- 1Department of Medical Social Sciences, Northwestern University Feinberg School of Medicine, Chicago, IL, United States
- 2Bill & Melinda Gates Foundation, Seattle, WA, United States
- 3Department of Psychological and Brain Sciences, George Washington University, Washington, DC, United States
- 4Counseling Psychology and Human Services, University of Oregon College of Education, Eugene, OR, United States
- 5Irving Medical Center, New York State Psychiatric Institute, Columbia University, New York, NY, United States
- 6Johns Hopkins Bloomberg School of Public Health, Baltimore, MD, United States
- 7Community Environmental Health Program, College of Pharmacy, University of New Mexico Health Sciences Center, Albuquerque, NM, United States
- 8University of North Carolina School of Medicine, Chapel Hill, NC, United States
- 9Anita Zucker Center for Excellence in Early Childhood Studies, University of Florida, Gainesville, FL, United States
- 10Department of Preventative Medicine, University of Tennessee Health Science Center, Memphis, TN, United States
- 11Department of Psychiatry, University of California, San Francisco, San Francisco, CA, United States
Introduction: National health policies to stop the spread of the COVID-19 virus in the US resulted in widespread school closures and disrupted learning in Spring 2020.
Methods: This study draws on unique individual-level data from n = 282 5–12 year olds enrolled in the NIH Environmental influences on Child Health Outcomes (ECHO) Research Program to investigate associations between caregiver-reported duration of Spring 2020 learning disruptions and academic achievement.
Results: Linear regression analyses estimated that children who experienced more than 4 weeks of instruction disruptions in Spring 2020 scored 4.5 points [95% CI: −8.77, −0.22] lower on age-normed math assessments compared to peers who had four or fewer weeks of disruption, adjusting for sociodemographic variables, pre-pandemic vocabulary, and COVID-19 family hardships and stress. No differences were found for reading. Children whose caregivers had higher levels of pandemic-related traumatic stress and lower educational attainment also had lower math scores, adjusting for all other covariates.
Discussion: Results suggest educators and schools focus additional attention on supporting math instruction for children who experienced extended learning disruptions.
1 Introduction
COVID-19-related school closures affected more than 90% of children worldwide (UNESCO, 2021), including over 55 million in the US (Peele et al., 2021). With the unexpected nature of the pandemic and subsequent closures, schools varied in how and when they made the transition from in-person to remote instruction. For more than a quarter of US school districts, it took over 3 weeks to begin remote learning (Malkus, 2020), and 1 month into school closures, only 37% districts were providing a formal remote learning curriculum with instruction (Lake and Dusseault, 2020). Understanding whether and to what extent differences in how long schools did not provide instruction of any kind (remote or in-person) impacted student achievement is critical for informing pandemic recovery efforts.
1.1 Potential impact of COVID-19 learning disruptions on academic achievement
Emerging work on COVID-19 learning disruptions primarily drawing on group-level data points to average group-level negative pandemic-related effects on achievement of 0.08–0.18 standard deviations, with generally worse outcomes in math compared to reading; disparities by socioeconomic status, race, ethnicity, and grade level have also been noted (for reviews, see Hammerstein et al., 2021 Zierer, 2021; Cohodes et al., 2022; König and Frey, 2022; Betthäuser et al., 2023). For example, Molnár and Hermann (2023) found Hungarian children in schools with higher proportions of low-SES students experienced little or no academic progress during pandemic-related school disruptions, replicating work by Engzell et al. (2021) who showed Dutch students, particularly those from lower SES households, made no gains on standard exams following COVID-19 school disruptions. In the US, most studies have been limited to school-level poverty indicators, with students in high-poverty schools more negatively impacted compared to those in low-poverty schools (e.g., Goldhaber et al., 2022a,b; Kuhfeld et al., 2022a, 2023). Findings by race and ethnicity suggest US Black and Hispanic students experienced greater declines in achievement compared to White and non-Hispanic students (e.g., Goldhaber et al., 2022a,b; Kuhfeld et al., 2022a,b, 2023). Several international studies also suggest younger students were harder hit by the pandemic (e.g., Tomasik et al., 2021; Molnár and Hermann, 2023). In the US, large-scale studies primarily focus on grades 3–8 and report 3–5th graders had larger declines in achievement compared to 6–8th graders (e.g., Goldhaber et al., 2022a,b; Kuhfeld et al., 2022a, 2023).
Despite concordance of emerging evidence, existing work is limited in its generalizability and specificity. Most studies have been conducted outside the US and with more homogenous samples, drawing on group-level historical comparisons of achievement trends without adjustment for important individual-level confounders that may help explain variation in outcomes and identify alternative intervention targets beyond remedial education (Hammerstein et al., 2021; König and Frey, 2022; Betthäuser et al., 2023). Even the most rigorous US studies that include individual-level achievement data are limited because they all use the same dataset (MAP Growth assessment data) and lack important individual-level sociodemographic and contextual factors (e.g., family stressors). This results in conclusions that attribute any change over time to the pandemic or, in some cases, school-level characteristics, even if such differences may be due to more proximal individual-level differences. For example, Kuhfeld et al. (2022b) evaluated within-child changes in achievement from Fall 2019 to Spring 2021 for 5 million 3rd–8th graders in US public schools and differences by school-and district-level racial composition but could not evaluate whether changes in achievement differed by individual-level SES or adjust for any other individual-level covariates. Goldhaber et al. (2022a) extended this work to evaluate individual-level achievement changes through Fall 2021 and include individual-level data on race but were similarly limited by the lack of individual-level SES. In a follow-up study, the authors acknowledge lacking the necessary data to evaluate additional factors, such as family stressors outside of the school environment, which might explain the significant relationship between school closures and achievement (Goldhaber et al., 2022b). The most recent meta-analysis of 42 international studies reported most extant work was at serious or critical risk of bias due to confounding (Betthäuser et al., 2023). Much of the variance in achievement outcomes remains unaccounted for and attributed to pandemic-related differences when other factors such as SES and family stress may be driving such differences. Thus, despite the large sample sizes and robust methodological frameworks utilized in a handful of prior studies, there remains a dearth of research that incorporates critical individual-level factors.
To overcome these limitations, the current study leverages individual-level data from a diverse sample of 5–12-year-old US children to investigate associations between the duration of Spring 2020 COVID-19 learning disruptions and student achievement. We hypothesized longer learning disruptions in Spring 2020 where students received no formal instruction of any kind, whether in-person or remote, would be associated with worse math and reading achievement compared to shorter learning disruptions. Additionally, we conducted exploratory analyses of effect modification by child and family characteristics to understand whether learning disruptions differentially impacted achievement outcomes based on such factors.
2 Materials and methods
Data came from the Environmental influences on Child Health Outcomes (ECHO) research program. An NIH-funded research consortia comprised of existing longitudinal observational pediatric cohorts, ECHO aims to evaluate a range of early environmental exposures (e.g., biological, chemical, social) on five child outcomes: pre/peri/postnatal (e.g., preterm birth), airways (e.g., asthma), obesity, neurodevelopment, and positive health (e.g., well-being; LeWinn et al., 2022; Knapp et al., 2023).
2.1 Participants
Two ECHO cohorts contributed data as part of a COVID-19 supplemental award. The Conditions Affecting Neurocognitive Development and Learning in Early childhood (CANDLE) cohort enrolled pregnant individuals at four hospitals in Memphis, TN between 2006 and 2011. Participants in the CANDLE study reflect the demographics of Shelby County, TN, with the majority of caregivers identifying as Black (63%) and having lower income (64% < $50,000/year; Sontag-Padilla et al., 2015; LeWinn et al., 2020). The Brown University Assessment of Myelination and Behavioral development Across Maturation (BAMBAM) cohort was designed as an accelerated longitudinal study with a community-based sample of healthy children from the Providence, RI area. Recruited began in 2010, with approximately half enrolled between 2 and 8 months of age and half between 2 and 4 years of age (Deoni et al., 2012). The subsample of participants included in this study (CANDLE: n = 151; BAMBAM: n = 131) were those with data on the primary exposure (COVID-19 instruction disruption) and outcome (AAB). Primary caregivers consented into ECHO and provided consent for their children to participate. Local Institutional Review Boards (IRB) and the ECHO central IRB approved all data collection procedures.
2.2 Measures
2.2.1 Academic achievement
Cohorts administered two subtests from the Academic Achievement Battery (AAB; Messer, 2014) to children in-person. The Letter/Word Reading subtest evaluates components of basic reading skills, including letter identification and word pronunciation (Cronbach’s 𝛼 = 0.94), and the Mathematical Calculation subtest assesses basic math skills by asking participants to provide oral and written responses to math problems and calculations (Cronbach’s 𝛼 = 0.92). The AAB is age-and grade-normed and aligns with national academic standards (e.g., Common Core). For this study, age-based norms were used. We restricted data to AAB scores obtained after the end of the 2019/2020 school year (i.e., post-Spring 2020), defined as on or after May 23rd, 2020, for the CANDLE cohort based on the Shelby County School District calendar, the county in which CANDLE participants live and go to school; and on or after June 26th, 2020, for the BAMBAM cohort based on the Rhode Island Public School calendar, which applies to all public schools in the state. To account for the n = 19 children from BAMBAM with more than one AAB administration during the study period, we selected scores from the assessment closest to June 26th, 2020. No repeated AAB administrations were present for CANDLE. This resulted in AAB assessments occurring between October 2020 and March 2022 (Figure 1). We also derived a variable describing the number of months from end of Spring 2020 to AAB, reflecting the time between when schools ended their school year (as described above) to when children completed the AAB.
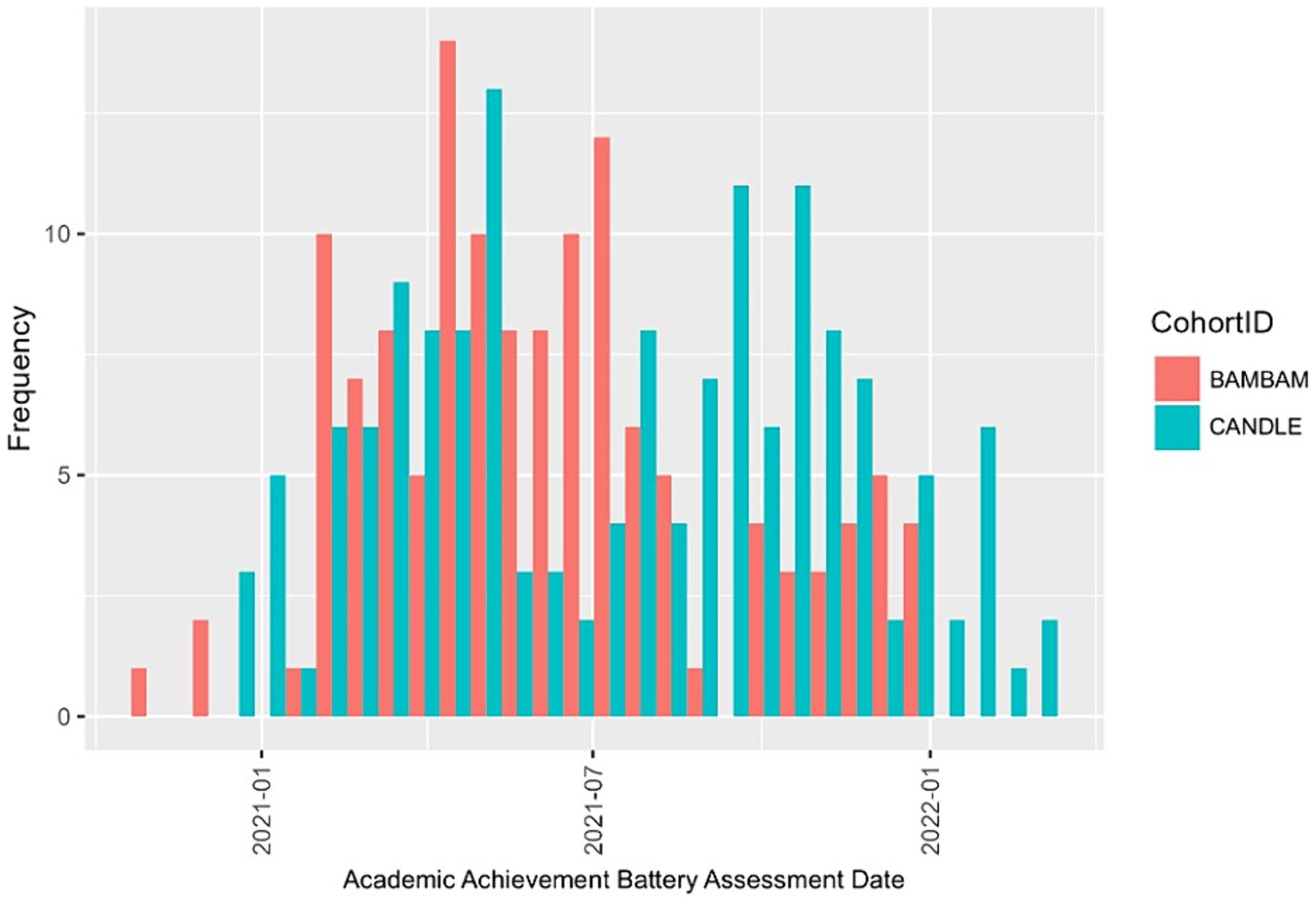
Figure 1. Academic achievement battery assessment date distribution by Cohort. Distribution of academic achievement battery assessment dates by ECHO Cohort. The first assessment occurred in October 2020 and the last assessment occurred in March 2022.
2.2.2 Spring 2020 instruction disruption
Caregivers reported the number of weeks their child’s school was closed to instruction of any kind—in-person or remote—in Spring 2020. This represented the time between when the child’s school building physically closed and when learning resumed in-person or remotely in Spring 2020. Response options included: no break between when the child’s school closed and when instruction resumed, less than 1 week, 1 week, 2 weeks, 3 weeks, 4 weeks, and more than 4 weeks. We derived a dichotomous variable where >4 weeks closed = 1 (“extended disruption” group) and 4 weeks = 0 (“non-extended disruption” group). We selected this threshold because anything less is similar to the cumulative standard breaks students experience throughout the school year, such as winter and spring vacations. Additionally, missing school for >4 weeks represents missing 11% or more of the standard 180 instructional days per school year required in most states (including Tennessee and Rhode Island), which is just above the general 10% threshold used for chronic absenteeism in the US (Lara et al., 2018).
2.2.3 Pre-pandemic academic functioning
In the absence of pre-pandemic AAB data, we controlled for pre-pandemic vocabulary scores, as vocabulary is a predictor of both reading and math achievement (Bleses et al., 2016). For most children (n = 206), vocabulary was measured using the NIH Toolbox Picture Vocabulary subtest (Gershon et al., 2013), and another 23 children had data on the Wechsler Intelligence Scale for Children, 5th Edition (WISC-5) Vocabulary subtest (Wechsler, 2014). Both instruments are norm-referenced to the general US population, use image-based stimuli, and correlated r = 0.72 for children in this sample with data on both measures (n = 42), reflecting excellent convergent validity (Weintraub et al., 2013). We therefore combined these data to maximize sample size. We linearly transformed vocabulary scores from T-scores to standard scores (standard = [T-50]/10*15 + 100) used by the AAB. We restricted vocabulary data to those completed on or before March 12th, 2020, reflecting the last day before the declaration of a national US emergency when schools in Shelby County and Rhode Island closed. We selected the assessment closest to March 12th, 2020 if children had more than one, resulting in assessments occurring between December 1, 2015 and March 7, 2020 (Figure 2). To account for differences in the time between the vocabulary assessment and AAB administration, we derived and controlled for a variable quantifying the number of months between assessments.
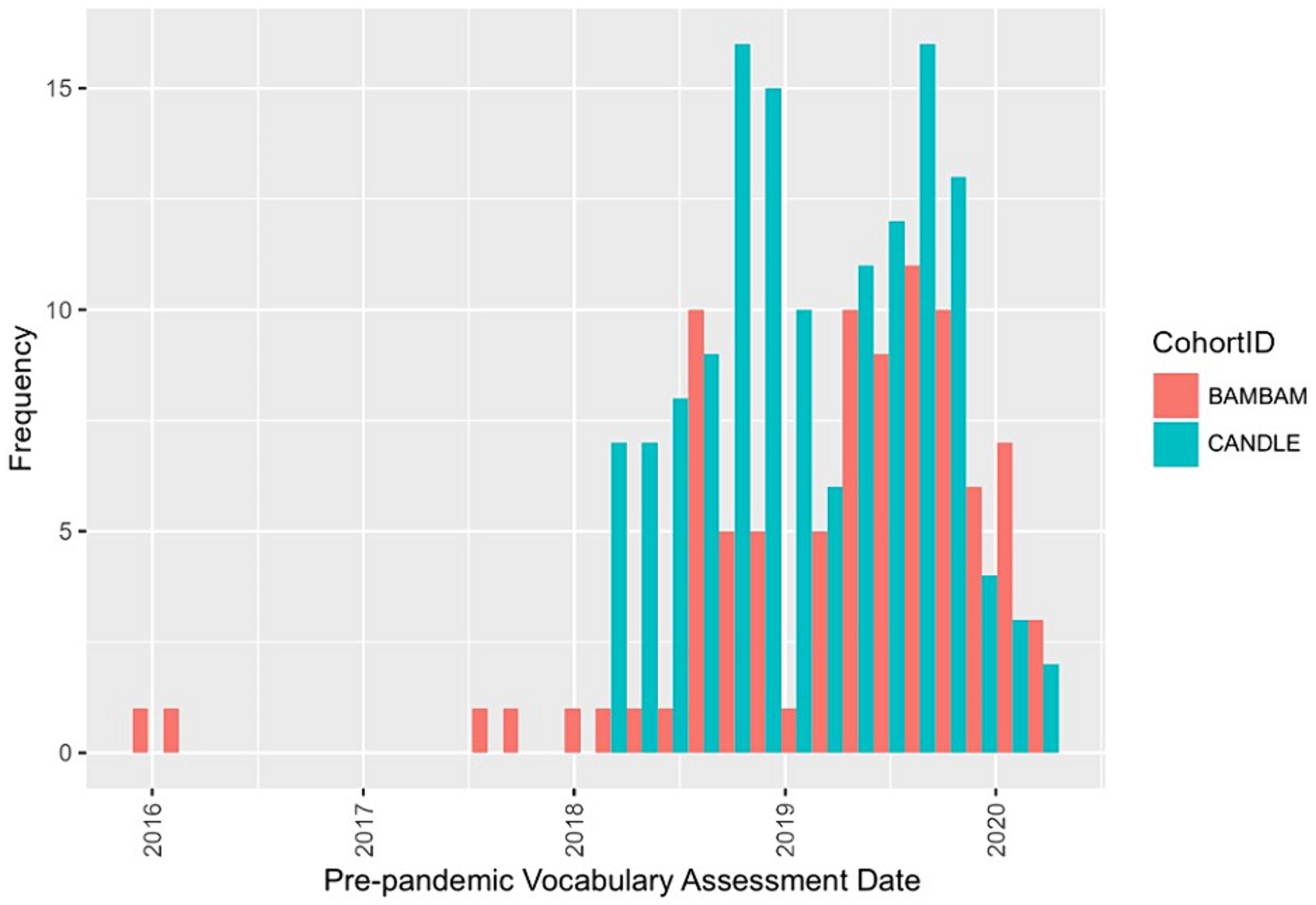
Figure 2. Pre-pandemic vocabulary assessment date distribution by Cohort. Distribution of harmonized pre-pandemic vocabulary assessment date by ECHO cohort. Dates ranged from December 2015 to March 2020.
2.2.4 Pandemic-related experiences
Caregivers reported whether they had difficulty accessing (1) food and (2) personal care products or household supplies; and whether they and/or their partner (3) became unemployed, (4) increased or decreased work hours, and (5) had a job that placed them at high risk for contracting coronavirus (Thomason et al., 2020). Items were summed to create a total COVID-19 family hardships score. Caregivers also completed the Pandemic-related Traumatic Stress Scale (PTSS; Cronbach’s 𝛼 = 0.84; Blackwell et al., 2023), which is a 9-item scale aligned to Diagnostic and Statistical Manual of Mental Disorders, 5th Edition (DSM-5) Acute Stress Disorder criteria (American Psychiatric Association, 2022). Items were framed as, “Since becoming aware of the COVID-19 outbreak, how often have you..” using a 5-point Likert response scale anchored by not at all and very often. Average total scores were computed.
2.2.5 Additional covariates
Variables included: child individualized education plan (IEP) described whether the child had an IEP (Yes = 1); child age in years (continuous) at AAB administration; child sex (reference = female); caregiver educational attainment, a dichotomous indicator with bachelor’s degree as the reference category; and cohort, a dichotomous indicator describing if the child was from CANDLE (reference category) or BAMBAM to account for differences across cohorts unaccounted for by other covariates. In our study, minoritized race and ethnicity were largely colinear with cohort (see Table 1). Therefore, we did not adjust for race or ethnicity, nor did we interpret the estimated coefficients for the cohort variable as we cannot distinguish between cohort effects and those related to being in a minoritized group.
2.3 Analytic procedure
All analyses were conducted in R version 4.1.0 (R Core Team, 2020). To account for missing data on covariates, multiple imputation was conducted using the smcfcs package (Bartlett et al., 2022) in R with 100 imputations and 100 burn-in iterations per imputation. Trace plots were examined to ensure convergence.
We conducted separate linear regressions by outcome (i.e., reading and math) and computed a series of nested models to evaluate associations between instruction disruption group status and each outcome, with each subsequent model adjusting for additional covariates as follows: Model A included pre-pandemic vocabulary, months between vocabulary and the AAB, months between end of the Spring 2020 school year and AAB administration, and the cohort indicator; Model B added child-and family-level sociodemographic variables (child age, sex, and caregiver education) and child IEP status; and Model C added COVID-19 family hardships and caregiver pandemic-related traumatic stress. We compared nested models using Meng and Rubin (1992) D3 multiple imputation likelihood ratio test statistic and adjusted R2 values. We then conducted a series of effect moderation models (Models D1-D8) whereby an individual effect moderator was added to the fully adjusted model (Model C). Regression coefficients and standard errors were combined across imputations using Rubin’s pooling rules (Rubin, 1987).
3 Results
Participant characteristics are reported in Table 1. Children came from diverse racial, ethnic, and economic backgrounds: 43.6% were White, 36.2% Black, and 18.1% multiracial; 10.3% were Hispanic; and 53.9% had caregivers with less than a bachelor’s degree. On average, children were 9.9 years old (SD = 1.75) at the time of the AAB assessment. The sample was approximately evenly split between females (46.1%) and males (53.9%), and 18% had an IEP. Most caregivers (64.9%) reported at least one COVID-19-related family hardship, and on average, caregivers had moderate pandemic-related traumatic stress (M = 1.9, SD = 0.7). On average, children’s pre-pandemic vocabulary scores were 103.2 (SD = 17.9). During the pandemic, children on average scored 108.7 (SD = 19.7) on AAB reading and 92.8 (SD = 18.6) on AAB math, with a relatively normal distribution of scores within and across cohorts (Figure 3). Approximately a third of children in the sample (32.6%) experienced >4 weeks of instruction disruption with no learning in Spring 2020.
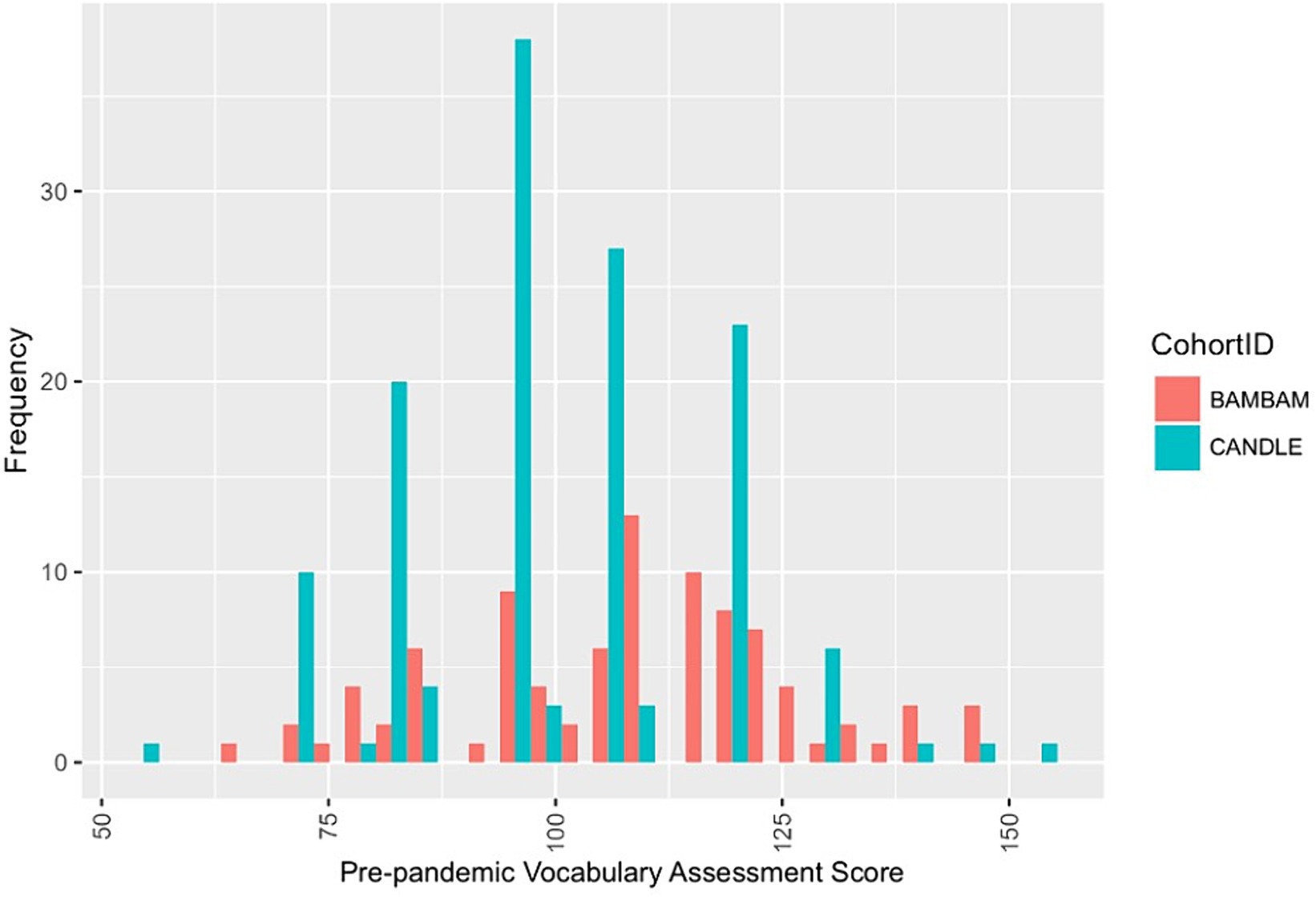
Figure 3. Pre-pandemic vocabulary score distribution by Cohort. Distribution of harmonized pre-pandemic vocabulary assessment score by ECHO cohort. Scores ranged from 53 to 153.
Linear regression results are provided in Table 2. Compared to our baseline Model A, the addition of child-and family-level demographics in Model B notably attenuated the association between instruction disruption and both outcomes. Further addition of family hardships and caregiver stress resulted in minimal additional attenuation. We only observed significant associations between instruction disruption duration and math achievement: children who experienced >4 weeks of disrupted learning scored 4.5 points [95% CI: −8.77, −0.22] lower on average compared to children whose experienced 4 weeks or less of learning disruption (Table 2, Model C: Math).
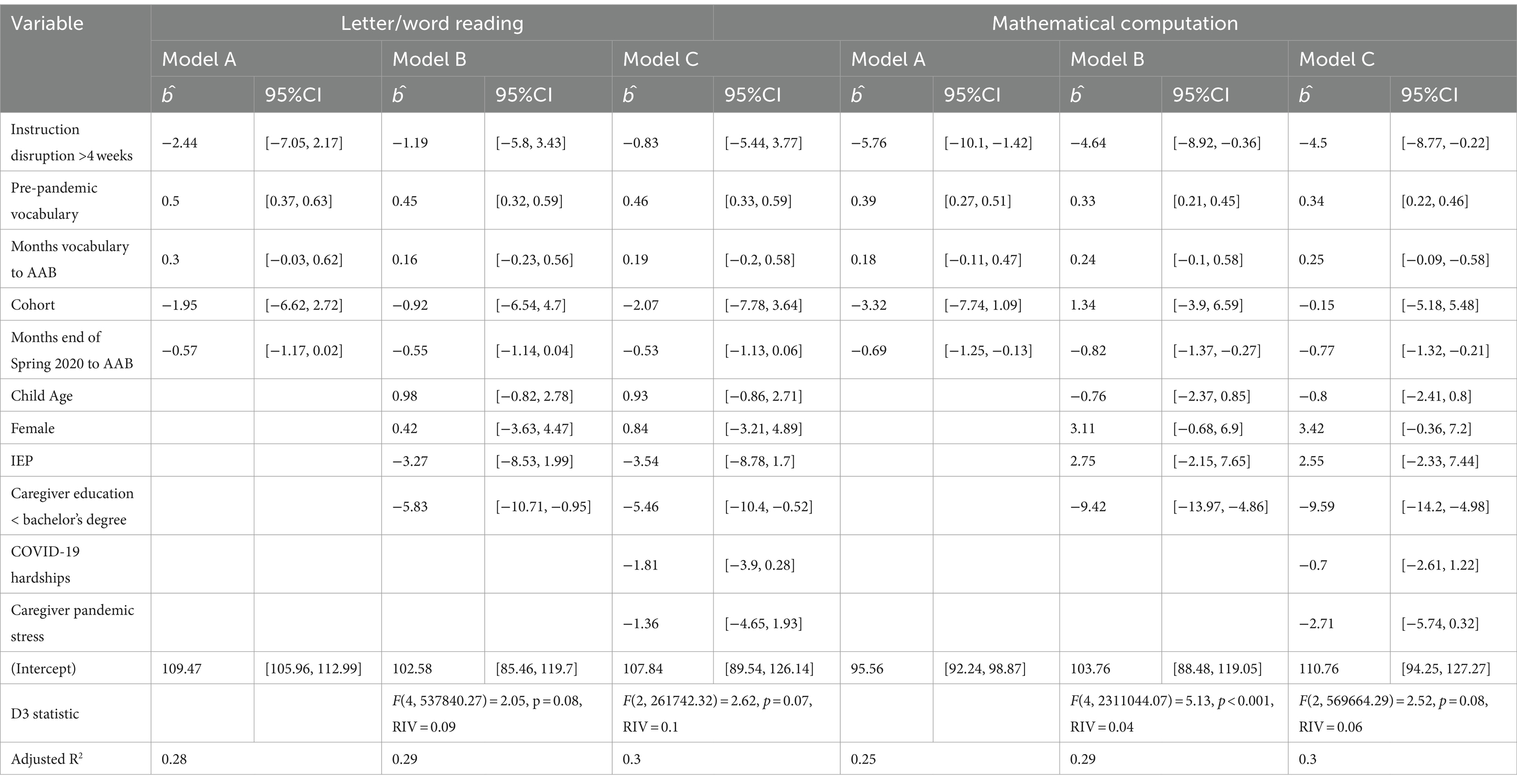
Table 2. Linear regression models predicting academic achievement from instruction disruption and covariates with mean centering.
Several child-and family-level covariates were significantly associated with children’s AAB scores. Compared to children with lower pre-pandemic vocabulary scores, those with higher scores had slightly higher math (b̂ = 0.34 [95% CI: 0.22, 0.46]) and reading (b̂ = 0.46 [95% CI: 0.33, 0.59]) achievement during the pandemic. Being female was marginally significant for math, where females scored 3.42 points [95% CI: −0.36, 7.2] higher in math compared to males (p = 0.08). Children whose caregivers had less than a BA education scored 9.59 [95% CI: −14.2, −4.98] and 5.46 [95% CI: −10.4, −0.52] points lower on math and reading, respectively. Higher caregiver pandemic-related traumatic stress was marginally significant for math (b̂ = 2.71 [95% CI: −5.74, 0.32], p = 0.08).
We found no evidence to suggest associations were moderated by child age, sex, IEP, pre-pandemic vocabulary, caregiver education, caregiver pandemic-related traumatic stress, or COVID-19-related family hardships (Supplementary Tables 1, 2).
4 Discussion
Children who experienced more than 4 weeks with no instruction (in-person or remote) had worse math performance compared to peers whose schools were closed for 4 weeks or less. The estimated 4.5-point difference in scores equates to nearly a one-third standard deviation lower score on average, which is three times higher than summer math learning loss estimates (Cooper et al., 1996) and larger than learning loss associated with student displacement from natural disasters (e.g., Sacerdote, 2012; Morrill and Westall, 2023). Reading scores were not associated with learning disruption duration, potentially due to reading being an activity that parents could easily accommodate without much guidance from educators, whereas math requires more technical knowledge. Even when parents engage in both reading and math activities at home, they spend twice as much time on reading compared to math (Napoli and Purpura, 2018), which may reflect higher levels of parent math anxiety (Maloney et al., 2015) that limited their ability to teach math when schools were closed for any type of learning – remote or otherwise. Our results reflect those found in group-level analyses (e.g., Hammerstein et al., 2021; König and Frey, 2022) and the broader phenomenon of stronger associations between math scores (compared to reading) and extended out-of-school time (e.g., Cooper et al., 1996; Kuhfled and Tarasawa, 2020). In the current study, analyses of individual-level data and staged modeling extends these findings by adjusting for confounding, enabling us to derive more valid estimates of the association between learning disruption and achievement. As most prior work on COVID-19 and student achievement uses group-level comparisons (e.g., school-level, historical cohorts), our results suggest prior findings may be over-estimates of the impact of pandemic-related school disruptions on child academic achievement. We further extend the literature by drawing on achievement data collected in-person and through the beginning of 2022 to examine the longer-term impact of Spring 2020 instruction disruptions, finding that students who completed the AAB farther from the start of the pandemic scored slightly worse in math compared to peers who completed the AAB closer to the start of the pandemic. Drawing on data through Spring 2021, Molnár and Hermann (2023) found 2nd–8th graders not only experienced an initial learning loss but a year later had nearly 0.25 of a standard deviation of accumulated learning loss compared to pre-pandemic peers. Our results further suggest COVID-19-related instruction disruptions may have even longer lingering impacts on student learning.
Individual level data can also be used to gain insights into factors beyond learning disruptions that may have impacted achievement. Our final models suggest that focusing on the length of disrupted learning fails to tell the entire story. Notably, children whose caregivers had less than bachelor’s education scored two-thirds of a standard deviation lower in math and one-third of a standard deviation lower in reading compared to children whose caregivers had a bachelor’s degree or higher. While the addition of pandemic-related factors only explained an additional 1–2% of the variance in our models, results showed caregiver stress was marginally associated with math scores, such that a one-point increase in stress was associated with approximately a fifth of a standard deviation lower math score. Caregivers who had lower educational attainment and experienced higher stress may have had more difficulty supplementing at-home math instruction due to increased psychological strain and limited resources and content knowledge to practice math directly with children. While this study was not explicitly designed to study these associations, our results indicate fruitful areas for future research that go beyond instruction disruption impacts.
Moreover, our individual-level data enabled investigation of the potential effect modification by important sociodemographic and pandemic factors. Though all tests were null, examining heterogeneity in the impact of the pandemic within different subgroups is of prime importance with respect to informing recovery efforts. Unfortunately, our study sample was largely underpowered for tests of effect modification. Mounting evidence suggests some children are more vulnerable to the effects of the pandemic (e.g., Hammerstein et al., 2021; Cohodes et al., 2022; König and Frey, 2022; Betthäuser et al., 2023), and larger studies with individual-level data like this one are necessary to examine differences.
4.1 Practical implications
The current study points to important considerations for elementary educators, schools, and families as the US continues to build back from the social and economic devastation of the COVID-19 pandemic. Even several years after the pandemic onset, students in our sample still showed lower math achievement regardless of how much time elapsed between school closures and AAB completion. Educators in schools that experienced extended learning disruptions in Spring 2020 may benefit from increased focus on math instruction, including incorporation of hands-on and materials-based math instruction that may have been missed during virtual instruction periods. High dosage math tutoring also holds promise, as Nickow et al. (2020) found students who engaged in at least 30 min of small group tutoring three to 5 days a week had 0.38 SD gains in math, nearly equivalent to the math learning loss found in this study.
Given the broader issue that math tends to be more impacted by extended out-of-school time, schools may benefit from building stronger foundational caregiver-school partnerships to support caregivers in at-home supplemental, hands-on math activities and instruction. Such partnerships require more than ensuring parents have the basic content knowledge necessary but also the confidence, as parental math anxiety can limit children’s math achievement (Maloney et al., 2015). Importantly, addressing broader family socioeconomic and psychosocial contextual factors is also critical, especially during highly stressful conditions such as the COVID-19 pandemic. Our study suggests that, in addition to learning disruptions resulting from school closures, child and family-level characteristics also played a role in student outcomes, unveiling important malleable mechanisms that can be targeted for future interventions. Wraparound services at the family level, such as caregiver mental health and material resource support, could have downstream impact on the child’s achievement and are therefore important factors for education policymakers to consider.
4.2 Limitations
This study is not without limitations. Our modest-sized sample from two primarily urban geographic locations may limit generalizability to children in other parts of the country. However, our findings are consistent with those of larger studies with more geographically diverse samples, and we uniquely contribute results drawing on individual-level data collected in person during a global pandemic—a feat unfeasible at large scale. Further, despite this being a partial cohort analysis, participants reflected the racial diversity of the full cohort samples but had caregivers who were, on average, more highly educated (Deoni et al., 2012; Sontag-Padilla et al., 2015). Such differences between our subsample and the full cohort may reflect a common challenge faced generally by other large pediatric cohort studies that continued data collection during the pandemic (e.g., Adolescent Brain Cognitive Development Study [ABCD]) whereby higher resourced families were able to participate and provide data during the COVID-19 pandemic (Yip et al., 2022). Thus, results presented here and those from other large-scale cohort studies conducted during the pandemic may not reflect the experiences of the hardest hit families who were not able to respond to surveys or assess children in person for academic achievement assessments mid-pandemic. Our findings may therefore underestimate the impact of COVID-19 learning disruptions and family contextual factors for these children.
Given children within each cohort came from the same geographical area, they were likely subject to district-level school closure plans, which would limit the variability of the number of weeks they experienced disrupted learning. Both Shelby County, TN and Providence, RI adopted various remote learning instructional strategies (e.g., digital and paper workbooks, recorded lessons) while school buildings were closed (Center on Reinviting Public Education, 2023) but known differences at the school and teacher level suggest learning disruptions did vary significantly (Hamilton and Ercikan, 2022). Therefore, while caregiver-report of instruction disruption may be slightly under or overestimated, caregivers were in the best position to know exactly how many weeks their children did not experience learning of any kind.
Like prior studies, we did not have individual-level pre-pandemic academic achievement data using the same during pandemic assessment (i.e., the AAB), which limited our ability to directly assess within-child changes resulting from the pandemic. Additionally, the skewed nature of our exposure measure required grouping children into those who experienced 4 weeks or less of no learning and those experienced more than 4 weeks of no learning. While this dichotomization was theoretically driven based on cumulative standard breaks that occur during a normal school year such as winter and spring vacation and thresholds for chronic absenteeism, the measure lacked granularity and may underestimate associations for those who experienced greater than 4 weeks of no learning. Indeed, 4 weeks into the pandemic, with only a third of US school districts offering a formal curriculum with instruction (Lake and Dusseault, 2020), many students likely experienced longer durations of no learning. Our measure of pre-pandemic vocabulary served as a robust proxy measure and enabled us to control for any pre-existing academic functioning differences. Finally, we did not have sufficient data on children’s 2020/21 school experiences, such as instructional type and quality, classroom environment, and curriculum content, which likely contribute to their achievement. However, prior research suggests the Spring 2020 school disruptions impacted achievement more so than later pandemic-related disruptions (König and Frey, 2022), suggesting Spring 2020 is the critical exposure period to assess as we do here.
Overall, this study extends our understanding of the impact of the COVID-19 pandemic on student achievement by providing one of the first investigations using individual-level data within a racially, ethnically, and economically diverse sample. Findings point to the potential need for remedial math instruction for children who experienced extended instruction disruptions and that children of caregivers with lower levels of education and higher stress may require the most support.
Data availability statement
The datasets presented in this study can be found in online repositories. The names of the repository/repositories and accession number (s) can be found at: individual-level de-identified ECHO data and the accompanying data dictionary are available in the NIH National Institute of Child Health and Human Development (NICHD) Data and Specimen Hub (DASH). To access these data, interested investigators can submit study proposals on the DASH website: https://dash.nichd.nih.gov.
Ethics statement
The studies involving humans were approved by Environmental influences on Child Health Outcomes (ECHO) single IRB. The studies were conducted in accordance with the local legislation and institutional requirements. Written informed consent for participation in this study was provided by the participants’ legal guardians/next of kin.
Program collaborators for environmental influences on child health outcomes program
ECHO components: coordinating Center: Duke Clinical Research Institute, Durham, NC: Smith PB, Newby KL; Data Analysis Center: Johns Hopkins University Bloomberg School of Public Health, Baltimore, MD: Jacobson LP, Churchill M; Research Triangle Institute, Durham, NC: Catellier D; Person-Reported Outcomes Core: Northwestern University Feinberg School of Medicine, Chicago, IL: Gershon R, Cella D.
ECHO awardees and cohorts: University of New Mexico, Albuquerque, NM: MacKenzie DA, Lewis J; Columbia University Mailman School of Public Health, New York, NY: Herbstman J.
Author contributions
CB: Conceptualization, Formal analysis, Investigation, Methodology, Project administration, Writing – original draft, Writing – review & editing. MaM: Data curation, Formal analysis, Methodology, Software, Writing – original draft, Writing – review & editing. SD: Data curation, Funding acquisition, Investigation, Methodology, Writing – review & editing. JG: Data curation, Funding acquisition, Investigation, Methodology, Writing – review & editing. LL: Data curation, Funding acquisition, Investigation, Methodology, Writing – review & editing. AM: Data curation, Funding acquisition, Investigation, Methodology, Writing – review & editing. MoM: Data curation, Investigation, Methodology, Writing – review & editing. SN: Methodology, Writing – review & editing. TO’S: Methodology, Writing – review & editing, Data curation, Funding acquisition. PS: Methodology, Writing – review & editing. QZ: Funding acquisition, Methodology, Writing – review & editing. KL: Conceptualization, Data curation, Formal analysis, Funding acquisition, Investigation, Methodology, Resources, Supervision, Writing – original draft, Writing – review & editing.
Funding
The author(s) declare financial support was received for the research, authorship, and/or publication of this article. Research reported in this publication was supported by the Environmental influences on Child Health Outcomes (ECHO) program, Office of The Director, National Institutes of Health, under Award Numbers U2COD023375 (Coordinating Center), U24OD023382 (Data Analysis Center, MPIs Jacobson, Catellier), U24OD023319 with co-funding from the Office of Behavioral and Social Sciences Research (OBSSR; Person Reported Outcomes Core, MPIs Cella, Gershon), UH3OD023285 (PI Deoni), UH3OD023290 (PI Herbstman), UH3OD02371 (MPIs LeWinn, Zhao, Karr, Bush, Sathyanarayana), UH3OD023344 (MPIs MacKenzie, Lewis), UH3OD023389 (MPIs Leve, Ganiban, Neiderhiser), UH3OD023348 (MPIs O’Shea, Fry). The content is solely the responsibility of the authors and does not necessarily represent the official views of the National Institutes of Health.
Acknowledgments
The authors wish to thank our ECHO colleagues; the medical, nursing, and program staff; and the children and families participating in the ECHO cohorts. We also acknowledge the contribution of the following ECHO program collaborators.
Conflict of interest
The authors declare that the research was conducted in the absence of any commercial or financial relationships that could be construed as a potential conflict of interest.
Publisher’s note
All claims expressed in this article are solely those of the authors and do not necessarily represent those of their affiliated organizations, or those of the publisher, the editors and the reviewers. Any product that may be evaluated in this article, or claim that may be made by its manufacturer, is not guaranteed or endorsed by the publisher.
Supplementary material
The Supplementary material for this article can be found online at: https://www.frontiersin.org/articles/10.3389/feduc.2024.1295910/full#supplementary-material
References
American Psychiatric Association . (2022). Diagnostic and statistical manual of mental disorders, fifth edition, text revision (DSM-5-TR). American Psychiatric Association, US.
Bartlett, J., Keogh, R., and Bonneville, E. (2022). Smcfcs: multiple imputation of covariates by substantive model compatible fully conditional specification. R package version 1.7.0, URL https://CRAN.R-project.org/package=smcfcs.
Betthäuser, B. A., Bach-Mortensen, A. M., and Engzell, P. (2023). A systematic review and meta analysis of the evidence on learning during the COVID-19 pandemic. Nat. Hum. Behav. 7, 375–385. doi: 10.1038/s41562-022-01506-4
Blackwell, C. K., Sherlock, P. R., Jackson, K. L., Hofheimer, J. A., Cella, D., and Algermissen, M. A. (2023). Development and psychometric validation of the pandemic-related traumatic stress scale for children and adults. Psychol. Assess. 35, 1054–1067. doi: 10.1037/pas0001211
Bleses, D., Makransky, G., Dale, P. S., Højen, A., and Ari, B. A. (2016). Early productive vocabulary predicts academic achievement 10 years later. Appl. Psycholinguist. 37, 1461–1476. doi: 10.1017/S0142716416000060
Center on Reinviting Public Education . (2023). Pandemic tracking data: school year 2019-20. Seattle, WA: center on reinventing public education. Available at: https://crpe.org/pandemic-learning/tracking-district-actions/ [Accessed September 15, 2023]
Cohodes, S., Goldhaber, D., Hill, P., Ho, A., Kogan, V., Polikoff, M., et al. (2022). Student achievement gaps and the pandemic: A new review of evidence from 2021–2022. Seattle, WA: Center on Reinventing Public Education.
Cooper, H., Nye, B., Charlton, K., Lindsay, J., and Greathouse, S. (1996). The effects of summer vacation on achievement test scores: a narrative and meta-analytic review. Rev. Educ. Res. 66, 227–268. doi: 10.3102/00346543066003227
Deoni, S. C., Dean, D. C. III, O'Muircheartaigh, J., Dirks, H., and Jerskey, B. A. (2012). Investigating white matter development in infancy and early childhood using myelin water faction and relaxation time mapping. NeuroImage 63, 1038–1053. doi: 10.1016/j.neuroimage.2012.07.037
Engzell, P., Frey, A., and Verhagen, M. D. (2021). Learning loss due to school closures during the COVID-19 pandemic. Proc. Natl. Acad. Sci. 118:e2022376118. doi: 10.1073/pnas.2022376118
Gershon, R. C., Wagster, M. V., Hendrie, H. C., Fox, N. A., Cook, K. F., and Nowinski, C. J. (2013). NIH toolbox for assessment of neurological and behavioral function. Neurology 80, S2–S6. doi: 10.1212/WNL.0b013e3182872e5f
Goldhaber, D., Kane, T. J., McEachin, A., and Morton, E. (2022a). A comprehensive picture of achievement across the COVID-19 pandemic years: Examining variation in test levels and growth across districts, schools, grades, and students. Arlington, VA: American Institutes for Research National Center for analysis of longitudinal data in education research (CALDER).
Goldhaber, D., Kane, T. J., McEachin, A., Morton, E., Patterson, T., and Staiger, D. O. (2022b). The consequences of remote and hybrid instruction during the pandemic (no. w30010). Cambridge, MA: National Bureau of Economic Research.
Hamilton, L. S., and Ercikan, K. (2022). “COVID-19 and US schools: using data to understand and mitigate inequities in instruction and learning” in Primary and secondary education during Covid-19: Disruptions to educational opportunity during a pandemic. ed. F. M. Reimers (New York, NY: Springer), 327–351.
Hammerstein, S., König, C., Dreisörner, T., and Frey, A. (2021). Effects of COVID-19-related school closures on student achievement-a systematic review. Front. Psychol. 12:746289. doi: 10.3389/fpsyg.2021.746289
Knapp, E. A., Kress, A. M., Parker, C. B., Page, G. P., McArthur, K., Gachigi, K. K., et al. (2023). The environmental influences on child health outcomes (ECHO)-wide cohort. Am. J. Epidemiol. 192:kwad071, 1249–1263. doi: 10.1093/aje/kwad071
König, C., and Frey, A. (2022). The impact of COVID-19-related school closures on studentachievement—a meta-analysis. Educ. Meas. Issues Pract. 41, 16–22. doi: 10.1111/emip.12495
Kuhfeld, M., Lewis, K., and Peltier, T. (2023). Reading achievement declines during the COVID-19 pandemic: evidence from 5 million US students in grades 3–8. Read. Writ. 36, 245–261. doi: 10.1007/s11145-022-10345-8
Kuhfeld, M., Soland, J., and Lewis, K. (2022a). Test score patterns across three COVID-19-impacted school years. Educ. Res. 51, 500–506. doi: 10.3102/0013189X221109178
Kuhfeld, M., Soland, J., Lewis, K., Ruzek, E., and Johnson, A. (2022b). The COVID-19 school year: learning and recovery across 2020-2021. Aera Open 8:23328584221099306. doi: 10.1177/23328584221099306
Kuhfled, M., and Tarasawa, B. (2020). The COVID-19 slide: What summer learning loss can tell us about the potential impact of school closures on student academic achievement. Washington, DC: NWEA.
Lake, R., and Dusseault, B. (2020). Districts and CMOs are making Progress on instruction and monitoring, but lag in grading and Attendance. The Lens. Available at: https://www.crpe.org/thelens/districts-and-cmos-are-making-progress-instruction-and-monitoring-lag-grading-and-attendance [Accessed September 15, 2023]
Lara, J., Noble, K., Pelika, S., and Coons, A. (2018). Chronic absenteeism. NEA research brief. NBI no. 57. Washington, DC: National Education Association.
LeWinn, K. Z., Bush, N. R., Batra, A., Tylavsky, F., and Rehkopf, D. (2020). Identification of modifiable social and behavioral factors associated with childhood cognitive performance. JAMA Pediatr. 174, 1063–1072. doi: 10.1001/jamapediatrics.2020.2904
LeWinn, K. Z., Caretta, E., Davis, A., Anderson, A. L., and Oken, E. (2022). SPR perspectives: environmental influences on child health outcomes (ECHO) program: overcoming challenges to generate engaged, multidisciplinary science. Pediatr. Res. 92, 1262–1269. doi: 10.1038/s41390-021-01598-0
Malkus, N. (2020). Too little, too late: A hard look at spring 2020 remote learning. Washington, DC: American Enterprise Institute.
Maloney, E. A., Ramirez, G., Gunderson, E. A., Levine, S. C., and Beilock, S. L. (2015). Intergenerational effects of parents’ math anxiety on children’s math achievement and anxiety. Psychol. Sci. 26, 1480–1488. doi: 10.1177/0956797615592630
Meng, X. L., and Rubin, D. B. (1992). Performing likelihood ratio tests with multiply-imputed data sets. Biometrika 79, 103–111. doi: 10.1093/biomet/79.1.103
Molnár, G., and Hermann, Z. (2023). Short-and long-term effects of COVID-related kindergarten and school closures on first-to eighth-grade students’ school readiness skills and mathematics, reading and science learning. Learn. Instr. 83:101706. doi: 10.1016/j.learninstruc.2022.101706
Morrill, M., and Westall, J. (2023). Heterogeneity in the educational impacts of natural disasters: evidence from hurricane Florence. Econ. Educ. Rev. 94:102373. doi: 10.1016/j.econedurev.2023.102373
Napoli, A. R., and Purpura, D. J. (2018). The home literacy and numeracy environment in preschool: cross-domain relations of parent-child practices and child outcomes. J. Exp. Child Psychol. 166, 581–603. doi: 10.1016/j.jecp.2017.10.002
Nickow, A., Oreopoulos, P., and Quan, V. (2020). The impressive effects of tutoring on prek-12 learning: a systematic review and meta-analysis of the experimental evidence. Cambridge, MA: National Bureau of Economic Research.
Peele, H., Riser-Kositsky, M., and Kim, H-Y. (2021). Map: coronavirus and school closures in 2019-2020. Education week. March 6, 2020 (updated October 13, 2021). Accessed January 30, 2023. Available at: https://www.edweek.org/leadership/map-coronavirus-and-school-closures-in-2019-2020/2020/03 [Accessed September 15, 2023]
R Core Team (2020). R: a language and environment for statistical computing. R foundation for statistical computing, Vienna, Austria. URL https://www.R-project.org/.
Rubin, D. B. (1987). Multiple imputation for nonresponse in surveys. Hoboken, NJ: John Wiley & Sons.
Sacerdote, B. (2012). When the saints go marching out: long-term outcomes for student evacuees from hurricanes Katrina and Rita. Am. Econ. J. Appl. Econ. 4, 109–135. doi: 10.1257/app.4.1.109
Sontag-Padilla, L., Burns, R. M., Shih, R. A., Griffin, B. A., Martin, L. T., Chandra, A., et al. (2015). The urban child institute CANDLE study. Santa Monica, CA: RAND Corporation.
Thomason, M. E., Graham, A., and VanTieghem, M. R. (2020). The COPE-IS: coronavirus perinatal experiences–impact survey. Available at: https://www.phenxtoolkit.org/toolkit_content/PDF/COS_COPE_IS.pdf [Accessed September 15, 2023]
Tomasik, M. J., Helbling, L. A., and Moser, U. (2021). Educational gains of in-person vs. distance learning in primary and secondary schools: a natural experiment during the COVID-19 pandemic school closures in Switzerland. Int. J. Psychol. 56, 566–576. doi: 10.1002/ijop.12728
UNESCO . (2021). Education: from disruption to recovery. Available at: https://en.unesco.org/covid19/educationresponse
Weintraub, S., Bauer, P. J., Zelazo, P. D., Wallner-Allen, K., Dikmen, S. S., Heaton, R. K., et al. (2013). I. NIH toolbox cognition battery (CB): introduction and pediatric data. Monogr. Soc. Res. Child Dev. 78, 1–15. doi: 10.1111/mono.12031
Yip, S. W., Jordan, A., Kohler, R. J., Holmes, A., and Bzdok, D. (2022). Multivariate, transgenerational associations of the COVID-19 pandemic across minoritized and marginalized communities. JAMA Psychiatry 79, 350–358. doi: 10.1001/jamapsychiatry.2021.4331
Keywords: COVID-19, pandemic, academic achievement, school closure, learning, children
Citation: Blackwell CK, Mansolf M, Deoni SCL, Ganiban JM, Leve LD, Margolis AE, McGrath M, Nozadi SS, O’Shea TM, Sherlock P, Zhao Q and LeWinn KZ on behalf of program collaborators for Environmental influences on Child Health Outcomes Program (2024) The impact of COVID-19 school disruptions on children’s learning. Front. Educ. 9:1295910. doi: 10.3389/feduc.2024.1295910
Edited by:
Douglas F. Kauffman, Medical University of the Americas – Nevis, United StatesReviewed by:
Armanda Pereira, University of Trás-os-Montes and Alto Douro, PortugalInês Carvalho Relva, University of Trás-os-Montes and Alto Douro, Portugal
Copyright © 2024 Blackwell, Mansolf, Deoni, Ganiban, Leve, Margolis, McGrath, Nozadi, O’Shea, Sherlock, Zhao, LeWinn and on behalf of program collaborators for Environmental influences on Child Health Outcomes Program. This is an open-access article distributed under the terms of the Creative Commons Attribution License (CC BY). The use, distribution or reproduction in other forums is permitted, provided the original author(s) and the copyright owner(s) are credited and that the original publication in this journal is cited, in accordance with accepted academic practice. No use, distribution or reproduction is permitted which does not comply with these terms.
*Correspondence: Courtney K. Blackwell, Y2tibGFja3dlbGxAbm9ydGh3ZXN0ZXJuLmVkdQ==