English speaking background are more likely to be critiqued in terms of their lecture delivery which is related to the pace, clarity of speech, and unfortunately accent which they cannot easily alter, but on the other hand, they also receive positive comments from the best features comments on the clarity of their explanation, suggesting that these lecturers may be aware of the potential negative effect of their accent, and compensate for it by making an extra effort to be clear. Non-native speakers, as well as female lecturers, are also perceived as less entertaining, with the male and native speakers praised for their ability to make the material feel relevant and engaging.
The findings from this study clearly highlight the different ways in which students praise and critique their lecturers' teaching, and in some cases, students may ask improvements on things that may not be easily actionable, making it difficult for some lecturers to improve their SET ratings. Lecturers are increasingly expected to respond to student comments (sometimes publicly), and any potential implicit biases should be considered by anyone using SET.
Data availability statement
The data analyzed in this study is subject to the following licenses/restrictions: the dataset can be made available upon request. Requests to access these datasets should be directed to yanan.fan@data61.csiro.au.
Ethics statement
The studies involving humans were approved by UNSW Human Research Ethics Advisory Panel (HREAP), HC17088. The studies were conducted in accordance with the local legislation and institutional requirements. Written informed consent for participation was not required from the participants or the participants' legal guardians/next of kin in accordance with the national legislation and institutional requirements.
Author contributions
FK: Conceptualization, Data curation, Formal analysis, Investigation, Methodology, Software, Validation, Visualization, Writing – original draft, Writing – review & editing. EJ: Conceptualization, Formal analysis, Funding acquisition, Investigation, Methodology, Project administration, Resources, Supervision, Visualization, Writing – original draft, Writing – review & editing. YF: Conceptualization, Data curation, Formal analysis, Funding acquisition, Investigation, Methodology, Project administration, Resources, Supervision, Validation, Visualization, Writing – original draft, Writing – review & editing.
Funding
The author(s) declare financial support was received for the research, authorship, and/or publication of this article. FK was able to conduct this research with support from the Australian Government Research Training Program (RTP) and UNSW's School of Mathematics and Statistics scholarship.
Conflict of interest
The authors declare that the research was conducted in the absence of any commercial or financial relationships that could be construed as a potential conflict of interest.
Publisher's note
All claims expressed in this article are solely those of the authors and do not necessarily represent those of their affiliated organizations, or those of the publisher, the editors and the reviewers. Any product that may be evaluated in this article, or claim that may be made by its manufacturer, is not guaranteed or endorsed by the publisher.
Supplementary material
The Supplementary Material for this article can be found online at: https://www.frontiersin.org/articles/10.3389/feduc.2024.1296771/full#supplementary-material
References
Adams, S., Bekker, S., Fan, Y., Gordon, T., Slavich, E., Shepherd, L. J., et al. (2021). Gender bias in student evaluations of teaching: ‘Punish[ing] Those Who Fail To Do Their Gender Right. Higher Educ. 83, 787–807. doi: 10.1007/s10734-021-00704-9
Aragón, O. R., Pietri, E. S., and Powell, B. A. (2023). Gender bias in teaching evaluations: the causal role of department gender composition. Proc. Nat. Acad. Sci. U. S. A. 120:e2118466120. doi: 10.1073/pnas.2118466120
Biernat, M. (1995). “The shifting standards model: implications of stereotype accuracy for social judgment,” in Stereotype Accuracy: Toward Appreciating Group Differences, eds Y.-T. Lee, L. J. Jussim, and C. R. McCauley (American Psychological Association), 87–114. doi: 10.1037/10495-004
Binderkrantz, A. S., and Bisgaard, M. (2022). A gender affinity effect: the role of gender in teaching evaluations at a danish university. PsyArXiv. doi: 10.31234/osf.io/bx6j3
Blei, D. M., Ng, A. Y., and Jordan, M. I. (2003). Latent Dirichlet allocation. J. Mach. Learn. Res. 3, 993–1022.
Boring, A. (2017). Gender biases in student evaluations of teaching. J. Public Econ. 145, 27–41. doi: 10.1016/j.jpubeco.2016.11.006
Brockx, B., Van Roy, K., and Mortelmans, D. (2012). The student as a commentator: students' comments in student evaluations of teaching. Proc. Soc. Behav. Sci. 69, 1122–1133. doi: 10.1016/j.sbspro.2012.12.042
Fan, Y., Shepherd, L. J., Slavich, E., Waters, D., Stone, M., Abel, R., et al. (2019). Gender and cultural bias in student evaluations: why representation matters. PLoS ONE 14:e0209749. doi: 10.1371/journal.pone.0209749
Gastaldi, F. G. M., Longobardi, C., Quaglia, R., and Settanni, M. (2015). Parent-teacher meetings as a unit of analysis for parent-teacher interactions. Cult. Psychol. 20:95–110. doi: 10.1177/1354067X15570488
Gelber, K., Brennan, K., Duriesmith, D., and Fenton, E. (2022). Gendered mundanities: gender bias in student evaluations of teaching in political science. Aust. J. Polit. Sci. 57, 199–220. doi: 10.1080/10361146.2022.2043241
Huang, S., and Cai, L. (2024). Cross-classified item response theory modeling with an application to student evaluation of teaching. J. Educ. Behav. Stat. 49, 311–341. doi: 10.3102/10769986231193351
Imbens, G. W., and Rubin, D. B. (2015). Causal Inference for Statistics, Social, and Biomedical Sciences: An Introduction. Cambridge: Cambridge University Press.
Kwok, K., and Potter, J. (2021). Gender stereotyping in student perceptions of teaching excellence: applying the shifting standards theory. High. Educ. Res. Dev. 41, 2201–2214. doi: 10.1080/07294360.2021.2014411
Mengel, F., Sauermann, J., and Zölitz, U. (2019). Gender bias in teaching evaluations. J. Eur. Econ. Assoc. 17, 535–566. doi: 10.1093/jeea/jvx057
Pearl, J. (2009). Causal inference in statistics: an overview. Stat. Surv. 3, 96–146. doi: 10.1214/09-SS057
Roberts, M. E., Stewart, B. M., and Airoldi, E. M. (2016). A model of text for experimentation in the social sciences. J. Am. Stat. Assoc. 111, 988–1003. doi: 10.1080/01621459.2016.1141684
Roberts, M. E., Stewart, B. M., and Tingley, D. (2019). stm: an R package for structural topic models. J. Stat. Softw. 91, 1–40. doi: 10.18637/jss.v091.i02
Roberts, M. E., Stewart, B. M., Tingley, D., Lucas, C., Leder-Luis, J., Gadarian, S. K., et al. (2014). Structural topic models for open-ended survey responses. Am. J. Pol. Sci. 58, 1064–1082. doi: 10.1111/ajps.12103
Sigudardottir, M. S., Rafnsdottir, G. L., Jónsdóttir, A. H., and Kristofersson, D. M. (2022). Student evaluation of teaching: gender bias in a country at the forefront of gender equality. High. Educ. Res. Dev. 42, 954–967. doi: 10.1080/07294360.2022.2087604
Sprague, J., and Massoni, K. (2005). Student evaluations and gendered expectations: what we can't count can hurt us. Sex Roles 53, 779–793. doi: 10.1007/s11199-005-8292-4
Keywords: higher education, gender bias, text analysis, topic models, SET surveys
Citation: Kim F, Johnston EL and Fan Y (2024) A topic model analysis of students' gendered expectations using surveyed critiques of lecturers. Front. Educ. 9:1296771. doi: 10.3389/feduc.2024.1296771
Received: 19 September 2023; Accepted: 20 August 2024;
Published: 16 September 2024.
Edited by:
Nishtha Lamba, Middlesex University Dubai, United Arab EmiratesReviewed by:
Jessica LaPaglia, Morningside College, United StatesSijia Huang, Indiana University, United States
Copyright © 2024 Kim, Johnston and Fan. This is an open-access article distributed under the terms of the Creative Commons Attribution License (CC BY). The use, distribution or reproduction in other forums is permitted, provided the original author(s) and the copyright owner(s) are credited and that the original publication in this journal is cited, in accordance with accepted academic practice. No use, distribution or reproduction is permitted which does not comply with these terms.
*Correspondence: Yanan Fan, yanan.fan@data61.csiro.au