- 1Engineering Education Transformations Institute, University of Georgia College of Engineering, Athens, GA, United States
- 2College of Education, Washington State University, Pullman, WA, United States
- 3Department of Kinesiology, College of Education, University of Georgia, Athens, GA, United States
Introduction: The ability to understand text is influenced by various factors, including the learner’s cognitive abilities and previous experiences. This study examined the correlation between two pre-existing learner characteristics, three comprehension strategies used while reading, and two cognitive outcomes related to text comprehension.
Methods: The study involved 109 undergraduate Biology students, who were instructed to perform think-aloud exercises while reading biology texts and complete post-reading tests. The participants typed out their thoughts about the text when prompted. Their verbal protocols were coded and analyzed.
Results: Statistically significant correlations were only observed between prior knowledge, presage skill, recall, and comprehension. The direct relationships between prior knowledge and cognitive strategies were not significant, and the relationship between participants’ presage skills and the comprehension strategies they employed while reading was not significant.
Discussion: The study revealed that prior knowledge had a more significant impact on cognitive learning outcomes than the students’ prior cognitive skills and the comprehension strategies they employed while reading. Additionally, the participant’s performance on the learning outcomes was influenced by at least one of the comprehension strategies and their prior knowledge.
1 Introduction
Whether students comprehend scientific text often depends on the complexity of the text, the difficulty of a science topic, and how learners engage with the text (Sinatra and Broughton, 2011; Kurby et al., 2012). For example, some students may find reading science texts challenging to comprehend because scientific texts can be replete with unfamiliar technical terms. Furthermore, authors of science texts often expect their readers to have prerequisite knowledge that their students may not already have (O’Reilly and McNamara, 2007; O’Reilly et al., 2019). To forge understanding, students with low or no prior knowledge about the topic of science text would often need to generate inferences to overcome their knowledge gaps and ensure they comprehend the text they read (Diakidoy et al., 2011; Kendeou et al., 2016). However, students can only generate the inferences necessary to foster text comprehension if they have the cognitive and metacognitive skills to monitor and facilitate comprehension while reading. However, some students may lack the ability to use such cognitive and meta-cognitive skills.
Comprehension problems are not limited to a lack of cognitive and meta-cognitive skills alone. Alternatively, some learners may have misconceptions about topics addressed in the science text they read, which may constitute additional barriers to comprehending the texts (Diakidoy et al., 2011; Kowalski and Taylor, 2017; Smith et al., 2021). For example, a student who wrongly believes that biological traits, such as eye color, are determined by single genes may struggle to unlearn such misconceptions to embrace the fact that traits are caused by a combination of different genes. Reading comprehension (a learning outcome) is affected by the quality of the learning processes or activities students engage in while reading. Similarly, the processes or activities that readers engage in while reading may depend on presage factors they bring to the learning situation. Presage factors (defined shortly) affect both the cognitive or non-cognitive processes of reading and the products of learning (Bohn-Gettlera and Kendeou, 2014).
This study uses Brigg’s 3-P (Presage-Process-Product) model of teaching and learning to explore whether three comprehension monitoring processes mediated the correlations between two cognitive factors (prior information processing skills and prior knowledge) and products of text comprehension when students read a text on genetics. Prior information processing skills will be referred to as Skills throughout the rest of the manuscript for brevity. The Learning and Strategies Inventory operationalizes information processing and selecting main ideas from learning content as strategic learning Skills (Weinstein et al., 2011). The 3-P model posits that the products of learning are affected by factors that occur before (presage factors) and during learning (learning processes and activities). Presage factors refer to variables that exist before any learning engagement even begins. They may include pre-existing factors inherent in the learner or learning environmental (or situational) factors that can impact quality learning engagement. Presage factors can significantly affect the learning processes and outcomes (Zhang, 2010). In text-based learning, presage factors may include prior knowledge, reading study strategies, and student reading goals. These variables exist with the students before the learning situation (Ozuru et al., 2009). Processes may include cognitive activities (e.g., paraphrasing and inference generation) and meta-cognitive activities (e.g., self-regulatory functioning) that learners engage in to overcome barriers to text comprehension (Clinton, 2014; Lupo et al., 2019). Lastly, products may include learning outcomes such as conceptual understanding, recall, and knowledge transfer (Diakidoy et al., 2016).
Many studies have examined relationships between learning processes and products, or different presage factors and learning products in isolation or by pairing any of these relationships (e.g., Diakidoy et al., 2011, 2016). However, very few studies have done so while considering the interactions of presage, process, and product variables that may be relevant to the learning context (e.g., Cromley and Azevedo, 2007; Cromley et al., 2010). Exploring the relative significance of presage factors and cognitive processes that foster text comprehension could have significant implications for intervention for text comprehension. For example, whether presage factors (e.g., domain prior knowledge) have more or less effects on text comprehension than process factors (e.g., inference generation) can affect how readers are primed to foster comprehension. Hence, a study that examines the relative effects of presage and process factors on text comprehension could highlight essential considerations for text comprehension research and intervention.
The current study examines the interrelationships between two presage variables, three reading comprehension processes or activities, and two learning outcomes that are relevant to learning from science texts. The following section will highlight important theoretical perspectives about these variables and their relationships.
1.1 Theoretical frameworks
1.1.1 Essentials of classical text processing models
Text comprehension occurs when readers assimilate emerging textual information into their prior knowledge to construct a coherent mental representation of the text (Diakidoy et al., 2011). According to Kintsch (1998), text processing comprises a construction and an integration phase. In the construction phase, a reader takes in a series of sentences parsed into proposition units. Each incoming proposition unit activates relevant linguistic and domain-specific prior knowledge and all relevant proposition units from previous processing cycles that are still active in working memory to facilitate the construction of coherent arguments (Sinatra and Broughton, 2011). Text coherence happens when arguments in adjacent proposition units overlap—a coherence break occurs when arguments fail to overlap. A lack of such overlap inhibits learners from being able to construct a coherent mental representation of the text that is necessary for text comprehension.
1.1.2 Text processing and comprehension difficulties
Readers comprehend texts if they can attain local and global coherence (Diakidoy et al., 2011). Local coherence describes the degree to which the reader can conceptually relate proximally situated proposition units. Global coherence is attained when the reader can relate distal proposition units to build thematic representations of the text (Diakidoy et al., 2011). Learners may use information that emerges from subsequent text processing cycles and any relevant prior knowledge that the text activates in their working memory to resolve any incoherence in their mental representation of the text.
Furthermore, whether comprehension occurs depends on whether learners can integrate the coherent mental model they construct from reading with their prior knowledge. Many readers frequently experience coherence breaks while reading texts on science matters because such texts activate many unfamiliar terminologies in their short-term memory when they process scientific text.
1.1.3 Process-to-product: the role of strategic cognitive processing in text comprehension
Based on the preceding, good comprehenders use strategic comprehension monitoring skills, such as paragraphing and generating, to fill gaps in their mental model when they experience coherence break (Diakidoy et al., 2011; Leopold and Leutner, 2015). For example, a reader might paraphrase all or part of the ideas in a text in their own words to deduce meaning from unfamiliar words or contexts to enhance comprehension (Gilliam et al., 2007; Stevens et al., 2020). Paraphrasing enables the reader to represent the discourses processed in more relatable terms to enhance comprehension (McNamara et al., 2007).
Readers may need to compensate for coherence gaps during text processing by making bridging and elaborative inferences. Readers make bridging inferences when they connect newly activated text information with discourses from prior activation that are still active in their working memory or with distal discourses in episodic memory to establish referential, logical, and causal relationships between ideas emerging from ongoing discourse in the text (Diakidoy et al., 2011). Elaboration involves enriching the text discourse being processed with relevant background knowledge to enhance understanding (Gilliam et al., 2007). Research shows that text comprehension is inhibited when readers are unable to use these strategies to enable them to process and integrate textual information with their prior knowledge (Dornisch et al., 2011; Diakidoy et al., 2016; Hagaman et al., 2016; O’Reilly and Sabatini, 2016).
1.1.4 Presage factors and the process-product relationship
Presage factors relevant to the learning situation may also influence text comprehension. For example, the quantity and quality of learners’ prior knowledge could be functional in text processing and reading comprehension outcomes (Diakidoy et al., 2011). Research has shown that prior knowledge influences how much inference readers generate while reading (Tarch, 2015) and their ability to recall the main ideas from text (Millis and Graesser, 1994). The quality of prior knowledge affects scientific understanding (Vosniadou, 2013).
Similarly, information processing skills and metacognitive awareness of strategies that enhance comprehension may influence cognitive processes and reading outcomes (Mokhtari and Reichard, 2002). Comprehension depends upon a self-regulated use of cognitive and metacognitive skills that enhance learning. Learners use strategic skills—such as verbal elaborations and imagery, selecting or organizing main ideas from the texts—when reading to bridge gaps in their prior knowledge and the content of the learning material (Weinstein et al., 2011). However, the degree to which they use such strategies while processing a text may depend on their predisposition to invoke them when needed. Invariably, the cognitive skills (presage factors) that students bring to learning and their awareness of when to use them could impact their cognitive processing and learning outcomes. Hence, some presage student variables could directly or indirectly affect the learning process and the product of learning.
1.1.5 A 3-P framework of text-based learning based on a strategic learning model
Weinstein et al. (2011) proposed that three components (Skill, Will, and Self-regulation) are essential to strategic learning. They defined Skill components of strategic learning as “critical knowledge about, and knowing how to use, learning strategies and other thinking skills.” These include awareness of the strategies needed to enhance performance in different academic tasks and effectively study different types of content material. The Will component includes motivational and affective variables that influence strategic learning, and Self-regulation includes monitory and regulatory strategies that enhance learning (Weinstein et al., 2011). Through the lens of Brigg’s 3-P learning model, Weinstein’s components of strategic learning can be construed as presage variables that learners bring to a learning environment that affects their learning processes and products.
1.2 The present study
Many prior studies have focused on the process-product (2-P) relationships of text-based learning. However, how the relationships observed between cognitive activities (processes) and learning outcomes (products) are moderated by presage factors are less often examined. Ranellucci et al. (2013) examined the effect of students’ achievement goal orientations and the depth of text processing on conceptual change. Specifically, they examined relationships between prior knowledge and achievement goal orientation (two presage variables), deep and shallow cognitive processing (learning process variables), and recall and conceptual understanding (two learning outcomes variables). They found direct relationships between mastery-approach goal orientation, deep processing strategies, shallow processing strategies, and conceptual change. They further highlighted the extent to which deep and shallow processing mediated the relationship between mastery-approach goals and conceptual change (Ranellucci et al., 2013). By focusing on the three 3-Ps in the learning context, their study shed more light on the causal links between students’ goal orientations, cognitive processes, and learning outcomes observed in their study. Bråten et al. (2014) examined relationships between individual differences, processing, and multiple-text comprehension in a different but related study based on a similar conceptual framework.
The present study explores whether the effects of two presage variables (prior knowledge and cognitive skill) on learning outcomes (recall and conceptual understanding) are mediated by the quantity of the paraphrasing, bridging, and elaborative inferences students made while reading a text on genetic biology. Hence, the study explores a model that hypothesizes the relationship between the variables based on the research questions below:
Research Question 1. What are the relationships between participants’ prior knowledge, the comprehension strategies they used while reading, and measures of text comprehension?
Research Question 2. What are the relationships between participants’ presage skills, the comprehension strategies they used while reading, and measures of text comprehension?
Research Question 3. What are the relationships between the comprehension strategies that participants used while reading and measures of text comprehension?
Research Question 4. Did the comprehension strategies used by participants in the study mediate the relationships between prior knowledge and skill and measures of text comprehension?
1.2.1 Hypothesized model
Drawing on extant theoretical and empirical studies of text comprehension, we hypothesized that the comprehension strategies (paraphrases and inferences) that participants generate while reading would be positively related to conceptual understanding. Specifically, because paraphrasing is considered a lower-order cognitive activity (Magliano et al., 2011), we hypothesized that the number of paraphrases participants generate as they process text will have a stronger positive correlation with recall than comprehension. Making bridging and elaboration inferences indicates deeper cognitive activities during text processing. Hence, we hypothesized that they would have a stronger direct correlation with conceptual understanding than recall (Magliano et al., 2011).
Prior research indicates that prior knowledge is often positively related to conceptual understanding (Tarch, 2015). Some other studies have suggested that the prior knowledge effect on recall is, at best, minimal (Erçetin, 2010). A similar argument was proposed by van den Broek (1994) in contrasting recall and summarization—the author argued that summarization requires an extensive probing of prior knowledge, while recall minimally engages inference and prior knowledge as learners probe a propositional textbase. Because recall puts less cognitive demand on memory, we predicted that the relationship between prior knowledge and recall would not be significant. Based on earlier studies, we anticipated that students’ awareness and predisposition to use cognitive processing skills (e.g., being able to select main ideas, use imagery, and perform executive functioning) would have a significant direct relationship with students’ ability to generate bridge and elaborative inferences (Mokhtari and Reichard, 2002), but would have no an indirect relationship with the learning outcomes we measured. Lastly, extant research suggests that prior knowledge is germane to making elaborative inferences (Gilliam et al., 2007). We anticipated that prior knowledge would have a significant direct effect on elaboration. Figure 1 depicts a conceptual model summarizing our hypothesis about the relationship between the variables. The dashed lines in the figure indicate that the hypothesized relationships between two variables are not statistically significant, while the solid lines indicate that hypothesized relationships are statistically significant.
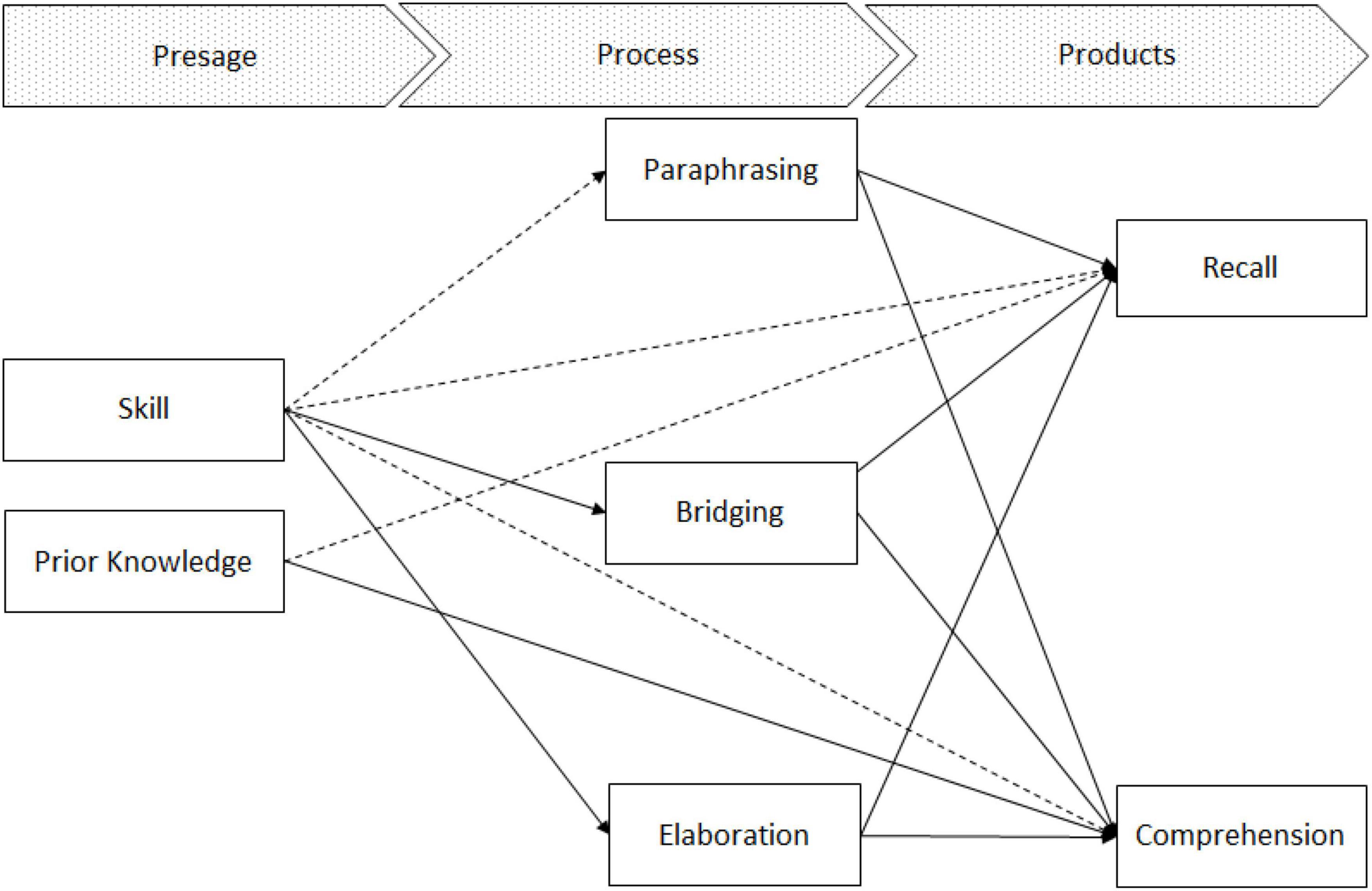
Figure 1. Hypothesized path model of presage variables, comprehension processes, and learning outcomes.
2 Materials and methods
2.1 Participants
Participants were 109 undergraduate students (82 females and 27 males) who enrolled in an introductory biology course at a large public research university in the Pacific Northwest of the United States. The study was conducted in the context of the weekly lab components of the course. Participation was voluntary, and participants could discontinue at any point in the study.
2.2 Materials
2.2.1 Experimental text
Participants read texts that addressed misconceptions commonly held by students in introductory biology classes. The researcher and the course instructor (the content expert) developed the texts for this study. The Dale-Chall readability index indicated that the texts used in this study were comprehensible for students in the eleventh grade.
2.2.2 Measurements
Presage Variables Measures: Presage variables included prior knowledge and the Skill components of strategic learning measured using items on the third edition of the Learning and Study Strategies Inventory (LASSI). Constructs related to the Skill components of strategic learning assessed by the LASSI include Information Processing, Selecting Main Ideas, and Test Strategies. We used only items from the Information processing (six items) and the Selecting main ideas (six items) subscales to assess the skill component of strategic learning of participants in our study. We selected only those items because they were the most relevant to the learning context in which participants in our study engaged. Items on the instruments are measured on a five-point Likert-type scale ranging from not at all typical of me (1) to very much typical of me (5). An estimate of the LASSI subscales’ internal reliability was acceptable (Cronbach’s alpha = 0.83).
Learning process measures—type-aloud protocol. A total of 872 typed responses (eight responses per participant) were coded and analyzed. Because the participants’ responses we needed to code were much, the Reading Strategy Assessment Tool (RSAT) was used to compute scores for the amount of paraphrasing, bridging, and elaboration participants produced as they typed their thoughts in responses to the prompts (verbal protocols). The RSAT scoring protocol uses a word-matching algorithm that matches participants’ responses with words in the assigned texts (Magliano et al., 2011). The algorithm scores paraphrasing using the sum of similar words from the text participants read that showed up in their typed responses. Bridging is scored using the sum of content words in participants’ verbal responses from the sentence immediately before a target sentence in the assigned text. Elaboration is scored using the sum of content words in participants’ typed responses not found in the text passage.
Learning product measures—Knowledge Inventory: The prior knowledge instrument used in the study was taken from the Genetics Literacy Assessment, an established genetic concept inventory (Bowling et al., 2008). Split-half analysis was conducted to determine the internal reliability coefficients of the test. The reliability of questionnaire data is estimated using a range of coefficients (e.g., Cronbach’s alpha). However, such coefficients may not be well suited to some cognitive tasks. Some authors have argued that the split-half reliability analysis is better suited for estimating the reliability of cognitive tasks (Steinke et al., 2021; Pronk et al., 2022).
The analysis showed that the Spearman-Brown split-half coefficient was 0.68. Between form correlation was 0.51 for the pre-test. A post-intervention inventory was used to assess students’ retention and conceptual knowledge of genetics. The recall test consisted of eight multiple-choice items that assessed the retention of facts from the texts, and recall scores ranged between 0 and 8. The genetic knowledge comprehension scores also ranged between 0 and 8. Split-half analysis to determine the internal reliability coefficients of the post-test showed that the Spearman-Brown split-half coefficient was 0.77, and between forms correlations were 0.63 for the pre-test.
2.3 Procedure
Participants took the LASSI survey online in the first weeks before the main study activities. A prior knowledge test was administered during class activities in the second week. They received a link to the experimental platform designed on Qualtrics® during their lab section in week four of the study as part of their lab exercises. The platform included an introductory biology text on genetics and a post-test. As participants read through the text, they were prompted to reflect on what they had read and type out their thoughts about the texts they had just read whenever they saw the prompt “What are you thinking about now?” at designated segments of the text. This procedure was adopted from the “think-aloud procedure” of earlier text comprehension studies that asked participants to type out their thoughts (Gilliam et al., 2007; Magliano et al., 2011).
The Qualtrics® platform began with an instruction section to familiarize participants with navigating the rest of the experimental platform. The instruction pages also included examples of good and poor typed responses to the prompts that asked them to think and type their thoughts. In addition to the examples, the participants also had practice samples before the actual study activities to familiarize them with the main activities of the study. Once they had completed the practice sections, they could progress and read through the text for the main study one page (screen) at a time. Upon completing the reading assignment, participants completed a post-intervention knowledge inventory that assessed their recall of the main ideas from the text and their comprehension of the text.
3 Data analysis and results
Before data analyses, we examined all variables for data entry accuracy, outliers, and normality of distributions. Preliminary analyses included computing descriptive statistics, such as means, standard deviations, and Pearson’s correlation coefficients. Missing data comprised 8.7% of all cases, and similarities between the complete sample and missing data on the variables were examined with independent sample t-tests. Cross-sectional theory-based path models were constructed to answer the research question. The term “path model” indicates a special case of structural equation modeling (SEM) in which only single indicators are employed for each variable in the causal model. Path analysis is an SEM with a structural but not a measurement model (Lei and Wu, 2007). All analyses were performed using the Mplus statistical package (Version 7.31; Muthén and Muthén, 2021). A good fit between the hypothesized model and the observed data (Hu and Bentler, 1999) is observed if the value of the Bentler comparative fit index (CFI) and Tucker-Lewis index (TLI) are above 0.95, and the value of the Root Mean Squared Error of Approximation (RMSEA) is below 0.06.
An independent sample t-test showed no differences between the baseline values of participants with no missing values and those with missing values (t-values ranging from 0.416 to 1.356), indicating that the missing values were missing at random. The Cronbach’s alphas observed were comparable to those reported in the LASSI user’s manual Information Processing (α = 0.82) and Selecting Main Ideas (α = 0.84). Descriptive statistics and correlation matrix are presented in Table 1.
Statistically significant correlations were only observed between prior knowledge, presage skill, recall, and comprehension. The result of the original model (Figure 1) conducted with the Mplus statistical package yielded a poor model fit: (χ2 (3) = 19.59; p < 0.001; CFI = 0.80; TLI = 0.33; RMSEA = 0.231; CI 90% [0.14, 0.33]). The initial model was modified post hoc by specifying prior knowledge as a moderator instead of a predictor variable (Figure 2) to explore causal relationships between elaboration, bridging, and paraphrasing. The modified model had a marginally good data fit: (χ2 (1) = 1.79; p = 0.181; CFI = 0.99; TLI = 0.89; RMSEA = 0.087; CI 90% [0.01, 0.29]).
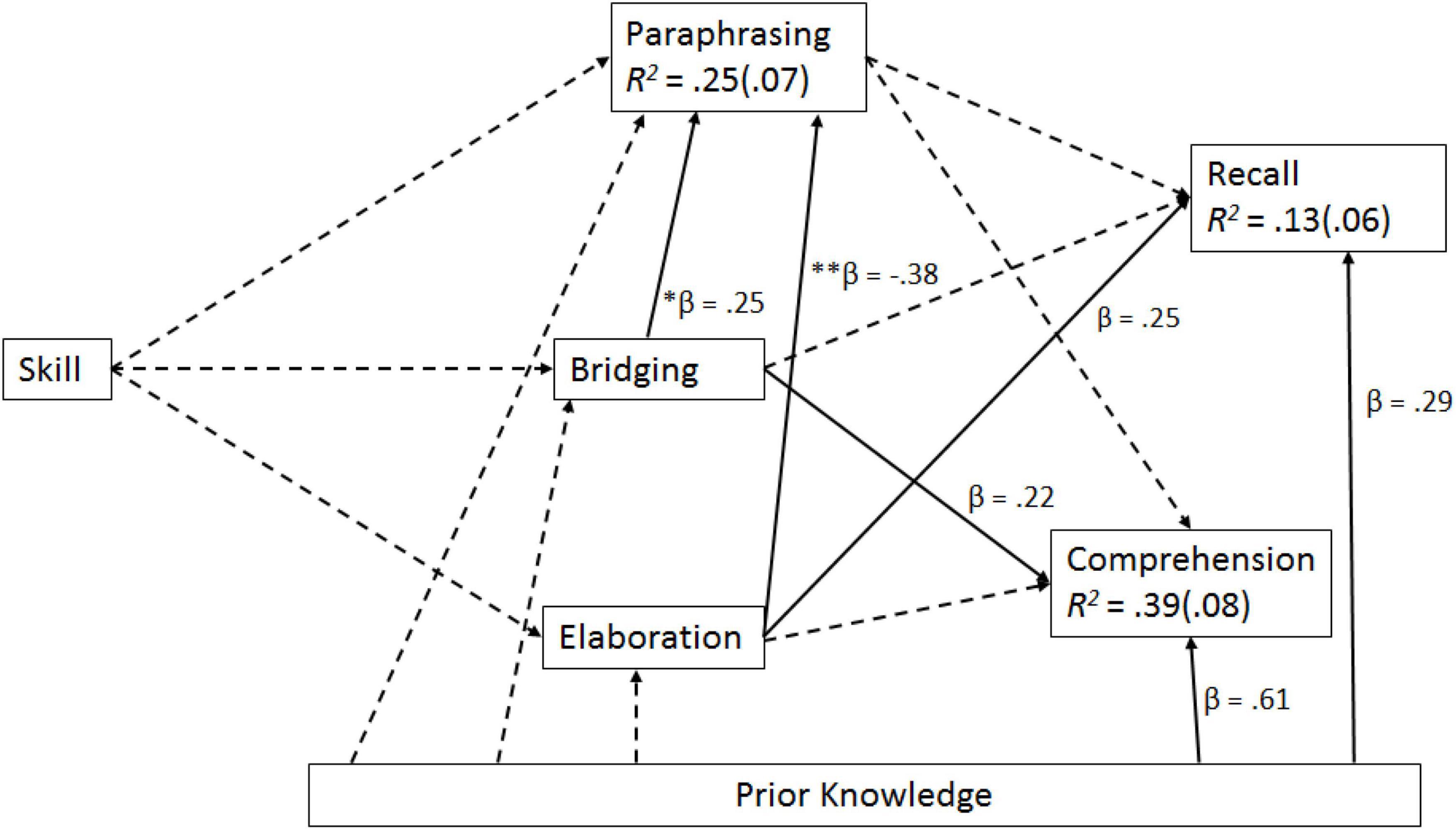
Figure 2. Estimated path model of presage variables, comprehension processes, and learning outcomes. *Significant at p < 0.05, **significant at p < 0.01.
With regard to the first research question, the findings supported the alternative hypothesis, which showed that prior knowledge was directly related to comprehension (β = 0.61) and recall (β = 0.29). The direct relationships between prior knowledge and cognitive strategies were not significant. In the second research question, the relationship between participants’ presage skills and the comprehension strategies they employed while reading was not significant; neither were the relationships between skills and learning outcomes significant. On the third research question, the analyses showed that elaboration predicted recall (β = 0.25) but not comprehension, and bridging predicted comprehension (β = 0.22) but not recall. In addition to the predicted paths, the ad hoc suggested bridging to be a positive predictor of paraphrasing (β = 0.25) and elaboration to be a negative predictor of paraphrasing (β = −0.38). The analyses indicated that the predictor variables predicted 25% variance in paraphrasing (R2 = 0.25 [0.07]), 13% in recall (R2 = 0.13 [0.06]), and 39% in comprehension (R2 = 0.39 [0.08]). The model resulting from the analysis is shown in Figure 2.
With regard to the final research question, we could not establish a mediation effect of the comprehension strategies on the relationships between any of the presage variables and the text comprehension variables. Prior knowledge was used as a covariate in our analysis of the modified final model.
Following this analysis above, we conducted a post hoc correlational analysis to investigate how the relationships between the process and outcome variables compared for students whose prior knowledge of cell genetic concepts (N = 59) was significantly higher and lower than average. Hence, we created two categories of participants based on their prior knowledge scores: those whose prior knowledge scores were below one standard deviation of the group mean score (Low prior knowledge, N = 26) and those whose prior knowledge scores were above one standard deviation of group mean score (High prior knowledge, N = 32).
Subsequently, bivariate correlational analyses were examined between the variables for each prior knowledge category. The result of the correlations between the variables for students with low and high prior knowledge is shown in Table 2.
4 Discussion
This study examined the relationships between two presage variables that students brought to a biology learning engagement and some process-product variables. We observed a moderately significant correlation between prior knowledge and text comprehension. Prior knowledge was also positively correlated with recall and participants’ presage skills needed in reading for comprehension. In addition, how much participants recalled was moderately correlated with the measure of comprehension observed in the study. All of the other relationships were not statistically significant.
We found that neither prior knowledge nor the skill components of strategic learning examined in this study predicted any text-processing activities (paraphrasing, bridging, and elaboration) that participants employed while processing or reading the text. Prior knowledge failed to predict paraphrasing and bridging, perhaps because both process variables are less dependent on students’ prior knowledge. This observation corroborates earlier views that students with high reading goals will expend metacognitive strategies (such as paraphrasing and bridging) to ensure they comprehend what they read, regardless of how much prior knowledge they have (Bohn-Gettlera and Kendeou, 2014). Because prior comprehension models suggest learners draw on their prior knowledge in making elaborative inferences (Gilliam et al., 2007), we anticipated that prior knowledge would have some effect on participants’ use of elaboration. However, that expectation was not supported by the data in this study. On the contrary, prior knowledge did not predict the use of elaboration among participants observed in our study. Perhaps this was due to a flooring effect in participants’ prior knowledge—since the mean score on the prior knowledge measure was already. Alternatively, it is also possible that students relied extensively on general background knowledge instead of domain-specific prior knowledge when making elaborative inferences.
Participants’ prior knowledge about genetics predicted recall and comprehension (learning product variables) observed in the study. We observed that prior knowledge and bridging jointly accounted for about 40% of the variance in participants’ comprehension scores. This finding is consistent with extant literature that shows prior knowledge has a considerable effect on cognitive learning outcomes (Chi, 2013). Apart from prior knowledge, Magliano et al. (2011) posited that text comprehension is affected by readers’ capacity to generate bridging inferences. Prior knowledge and Elaboration scores accounted for 13% of the variance in participants’ recall scores. Theorists argue that making elaborative inferences is a higher-order, more mentally demanding cognitive process involving drawing on learners’ prior knowledge (Gilliam et al., 2007; Diakidoy et al., 2011). We observed from this study that making elaborative inferences while reading facilitated recall. Perhaps learners drew on prior knowledge to facilitate elaborations, which strengthened the mental connections they made with the text contents and thus could recall more. However, we could not validate this perspective from the data used in this study.
Based on the observation in this study, participants’ presage skills measured on the LASSI neither predicted any of the learning processing nor the learning product variables investigated—perhaps because the presage skill components of strategic learning that were measured in this study were not particularly relevant to the difficulty level of the learning task that the average participants experienced. That notwithstanding, it appears that the presage skill variable may have been needed by students with low prior knowledge of the domain who needed to compensate for their low prior knowledge to aid their comprehension of the test material. We conducted a post hoc analysis to explore the relationship between these variables for students who scored one standard deviation below or above the sample mean prior knowledge score to examine this assumption further. The result in Table 2 indicates that the Skill component variable had higher correlations with bridging, recall, and elaboration for participants with the lowest prior knowledge scores compared to those with the highest prior knowledge scores. Future studies may explore these relationships and the relevance of presage strategic learning skills for students with low prior knowledge.
Both bridging and elaboration contributed significantly to participants’ recall or comprehension scores. However, paraphrasing scores did not predict either of the two learning outcomes. Some have argued that paraphrasing is less cognitively engaging than bridging and elaborating inferences (Magliano et al., 2011). The result also indicated that paraphrasing was negatively correlated with elaboration. This could imply that students who paraphrase more may also engage less in other cognitive strategies that require deeper cognitive engagement they need to integrate the learning material into learners’ prior knowledge. The cognitive process variables did not mediate the presage-product relationships observed in this study as expected.
4.1 Implications of study findings
In summary, prior knowledge and bridging predicted participants’ comprehension scores, while prior knowledge and elaboration predicted recall scores. However, domain-based prior knowledge did not significantly predict the comprehension strategies participants used during text processing. This observation is consistent with prior empirical or theoretical conjectures about the effects of prior knowledge and the three process variables on learning products. Text processing skills (such as paraphrasing and inference-making) were significant predictors of comprehension. However, the effects of domain prior knowledge on these skills were insignificant. We infer from this observation that being deficient in domain-based prior knowledge would not limit students’ ability to use strategic cognitive skills to foster comprehension when reading science texts. However, students need to know what and how to use reading skills that facilitate comprehension.
Students who need both the requisite domain prior knowledge in science fields and the strategic comprehension skills essential for processing text may find science texts challenging to read. We infer from the preceding that the efficacy of interventions aimed at training or scaffolding students to use deep comprehension strategies may not be impacted by a lack of relevant domain prior knowledge. Hence, such students’ effort to hone their text comprehension skills is unlikely to be undermined by their domain-specific prior knowledge deficiency. As such, instead of focusing on cognitive factors that foster reading comprehension, reading intervention can also be focused on affective variables such as reading goals, task value, and learning motivation (Mokhtari and Reichard, 2002) because affective variables can also play equally substantive roles in whether students are deeply engaged in efforts that foster comprehension than prior knowledge.
4.2 Limitations and future research directions
Relative to the number of variables specified in the hypothesized model, the study was based on a relatively small sample size (N = 108). Consequently, the study may have lacked the statistical power to observe significant effects across variables. Hence, the failure to observe significant effects between some variables may have been due to a lack of statistical power.
In this study, the presage skill component of strategic learning was based on a subjective rather than an objective measure. Some researchers have questioned the reliability of students’ self-reports (Schellings and Van Hout-Wolters, 2011). While reliability coefficients indicate that the scales used in this study have good internal consistency reliability, the veracity of the Skill components scale on the LASSI has been questioned because the authors of the scale based their validity claim only on expert opinion (Prevatt et al., 2006). The presage skill component of strategic learning measured on the LASSI did not predict readers’ text-processing activities and the expected learning outcomes—although its relationship with both the process and outcomes variables was significant for participants with low prior knowledge of the domain. We could have used a more definitive presage cognitive construct (such as critical thinking or meta-cognition) or a more objective operationalization and measure of presage cognitive skill than the variable we used in the current study. Furthermore, we presume that other presage cognitive variables such as meta-cognition, critical thinking, epistemic cognition, topic interests, and prior knowledge could have had better explanatory relevance to the learning context that the participants in our study experienced than the more amorphous skill components that we measured in this study. Future studies could further explore the implications of prior knowledge and presage cognitive skills on learning process-product relationships using a more objective measure of cognitive processing. Future studies may also explore the interaction between these variables and process-product relationships based on a 3-P conceptual framework.
The text process variables were obtained using a typed thin-aloud protocol. However, this methodology only captures a subset of reading processes, perhaps only those consciously expressed by readers (Kendeou and van den Broek, 2007). Think-aloud procedures cannot assess other latent indicators of cognitive processes associated with the comprehension strategies learners may have engaged in while reading. The procedure used in this study could also have introduced confounds into the measurement, which cannot be ascertained (Kendeou and van den Broek, 2007; McCrudden and Kendeou, 2014). Participants were required interrupted while reading to type out their thoughts about the text they had just read. Such interruption intrudes into learners’ natural reading process, which may interfere with their cognitive processing.
Prior knowledge is pivotal to text processing and the learning gains of good comprehension skills. For example, learners’ capacity to make the elaborative inferences necessary to foster comprehension depends on their access to robust background knowledge, including domain-based and linguistic-based background knowledge (Gilliam et al., 2007). Elaboration was a better predictor of recall than comprehension in this study. Future studies could reexamine the prior knowledge effect on the process variables we examined by distinguishing between domain-based and linguistic-specific prior knowledge. Lastly, the prior knowledge effect may be more salient to comprehending science texts within the physical science knowledge domains rooted in conceptual and procedural mathematical prior knowledge.
Data availability statement
The raw data supporting the conclusions of this article will be made available by the authors, without undue reservation.
Author contributions
NH involved in conceptualizing, designing, writing, and revising the manuscript. OA involved in conceptualizing, designing, supervising, and revising the manuscript. SY-P was involved in conducting data analysis and writing the result section of the manuscript. All authors contributed to the article and approved the submitted version.
Conflict of interest
The authors declare that the research was conducted in the absence of any commercial or financial relationships that could be construed as a potential conflict of interest.
Publisher’s note
All claims expressed in this article are solely those of the authors and do not necessarily represent those of their affiliated organizations, or those of the publisher, the editors and the reviewers. Any product that may be evaluated in this article, or claim that may be made by its manufacturer, is not guaranteed or endorsed by the publisher.
Supplementary material
The Supplementary Material for this article can be found online at: https://www.frontiersin.org/articles/10.3389/feduc.2024.1239261/full#supplementary-material
References
Bohn-Gettlera, C. M., and Kendeou, P. (2014). The interplay of reader goals, working memory, and text structure during reading. Contemp. Educ. Psychol. 39, 206–219. doi: 10.1016/j.cedpsych.2014.05.003
Bowling, B. V., Acra, E. E., Wang, L., Myers, M. F., Dean, G. E., Markle, G. C., et al. (2008). Development and evaluation of a genetics literacy assessment instrument for undergraduates. Genetics 178, 15–22. doi: 10.1534/genetics.107.079533
Bråten, I., Anmarkrud, Ø, Brandmo, C., and Strømsø, H. I. (2014). Developing and testing a model of direct and indirect relationships between individual differences, processing, and multiple-text comprehension. Learn. Instruction 30, 9–24. doi: 10.1016/j.learninstruc.2013.11.002
Chi, M. T. H. (2013). “Three types of conceptual change: belief revision, mental model transformation, and categorical shift,” in Handbook of Research on Conceptual Change, ed. S. Vosniadou (Hillsdale, NJ: Erlbaum), 61–82.
Clinton, V. (2014). The relationship between students’ preferred approaches to learning and behaviors during learning: an examination of the process stage of the 3P model. Instr. Sci. 42, 817–837. doi: 10.1007/s11251-013-9308-z
Cromley, J. G., and Azevedo, R. (2007). Testing and refining the direct and inferential mediation model of reading comprehension. J. Educ. Psychol. 99, 311–325. doi: 10.1037/0022-0663.99.2.311
Cromley, J. G., Snyder-Hogan, L. E., and Luciw-Dubas, U. A. (2010). Reading comprehension of scientific text: a domain-specific test of the direct and inferential mediation model of reading comprehension. J. Educ. Psychol. 102, 687–700. doi: 10.1037/a0019452
Diakidoy, I. A. N., Mouskounti, T., Fella, A., and Ioannides, C. (2016). Comprehension processes and outcomes with refutation and expository texts and their contribution to learning. Learn. Instruction 41, 60–69. doi: 10.1016/j.learninstruc.2015.10.002
Diakidoy, I. N., Mouskounti, T., and Ioannidis, C. (2011). Comprehension and learning from refutation and expository texts. Read. Res. Q. 46, 22–38. doi: 10.1598/RRQ.46.1.2
Dornisch, M., Sperling, R. A., and Zeruth, J. A. (2011). The effects of levels of elaboration on learners’ strategic processing of text. Instr. Sci. 39, 1–26. doi: 10.1007/s11251-009-9111-z
Erçetin, G. (2010). Effects of topic interest and prior knowledge on text recall and annotation use in reading a hypermedia text in the L2. ReCALL 22, 228–246. doi: 10.1017/S0958344010000091
Gilliam, S., Magliano, J. P., Millis, K., Levinstein, I., and Boothum, C. (2007). Assessing the format of the presentation of text in developing a reading strategy assessment Tool (R-SAT). Behav. Res. Methods 39, 199–204. doi: 10.3758/BF03193148
Hagaman, J. L., Casey, K. L., and Reid, R. (2016). Paraphrasing strategy instruction for struggling readers. Prev. Sch. Fail. 60, 43–52. doi: 10.1080/1045988X.2014.966802
Hu, L., and Bentler, P. M. (1999). Cutoff criteria for fit indexes in covariance structure analysis: conventional criteria versus new alternatives. Struct. Equ. Model. Multidiscip. J. 6, 1–55. doi: 10.1080/10705519909540118
Kendeou, P., McMaster, K. L., and Christ, T. J. (2016). Reading comprehension: core components and processes. Policy Insights Behav. Brain Sci. 3, 62–69. doi: 10.1177/2372732215624707
Kendeou, P., and van den Broek, P. (2007). Interactions between prior knowledge and text structure during comprehension of scientific texts. Mem. Cogn. 35, 1567–1577. doi: 10.3758/BF03193491
Kowalski, P., and Taylor, A. K. (2017). Reducing students’ misconceptions with refutational teaching: for long-term retention, comprehension matters. Scholarship Teach. Learn. Psychol. 3:90. doi: 10.1037/stl0000082
Kurby, C. A., Magliano, J. P., Dandotkar, S., Woehrle, J., Gilliam, S., and McNamara, D. S. (2012). Changing how students process and comprehend texts with computer-based self-explanation training. J. Educ. Comput. Res. 47, 429–459. doi: 10.2190/EC.47.4.e
Lei, P. W., and Wu, Q. (2007). An NCME instructional model on introduction to structural equation modeling: issues and practical considerations. Educ. Meas. Issues Pract. 26, 33–44. doi: 10.1111/j.1745-3992.2007.00099.x
Leopold, C., and Leutner, D. (2015). Improving students’ science text comprehension through metacognitive self-regulation when applying learning strategies. Metacogn. Learn. 10, 313–346. doi: 10.1007/s11409-014-9130-2
Lupo, S. M., Strong, J. Z., and Conradi Smith, K. (2019). Struggle is not a bad word: misconceptions and recommendations about readers struggling with difficult texts. J Adolesc. Adult Literacy 62, 551–560. doi: 10.1002/jaal.926
Magliano, J. P., Millis, K. K., The R-SAT Development Team, Levinstein, I., and Boonthum, C. (2011). Assessing comprehension during reading with the Reading Strategy Assessment Tool (RSAT). Metacogn. Learn. 6, 131–354. doi: 10.1007/s11409-010-9064-2
McCrudden, M. T., and Kendeou, P. (2014). Exploring the link between cognitive processes and learning from refutational text. J. Res. Read. 37, S116–S140. doi: 10.1111/j.1467-9817.2011.01527.x
McNamara, D. S., O’Reilly, T., Rowe, M., Boonthum, C., and Levinstein, I. (2007). “iSTART: a web-based tutor that teaches self-explanation and metacognitive reading strategies,” in Reading Comprehension Strategies: Theories, Interventions, and Technologies, ed. D. S. McNamara (Mahwah, NJ: Lawrence Erlbaum Associates Publishers), 397–420. doi: 10.4324/9780203810033
Millis, K. K., and Graesser, A. C. (1994). The time-course of constructing knowledge-based inferences for scientific texts. J. Mem. Lang. 33, 583–599. doi: 10.1006/jmla.1994.1028
Mokhtari, K., and Reichard, C. A. (2002). Assessing students’ metacognitive awareness of reading strategies. J. Educ. Psychol. 94, 249–259. doi: 10.1037/0022-0663.94.2.249
Muthén, L. K., and Muthén, B. O. (2021). Mplus (version 8.6) [statistical software]. Los Angeles, CA: Muthén and Muthén.
O’Reilly, T., and McNamara, D. S. (2007). The impact of science knowledge, reading skill, and reading strategy knowledge on more traditional “high stakes” measures of high school students’ science achievement. Am. Educ. Res. J. 44, 161–196. doi: 10.3102/0002831206298171
O’Reilly, T., and Sabatini, J. (2016). “Using advances in cognitive science to improve students study skills and reading comprehension,” in Interventions in Learning Disabilities. Literacy Studies (Perspectives from Cognitive Neurosciences, Linguistics, Psychology and Education, Vol 13, eds R. Schiff and R. Joshi (Cham: Springer). doi: 10.1007/978-3-319-31235-4_9
O’Reilly, T., Wang, Z., and Sabatini, J. (2019). How much knowledge is too little? When a lack of knowledge becomes a barrier to comprehension. Psychol. Sci. 30, 1344–1351. doi: 10.1177/0956797619862276
Ozuru, Y., Dempsey, K., and McNamara, D. S. (2009). Prior knowledge, reading skill, and text cohesion in the comprehension of science texts. Learn Instruction 19, 228–242. doi: 10.1016/j.learninstruc.2008.04.003
Prevatt, F., Petscher, Y., Proctor, B., Hurst, A., and Adams, K. (2006). The revised learning and study strategies inventory (LASSI): an evaluation of competing models. Educ. Psychol. Meas. 66, 448–458. doi: 10.1177/0013164405282454
Pronk, T., Molenaar, D., Wiers, R. W., and Murre, J. (2022). Methods to split cognitive task data for estimating split-half reliability: a comprehensive review and systematic assessment. Psychon. Bull. Rev. 29, 44–54. doi: 10.3758/s13423-021-01948-3
Ranellucci, J., Muis, K. R., Duffy, M., Wang, X., Sampasivam, L., and Franco, G. M. (2013). To master or perform? exploring relations between achievement goals and conceptual change learning. Br. J. Educ. Psychol. 83, 431–451. doi: 10.1111/j.2044-8279.2012.02072.x
Schellings, G., and Van Hout-Wolters, B. (2011). Measuring strategy use with self-report instruments: theoretical and empirical considerations. Metacogn. Learn. 83, 83–90. doi: 10.1007/s11409-011-9081-9
Sinatra, G. M., and Broughton, S. H. (2011). Bridging reading comprehension and conceptual change in science education: the promise of refutation text. Read. Res. Q. 46, 374–393. doi: 10.1002/RRQ.005
Smith, R., Snow, P., Serry, T., and Hammond, L. (2021). The role of background knowledge in reading comprehension: a critical review. Read. Psychol. 42, 214–240. doi: 10.1080/02702711.2021.1888348
Steinke, A., Kopp, B., and Lange, F. (2021). The Wisconsin card sorting test: split-half reliability estimates for a self-administered computerized variant. Brain Sci. 11, 529. doi: 10.3390/brainsci11050529
Stevens, E. A., Vaughn, S., House, L., and Stillman-Spisak, S. (2020). The effects of a paraphrasing and text structure intervention on the main idea generation and reading comprehension of students with reading disabilities in grades 4 and 5. Sci. Stud. Read. 24, 365–379. doi: 10.1080/10888438.2019.1684925
Tarch, C. (2015). Fostering reading comprehension of expository texts through the activation of readers’ prior knowledge and inference-making skills. Int. J. Educ. Res. 72, 80–88. doi: 10.1016/j.ijer.2015.04.013
van den Broek, P. (1994). “Comprehension and memory of narrative texts: Inferences and coherence,” in Handbook of psycholinguistics, ed. M. A. Gernsbacher (Academic Press), 539–588.
Vosniadou S. (ed.). (2008). International handbook of research on conceptual change, Vol. 259. New York, NY: Routledge.
Weinstein, C. E., Acee, T. W., and Jung, J. (2011). Self-regulation and learning strategies. New Dir. Teach. Learn. 126, 45–53. doi: 10.1002/tl.443
Keywords: comprehension strategies, path analysis, reading comprehension, scientific text modeling, text comprehension
Citation: Hunsu NJ, Adesope OO and Yli-Piipari SR (2024) A path model analysis of preform students’ factors and comprehension strategies on cognitive learning outcomes associated with text comprehension. Front. Educ. 9:1239261. doi: 10.3389/feduc.2024.1239261
Received: 13 June 2023; Accepted: 28 February 2024;
Published: 30 April 2024.
Edited by:
Joseph Magliano, Georgia State University, United StatesReviewed by:
Virginia Clinton-Lisell, University of North Dakota, United StatesGal Kaldes, Georgia State University, United States
Marloes Van Moort, Utrecht University, Netherlands
Copyright © 2024 Hunsu, Adesope and Yli-Piipari. This is an open-access article distributed under the terms of the Creative Commons Attribution License (CC BY). The use, distribution or reproduction in other forums is permitted, provided the original author(s) and the copyright owner(s) are credited and that the original publication in this journal is cited, in accordance with accepted academic practice. No use, distribution or reproduction is permitted which does not comply with these terms.
*Correspondence: Nathaniel J. Hunsu, bmF0aGFuaWVsLmh1bnN1QHVnYS5lZHU=