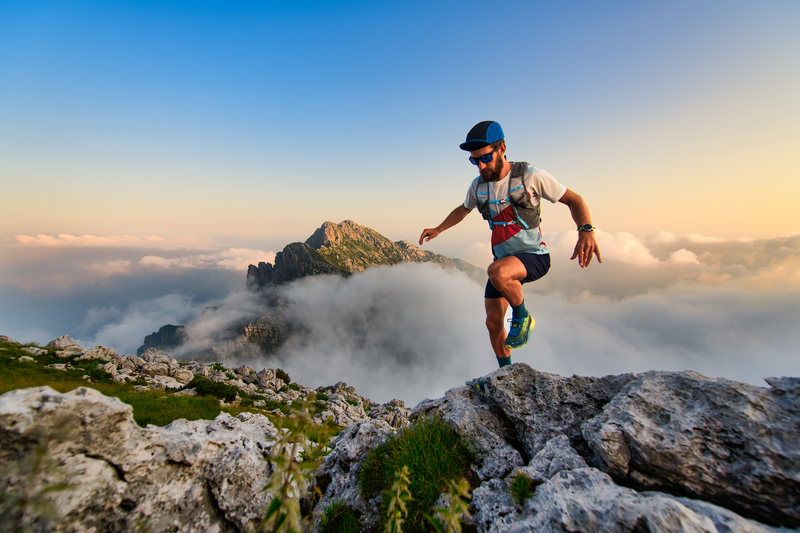
94% of researchers rate our articles as excellent or good
Learn more about the work of our research integrity team to safeguard the quality of each article we publish.
Find out more
BRIEF RESEARCH REPORT article
Front. Educ. , 30 January 2024
Sec. Special Educational Needs
Volume 9 - 2024 | https://doi.org/10.3389/feduc.2024.1270484
This article is part of the Research Topic Interventions for Students with Combined Learning and Behavioral Difficulties View all 6 articles
This study examines the effectiveness of an app-based self-monitoring intervention to support attention in students with learning difficulties. Two quantitative single-case studies were conducted in special education school settings. Study 1 used an AB design in which 12 seventh-grade students with learning difficulties were assessed for attentional behavior during a math exercise by systematic observation using the Munich Attention Inventory with five-second time sampling by two raters. Study 2 used a multiple baseline design to assess the attentional behavior of three students with combined learning and attention difficulties during a math exercise by systematic observation using Direct Behavior Rating to measure on-task and off-task behavior. Both studies also used a competency screening to elicit teacher ratings of change in attention behavior in a pre-post measurement. The results indicate that the app-based self-monitoring interventions were successful, highlighting the potential of app-based self-monitoring to support students with learning and attention difficulties. In particular, the development of personalized self-monitoring interventions holds promising potential for improving learning outcomes in this target group.
Self-regulatory competence is of great importance for adapting to constantly changing environments (Landmann and Schmitz, 2007, p. 151). Specifically, self-regulated learning, understood as the ability to set learning goals, choose appropriate techniques and strategies to achieve those goals, and stay motivated to complete and to reflect on the learning process, is an essential skill relevant to academic success (Aschermann and Armbrüster, 2011). However, students with learning difficulties1 are more likely to struggle with self-regulation and, in particular, with self-regulated learning in school, as the basic prerequisites required for this (e.g., attention, motivation, learning strategies, memory) are often limited (cf. Matthes, 2018, p. 42; Gold, 2018, p. 33). This also affects students’ self-determination capacities. Self-determination is an umbrella term that encompasses all behaviors, beliefs, and skills aimed at improving the quality of life through increased self-reliance. Within the field of self-determination, self-management is a subcategory that overlaps with the concept of self-regulated learning (Bruhn et al., 2015, pp. 102–103). The term self-regulation in learning is sometimes used synonymously, sometimes in distinction to self-management. In this article, we use the two terms interchangeably. Overall, we are interested in how students with learning difficulties can stay motivated, and focused on the learning process.
Five aspects of self-regulated learning are particularly salient intervention points for supporting individuals who wish to change, maintain, or extend certain behaviors: Self-monitoring, self-evaluation, self-regulated strategy development, self-instruction, and goal setting (Mooney et al., 2005; Niesyn, 2009). In this study, we focus on self-monitoring as a learning strategy and promising intervention point to support self-regulated learning because it can be used to address attention problems in students with learning difficulties, as shown in a systematic review by Pötters et al. (2020). Self-monitoring refers to the deliberate focusing of attention on specific, pre-identified aspects of one’s behavior (Clemons et al., 2016; Bruhn et al., 2017). Self-monitoring strategies are used to a) monitor learning progress, b) identify knowledge gaps or learning barriers, and c) take corrective action (Tröster, 2019, p. 307). Self-monitoring can be implemented as an intervention through auditory, visual, or tactile prompts, using analog means or digital tools (Pötters et al., 2020).
Self-monitoring has shown particularly positive results when used as an intervention for children with a combination of learning difficulties and Attention Deficit Hyperactivity Disorder (ADHD) (Pötters et al., p. 109). This may be the case because, according to a systematic review by Visser, ADHD appears to have a high comorbidity with learning difficulties in the area of social–emotional problems (Visser et al., 2018, p. 16). Children with learning difficulties also show more internalizing and externalizing behavior problems than children without learning difficulties (Visser et al., 2018, p. 17). Previous studies have identified deficits in working memory or attention as potential causes, or at least as mediators, of this comorbidity (ibid., p. 7).
While previous studies centered on students with learning difficulties have explored a variety of ways to deliver self-monitoring interventions (cf. Bedesem and Dieker, 2013; Schardt et al., 2018; Pötters et al., 2020), only a few studies could be identified that delivered self-monitoring through modern digital apps that support self-monitoring in a comprehensive and integrated way. For example, the systematic review by Pötters et al. (2020) found six controlled single case studies using three different digital apps that implemented self-monitoring either primarily attention-related (by asking “are you on task?” at predetermined intervals; I-connect, CellF-Monitor) or primarily task-related (with checklists; Choicework) (Bedesem, 2012; Wills and Mason, 2014; Miller et al., 2015; Rosenbloom et al., 2016; Xin et al., 2017; Schardt et al., 2018).2 No studies were found that used self-monitoring via a digital app as a short-term intervention. We also could not find a study on this topic from the German-speaking area. This study aims to fill this research gap with the help of two complementary single case studies focusing on the use of the “Selbstlernen.app,” a sophisticated digital app that has some advantages over the aforementioned apps:
• It is in German: this is important because the studies take place in a German-speaking country and the app is used by students with learning and attention difficulties, who may face language barriers if an English-language app were used.
• It offers maximum flexibility in pedagogical use: teachers can use text blocks, checklists, ratings, attention-check questions, counters, and free response options to tailor scaffolding for self-regulated learning sessions for individual students. It can be used to support both task- and attention-related self-monitoring.
• It provides the ability to assign scaffolds for self-regulated learning sessions to one or a few students, or to the whole class, so that they can be used by multiple students in parallel.
• It allows students to experiment with creating scaffolds for their own self-regulated learning sessions.
The purpose of this study is to investigate the extent to which the attention of students with learning difficulties can be improved through the use of app-based self-monitoring.
Research Question 1: How does the use of the Selbstlernen.app affect the on-task behavior of students with learning difficulties in secondary school?
Hypothesis 1.1: Self-monitoring with the Selbstlernen.app will have a positive effect on students’ attentional behavior (on/off-task).
Hypothesis 1.2: The classroom teacher will judge the students’ attention competencies to be higher after the intervention than before the intervention.
Research Question 2: What is the acceptance and personal desirability of self-monitoring with the Selbstlernen.app in a school setting from the perspective of teachers and students?
Single case research designs (SCRDs) are used to answer the research questions. Although they involve only a small number of subjects, they allow causal inferences about the influence of an independent variable (usually an intervention) on behavior. They can thus be seen as a complement to (quasi-) experimental control group designs (Jain and Spieß, 2012, p. 212). SCRDs have common features (Dowdy and Jessel, 2021, p. 168), which can also be found in the two studies:
1. The behaviors under investigation are operationalized and assessed through behavioral observation.
2. The dependent variables (in this case, on-task or off-task behavior) are measured repeatedly over time in order to detect a change in behavior when the independent variable (usually an intervention, and in these studies, the use of the Selbstlernen.app) is introduced.
3. The dependent variable should be measured by multiple observers to ensure the reliability of the observation.
4. In addition, the acceptance or implementation of the independent variable can be measured.
The internal validity of the study can be increased by interleaving several intervention phases (B phases) and baselines (A phases).
To answer the research questions, two quantitative single case studies were conducted in combination with assessments of student and teacher acceptance of the Selbstlernen.app. Both studies included only children with severe learning difficulties who had undergone a formal diagnostic procedure and were receiving additional special education services in two different special schools for students with learning difficulties.
Study 1 was conducted in an AB design with 17 measurement time points (5 in the A phase) and includes the results of 12 students. All 12 students of a seventh grade class (6 males and 6 females, 12–14 years old) participated in the self-monitoring intervention to gain an understanding of how such an intervention can be implemented as a whole class activity. The study was conducted in a regular math class as a 12-min, self-directed learning activity that was completed three times a week. Students were given addition, subtraction, multiplication, and division problems in the number range up to 10,000 and used the Selbstlernen.app in the intervention phase to receive audible self-monitoring notifications via headphones every 60 s, prompting them to log whether they were still focused on the learning task. For systematic observation during the study, the Munich Attention Inventory (MAI, Helmke and Renkl, 1992) was used in both phases to assess on-task behavior. Systematic observation with the MAI was conducted by two external raters over four rounds of time sampling with four time intervals of five seconds each, resulting in 16 observations per student. The two raters each focused on 6 students and were informed of the beginning of the next time interval by an auditory signal delivered through headphones. Both on-task and off-task behaviors were observed and recorded. Additionally, a pre-post measurement using the Leipzig Competence Screening3 (Hartmann and Methner, 2015) was administered by the teacher before and after the intervention to assess changes in students’ attentional behavior.
Finally, in order to survey students’ acceptance of the Selbstlernen.app, a questionnaire was developed based on the variables of the technology acceptance model (TAM, Teo, 2010), which is widely used in the context of educational research (Granić and Marangunić, 2019). The following variables were used: Complexity of use, Design of the app, Usefulness of the components, Ease of use, Attitude towards the app, Behavioral intention. The formulation of the items of the questionnaire was based on (Cheng and Yuen, 2019, p. 1622; Davis et al., 1989, p. 324; Luan and Teo, 2009, p. 265 and Teo, 2010, p. 71), but was translated into German and simplified according to the learning needs of the students with learning difficulties. The rating was done on a 4-point scale expressing agreement (strongly disagree, disagree, agree, strongly agree) with the items. To support the students, different smiley faces were used in addition to the text labels.
Study 2 used a multiple baseline design with 13 measurement time points (3, 5, and 8 in the A phase) and includes the results of three male students, ages 13 and 14, who were selected in a two-step procedure from a mixed-grade learning group (grades 6 to 8) on the basis of their combined attention and learning difficulties. First, the sample for the study was preselected based on the teacher’s assessment of the students’ attention behavior over the past four weeks using the attention scale of the Leipzig Competence Screening. Using age- and gender-specific norms, the five students with the lowest scores were preselected. Second, additional participation criteria were discussed in a personal interview with the teacher, resulting in the selection of four students to participate in the study. However, due to the long-term illness of one student and the ineligibility of other students, the final sample size was reduced to three students. This research design was chosen to gain an understanding of how the Selbstlernen.app can be used to tailor support interventions for individual students that are adapted to their learning needs.
Study 2 also took place in a regular math class in the form of a 20-min self-directed learning activity that was completed three times a week. Students were given multiplication and division problems in the number range up to 10,000 and used the Selbstlernen.app in the intervention phase to self-monitor their attentional behavior, similar to Study 1. Direct Behavior Rating (Volpe and Briesch, 2012; Casale et al., 2019) was used to measure on-task and off-task behavior throughout the study. On-task behavior was assessed globally using a single-item scale (DBR-SIS): “Focuses attention on the lesson or task at hand.” This item includes behaviors such as completing work assignments, directing the gaze or body toward the current focus of the lesson, or asking appropriate questions related to lesson content. The observation interval was 20 min. The DBR-SIS was conducted with paper and pencil by two raters with almost perfect intercoder reliability (ρ = 0.899, p < 0.001) using a unipolar 11-point Likert scale in 10% increments with numeric and some additional verbal markers (i.e., 0% = never, 50% = sometimes 100% = always). Off-task behavior was specifically assessed using a multi-item scale (DBR-MIS) with six items (a-f):
a. is otherwise occupied without disrupting the lesson,
b. makes (potentially) disturbing noises,
c. averts the gaze from the subject matter,
d. interacts actively with others or attempts to do so,
e. has a blank look or is dreaming,
f. responds to others’ attempts to interact.
For the DBR-MIS (off-task), the 20-min learning period was divided into four observation intervals of five minutes each. The DBR-MIS was conducted with paper and pencil by up to two raters with good intercoder reliability (53.85% coverage, ρ = 0.574, p = 0.001) using a unipolar 6-point Likert scale with verbal and numerical markers (i.e., 0 = never, 5 = always). Additionally, the teacher used the Leipzig Competence Screening before and after the intervention to assess changes in attentional behavior.
Finally, student and teacher acceptance of the app-based self-monitoring intervention was assessed using the Factor I of the CURP (Briesch and Chafouleas, 2009) and the URP-IR (Chafouleas et al., 2011). For this purpose, the researcher carrying out the intervention translated the relevant items into German and asked the students to rate them on a 4-point scale expressing agreement using emoticons (e.g., a rocket). The teacher rated the items on a standard 6-point Likert scale.
In both studies, several software packages were used to perform parts of the analyses: Microsoft Excel (version 2,209) for general data analysis, IBM SPSS Statistics (version 28.0.1.1 (15)) to calculate intercoder reliability, and the online calculator at http://singlecaseresearch.org/ to calculate variables specific to the single case study (Vannest et al., 2016).
The results of the systematic observations of the on-task behavior of all students are visualized in Figure 1. For all students, the mean of the intervention phase is above the mean of the baseline. The differences between the students vary in size, and not all of them are significant. This may be due to the fact that some students (2, 4, 8, 11) already had high baseline scores, making it much more difficult to achieve a high increase. Students 3 and 5 have lower baseline scores but show a clear fluctuation in both baseline and intervention, suggesting that attention is more dependent on the form of the day and less on the use of the Selbstlernen.app. It may be necessary to use additional interventions with these students. Students 1, 6, 7, and 9 show particularly large differences with mean differences greater than 4 and correspondingly large effects in Tau U (see also Table 1, Study 1).
Table 1 summarizes all the results of the systematic observations as well as the results of the competence screening for both Study 1 and Study 2. To assess the effectiveness of the self-monitoring intervention, a trend analysis and various overlap indices were calculated (see Table 1, Study 1). According to Brossart et al. (2018, p. 7), we interpret the Tau-U values as small, moderate, large, and very large change effects. Changes can be seen for all students, so an increase in on-task behavior can be assumed. Seven students show large or very large change effects, six of which can be considered significant. However, it can be seen that the classification of the results for the individual students is very mixed. There may be several reasons for this. Especially for Student 6 it should be noted that all values (PND, PEM, NAP, PAND, and Phi) are around 1.00, which cannot be considered reliable due to the small number of data points (only two data points in the baseline and seven in the intervention). Therefore, no Cohen’s d is calculated for Student 6. The calculation of PAND is only recommended when the number of data points exceeds 20 (Parker et al., 2007, p. 196). The maximum number of data points for students in Study 1 was 17, so the results can only be interpreted as indicative. Based on these considerations, the Selbstlernen.app intervention is estimated to be effective for six students (1, 2, 7, 9, 11, 12) with medium to strong effects.
In addition to the systematic observations, the teacher rated attention behavior using the Leipzig Competence Screening in a pre-post assessment (see Table 1, Study 1). On average, students improved by 4.417 points on a range of 8 (min = 0, max = 8). No student was rated worse by the teacher on attention behavior after the intervention. With the exception of Student 9, the students for whom the systematic observation results indicated an effective intervention also had above average teacher ratings of change in attention behavior. For Student 9, it can be assumed that ceiling effects are at work because he already had the highest baseline score. Accordingly, the teacher’s perception and the systematic observation on the part of the (external) raters are in agreement.
The acceptance of the Selbstlernen.app was rated high by the students. Complexity of use was rated with 111 out of 132 possible points (84%), Design of the app with 113 out of 132 (86%), Usefulness of the components with 144 out of 176 (82%), Ease of use with 169 out of 176 (96%), Attitude towards the app with 96 out of 132 (73%), and Behavioral intention with 88 out of 132 (66%). The teacher was also very enthusiastic about the app, calling it a “great” addition to the lessons that she would like to continue using.
As shown in Figure 2, Students A and B show a higher level of on-task behavior in the intervention phase than in the baseline. There is also a lower level of off-task behavior in the intervention phase than in the baseline. Both developments indicate the effectiveness of the intervention with the Selbstlernen.app. Furthermore, looking at the systematic observation results in Table 1 (Study 2), all three students’ ratings of on-task behavior increase and off-task behavior decreases in the intervention phase, with two students showing significant results with a combined Tau U of 0.84* (p = 0.001) and 0.86* (p = 0.001), respectively. However, Student C’s results must be viewed with caution. Although the measures show a positive direction, the results must be considered unreliable due to missing data points.
In addition to the systematic observations, the teacher rated attention behavior using the Leipzig Competence Screening in a pre-post assessment (see Table 1, Study 2). On average, students improved by 5.333 points on a range of 4 (min = 3, max = 7). No student was rated worse by the teacher in attention behavior after the intervention.
The acceptance of the Selbstlernen.app was rated high by both students and teacher. While only two students (Student A and Student B) participated in the evaluation survey, both rated the personal desirability of the app as high as possible. The teacher rated the app in a more nuanced but still very positive way, with all of her ratings being positive (i.e., 2 x slightly positive, 2 x positive, and 5 x strongly positive).
The results show that self-monitoring interventions for students with learning and attention difficulties can be successfully implemented using the Selbstlernen.app. In particular, our results suggest that students with a combination of learning difficulties and attention problems can benefit from a self-monitoring intervention. However, even individual students with learning difficulties and no attention problems also show more on-task behavior and thus may benefit from the intervention. The results were particularly positive in Study 2, where the intervention consistently showed very large effects on both on-task and off-task behavior for two students for whom sufficient time points were available. The results are supported by both pre-post teacher assessments and systematic observations by trained raters who were not directly involved in classroom activities. Accordingly, the validity of the results can assumed to be good. Furthermore, our assessments of student acceptance of the Selbstlernen.app show that it is perceived as useful and easy to use by students, which leads us to conclude that the app seems to be suitable for use with students with learning difficulties. Overall, app-based self-monitoring seems to be a promising intervention to support students with learning and attention difficulties. In particular, individual adaptation of self-monitoring interventions seems desirable, as this promises the best results. The potential of such highly personalized self-monitoring interventions should be investigated in further studies.
There was no additional assessment of students’ academic achievement or academic self-concept in mathematics, even though the study took place in the context of math lessons. These variables could act as moderators and support or hinder the effectiveness of the Selbstlernen.app. In the case of strongly negative attitudes or a very low self-concept in mathematics (or the subject in which the app is used), the effectiveness could even be completely undermined. Therefore, future studies should also examine the variables of academic achievement and academic self-concept to determine their influence.
Another possible concern is that both studies use the Leipzig Competence Screening to measure the change in the teachers’ assessment of attention. Reliability values for the instrument are only available for internal consistency, but not for retest reliability. Therefore, these changes must be interpreted with caution.
Other limitations relate to the AB design of Study 1, which is not very robust to confounding variables in the classroom. We attempted to minimize this threat to validity by enrolling a relatively large number of students in the study. The multiple baseline design of Study 2 also attempts to address this limitation of Study 1. The limiting factor in Study 2 is the missing data on Student C’s results, which means that they cannot be meaningfully used to assess the effectiveness of the intervention.
In addition, Study 1 and Study 2 must be viewed critically in terms of the number of time points measured in the A and B phases and the maximum frequency at which the observed behavior could be detected. Wilbert et al. (2022) performed simulations of different study conditions and designs to derive recommendations for the design of SCRDs based on a power analysis. According to them, observed behaviors should be detectable at least 20 times (ibid., p. 11). In Study 1, however, the maximum is 16 times. This limitation should be taken into account in future studies. Wilbert et al. (2022, p. 11) further summarize that most single case studies with less than seven measurement points in the A-phase have low explanatory power. Due to the implementation difficulties in the school settings, the A-phases in both studies are very short with 2–5 measurement time points. Accordingly, the explanatory power of both studies should be considered low. In future studies, a minimum length of 7 measurement time points in the A-phase should be planned, but ideally an a priori power analysis should be performed to determine a sufficiently long A-phase (ibid., p. 12).
Finally, the two studies build on each other, but are not fully comparable because different observation systems had to be used due to the different numbers of participants. Study 1 used the MAI for time sampling, which is not a very differentiated measure. Study 2 uses the Direct Behavior Rating, which is a somewhat more differentiated measure, especially in the area of off-task behavior, but has the disadvantage of being administered retrospectively at the end of an entire phase rather than continuously during individual situations. To overcome this limitation and to improve the reliability of the results, an observational approach using video recording in combination with event sampling could be useful. For example, the use of the Selbstlernen.app could be videotaped, which would allow us to more reliably match observed behaviors with students’ activities in the app. However, implementing such a sophisticated observation approach in practice is very demanding and has drawbacks in terms of practicality.
The original contributions presented in the study are included in the article/Supplementary material, further inquiries can be directed to the corresponding author.
Ethical approval was not required for the study involving human samples in accordance with the local legislation and institutional requirements because the study was carried out as part of regular school classes. Written informed consent for participation in this study was provided by the participants’ legal guardians/next of kin. No potentially identifiable personal data was included in this article.
CM: Formal analysis, Methodology, Supervision, Validation, Visualization, Writing – original draft, Writing – review & editing. AH: Project administration, Supervision, Writing – original draft, Writing – review & editing.
The author(s) declare financial support was received for the research, authorship, and/or publication of this article. This work was funded by the Open Access Publishing Fund of Leipzig University, which is supported by the German Research Foundation within the program Open Access Publication Funding.
The author(s) would like to acknowledge and thank the excellent work of several students who contributed significantly to the realization of the studies presented in this paper. Selbstlernen.app was developed by David Mitrus, Malte Hain and Michael Ulko as part of their master theses at the University of Cologne. The micro studies themselves were planned and carried out by Michèle Evers, Linda Schöne, and Sophie Sternkiker as part of their state exams at Leipzig University.
The authors declare that the research was conducted in the absence of any commercial or financial relationships that could be construed as a potential conflict of interest.
All claims expressed in this article are solely those of the authors and do not necessarily represent those of their affiliated organizations, or those of the publisher, the editors and the reviewers. Any product that may be evaluated in this article, or claim that may be made by its manufacturer, is not guaranteed or endorsed by the publisher.
The Supplementary material for this article can be found online at: https://www.frontiersin.org/articles/10.3389/feduc.2024.1270484/full#supplementary-material
1. ^Learning difficulties is used in this paper as a generic term for all forms of impaired academic performance and describes particular difficulties in dealing with learning requirements of all kinds that can vary in severity from mild to severe and from temporary to persistent (Gold, 2018, p. 10, p. 12). Specific learning disabilities, such as dyslexia or dyscalculia, are also included in this definition.
2. ^See the Supplementary materials for a table summarizing the research design of the six studies and their results.
3. ^The Leipzig Competence Screening was standardized on the basis of 1,450 cases. The reliability values (i.e., internal consistency) of the individual scales are between 0.7747 and 0.93242 and are acceptable to excellent (Hartmann and Methner, 2015, p. 30).
Aschermann, E., and Armbrüster, C. (2011). get involved – Persönliche Kompetenzen erkennen und fördern. Implementierung und Evaluation eines Programms zur Förderung von selbstgesteuertem Lernen an Schulen in Köln/Bonn im Rahmen des Schwerpunktes Individuelle Förderung. Abschlussbericht des Forschungsberichts. Germany: Universität zu Köln.
Bedesem, P. L. (2012). Using cell phone Technology for Self-Monitoring Procedures in inclusive settings. J. Spec. Educ. Technol. 27, 33–46. doi: 10.1177/016264341202700403
Bedesem, P. L., and Dieker, L. A. (2013). Self-monitoring with a twist: using cell phones to CellF-monitor on-task behavior. J. Posit. Behav. Interv. 16, 246–254. doi: 10.1177/1098300713492857
Briesch, A. M., and Chafouleas, S. M. (2009). Children’s usage rating profile (predicted). Storrs, CT: University of Connecticut.
Brossart, D. F., Laird, V. C., and Armstrong, T. W. (2018). Interpreting Kendall’s tau and tau-U for single-case experimental designs. Cogent Psychology 5, 1–26. doi: 10.1080/23311908.2018.1518687
Bruhn, A., McDaniel, S., and Kreigh, C. (2015). Self-monitoring interventions for students with behavior problems: a systematic review of current research. Behav. Disord. 40, 102–121. doi: 10.17988/BD-13-45.1
Bruhn, A. L., Woods-Groves, S., Fernando, J., Choi, T., and Troughton, L. (2017). Evaluating technology-based self-monitoring as a tier 2 intervention across middle school settings. Behav. Disord. 42, 119–131. doi: 10.1177/0198742917691534
Casale, G., Huber, C., Hennemann, T., and Grosche, M. (2019). Direkte Verhaltensbeurteilung in der Schule. Eine Einführung für die Praxis. München: Ernst Reinhardt Verlag.
Chafouleas, S. M., Briesch, A. M., Neugebauer, S. R., and Riley-Tillman, T. C. (2011). Usage rating profile - intervention (revised). Storrs, CT: University of Connecticut.
Cheng, M., and Yuen, A. H. K. (2019). Cultural divides in acceptance and continuance of learning management system use: a longitudinal study of teenagers. Educ. Technol. Res. Dev. 67, 1613–1637. doi: 10.1007/s11423-019-09680-5
Clemons, L. L., Mason, B. A., Garrison-Kane, L., and Wills, H. P. (2016). Self-monitoring for high school students with disabilities: a cross-categorical investigation of I-connect. J. Posit. Behav. Interv. 18, 145–155. doi: 10.1177/1098300715596134
Davis, F. D., Bagozzi, R. P., and Warshaw, P. R. (1989). User acceptance of computer technology: a comparison of two theoretical models. Manag. Sci. 35, 982–1003. doi: 10.1287/mnsc.35.8.982
Dowdy, A., and Jessel, J. (2021). “Single case research designs” in Research methods in special education. eds. B. L. Hott, F. J. Brigham, and C. Peltier (Thorofare, NJ: SLACK Incorporated), 165–198.
Granić, A., and Marangunić, N. (2019). Technology acceptance model in educational context: a systematic literature review. Br. J. Educ. Technol. 50, 2572–2593. doi: 10.1111/bjet.12864
Hartmann, B., and Methner, A. (2015). Leipziger Kompetenz-Screening für die Schule (LKS). Diagnostik und Förderplanung: soziale und emotionale Fähigkeiten, Lern- und Arbeitsverhalten. München: Ernst Reinhardt.
Helmke, A., and Renkl, A. (1992). Das Münchener Aufmerksamkeitsinventar (MAI): Ein Instrument zur systematischen Verhaltensbeobachtung der Schüleraufmerksamkeit im Unterricht. Diagnostica 2, 130–141.
Jain, A., and Spieß, R. (2012). Versuchspläne der experimentellen Einzelfallforschung. Empirische Sonderpädagogik 3/4, 211–245. doi: 10.25656/01:9300
Landmann, M., and Schmitz, B. (2007). Die Kombination von Trainings mit standardisierten Tagebüchern: Angeleitete Selbstbeobachtung als Möglichkeit der Unterstützung von Trainingsmaßnahmen. In M. Landmann & B, Schmitz (Hrsg.), Selbstregulation erfolgreich fördern. Praxisnahe Trainingsprogramme für effektives Lernen (S. 151–159). Stuttgart: Kohlhammer
Luan, W. S., and Teo, T. (2009). Investigating the technology acceptance among student teachers in Malaysia: an application of the technology acceptance model (TAM). Asia Pac. Educ. Res. 18, 261–272. doi: 10.3860/taper.v18i2.1327
Matthes, G. (2018). Förderkonzepte einfühlsam und gelingend. Psychologische Grundlagen und Methoden der Entwicklung individueller Förderkonzepte. Germany: Dortmund.
Miller, B., Doughty, T., and Krockover, G. (2015). Using science inquiry methods to promote self-determination and problem-solving skills for students with moderate intellectual disability. Education and Training in Autism and Developmental Disabilities 50, 356–368.
Mooney, P., Ryan, J. B., Uhing, B. M., Reid, R., and Epstein, M. H. (2005). A review of self-management interventions targeting academic outcomes for students with emotional and behavioral disorders. J. Behav. Educ. 14, 203–221. doi: 10.1007/s10864-005-6298-1
Niesyn, M. E. (2009). Strategies for success: evidence-based instructional practices for students with emotional and behavioral disorders. Prev. Sch. Fail. 53, 227–234. doi: 10.3200/PSFL.53.4.227-234
Parker, R. I., Hagan-Burke, S., and Vannest, K. (2007). Percentage of all non-overlapping data (PAND). J. Spec. Educ. 40, 194–204. doi: 10.1177/00224669070400040101
Pötters, B., Flüchter, I., and Melzer, C. (2020). Self-Monitoring als Möglichkeit der Diagnostik und Intervention bei Lernbeeinträchtigungen. Pädagogische Handlungsmöglichkeiten auf der Grundlage empirischer Erkenntnisse. Zeitschrift für Heilpädagogik 71, 100–112.
Rosenbloom, R., Mason, R. A., Wills, H. P., and Mason, B. A. (2016). Technology delivered self-monitoring application to promote successful inclusion of an elementary student with autism. Assist. Technol. 28, 9–16. doi: 10.1080/10400435.2015.1059384
Schardt, A. A., Miller, F. G., and Bedesem, P. L. (2018). The effects of CellF-monitoring on students’ academic engagement: a technology-based self-monitoring intervention. J. Posit. Behav. Interv. 21, 42–49. doi: 10.1177/109830071877346
Teo, T. (2010). A path analysis of pre-service teachers' attitudes to computer use: applying and extending the technology acceptance model in an educational context. Interact. Learn. Environ. 18, 65–79. doi: 10.1080/10494820802231327
Tröster, H. (2019). Diagnostik in schulischen Handlungsfeldern. Methoden, Konzepte, praktische Ansätze. Stuttgart: Kohlhammer.
Vannest, K. J., Parker, R. I., Gonen, O., and Adiguzel, T. (2016). Single case research: Web based calculators for SCR analysis (version 2.0) [web-based application] Available at: http://singlecaseresearch.org/
Visser, L., Büttner, G., and Hasselhorn, M. (2018). Komorbidität spezifischer Lernstörungen und psychischer Auffälligkeiten. Ein Literaturüberblick. Lernen und Lernstörungen 8, 7–20. doi: 10.1024/2235-0977/a000246
Volpe, R. J., and Briesch, A. M. (2012). Generalizability and dependability of single-item and multiple-item direct behavior rating scales for engagement and disruptive behavior. Sch. Psychol. Rev. 41, 246–261. doi: 10.1080/02796015.2012.12087506
Wilbert, J., Börnert-Ringleb, M., and Lüke, T. (2022). Statistical power of piecewise regression analyses of single-case experimental studies addressing behavior problems. Frontiers in Education 7:917944. doi: 10.3389/feduc.2022.917944
Wills, H. P., and Mason, B. A. (2014). Implementation of a self-monitoring application to improve on-task behavior: a high-school pilot study. J. Behav. Educ. 23, 421–434. doi: 10.1007/s10864-014-9204-x
Keywords: self-monitoring, self-regulated learning, mobile application, learning difficulties, single case research
Citation: Melzer C and Herwix A (2024) App-based self-monitoring as an intervention to support attention in students with learning difficulties. Front. Educ. 9:1270484. doi: 10.3389/feduc.2024.1270484
Received: 31 July 2023; Accepted: 04 January 2024;
Published: 30 January 2024.
Edited by:
Miriam Balt, University of Wuppertal, GermanyReviewed by:
Joanne Mosen, The University of Melbourne, AustraliaCopyright © 2024 Melzer and Herwix. This is an open-access article distributed under the terms of the Creative Commons Attribution License (CC BY). The use, distribution or reproduction in other forums is permitted, provided the original author(s) and the copyright owner(s) are credited and that the original publication in this journal is cited, in accordance with accepted academic practice. No use, distribution or reproduction is permitted which does not comply with these terms.
*Correspondence: Conny Melzer, Y29ubnkubWVsemVyQHVuaS1sZWlwemlnLmRl
†These authors have contributed equally to this work and share first authorship
Disclaimer: All claims expressed in this article are solely those of the authors and do not necessarily represent those of their affiliated organizations, or those of the publisher, the editors and the reviewers. Any product that may be evaluated in this article or claim that may be made by its manufacturer is not guaranteed or endorsed by the publisher.
Research integrity at Frontiers
Learn more about the work of our research integrity team to safeguard the quality of each article we publish.