- 1Faculty of Mathematics and Computer Science, Institute of Mathematics Education and Computer Science Education, University of Münster, Münster, Germany
- 2Faculty of Psychology/Sport and Exercise Sciences, Institute for Psychology, University of Münster, Münster, Germany
- 3Faculty of Chemistry and Pharmacy, Institute of Chemistry Education, University of Münster, Münster, Germany
- 4Faculty of Educational and Social Sciences, Department of Communication, University of Münster, Münster, Germany
- 5Faculty of Physics, Institute of Physics Education, University of Münster, Münster, Germany
- 6Faculty of Physics, Institute of Applied Physics, University of Münster, Münster, Germany
- 7Physikalisch-Technische Bundesanstalt — The National Institute of Metrology, Braunschweig, Germany
- 8Faculty of Psychology/Sport and Exercise Sciences, Institute for Psychology in Education and Instruction, University of Münster, Münster, Germany
Introduction: Nowadays, more and more digital resources are used in modern mathematical modeling classes. In order to access these resources, students need a suitable digital device—often mobile devices are used for this purpose. There are several concepts to enable students access to such devices. For example, students can be allowed to use their self-owned devices [Bring Your Own Device (BYOD) concept] or teachers can hand out school-owned devices to their students [device pool (pool) concept]. Currently, little is known about possible effects of different mobile device access concepts on student learning. Hence, in this study, we investigated their effects on students’ mathematical modeling competence. In doing so, we also considered an interaction between the access concept and the effects of (a) students’ problematic smartphone use and (b) students’ fear of missing out on learning mathematical modeling.
Method: To this end, we conducted an experiment, measured students’ mathematical modeling competence as the outcome variable, and analyzed data of 263 German students in grades 8 and 9 using a multilevel model. In the experiment, students were randomly assigned to one of two study conditions and completed a mathematics modeling workshop. In the BYOD condition, students utilized their self-owned smartphones to work on the workshop tasks, whereas in the pool condition, students utilized institutionally provided smartphones.
Results: As a main finding, our results showed an interaction effect between the mobile device access concept and students’ problematic smartphone use on their competence (β = −0.24, 95% CI [−0.47, −0.01]). Students utilizing their self-owned smartphones were negatively affected by their problematic smartphone use (B = −1.45, 95% CI [−2.45, −0.46]), whereas students utilizing provided smartphones were not affected (B = 0.04, 95% CI [−1.01, 1.09]). Students with maximal problematic smartphone use achieved higher competences when utilizing provided devices (BBYOD−Pool = −1.20, 95% CI [–2.35, –0.05]).
Discussion: Our study demonstrates the importance of thinking about effects of student-owned and provided digital devices on mathematics learning. Finally, we discuss (a) that our results do not reveal a general preferability for one of the two access concepts, as well as (b) the relevance of student characteristics when choosing an access concept.
1 Introduction
Over the past years, a variety of studies investigated the effects of digital media and digital tools in the context of learning mathematics and other subjects. Of central interest here—apart from the effects on students’ affect—is especially the effect on learning outcomes. In their meta-analysis, Hillmayr et al. (2020) found an overall positive and moderately sized effect of digital tool usage compared to non-usage (Hedges’s g = 0.65, 95% CI [0.54, 0.75]). Also, when considering only data regarding the subject mathematics, the effect was positive and moderate in size (Hedges’s g = 0.55, 95% CI [0.37, 0.72]).
Hence, it seems reasonable to create the conditions for school learning with digital resources for as many students as possible. A crucial point here is the availability of digital devices that enable students to access these resources. To allow uncomplicated and also spontaneous usage, mobile devices are particularly suitable. However, German schools are currently still poorly positioned in terms of the number of any type of digital devices. The ICILS Study 2018 (Eickelmann et al., 2019) has shown that, counting all types of devices, the student-to-device ratio in Germany was 9.7:1. An alternative to providing school-owned devices could be the utilization of student-owned devices (BYOD). This would allow more students to access digital resources during modeling classes.
As the JIM Study 2022 (Medienpädagogischer Forschungsverbund Südwest, 2022) has shown, almost every German 12- to 19-year-old personally owned a smartphone (95%). Besides, half of them also owned a tablet—which has increased over the past years (2022: 51%, 2021: 43%, 2020: 38%, 2019: 25%). German 12- to 19-year-olds did not only have access to those devices but also used them on a regular basis. Overall, 96 percent stated that they used a smartphone daily or multiple times a week in their free time. Analogously, 49 percent reported tablet use.
As we can see, smartphones and tablets are widespread and often used by students in their free time. However, less is known about whether these personal devices should be used for in-class and out-of-class schoolwork or if it is more beneficial for learning to provide students with digital devices. One possible solution for the latter is the usage of school-owned portable class sets. But, as the ICILS Study 2018 (Eickelmann et al., 2019) has shown, only 49.0 percent of German students visited schools, that held such devices.
In our study, to help with decision making, we comparatively examine these contrasting mobile device access concepts in terms of their relation to learning mathematical modeling, and thereby enabling teachers to, in good conscience, design mathematical modeling classes where students access digital resources on their own.
1.1 Mathematical modeling with the use of digital resources
All over the world, mathematical modeling is finding its way into mathematics teaching, as well as into curricula and assessments (National Council of Teachers of Mathematics, 2000; Kaiser, 2017). At the same time, digital resources are being used in the classroom and the combination of both has led to a wide variety of mathematical modeling tasks (Drijvers et al., 2016), which emphasizes clear definitions of constructs and their operational counterparts for the context of our work. We conceptualize modeling competence as abilities to identify a problem in a given real-world situation, to translate it into mathematics, and to interpret and validate the solution of the corresponding mathematical problem in relation to the given situation (Niss et al., 2007). Modeling competence also includes willingness to use these abilities independently and their reflective use in life (Blomhøj and Jensen, 2007).
The use of digital tools in modeling can be described with the help of a detailed modeling cycle that takes into account a digital world in addition to the real world and the mathematical world (Greefrath et al., 2018). First, the modeling problem must be understood, simplified, and translated into the language of mathematics. Then, the mathematical model can be defined. To use the digital tool to work on the mathematical model, the mathematical expressions must be translated into the language of the digital tool, e.g., as commands for a computer algebra system, and finally the results must be transformed back into the language of mathematics where they can be documented. Finally, when the mathematical results are related to the real situation, the original problem can be solved (Galbraith et al., 2003; cf. Pierce, 2005). In addition to this detailed view of the use of the digital tool in mathematical working, observations of modeling processes show that digital resources can also be used at many other points in the modeling process (Greefrath and Siller, 2017). Digital resources can help students develop mathematical modeling competence in several ways. For example, they can be used to understand the context, construct a real model, experiment, or check the mathematical results through the possibilities of visualization (Borba and Villarreal, 2005; Greefrath et al., 2018; Molina-Toro et al., 2019; Villa-Ochoa and Suárez-Téllez, 2021). This leads to a different perspective on digital resource use in modeling, in which the use of tools in mathematical work is only a small part of media use.
With regard to the possibilities of competence acquisition for modeling with digital tools, it is known that students were able to work more comfortably with real data through the use of digital tools, in addition to more frequent use of graphs and greater flexibility in solution strategies (Burrill et al., 2002). Furthermore, problem-solving and conceptual skills, which are important for modeling, may have been enhanced by the use of digital tools (Ellington, 2003). However, not all studies showed a stronger increase in performance in modeling in an experimental group with digital tools compared to a control group without these tools. Villa-Ochoa and Suárez-Téllez (2021) described that technology provides an organization of modeling processes and that it may or may not contribute to the production of meanings and competences. For example, in a study of students in a digital learning environment, it was found that difficulties with the technology and the modeling decisions they had to make in the module activities were in some cases barriers that affected students’ ability to learn (Merck et al., 2021).
The competence development in mathematical modeling with digital tools may also depend on the influence of affective characteristics of the students in relation to the confidence in their own ability to operate the digital tool. It was shown that a central sub-competence of mathematical modeling is promoted more strongly when students work with digital tools and have a correspondingly positive attitude (Greefrath et al., 2018).
1.2 Effects of mobile device access concepts
Depending on the specific use case in which the digital device is to be used, different device types seem suitable. Beyond calculators—even those equipped with a computer algebra system—, universal computers offer a wide range of possible application scenarios, regardless of whether they are desktop computers, laptop computers, mobile devices or others. Due to the students’ previous life experiences, the use of smartphones or tablets appears to be more low-threshold with regard to the required digital competences and thus more favorable. Additionally, most modern smartphones and tablets bring along further advantages compared to desktop and laptop computers as they are portable, lightweight, and include different sensors (e.g., photosensor, GPS sensor, and accelerometer). Also, these devices are relatively affordable and the characteristic features of desktop and laptop computers (e.g., professional software and enhanced connectivity) are not required for school use most of the time.
When deciding on mobile devices, the question arises as to how these devices should be accessed by students. Should school-owned devices be handed out and re-collected by teachers before and after each utilization? Or should students bring their own devices to class? These options clearly represent two very contrasting mobile device access concepts. Applying the former concept, the school purchases several sets of devices and rents them out through the teaching staff—we will refer to this as the device pool concept (pool concept). The latter concept systematically relies on the utilization of student-owned devices for learning, which is generally called the Bring Your Own Device concept (BYOD concept). Of course, there is a continuum of mobile device access concepts between BYOD and pool, with both conceptualized as its end-poles. A variant of the BYOD concept, for example, is the Get Your Own Device concept (GYOD concept). In this case, to counter the heterogeneity of devices, students are told which device(s) they are allowed to use and should purchase. A concept closer to pool, is the Corporate Owned, Personally Enabled concept (COPE concept). The main difference is that the mobile devices are handed out on a long-term basis to the students (see also United Nations Educational, Scientific and Cultural Organization, 2013; Murauer, 2017). These different concepts are often accompanied by additional rights and responsibilities (e.g., device maintenance). Table 1 provides an overview of the characteristics of selected mobile device access concepts. However, there are further concepts that are not listed.
Originating from the variations in the concepts’ characteristics, further disparities between them emerge, like (a) different possibilities for individualization of minor and major settings or cosmetics (e.g., standard apps, in-app settings, password manager, dark mode, or background image), (b) in-/sufficient rights to install any app (e.g., educational apps as well as social media apps, gaming apps, music streaming apps, video streaming apps, or news apps), (c) different familiarity with the device, (d) different levels of control over the saved data (i.e., different levels of data privacy), or (e) different student responsibility for the device. As we will discuss in the following, the differences between the concepts might affect students’ competences when mobile devices are used for learning. As the concepts BYOD and pool seem to exhibit the greatest differences, we decided to constrain our comparison on these two concepts.
Generally, in their everyday life, students use digital devices, especially smartphones, for various activities, like being on social media, watching videos, playing games, messaging, or listening to music (Radesky et al., 2023). Inherently, a utilization of digital devices for learning activities brings along a certain potential for distraction. In Karsenti and Fievez’s (2013) study, almost every student and teacher reported distraction as a challenge for digital devices in classes. In the accompanying interviews, concretely the access to social media and games was mentioned. As such and comparable smartphones activities (e.g., social media use, gaming, or messaging) heavily rely on the usage of specific apps, which in turn are usually not installed on school-owned devices, it seems to be important for learning whether student-owned or provided devices are utilized. In contrast, Burden et al. (2012) found indications that personal ownership of the digital device is a highly important factor in terms of positively affecting students’ autonomy, engagement, interest, motivation, self-efficacy, and responsibility for their own learning. Further, in the contexts of employees utilizing self-owned devices, Doargajudhur and Dell (2020) showed that such a utilization positively affected technology self-efficacy (β = 0.62), perceived job autonomy (β = 0.54), and perceived workload (β = 0.21), which in turn positively influence perceived job performance (β = 0.22, β = 0.24, and β = 0.37, respectively).
1.3 Effects of problematic smartphone use
Besides different mobile device access concepts, also other technology related constructs seem to influence student learning. In current research, an increasing number of studies investigated the relationship between problematic smartphone use and academic performance (see below). Problematic smartphone use, which is also referred to as smartphone addiction or smartphone use disorder, is a rather new construct in psychopathology (Elhai et al., 2017). To date, the construct is neither included in the Diagnostic and Statistical Manual of Mental Disorders (DSM-5-TR; American Psychiatric Association, 2022) nor uniformly conceptualized (Sunday et al., 2021). De-Sola Gutiérrez et al. (2016) summarized, that mainly two conceptualizations are prevalent: the first views the concept of addiction as not limited to substances, and the second is based on an interplay of lack of impulse control and addiction. Sunday et al. (2021) collected overuse, excessive use, compulsive use, heavy use, problematic use, addiction, and dependence as operationalization attempts in previous works. According to Elhai et al. (2017), problematic smartphone use is conceptualized similarly to internet addiction and internet gaming addiction, with the main difference being the hardware involved. In previous works, the criteria for problematic smartphone use were often based on criteria for substance use disorders, gambling disorder, or internet addiction (see De-Sola Gutiérrez et al., 2016). Since the fifth edition (DSM-5; American Psychiatric Association, 2013), the DSM tentatively includes the first internet-related disorder, namely internet gaming disorder. The proposed criteria are preoccupation, withdrawal, tolerance, persistence, displacement, problems, deception, escape, and conflict. To define problematic smartphone use, we adopted the DSM-5 / DSM-5-TR criteria for internet gaming disorder (see, e.g., Hussain et al., 2017; Mitchell and Hussain, 2018; Richardson et al., 2018; Reer et al., 2022, for a similar approach). As most of these criteria can also be found as DSM criteria for substance use disorders or gambling disorder, this approach seemed generally reasonable and in line with previous research.
Comprising the findings of 44 individual studies, Sunday et al.’s (2021) meta-analysis found a small negative effect of problematic smartphone use on academic performance (r = −0.12, 95% CI [–0.17, –0.08]). Conducting a moderation analysis, they further found in-class multitasking to negatively influence this effect (r = −0.16, 95% CI [–0.22, –0.11]). Two recent studies (Zhou et al., 2022a,b) found similar effects to the meta-analysis, but specifically for the subject mathematics. Students’ problematic smartphone use had a small negative effect on their mathematics achievement test score (β = −0.18, p < 0.001 and β = −0.16, p < 0.001, respectively).
1.4 Effects of fear of missing out
Another arising research topic focuses the effects of fear of missing out on learning. Fear of Missing Out (FoMO) is “defined as a pervasive apprehension that others might be having rewarding experiences from which one is absent[.] FoMO is characterized by the desire to stay continually connected with what others are doing” (Przybylski et al., 2013, p. 1841).
Through messaging apps and social media combined with mobile devices, it is easier than ever to stay in constant passive and active connection with others and thus to fulfill this desire. Shane-Simpson and Bakken (2022) showed that the in-class use of some social media platforms (Instagram and Twitter) was predicted by university students’ fear of missing out, Exp(B) = 1.53, 95% CI [1.05, 2.24] and Exp(B) = 1.70, 95% CI [1.07, 2.71], respectively. In addition, van der Schuur et al. (2015) performed a literature review regarding the consequences of media multitasking by adolescents and young adults. They found 3 correlational and 11 experimental studies investigating the relationship between in-class media use and course/lecture outcomes—which van der Schuur et al. (2015) intentionally distinguish from effects on overall grades and test scores. All 14 studies found a negative relation between in-class media use and course grades (−0.16 < r < −0.28), respectively, in-class media use and test scores (−0.21 < r < −0.48). A recent study (Zhao, 2023) also investigated relations between fear of missing out, social media multitasking, and academic performance. It was found that students’ fear of missing out positively influenced their social media multitasking (β = 0.35, p < 0.001), which in turn negatively influenced their academic performance (β = −0.18, p = 0.005).
Related to the effects of fear of missing out is the question of the consequences if someone is unable to fulfill their “desire to stay continually connected” (Przybylski et al., 2013, p. 1841). Mobile unavailability could be a possible cause of such an inability. Kneidinger-Müller (2019) presented participants different vignettes, all describing situations of mobile unavailability, and especially investigated the effects of participants’ fear of missing out and the reason for the unavailability on their rating of the situation. However, an interaction of both was not considered. Nevertheless, she found that, for vignettes describing a situation where one is mobile unavailable due to their own decision, participants’ fear of missing out still negatively influenced their rating of the situation (B = −0.23, 95% CI [−0.44,−0.02]). Regarding participants with average fear of missing out, she found that scenarios were rated significantly worse, when there was an external reason (i.e., empty battery, forgotten smartphone, or no mobile phone connection) for the mobile unavailability compared to a mobile unavailability due to one’s own decision (B = −0.61, 95% CI [−0.84,−0.38]; B = −0.76, 95% CI [−0.98,−0.53]; and B = −0.51, 95% CI [−0.73,−0.28]; respectively).
1.5 The present research
In summary, students’ subsequent mathematical modeling competence after a digitally enriched workshop may be influenced by the mobile device access concept applied (Burden et al., 2012; Karsenti and Fievez, 2013; Doargajudhur and Dell, 2020), students’ problematic smartphone use (Sunday et al., 2021; Zhou et al., 2022a,b) and their fear of missing out (van der Schuur et al., 2015; Kneidinger-Müller, 2019; Shane-Simpson and Bakken, 2022; Zhao, 2023). Thus, as a first approach, we focused on students with average problematic smartphone use and average fear of missing out and thereby considered only the effect of the access concept on those students’ competence. As the concepts BYOD and pool seemed to exhibit the greatest differences, we decided to investigate their effects on students’ competence. So, our first research question was:
Research Question 1: To what extent does the subsequent mathematical modeling competence after a digitally enriched workshop differ between students with average problematic smartphone use and average fear of missing out utilizing their self-owned smartphones and those utilizing provided smartphones instead?
On the one hand, there were indications that the personal ownership of the BYOD devices positively affects students’ autonomy, engagement, interest, motivation, self-efficacy, and responsibility for their own learning (Burden et al., 2012). Further, positive effects on technology self-efficacy, perceived job autonomy, and perceived workload were shown, which in turn positively affected perceived job performance (Doargajudhur and Dell, 2020). Both findings indicated an advantageous of the BYOD concept compared to the pool concept. However, on the other side, the BYOD devices seemed to be accompanied by a higher potential for distraction (Karsenti and Fievez, 2013). We thus formulated the following non-directional hypothesis:
Hypothesis 1: The mathematical modeling competence of students with average problematic smartphone use and average fear of missing out is affected by the mobile device access concept (BYOD vs. pool).
1.5.1 Mobile device access concepts and problematic smartphone use
Besides the possible relevance of the mobile device access concept for students’ academic performance (see above), it was found that students’ problematic smartphone use negatively affects their academic performance (Sunday et al., 2021; Zhou et al., 2022a,b). Further, in-class multitasking was found to moderate this effect (Sunday et al., 2021). As already mentioned above, common smartphone activities as social media use, gaming, or messaging (Radesky et al., 2023) heavily rely on the usage of specific apps. Without such apps being installed, a barrier to multitask during class arises for students learning with provided pool devices. Hence, the following question emerged:
Research Question 2: How is students’ subsequent mathematical modeling competence after a digitally enriched workshop influenced by their problematic smartphone use and the access concept applied (BYOD vs. pool)?
Due to the mentioned barrier in the pool concept and the absence of a barrier in the BYOD concept combined with the findings on in-class multitasking moderating the effect of problematic smartphone use (Sunday et al., 2021), we expected an interaction between these two predictors.
Hypothesis 2.1: The effects of students’ problematic smartphone use and the access concept applied (BYOD vs. pool) interact with each other.
Viewing at each access concept at a time, we expected to reproduce the negative effect of problematic smartphone use (Sunday et al., 2021; Zhou et al., 2022a,b) in the BYOD concept and, due to the moderating role of in-class multitasking (Sunday et al., 2021), we further expected no effect of students’ problematic smartphone use in the pool concept.
Hypothesis 2.2: In the BYOD concept, students’ mathematical modeling competence is influenced negatively by their problematic smartphone use.
Hypothesis 2.3: In the pool concept, students’ mathematical modeling competence is independent of their problematic smartphone use.
Viewed from the other perspective, that is, comparing students with the same level of problematic smartphone use each, we expected the BYOD concept to be advantageous for students with minimal (i.e., not average) problematic smartphone use because of the positive effects of BYOD itself (Burden et al., 2012; Doargajudhur and Dell, 2020). Due to the negative relation of problematic smartphone use and its reinforcement by in-class multitasking (Sunday et al., 2021), we further expected the pool concept to advantageous for students with higher problematic smartphone use.
Hypothesis 2.4: Comparing students with a minimal problematic smartphone use, the mathematical modeling competence of students in the BYOD group is higher than the one of students in the pool group.
Hypothesis 2.5: Comparing students with a rather high problematic smartphone use, the mathematical modeling competence of students in the BYOD group is lower than the one of students in the pool group.
1.5.2 Mobile device access concepts and fear of missing out
Just as students’ problematic smartphone use, also their fear of missing out was found to be related to their (in-class) use of social media (Shane-Simpson and Bakken, 2022; Zhao, 2023). Additionally, social media multitasking was found to be related to students’ academic performance (van der Schuur et al., 2015; Zhao, 2023). Again, the use of social media heavily relies on the usage of specific apps. Without such apps being installed, again a barrier to multitask during class arises for students learning with provided pool devices. However, the mobile unavailability in the pool concept seemed to be a relevant negative factor, especially regarding students with higher fear of missing out (see Kneidinger-Müller, 2019; and consider Przybylski et al., 2013). Hence, the following question emerged:
Research Question 3: How is students’ mathematical modeling competence influenced by their fear of missing out and the access concept applied (BYOD vs. pool)?
Analogously to the argumentation above, we expected an interaction between students’ fear of missing out and the access concept. This was due to the mentioned barrier in the pool concept and the absence of a barrier in the BYOD concept combined with the role of in-class multitasking and mobile unavailability (van der Schuur et al., 2015; Kneidinger-Müller, 2019; Shane-Simpson and Bakken, 2022; Zhao, 2023).
Hypothesis 3.1: The effects of students’ fear of missing out and the access concept applied (BYOD vs. pool) interact with each other.
Viewing at each access concept at a time, we expected to reproduce the negative effect of fear of missing out in the BYOD concept, which is due to the role of in-class multitasking (van der Schuur et al., 2015; Shane-Simpson and Bakken, 2022; Zhao, 2023). In the pool concept, we also expected a negative effect of fear of missing out, but due to students’ evaluation of their mobile unavailability (Kneidinger-Müller, 2019). However, it remained open whether the effect of fear of missing out would be greater in the BYOD or in the pool concept.
Hypothesis 3.2: In the BYOD concept, students’ mathematical modeling competence is influenced negatively by their fear of missing out.
Hypothesis 3.3: In the pool concept, students’ mathematical modeling competence is influenced negatively by their fear of missing out.
Viewed from the other perspective, that is, comparing students with the same level of fear of missing out each, we expected the BYOD concept to be advantageous for students with minimal (i.e., not average) fear of missing out because of the positive effects of BYOD itself (Burden et al., 2012; Doargajudhur and Dell, 2020). For students with higher fear of missing out, it remained open whether the BYOD concept or the pool concept is advantageous. It could be, that students learning with pool devices show a higher mathematical modeling competence because they were less distracted from social media, but it could also be, that students learning with their own devices (BYOD) show a higher mathematical modeling competence because they actually do not fear to miss experiences (see van der Schuur et al., 2015; Shane-Simpson and Bakken, 2022; Zhao, 2023; but compare Kneidinger-Müller, 2019). We thus phrased a non-directional hypothesis for this case.
Hypothesis 3.4: Comparing students with a minimal fear of missing out, the mathematical modeling competence of students in the BYOD group is higher than the one of students in the pool group.
Hypothesis 3.5: Comparing students with a rather high fear of missing out, the mathematical modeling competence of the students differs between the access concepts (BYOD vs. pool).
1.5.3 Aims and objectives
In summary, the role of the mobile device access concept seemed vague and only sparsely researched. However, the existing literature gave cause to assume a certain relevance for student learning. Thus, we decided to perform the present study. To test the presented hypotheses, we conducted an experimental study with treatment groups BYOD and pool. Both treatment groups attended the identical digitally enriched mathematics modeling workshop. Afterward, we measured students’ mathematical modeling competence. Additionally, we collected data on students’ problematic smartphone use and fear of missing out prior to the workshop.
As the hypotheses form relations as illustrated in Figure 1, we applied a linear regression analysis to investigate these effects. We grand mean centered students’ problematic smartphone use and fear of missing out, whereby Hypothesis 1 corresponded to the simple effect of the access concept on students’ mathematical modeling competence. Hypotheses 2.1 and 3.1 matched the interaction effects in the model. For supported hypotheses 2.1 or 3.1, we further estimated marginal means of both concepts and of the corresponding linear trends to answer Hypotheses 2.2–2.5 and 3.2–3.5, respectively. And, with regard to Hypothesis 2.3, we compared this model to a reduced model in which we excluded the direct effect of students’ problematic smartphone use but kept the interaction effect between problematic smartphone use and the access concept.
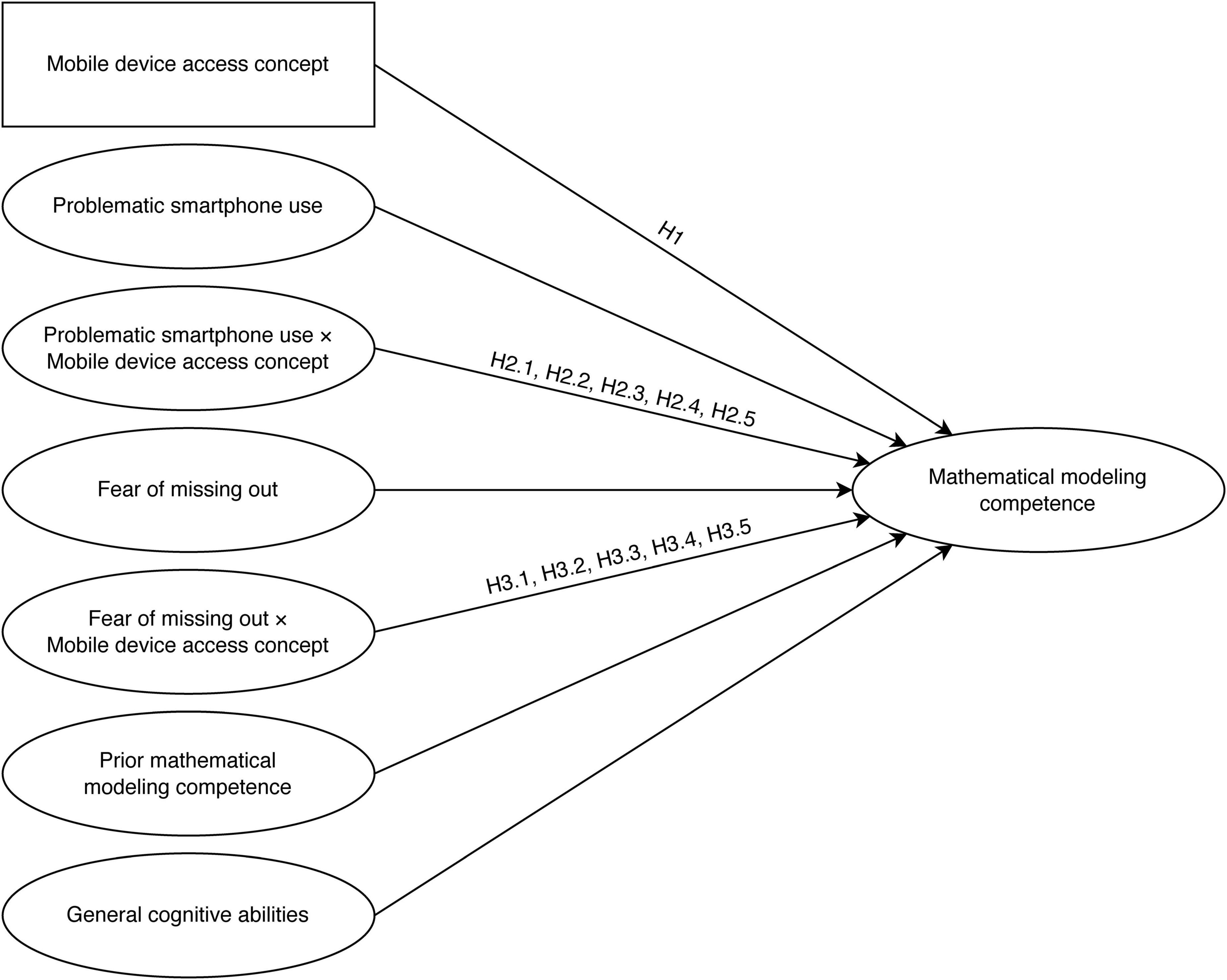
Figure 1. Multilevel regression model under investigation. Note: All variables are located on the student level (level 1). The school class level (level 2) remains empty. H1 = Hypothesis 1; H2.1 = Hypothesis 2.1; H2.2 = Hypothesis 2.2; H2.3 = Hypothesis 2.3; H2.4 = Hypothesis 2.4; H2.5 = Hypothesis 2.5; H3.1 = Hypothesis 3.1; H3.2 = Hypothesis 3.2; H3.3 = Hypothesis 3.3; H3.4 = Hypothesis 3.4; H3.5 = Hypothesis 3.5.
To handle the fact that the participating students were nested in school classes, we decided for a multilevel model (see Hox et al., 2017; Huang, 2018) with the criterion variable and all predictor variables on the student level. As many studies have shown, students’ competence is highly predicted by their prior competence (Dochy et al., 1999) and general cognitive abilities (Peng et al., 2019). Therefore, we also collected respective data and included both effects in the model to control for them.
2 Methods
2.1 Participant characteristics
The sample of the study consisted of 399 students attending grades 8 and 9 of secondary schools allowing students to obtain a higher education entrance qualification. The students were distributed among 17 classes of 9 schools in North Rhine-Westphalia, Germany.
Some study participants had to be excluded from the analysis for different reasons (see below). Thereby, the sample analyzed consisted of 261 students aged 12–17 (M = 14.17, SD = 0.68; 54.79% female, 1.53% non-binary, 0.38% did not respond). The students were distributed among 15 classes of 8 schools.
2.2 Sampling procedures, inclusion and exclusion criteria
We generated the study sample by convenience sampling and self-selection by the students’ teachers. Specifically, we contacted school principals and teachers through official, administrative, or organizational school ministry and community mailing lists, made use of public calls for participation, and directly addressed existing contacts. Participation in this study was open only to students visiting secondary schools allowing to obtain a higher education entrance qualification (viz., the German school types Gymnasium and Gesamtschule). Additionally, we limited participation to 8th and 9th grade students. Both exclusion criteria were set, so that we could implement a single workshop that fitted to the curriculum (see Ministerium für Schule, Jugend und Kinder des Landes Nordrhein-Westfalen, 2004; Ministerium für Schule und Weiterbildung des Landes Nordrhein-Westfalen, 2007). Students who did not own a smartphone were allowed to participate in the workshop (utilizing a provided device), but were excluded from the analysis because it was not possible to randomly assign them to a treatment group.
To collect the data, we visited each of the classes twice. During the first visit, we introduced ourselves as well as the topic of the workshop (i.e., the environmental impact of electric mobility) and collected data on the independent variables that are generally considered stable over time (viz., problematic smartphone use, fear of missing out, and general cognitive ability). This visit lasted one lesson (i.e., 45 to 60 min). The second visit lasted one school day (i.e., 4 hr 30 min to 5 hr), during which we measured students’ prior mathematical modeling competence, carried out the workshop, and finally measured students’ outcome mathematical modeling competence. The data collection phase began in October 2020 and ended in February 2022.
2.3 Measurements and instrumentation
We collected data for one criterion variable (viz., outcome mathematical modeling competence) and five predictor variables (viz., mobile device access concept, prior mathematical modeling competence, general cognitive abilities, fear of missing out, and problematic smartphone use). Table 2 provides an overview of the variables and the corresponding instruments.
To enhance the quality of measurements, multiple steps were taken. Since we are investigating potential effects of digital devices, all measurements were carried out on paper. We also created new or implemented existing manuals for all data collections, the workshop execution, data codings, and data ratings. All involved researchers were trained to follow these manuals.
The variable mobile device access concept recorded which study group (BYOD or pool) the participants were assigned to. The assignment was determined at random. To include this categorical variable in the model, we applied dummy variable coding (0 = Pool and 1 = BYOD). To assess the other constructs, we used the instruments described in the following subsections.
2.3.1 Measuring mathematical modeling competence
Students’ mathematical modeling competence was measured twice, before and after the workshop, respectively. The two scores obtained for each student represented their prior mathematical modeling competence and their outcome mathematical modeling competence, respectively.
Modeling competence is measurable and demonstrably different from purely technical mathematical competences (Blum et al., 2004). Based on different understandings of modeling competence, different formats are used to measure modeling competence in tests. Two basic principles of task design can be distinguished. On the one hand, tasks are used that only ask for partial processes of modeling (atomistic approach) and on the other hand, tasks that require the complete running through of a modeling cycle (holistic approach; Blomhøj and Jensen, 2003). It should be noted that modeling competence consists of more than just the sum of individual sub-competences (Maaß, 2006) and the sub-processes cannot be assessed independently of each other.
To measure the students’ modeling competence, we created a competence-based performance test of atomistic and holistic test items. Thereto, we selected and slightly modified 16 items, which were previously used in the German national comparative test in grade 8 (Institut zur Qualitätsentwicklung im Bildungswesen [IQB], n.d.). All 16 items measured the competence mathematical modeling within the core theme functional relations. Out of the 16 items, we formed four test versions with 8 items each. To measure students’ mathematical modeling competence at the two measurement points (before and after the workshop participation), we assigned two different test versions to each student with no overlapping items. To determine scores for students’ mathematical modeling competence before and after the workshop, we rated student answers on the items for accuracy (0 = incorrect, 1 = correct).
We then estimated a Rasch model using marginal maximum likelihood estimation. The estimation of the model was run with the R package TAM version 4.1-4 (Robitzsch et al., 2022). In a first step, combining students’ responses before and after the workshop, we estimated the item difficulty. We then fixed the obtained item difficulty and estimated students’ abilities prior and subsequent to the workshop in a two-dimensional model. The reliability for the mathematical modeling competence test was sufficient (Rost, 2004), as of an expected a posteriori reliability (EAP reliability) of 0.704 for the prior measurement and 0.702 for the subsequent measurement. All Items showed a satisfactory infit between 0.83 and 1.17.
As the selected items originate from the German national comparative test item pool (IQB, n.d.), they have originally been developed in a multi-stage process under supervision of the IQB to ensure that they validly assess the competences described in the German educational standards. This process comprised especially the development of items, a revision based on feedback of experts in empirical educational research and mathematics didactics, and several item pilots and empirical tests (personal information of the authors; for a comparable process, see Pant et al., 2013; Köller, 2016; Mahler et al., 2019).
2.3.2 Measuring general cognitive abilities
To efficiently measure general cognitive ability, we used two subtests (viz., Series Completion and Matrices) in the short version 1 type of implementation of the culture fair intelligence test CFT 20-R (Weiß, 2019). The CFT 20-R displayed construct and factorial validity (e.g., strong correlations between its subfacets and general cognitive ability), external validity (i.e., moderate to large correlations with other intelligence test batteries), and predictive validity (e.g., correlations with grades were small to large; see Weiß, 2019). Conducting a factor analysis, Weiß has shown that both subtests mainly represented the factor reasoning, which is a critically component of fluid intelligence (e.g., McGrew, 2009). Some researchers even found fluid intelligence to be indistinguishable from general cognitive ability (cf. Kyllonen and Kell, 2017).
Students were worked on 15 multiple choice items per subtest and their responses were scored for accuracy (0 = incorrect, 1 = correct). Then, multiple steps followed. First, we created a sum score for each subtest. Second, we z-standardized both subtests separately. Third, we incorporated the standardized scores in a simple unidimensional confirmatory factor analysis model using the R package lavaan (version 0.6-14; Rosseel, 2012) with both factor loadings fixed to the values obtained by Weiß (i.e., 0.76 for both subtests). We estimated the factor variance and both residual variances freely. Finally, we derived factor scores for each student based on the Bartlett method. We found acceptable marginal reliability (Brown and Croudace, 2015) for the factor scores, ρ = 0.75. In addition, the factor determinacy index was 0.87 which surpassed recommended cut-offs for using factor scores in research (Ferrando and Lorenzo-Seva, 2018).
2.3.3 Measuring fear of missing out
To measure fear of missing out, we used the German version (Reer et al., 2019) of the Fear of Missing Out scale (Przybylski et al., 2013). The scale consisted of 10 items, which were rated from 1 (Not at all true of me) to 5 (Extremely true of me). For example, one item was “I fear others have more rewarding experiences than me.” The scale score was built by calculating the arithmetic mean and grand centering it, without scaling.
The internal consistency for the original Fear of Missing Out scale (Przybylski et al., 2013) has been determined with three samples. For the sample most similar to ours (N = 87 first-year undergraduate students; aged 18–33, M = 20.00, SD = 2.96; 77% women), the reliability was Cronbach’s α = 0.89. Cronbach’s α for the other two samples has been almost identical. The German version of the Fear of Missing Out scale (Reer et al., 2019) has also shown a good reliability of Cronbach’s α = 0.87 for a representative sample of 1,865 German internet users (aged 14–39, M = 27.65,SD = 6.85; 51.5% females). In our study the internal consistency of the German version was Cronbach’s α = 0.82.
2.3.4 Measuring problematic smartphone use
To measure problematic smartphone use based on the DSM-5 / DSM-5-TR criteria for internet gaming disorder, we used an adapted version of the Social Media Disorder Scale (van den Eijnden et al., 2016). Just as we adopted the DSM-5 / DSM-5-TR criteria for internet gaming disorder for problematic smartphone use, van den Eijnden et al. (2016) did so for social media disorder. The Social Media Disorder Scale captures the nine DSM criteria (preoccupation, withdrawal, tolerance, persistence, displacement, problems, deception, escape, and conflict) with one item each on a dichotomous yes-no scale (0 = No, 1 = Yes). Good internal consistency has been shown for three samples of Dutch adolescents with Cronbach’s α of 0.81, 0.76, and 0.82, respectively, as well as satisfactory convergent validity and criterion validity. Additionally, in comparison with a longer 27-item scale, it has been shown that a short 9-item scale based on the DSM criteria provides a valid instrument for internet gaming disorder (Lemmens et al., 2015) and social media disorder (van den Eijnden et al., 2016). Moreover, for the similar Internet Gaming Disorder Scale (Lemmens et al., 2015), criterion-related validity was shown to be higher for a dichotomous scale than for a 6-point polytomous scale. Overall, we considered the adaptation of the Social Media Disorder Scale to be a promising approach.
To derive our problematic smartphone use instrument from the Social Media Disorder Scale (van den Eijnden et al., 2016), we first replaced the term social media by your smartphone or just smartphone, respectively. For example, one item was “During the past year, have you had serious conflict with your parent(s) and sibling(s) because of your smartphone use?” We then translated the instrument to German. The instrument thereby obtained showed an internal consistency of Cronbach’s α = 0.69. For the further analysis, we built a scale score by calculating the arithmetic mean and grand centering it, without scaling.
2.4 Data collection
The data was collected between October 2020 and February 2022. We collected all data in written paper-based form. The data regarding fear of missing out, problematic smartphone use, and general cognitive abilities was collected at a preliminary day to the workshop. On the day of the workshop, we measured students’ mathematical modeling competence prior to and after the workshop.
2.5 Research design
We conducted an experimental manipulation with students randomly assigned to the experimental groups BYOD (students utilize self-owned smartphones) and pool (students utilize provided smartphones). Except for that difference, both experimental groups were treated the same, that is, in particular the mathematics workshop was the same for both groups. We assigned the students ourselves using a computer-generated random sequence and the list of participants. The unit of delivery was half a school class, concretely 6 to 15 students. The unit of randomization was an individual student and the only restriction on randomization procedures was the requirement that the number of students assigned to the two experimental groups should not differ by more than one for each class. The study followed a between-subjects design.
2.6 Intervention
To investigate the effects of the two mobile device access concepts BYOD and pool on students’ competence, we randomly assigned the students to either condition and then conducted a mathematics workshop with them. The participating classes were split in half and taught in two separate school rooms by trained project members. Thus, 6 to 15 students were taught in each room. During the workshop, the students worked in pairs except for one relatively short task and each student had one smartphone at their disposal. All students received the identical workshop except for the fact, that BYOD students used their own smartphones to accomplish the workshop, whereas pool students used project-owned smartphones. To control for effects of the pool students’ private smartphones, that is, those that were not utilized intentionally during the workshop, we simulated a strict school wide smartphone prohibition for these students. Students in the pool condition had to hand over their private smartphones to the project members for the workshop day. To not handicap the pool students because of an unfamiliar smartphone operating system, they were handed a smartphone with an operating system that closely matched their private one—as far as feasible. That is, pool students owning an iPhone were also provided with an iPhone; all other students were provided with an Android smartphone. For the purposes of this study, we found this to be important because otherwise it may have been the first contact with an alternative operating system for some students, which in turn may limit the interpretability of the study results. Students not owning a smartphone freely chose an operating system. Their study participation was only agreed for convenience (see below).
On the workshop day, we visited each participating class for a total of around 5 hr 15 min to 5 hr 45 min—depending on their school’s general timetable. This included also time for the data collections and school typical breaks. The subject-related working time was approximately 3 hr to 3 hr 30 min. During this working time, students completed eight mandatory and two optional tasks in the area of mathematical models describing the relationship between the mileage of an electric car and the emissions of climate-changing greenhouse gases it causes. The different tasks involved a variety of diverse digital actions, ranging from general to subject specific ones. For example, students were utilizing the smartphones to perform a web search, to work on an interactive video, to digitally collaborate with their peers, to use a CO2-equivalent-calculator, to perform calculations, to visualize data with an interactive applet, to simulate real-world situations, to plot functions, and to work with a multi-representation system.
2.7 Data diagnostics
After data collection, we excluded all students from the analysis, who met at least one of the following criteria:
• Students who stated not to own a smartphone, as these students could not be randomized to the study groups.
• Students who did not reply whether they owned a smartphone, as these students could possibly not be randomized to the study groups. For reasons of comparability, we have also removed these students from the BYOD group.
• Students who were (partly) absent at the preliminary data collection, as the data on their fear of missing out and problematic smartphone use and/or on their general cognitive abilities was completely missing.
• Classes whose workshop realization deviated from the manual, as their received intervention is not comparable to that of the other classes.
• Students who did not fully participate in the workshop (i.e., students who showed up late, were absent in the interim, or had to leave early), as their received intervention is not comparable to that of the other students.
2.8 Analytic strategy
As we wanted to treat students’ problematic smartphone use and their fear of missing out as continuous spectrums (see also Maser and Akiskal, 2002; cf. American Psychiatric Association, 2022) in this analysis, we applied a linear regression analysis. To include the study condition (i.e., the mobile device access concept) as a categorical predictor, we applied dummy variable coding (0 = Pool and 1 = BYOD). As the independence of the observations is a major assumption of linear regression analysis (Hox et al., 2017) and the data used for this analysis consisted of students that were clustered in classes, we estimated a multilevel model (level 1 = students, level 2 = classes) to account for the nested data structure (Hox et al., 2017; Huang, 2018). Failure to consider this structure can lead to biased statistical inference. Additionally, the data showed an intraclass correlation coefficient of 0.167 for students’ outcome mathematical modeling competence, which also exceeded discussed thresholds (e.g., 0.02; Bühner and Ziegler, 2017; see Huang, 2018 for a discussion) and thereby underlined this decision. As we did not expect any random slopes, we stated a random intercept model consisting of variables on the student level only. Hypothesis 1 matched the simple effect of the access concept on students’ mathematical modeling competence. Hypotheses 2.1 and 3.1 matched the interaction effects in the model. For supported hypotheses 2.1 or 3.1, we further estimated marginal means of both concepts and of the corresponding linear trends to answer Hypotheses 2.2–2.5 and 3.2–3.5, respectively. We tested all hypotheses against an alpha level of 5 percent. To answer Hypothesis 2.3, we further estimated a reduced model in which we excluded the simple effect of problematic smartphone use but kept the interaction effect of problematic smartphone use and the access concept. We than compared the Akaike information criterion (AIC) and the Bayesian information criterion (BIC) of the full model and the reduced model. A lower AIC and BIC in the reduced model would indicate an independence of students’ mathematical modeling competence from their problematic smartphone use for the pool group.
3 Results
3.1 Participant flow
Participant flow through the study is illustrated in Figure 2.
3.2 Descriptive statistics
The observed range, mean and standard deviation of the study variables are given in Table 3. Their correlations are given in Table 4. Prior mathematical modeling competence and outcome mathematical modeling competence showed a large positive correlation. Additionally, general cognitive abilities correlated moderately and positive with both of them. Furthermore, there was a small negative correlation between problematic smartphone use and outcome mathematical modeling competence and a moderate positive one between problematic smartphone use and fear of missing out. All correlations were as expected.
3.3 Data analysis
The maximum likelihood estimation of the model was run with the R package lme4 version 1.1.30 (Bates et al., 2015). The model showed a marginal R2 = 0.333 and a conditional R2 = 0.361. The model estimates are reported in Table 5. Additionally, the two considered interactions on students’ mathematical modeling competence are visualized in Figure 3.
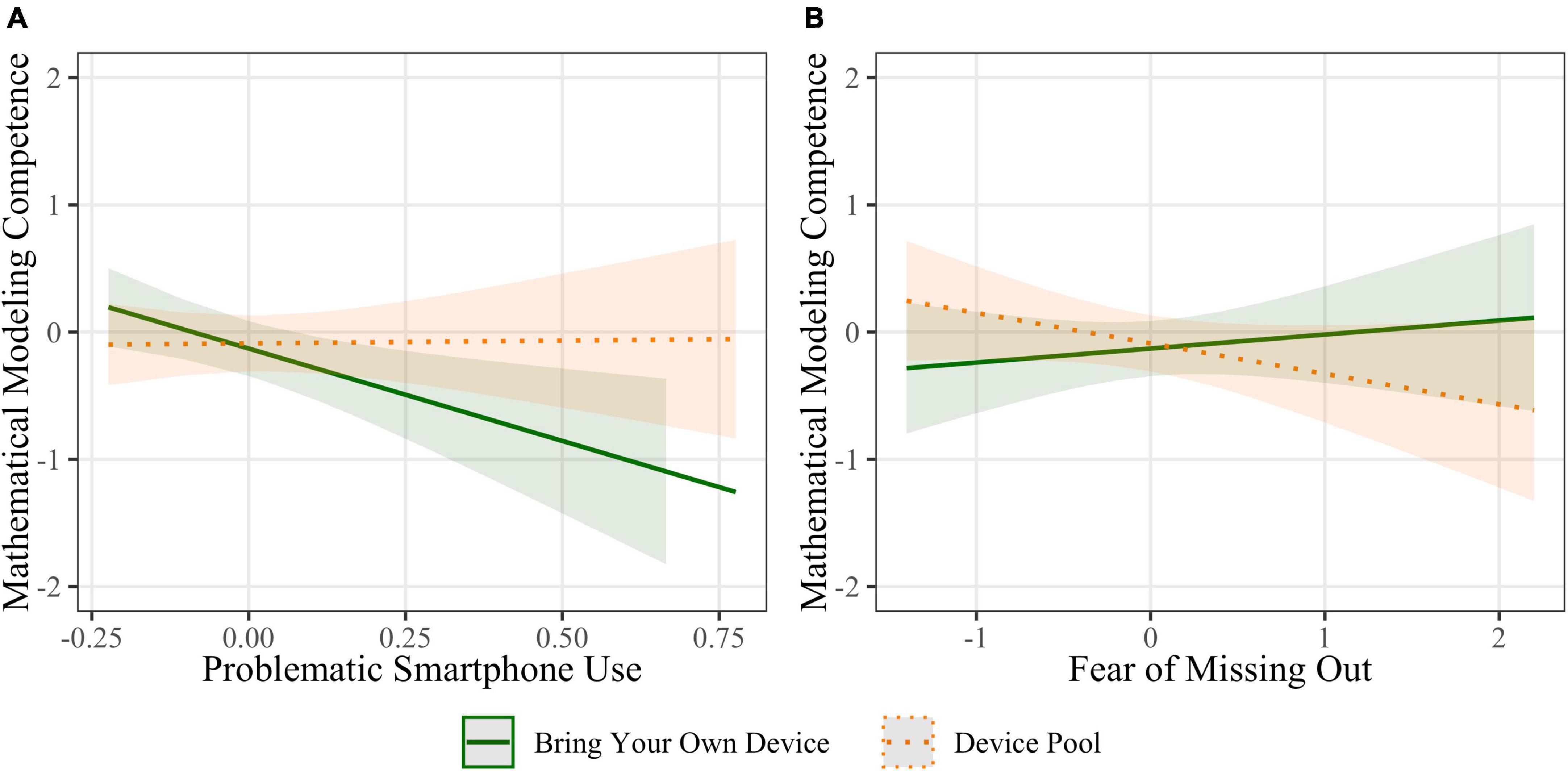
Figure 3. Interaction effects of the mobile device access concept with (A) students’ problematic smartphone use or (B) students’ fear of missing out on their mathematical modeling competence along with the 95% CIs.
For students with average problematic smartphone use and average fear of missing out, we did not find a simple effect (p = 0.76) of the mobile device access concept on students’ mathematical modeling competence after the workshop (cf. Hypothesis 1).
However, we found an interaction effect (p = 0.039) between the mobile device access concept and students’ problematic smartphone use (Hypothesis 2.1, see Figure 3A). We then estimated marginal means of the problematic smartphone use effect and of students’ outcome mathematical modeling competence using the R package emmeans version 1.8.8 (Lenth, 2023). The effect of students’ problematic smartphone use on their mathematical modeling competence differed, t (265) = −2.03, p = 0.04, between the BYOD group (B = −1.45, 95% CI [−2.45, −0.46]) and the pool group (B = 0.04, 95% CI [−1.01, 1.09]). While the effect was significantly different from zero in the BYOD group (Hypothesis 2.2), t (261) = −2.87, p = 0.004, it was not in the pool group (Hypothesis 2.3), t (267) = 0.08, p = 0.94. The reduced model (i.e., the one without the simple effect of problematic smartphone use) showed an AIC of 799.69 and a BIC of 831.77. These were both lower than the AIC of 801.69 and the BIC of 837.33 obtained for the full model. The estimated marginal means of students’ outcome mathematical modeling competence are reported in Table 6 (Hypotheses 2.4 and 2.5). While the mathematical modeling competence was not significantly different between the two groups for students with minimal problematic smartphone use (p = 0.17), for students with maximal problematic smartphone use it was. These students achieved a higher competence when utilizing provided devices (BBYOD−Pool = −1.20, 95% CI [–2.35, –0.05]).
We did not find an interaction effect (p = 0.12) between the mobile device access concept and students’ fear of missing out (see Figure 3B, cf. Hypothesis 3.1). Also, the simple effect of students’ fear of missing out was non-significant (p = 0.15).
4 Discussion
Hypothesis 1, that the mobile device access concept affects the mathematical modeling competence of students with average problematic smartphone use and average fear of missing out, is not supported by the results. As outlined in the introduction, there are contrary indications on the preference for a mobile device access concept. The potential for distraction of the digital devices, as found by Karsenti and Fievez (2013), rather favors the pool concept, whereas the effects of the personal device ownership indicate benefits of the BYOD concept (Burden et al., 2012; Doargajudhur and Dell, 2020). We interpret our result as an indication that the different characteristics of BYOD and pool might actually be counterbalancing each other. And thus, other mobile device access concepts combining the positive effects and, at the same time, encountering the negative ones, could reveal as favorable.
Hypothesis 2.1, that the mobile device access concept interacts with students’ problematic smartphone use, is supported by the results. Further, also Hypotheses 2.2 and 2.3 are supported. While Hypothesis 2.4 is not supported by the data, Hypothesis 2.5 is. When learning with their own devices, students’ mathematical modeling competence was negatively affected by an increasing level of problematic smartphone use (Hypothesis 2.2). This is in line with the previous findings (Sunday et al., 2021; Zhou et al., 2022a,b). When learning with a provided device, however, there is no such effect (Hypothesis 2.3). This also fits with the finding that the effect of students’ problematic smartphone use is moderated by in-class multitasking, given that multitasking in the pool concept requires a lot of effort. Regarding students with minimal problematic smartphone use, we were not able to show a difference in students’ mathematical modeling competence between the two access concepts (cf. Hypothesis 2.4). Regarding students with a rather high problematic smartphone use, the mathematical modeling competence of students in the BYOD group was lower than the one of students in the pool group (Hypothesis 2.5). This again fits the finding of the moderating role of in-class multitasking (Sunday et al., 2021), since it is much easier in the BYOD concepts than in the pool concept to use social media. Overall, in terms of competences, the choice of the mobile device access concept thus may be of great importance for certain students. We interpret our results such that the appropriate choice of a concept can help counter negative effects caused by unfavorable student characteristics.
Hypothesis 3.1, that the mobile device access concept interacts with students’ fear of missing out, is not supported by the results. Taking a close look at Hypotheses 3.2 to 3.5 and the research findings forming the basis of them, it seems reasonable that we could not detect an interaction. We expected a negative effect for both access concepts with either in-class social media use or mobile unavailability being involved (Hypotheses 3.2 and 3.3) and we were also unsure about which access concept would be advantageous for students with high fear of missing out (Hypothesis 3.5). As there was no interaction effect, we therefore did not test Hypotheses 3.2 to 3.5, but instead directly evaluated the simple effect of students’ fear of missing out. However, this effect also was not significant. While this is contrary to our assumptions (Hypotheses 3.2 and 3.3) based on the previous findings (van der Schuur et al., 2015; Shane-Simpson and Bakken, 2022; Zhao, 2023), a possible explanation might be the fact that we included students’ problematic smartphone use in the same model and the correlation between these two predictors (see Table 4).
4.1 Limitations and strengths
In this study, we examined different effects on students’ mathematical modeling competence. We did so because of the diverse utilization possibilities of the digital devices during the modeling process (Greefrath and Siller, 2017). We do not explicitly claim any generalizability of the results to other mathematical or non-mathematical competences. However, it is conceivable that the results would generalize to other competences and subjects, as the constructs problematic smartphone use and fear of missing out as well as the different mobile device access concepts are not related to the content of mathematical modeling.
For this study, we did not purposefully select a representative sample. However, as the convenience sampling and self-selection took place on the teacher level, we assume that this does not bias our results as much as they would have been by convenience sampling and self-selection on student level. Nonetheless, also in our study, students could refuse to participate—but only a few did.
To test our hypotheses, we limited our research to 8th and 9th grade students in the academic track of the German school system. While we tend to assume the generalizability of the results to same-aged students visiting other types of schools, the question remains open regarding differences to younger and older students.
Because ownership of personal tablets by students was not as widespread as ownership of personal smartphones at the time of study planning and is still not today, we could only examine the BYOD concept on the basis of smartphones. It remains unclear, whether the results can be generalized to tablets. On a technical level, smartphones and tablets appear to be quite similar, but very different types of use cannot be ruled out. When investigating other mobile device access concepts, like the COPE concept, future studies should consider using tablets for this.
4.2 Implications
Our results do not reveal a mobile device access concept (BYOD or pool) to be favored in principle (i.e., for an average student). Rather, we found an interaction between the access concept and students’ problematic smartphone use. This is a new contribution to the field of research on problematic smartphone use and its effect on student achievement. We found that there is a negative effect of problematic smartphone use in the BYOD concept only and not in the pool concept. Further research should investigate how other access concepts, that could not be considered in this study, align with the findings.
Further research should also investigate the exact role of in-class multitasking. It might be worth to consider in-class multitasking as a mediator rather than a moderator between students’ problematic smartphone use and their academic achievement.
Our study revealed that the effect of students’ problematic smartphone use on their competence depends on the chosen mobile device access concept. As a consequence, teachers noticing students that are negatively affected when utilizing digital devices should consider the application of a stricter access concept.
Data availability statement
The raw data supporting the conclusions of this article will be made available by the authors, without undue reservation.
Ethics statement
Ethical approval was not required for the study involving human samples in accordance with the local legislation and institutional requirements. Written informed consent for participation in this study was provided by the participants’ legal guardians/next of kin.
Author contributions
MK: Methodology, Software, Formal analysis, Investigation, Data curation, Writing – original draft, Writing – review & editing, and Visualization. GG, CD, and AM: Conceptualization, Writing – review & editing, Supervision, and Funding acquisition. BF: Methodology, Formal analysis, and Writing – review & editing. FK: Investigation, Data curation, and Writing – review & editing. FR and DM: Writing – review & editing. DL: Conceptualization, Data curation, Writing – review & editing, and Funding acquisition. SuH, TQ, and ES: Conceptualization, Writing – review & editing, and Funding acquisition. BL: Writing – review & editing and Project administration. MU: Investigation and Writing – review & editing. StH: Conceptualization, Writing – review & editing, Supervision, Project administration, and Funding acquisition. All authors contributed to the article and approved the submitted version.
Funding
This research was part of the smart for science project. The project smart for science was funded by the German Federal Ministry of Education and Research under grant number 01JD1827. The funding source was not involved in the study design, the data collection, the data analysis, the data interpretation, the writing of the report, or the decision to submit the article for publication.
Conflict of interest
The authors declare that the research was conducted in the absence of any commercial or financial relationships that could be construed as a potential conflict of interest.
Publisher’s note
All claims expressed in this article are solely those of the authors and do not necessarily represent those of their affiliated organizations, or those of the publisher, the editors and the reviewers. Any product that may be evaluated in this article, or claim that may be made by its manufacturer, is not guaranteed or endorsed by the publisher.
References
American Psychiatric Association (2013). Diagnostic and statistical manual of mental disorders, 5th Edn. Washington, DC: American Psychiatric Publishing. doi: 10.1176/appi.books.9780890425596
American Psychiatric Association (2022). Diagnostic and statistical manual of mental disorders, 5th-TR Edn. Washington, DC: American Psychiatric Publishing. doi: 10.1176/appi.books.9780890425787
Bates, D., Mächler, M., Bolker, B., and Walker, S. (2015). Fitting linear mixed-effects models using lme4. J. Stat. Soft. 67, 1–48. doi: 10.18637/jss.v067.i01
Blomhøj, M., and Jensen, T. H. (2003). Developing mathematical modelling competence: Conceptual clarification and educational planning. Teach. Math. Appl. 22, 123–139. doi: 10.1093/teamat/22.3.123
Blomhøj, M., and Jensen, T. H. (2007). “What’s all the fuss about competencies?,” in Modelling and applications in mathematics education: The 14th ICMI study New ICMI Study Series, eds W. Blum, P. L. Galbraith, H.-W. Henn, and M. Niss (Boston, MA: Springer), 45–56. doi: 10.1007/978-0-387-29822-1_3
Blum, W., Neubrand, M., Ehmke, T., Senkbeil, M., Jordan, A., Ulfig, F., et al. (2004). “Mathematische Kompetenz,” in PISA 2003: Der Bildungsstand der Jugendlichen in Deutschland: Ergebnisse des zweiten internationalen Vergleichs, eds M. Prenzel, J. Baumert, W. Blum, R. Lehmann, D. Leutner, M. Neubrand, et al. (Münster: Waxmann), 47–92.
Borba, M. C., and Villarreal, M. E. (2005). Humans-with-media and the reorganization of mathematical thinking. New York, NY: Springer. doi: 10.1007/b105001
Brown, A., and Croudace, T. J. (2015). “Scoring and estimating score precision using multidimensional IRT models,” in Handbook of item response theory modeling: Applications to typical performance assessment, eds S. P. Reise and D. A. Revicki (New York, NY: Routledge), 307–333. doi: 10.4324/9781315736013
Bühner, M., and Ziegler, M. (2017). Statistik für Psychologen und Sozialwissenschaftler, 2nd Edn. Hallbergmoos: Pearson Deutschland.
Burden, K., Hopkins, P., Male, T., Martin, S., and Trala, C. (2012). iPad Scotland evaluation. Hull: The University of Hull. doi: 10.13140/2.1.3593.5363
Burrill, G., Allison, J., Breaux, G., Kastberg, S., Leatham, K., and Sanchez, W. (2002). Handheld graphing technology in secondary mathematics: Research findings and implications for classroom practice. Dallas, TX: Texas Instruments. Available online at: http://ti-researchlibrary.com/Lists/TI%20Education%20Technology%20%20Research%20Library/Attachments/122/GC%20in%20secondary%20math%20-%20research%20findings%20and%20implications%20-%20Burrill%202002%20-%20(yellow%20book)%20CL2872,%20review.pdf
De-Sola Gutiérrez, J., Rodríguez De Fonseca, F., and Rubio, G. (2016). Cell-phone addiction: A review. Front. Psychiatry 7:175. doi: 10.3389/fpsyt.2016.00175
Doargajudhur, M. S., and Dell, P. (2020). The effect of bring your own device (BYOD) adoption on work performance and motivation. J. Comput. Inf. Syst. 60, 518–529. doi: 10.1080/08874417.2018.1543001
Dochy, F., Segers, M., and Buehl, M. M. (1999). The relation between assessment practices and outcomes of studies: The case of research on prior knowledge. Rev. Educ. Res. 69, 145–186. doi: 10.3102/00346543069002145
Drijvers, P., Ball, L., Barzel, B., Heid, M. K., Cao, Y., and Maschietto, M. (2016). Uses of technology in lower secondary mathematics education: A concise topical survey. Cham: Springer Open. doi: 10.1007/978-3-319-33666-4
Eickelmann, B., Bos, W., Gerick, J., Goldhammer, F., Schaumburg, H., Schwippert, K., et al. (eds.) (2019). ICILS 2018 #Deutschland: Computer- und informationsbezogene Kompetenzen von Schülerinnen und Schülern im zweiten internationalen Vergleich und Kompetenzen im Bereich Computational Thinking. Münster: Waxmann. Available online at: https://www.waxmann.com/index.php?eID=download&buchnr=4000
Elhai, J. D., Dvorak, R. D., Levine, J. C., and Hall, B. J. (2017). Problematic smartphone use: A conceptual overview and systematic review of relations with anxiety and depression psychopathology. J. Affect. Disord. 207, 251–259. doi: 10.1016/j.jad.2016.08.030
Ellington, A. J. (2003). A meta-analysis of the effects of calculators on students’ achievement and attitude levels in precollege mathematics classes. J. Res. Math. Educ. 34, 433–463. doi: 10.2307/30034795
Ferrando, P. J., and Lorenzo-Seva, U. (2018). Assessing the quality and appropriateness of factor solutions and factor score estimates in exploratory item factor analysis. Educ. Psychol. Meas. 78, 762–780. doi: 10.1177/0013164417719308
Galbraith, P., Goos, M., Renshaw, P., and Geiger, V. (2003). “Technology enriched classrooms: Some implications for teaching applications and modelling,” in Mathematical modelling in education and culture: ICTMA 10, eds Q.-X. Ye, W. Blum, K. S. Houston, and Q.-Y. Jiang (Chichester: Horwood Publishing), 111–125. doi: 10.1533/9780857099556.3.111
Greefrath, G., and Siller, H.-S. (2017). “Modelling and simulation with the help of digital tools,” in Mathematical modelling and applications, eds G. A. Stillman, W. Blum, and G. Kaiser (Cham: Springer International Publishing), 529–539. doi: 10.1007/978-3-319-62968-1_44
Greefrath, G., Hertleif, C., and Siller, H.-S. (2018). Mathematical modelling with digital tools: A quantitative study on mathematising with dynamic geometry software. ZDM Math. Educ. 50, 233–244. doi: 10.1007/s11858-018-0924-6
Hillmayr, D., Ziernwald, L., Reinhold, F., Hofer, S. I., and Reiss, K. M. (2020). The potential of digital tools to enhance mathematics and science learning in secondary schools: A context-specific meta-analysis. Comput. Educ. 153:103897. doi: 10.1016/j.compedu.2020.103897
Hox, J. J., Moerbeek, M., and van de Schoot, R. (2017). Multilevel analysis: Techniques and applications, 3rd Edn. New York, NY: Routledge. doi: 10.4324/9781315650982
Huang, F. L. (2018). Multilevel modeling myths. Sch. Psychol. Q. 33, 492–499. doi: 10.1037/spq0000272
Hussain, Z., Griffiths, M. D., and Sheffield, D. (2017). An investigation into problematic smartphone use: The role of narcissism, anxiety, and personality factors. J. Behav. Addict. 6, 378–386. doi: 10.1556/2006.6.2017.052
Institut zur Qualitätsentwicklung im Bildungswesen (n.d.). Beispielaufgaben Mathematik Sek I. Available online at: https://www.iqb.hu-berlin.de/vera/aufgaben/ma1/
Kaiser, G. (2017). “The teaching and learning of mathematical modeling,” in Compendium for research in mathematics education, ed. J. Cai (Reston, VA: National Council of Teachers of Mathematics), 267–291.
Karsenti, T., and Fievez, A. (2013). The iPad in education: Uses, benefits, and challenges: A survey of 6,057 students and 302 teachers in Quebec (Canada). Montreal, QC: CRIFPE.
Kneidinger-Müller, B. (2019). When the smartphone goes offline: A factorial survey of smartphone users’ experiences of mobile unavailability. Comput. Hum. Behav. 98, 1–10. doi: 10.1016/j.chb.2019.03.037
Köller, O. (2016). “Bildungsstandards,” in Handbuch Bildungsforschung, eds R. Tippelt and B. Schmidt-Hertha (Wiesbaden: Springer Fachmedien), 1–24. doi: 10.1007/978-3-531-20002-6_26-1
Krause, M. (2022). “Schülereigene oder gestellte Geräte? Eine Analyse der Auswirkungen auf die Kompetenzentwicklung,” in Digitales Lernen in Distanz und Präsenz: Herbsttagung 2021 des Arbeitskreises Mathematikunterricht und digitale Werkzeuge in der Gesellschaft für Didaktik der Mathematik am 24.09.2021, eds F. Reinhold and F. Schacht (Essen: DuEPublico), 73–79. doi: 10.17185/duepublico/76035
Kyllonen, P., and Kell, H. (2017). “What is fluid intelligence? Can it be improved?,” in Cognitive abilities and educational outcomes, eds M. Rosén, K. Yang Hansen, and U. Wolff (Cham: Springer), 15–37. doi: 10.1007/978-3-319-43473-5_2
Lemmens, J. S., Valkenburg, P. M., and Gentile, D. A. (2015). The internet gaming disorder scale. Psychol. Assess. 27, 567–582. doi: 10.1037/pas0000062
Lenth, R. V. (2023). Emmeans: Estimated marginal means, aka least-squares means. Available online at: https://cran.r-project.org/package=emmeans (accessed October 02, 2023).
Maaß, K. (2006). What are modelling competencies? ZDM Math. Educ. 38, 113–142. doi: 10.1007/BF02655885
Mahler, N., Schipolowski, S., and Weirich, S. (2019). “Anlage und Durchführung,” in IQB-Bildungstrend 2018: Mathematische und naturwissenschaftliche Kompetenzen am Ende der Sekundarstufe I im zweiten Ländervergleich, eds P. Stanat, S. Schipolowski, N. Mahler, S. Weirich, and S. Henschel (Münster: Waxmann), 99–124.
Maser, J. D., and Akiskal, H. S. (2002). Spectrum concepts in major mental disorders. Psychiatr. Clin. North Am. 25, xi–xiii. doi: 10.1016/S0193-953X(02)00034-5
McGrew, K. S. (2009). CHC theory and the human cognitive abilities project: Standing on the shoulders of the giants of psychometric intelligence research. Intelligence 37, 1–10. doi: 10.1016/j.intell.2008.08.004
Medienpädagogischer Forschungsverbund Südwest (2022). JIM-Studie 2022: Jugend, Information, Medien: Basisuntersuchung zum Medienumgang 12- bis 19-Jähriger in Deutschland. Available online at: https://www.mpfs.de/fileadmin/files/Studien/JIM/2022/JIM_2022_Web_final.pdf (accessed December 01, 2023).
Merck, M. F., Gallagher, M. A., Habib, E., and Tarboton, D. (2021). Engineering students’ perceptions of mathematical modeling in a learning module centered on a hydrologic design case study. Int. J. Res. Undergrad. Math. Educ. 7, 351–377. doi: 10.1007/s40753-020-00131-8
Ministerium für Schule, Jugend und Kinder des Landes Nordrhein-Westfalen (ed.) (2004). Kernlehrplan für die Gesamtschule – Sekundarstufe I in Nordrhein-Westfalen: Mathematik. 1st Edn. Frechen: Ritterbach Verlag. Available online at: https://www.schulentwicklung.nrw.de/lehrplaene/upload/lehrplaene_download/gesamtschule/gs_mathematik.pdf
Ministerium für Schule und Weiterbildung des Landes Nordrhein-Westfalen (ed.) (2007). Kernlehrplan für das Gymnasium – Sekundarstufe I (G8) in Nordrhein-Westfalen: Mathematik, 1st Edn. Frechen: Ritterbach Verlag. Available online at: https://www.schulentwicklung.nrw.de/lehrplaene/lehrplan/46/gym8_mathematik.pdf
Mitchell, L., and Hussain, Z. (2018). Predictors of problematic smartphone use: An examination of the integrative pathways model and the role of age, gender, impulsiveness, excessive reassurance seeking, extraversion, and depression. Behav. Sci. 8:74. doi: 10.3390/bs8080074
Molina-Toro, J. F., Rendón-Mesa, P. A., and Villa-Ochoa, J. A. (2019). Research trends in digital technologies and modeling in mathematics education. Eurasia J. Math. Sci. Technol. Educ. 15:em1736. doi: 10.29333/ejmste/108438
Murauer, R. (2017). BYO[m]D – bring your own [mobile] device: Eine empirische Analyse der, aus Sicht der Lehrkräfte, erforderlichen Rahmenbedingungen für die Implementierung von schülereigenen Smartphones und Tablets im Unterricht. Available online at: https://ediss.sub.uni-hamburg.de/handle/ediss/7073 (accessed December 01, 2023).
National Council of Teachers of Mathematics (2000). Principles and standards for school mathematics. Reston, VA: National Council of Teachers of Mathematics.
Niss, M., Blum, W., and Galbraith, P. (2007). “Introduction,” in Modelling and applications in mathematics education: The 14th ICMI study New ICMI Study Series, eds W. Blum, P. L. Galbraith, H.-W. Henn, and M. Niss (Boston, MA: Springer), 3–32. doi: 10.1007/978-0-387-29822-1_1
Pant, H. A., Stanat, P., Pöhlmann, C., and Böhme, K. (2013). “Die Bildungsstandards im allgemeinbildenden Schulsystem,” in IQB-Ländervergleich 2012: Mathematische und naturwissenschaftliche Kompetenzen am Ende der Sekundarstufe I, eds H. A. Pant, P. Stanat, U. Schroeders, A. Roppelt, T. Siegle, and C. Pöhlmann (Münster: Waxmann), 13–21. Available online at: https://www.iqb.hu-berlin.de/bt/lv2012/Bericht/Bericht.pdf
Peng, P., Wang, T., Wang, C., and Lin, X. (2019). A meta-analysis on the relation between fluid intelligence and reading/mathematics: Effects of tasks, age, and social economics status. Psychol. Bull. 145, 189–236. doi: 10.1037/bul0000182
Pierce, R. (2005). Algebraic insight underpins the use of CAS for modelling. Math. Enthus. 2, 107–117. doi: 10.54870/1551-3440.1027
Przybylski, A. K., Murayama, K., DeHaan, C. R., and Gladwell, V. (2013). Motivational, emotional, and behavioral correlates of fear of missing out. Comput. Hum. Behav. 29, 1841–1848. doi: 10.1016/j.chb.2013.02.014
Radesky, J. S., Weeks, H. M., Schaller, A., Robb, M. B., Mann, S., and Lenhart, A. (2023). Constant companion: A week in the life of a young person’s smartphone use. San Francisco, CA: Common Sense. Available online at: https://www.commonsensemedia.org/sites/default/files/research/report/2023-cs-smartphone-research-report_final-for-web.pdf
Reer, F., Tang, W. Y., and Quandt, T. (2019). Psychosocial well-being and social media engagement: The mediating roles of social comparison orientation and fear of missing out. New Media Soc. 21, 1486–1505. doi: 10.1177/1461444818823719
Reer, F., Wehden, L.-O., Janzik, R., and Quandt, T. (2022). Examining the interplay of smartphone use disorder, mental health, and physical symptoms. Front. Public Health 10:834835. doi: 10.3389/fpubh.2022.834835
Richardson, M., Hussain, Z., and Griffiths, M. D. (2018). Problematic smartphone use, nature connectedness, and anxiety. J. Behav. Addict. 7, 109–116. doi: 10.1556/2006.7.2018.10
Robitzsch, A., Kiefer, T., and Wu, M. (2022). TAM: Test analysis modules. Available online at: https://CRAN.R-project.org/package=TAM (accessed October 02, 2023).
Rosseel, Y. (2012). Lavaan: An R package for structural equation modeling. J. Stat. Softw. 48, 1–36. doi: 10.18637/jss.v048.i02
Shane-Simpson, C., and Bakken, T. (2022). Students’ fear of missing out predicts in-class social media use. Teach. Psychol. doi: 10.1177/00986283211060752 [Epub ahead of print].
Sunday, O. J., Adesope, O. O., and Maarhuis, P. L. (2021). The effects of smartphone addiction on learning: A meta-analysis. Comput. Hum. Behav. Rep. 4:100114. doi: 10.1016/j.chbr.2021.100114
United Nations Educational, Scientific and Cultural Organization (2013). The future of mobile learning: Implications for policy makers and planners. Paris: UNESCO.
van den Eijnden, R. J. J. M., Lemmens, J. S., and Valkenburg, P. M. (2016). The social media disorder scale. Comput. Hum. Behav. 61, 478–487. doi: 10.1016/j.chb.2016.03.038
van der Schuur, W. A., Baumgartner, S. E., Sumter, S. R., and Valkenburg, P. M. (2015). The consequences of media multitasking for youth: A review. Comput. Hum. Behav. 53, 204–215. doi: 10.1016/j.chb.2015.06.035
Villa-Ochoa, J. A., and Suárez-Téllez, L. (2021). “Computer algebra systems and dynamic geometry for mathematical thinking,” in Handbook of cognitive mathematics, ed. M. Danesi (Cham: Springer), 1–27. doi: 10.1007/978-3-030-44982-7_36-1
Weiß, R. H. (2019). CFT 20-R: Grundintelligenztest Skala 2 – Revision, 2nd Edn. Göttingen: Hogrefe Verlag.
Zhao, L. (2023). Social media multitasking and college students’ academic performance: A situation–organism–behavior–consequence perspective. Psychol. Sch. 60, 3151–3168. doi: 10.1002/pits.22912
Zhou, D., Liu, J., Wang, T., Liu, J., and Li, G. (2022a). Relationships among problematic smartphone use, mathematics anxiety, learning interest, and achievement: A multiple mediation model. Comput. Hum. Behav. 129:107171. doi: 10.1016/j.chb.2021.107171
Keywords: bring your own device, mobile device access concept, learning with digital resources, media in education, mathematical modeling, problematic smartphone use, fear of missing out, technology planning
Citation: Krause M, Greefrath G, Forthmann B, Kremer FE, Reer F, Laumann D, Masemann D, Denz C, Heinicke S, Leibrock B, Marohn A, Quandt T, Souvignier E, Ubben M and Heusler S (2024) Effects of student-owned and provided mobile devices on mathematical modeling competence: investigating interaction effects with problematic smartphone use and fear of missing out. Front. Educ. 9:1167114. doi: 10.3389/feduc.2024.1167114
Received: 16 February 2023; Accepted: 08 January 2024;
Published: 01 March 2024.
Edited by:
Graham Pluck, Chulalongkorn University, ThailandReviewed by:
Yingxiao Qian, University of South Carolina, United StatesMayra Urrea-Solano, University of Alicante, Spain
Copyright © 2024 Krause, Greefrath, Forthmann, Kremer, Reer, Laumann, Masemann, Denz, Heinicke, Leibrock, Marohn, Quandt, Souvignier, Ubben and Heusler. This is an open-access article distributed under the terms of the Creative Commons Attribution License (CC BY). The use, distribution or reproduction in other forums is permitted, provided the original author(s) and the copyright owner(s) are credited and that the original publication in this journal is cited, in accordance with accepted academic practice. No use, distribution or reproduction is permitted which does not comply with these terms.
*Correspondence: Maurice Krause, bWF1cmljZS5rcmF1c2VAdW5pLW11ZW5zdGVyLmRl