- 1Promoter Network of Diagnostic Evaluation Methods and Educational Innovation, Ensenada, Mexico
- 2Autonomous University of Baja California, Mexicali, Mexico
The psychometric properties of the Scale to measure the attitude of researchers for scientific-technological collaboration between universities and industry (EA-COOPTEC, for its acronym in Spanish) were analyzed, specifically evidence of construct validity of the internal structure and invariance for gender and age was obtained. The EA-COOPTEC was administered to 179 academic Teachers–Researchers (T-Rs) involved in Universities-Industry Collaboration (UIC) activities: 50 women and 129 men. Participants had a median age of 36–40 years. A descriptive analysis was implemented, as well as an Exploratory and Confirmatory Factor Analysis (EFA and CFA), and a method of succession of nested models for invariance testing. A four-factor model explaining the perception of UIC activities was generated and evidence of invariance for gender and partial invariance for age was obtained. Acceptable fit indices were obtained for the configurational, weak, strong and strict. Given the results, we recommend the use of the EA-COOPTEC v0.1 for the analysis of the mean differences between genders and age in T-Rs.
1. Introduction
Cooperation is seen as a process in which human capital skills are focused to produce ideas and knowledge (Bozeman et al., 2013). For cooperation to work, human capital must be able to communicate effectively with each other, have a willingness to work cooperatively or collaboratively, and be driven by a common goal (Taboada, 2004; Smit et al., 2020). Given its importance, organizations are increasingly focused on participating in a cooperative manner with the objective of obtaining competitive advantages; due to the fact that collaboration between organizations encourages the production of economic, material and intellectual resources (Etzkowitz, 1998; Agrawal, 2001; Lundvall, 2004).
García-Galván (2011) identifies different types of collaboration between organizations: vertical, when collaborative occurs between organizations with complementary activities; horizontal, when it occurs between competitors and the objective is to achieve economies of scale; and inter-organizational or transversal, when it occurs between universities and industry. In particular, the relationship between universities-industry brings notable benefits to society; some of them are: increasing competitiveness among industry (Burrone, 2005), promoting theoretical and methodological progress in the field of science, encouraging the capitalization of knowledge (Etzkowitz, 1998, 2003), and turning universities and research centers into an important actor for economic development (García-Galván, 2018). According to Santoro (2000), and Santoro and Chakrabarti (2002), there are four types of Universities-Industry Collaboration (UIC): research support, cooperative research, knowledge transfer, and technology transfer. Research support, refers to financial and equipment contributions made to universities by industry. Cooperative research includes contract research with individual investigators, consulting by faculty, and certain group arrangements specifically to address immediate industry problems. Knowledge transfer encompasses highly interactive activities that include ongoing formal and informal personal interactions, cooperative education, curriculum development, and staff exchanges. Technology transfer also involves highly interactive activities, but its focus is on addressing immediate and more industry-specific issues.
Likewise, the relationships between universities-industry are not static and are influenced by different factors (García-Galván, 2008). In this regard, Ankrah and AL-Tabbaa (2015) identified that these can be classified as: informal personal relationships (e.g., individual consulting and conferences), formal personal relationships (e.g., internships and use of facilities), third-party relationship (e.g., institutional consulting and industry partnerships), formal directed agreements (e.g., patent and licensing agreements and joint research projects), formal non-directed agreements (e.g., funding of university positions and research grants), and focused structures (e.g., incubators and subsidiaries). Additionally, Morales and García-Galván (2019) distinguished that among the motivations for engaging in techno-scientific cooperation, the search for additional sources of funding and changing policies that promote collaboration stand out. They also emphasized other motivations such as technology transfer between organizations, technological complementarity, individual risk and cost reduction, and new business opportunities.
Since its origins, UIC has been studied from various perspectives: Innovation systems, as a normative approach to public policies (Lundvall, 1992; Freeman, 1995; Edquist, 1997; Vertova, 2014); Mode 2 of knowledge production (Gibbons et al., 1997), which integrates the business/industrial vision; the Triple helix model (Etzkowitz and Leydesdorff, 1995; Leydesdorff and Etzkowitz, 1996), as a pioneering antecedent of the university with a business vision; and, finally, the Integrated Contemporary Institutionalism (ICI) (Taboada, 2004; García-Galván, 2008), as an emerging theoretical approach that supports the formal study of the UIC. Especially, ICI gives meaning to the role of institutions through the multidisciplinary study of their relationships and their effects (García-Galván, 2008). From this point of view, UIC is considered a key asset for economic development and competitive advantages in the production sector (Teece et al., 1998). In turn, this relationship is motivated by the scarce public investment for scientific-technological development (García-Galván, 2008). The ICI, unlike classical models, does not make use of articles or patents as indicators of scientific-technological UIC (García-Galván, 2008) but employs both qualitative and quantitative methods to study the phenomenon and different factors organized in three domains: Individual, Organizational, and Institutional (Morales, 2019).
There are currently a variety of indicators to measure different types of UIC activities (Gardner et al., 2010; Seppo and Lilles, 2012). In particular, the measurement of perception of T-Rs to UIC is a little explored field, the closest studies are those of López-Martínez et al. (2007), Boardman and Ponomariov (2009), Giuliani et al. (2010), Cudic et al. (2021), Da Silva and Sartori (2022), and Morales (2019). Cudic et al. (2021) measured UIC by constructing indicators based on data reported by different sources with the purpose of studying the relationship between predictors of UIC and its outcomes. For the construct measures, the authors based the theoretical framework of this research on a systematic review of the relevant academic literature, taking into account the availability of variable data. Initially, the authors obtained 36 measures. These were grouped into six constructs, four of which focus on input factors and the remaining two on output factors: institutional factors, human factors, linkage factors, framework factors, intangible output indicators and tangible output indicators. The authors found that European countries that invest in measuring predictors of UIC perform better. Based on statistical analysis (partial least squares structural equation modeling), the authors identified that investments in knowledge, networking, and investment in Research and Development (R&D), in general, are the most significant predictors of fostering UIC. Likewise, the authors identified that some of the main obstacles to UIC are: the lack of alignment of incentives between researchers and companies (conflicts with companies), and the lack of academic procedures or intermediaries that facilitate interaction with companies (academic networking problems) where the attitude and perception of researchers play a relevant role (Muscio and Vallanti, 2014).
Da Silva and Sartori (2022) designed a questionnaire based on a systematic review of the literature to highlight and compare the main motivations and barriers to UIC in Brazilian and Irish Technology Transfer Offices (TTOs). The questionnaire was applied to the heads of nine TTOs and consisted of six questions (four open and two closed). The closed questions, which sought to analyze the degree of frequency, were transformed into quantitative data in percentage form, which were later tabulated. The open questions which were intended to raise general information about the university and the TTOs. The authors concluded that the TTOs from Ireland and Brazil have different motivations for cooperation, but face similar barriers despite the completely different contexts they are in. This highlights the importance of measuring indicators constructed based on stakeholder perspectives that help explain UIC barriers.
Boardman and Ponomariov (2009) used a national survey of tenured scientists and their careers in the United States to identify those personal and professional characteristics that arise from the interaction of university scientists with private companies, as well as the different forms of interaction. In particular, among their demonstrations, they identify professional and personal variables as significant predictors of the interactions of scientists with the private sector, including: funding sources, institutional affiliations, type of ownership, student support, scientific values and demographic attributes. Giuliani et al. (2010) analyzed the importance of the individual characteristics of researchers and their institutional environments to explain the propensity to participate in different types of UIC. Drawing on original databases, it presents evidence on three wine-producing areas: Piedmont, in Italy, Chile, and South Africa. Their findings reveal those individual characteristics of researchers, such as centrality in the academic system, age, and gender, matter more than the number of publications or their formal titles.
López-Martínez et al. (2007) conducted an exploratory survey of 31 researchers at the National Autonomous University of Mexico (UNAM, by acronyms in Spanish) and 28 Mexican entrepreneurs; besides, they conducted a panel discussion among the respondents designed to obtain in-depth qualitative data on motivations and obstacles for UIC. The questionnaire scales were used to explore 19 motivations and 27 obstacles reported by academic researchers for UIC; and 15 motivations and 27 obstacles reported by business executives. The items were to be scored on a 5-point rating scale (0 = not important, to 4 = determinant), and respondents were asked to suggest and score additional motivations and obstacles that were not included in the instruments. With these instruments, the authors were able to: (a) learn the main motivations of university researchers to conduct technological research and seek links with industry, (b) learn the main motivations of industrial entrepreneurs to establish cooperation with universities to develop technology, and (c) identify the main barriers to a healthy relationship between the two institutions. However, the authors did not provide evidence of validity or reliability of the scales used. They concluded by presenting the factors identified in the study as key elements in the development of relations between the two sectors in Latin American countries, and argued the need to rethink our conceptions of motivations and obstacles to UIC within a theoretical framework of inter-institutional communication and organizational cultural change.
In particular, one of the most recent instruments in this field is the Questionnaire to measure the perception of scientific-technological collaboration between universities and industry (CP-COOPTEC), developed by Morales (2019). The CP-COOPTEC was made up of 71 items (k), organized into four sections: (a) Sociodemographic and labor data (k = 7), (b) Individuals variables associated with scientific-technological UIC (k = 39), (c) Organizational variables (k = 13), and (d) Institutional variables (k = 12). The Individual section refers to the knowledge, experiences, attitudes and motivations with respect to the Teacher-Researchers’ (T-Rs) UIC. This is divided into two dimensions: Attitudes about UIC and Institutional Supports. The first, are defined as the beliefs, attitudes and values of individuals, and how these are continuously related, forming a system, which is a predictor of the individual’s behaviors and responses (Rokeach, 1968). For its part, the dimension of Institutional Supports refers to the financial, formative and procedural help provided by universities and research centers to industries (Casalet and Casas, 1998; Etzkowitz, 1998; Bajo, 2006; García-Galván, 2013; López-Leyva, 2014). The Organizational section refers to the organizational behavior of UIC. It is made up of three dimensions: (a) Structure, (b) Resources and Capabilities, and (c) Incentives. The Structure dimension refers to the mode of organization and set of relationships, as well as internal and external factors that affect the internal behavior of universities (García-Galván, 2013). The Resources and Capacities dimension are all the human, material, intellectual and intangible elements available to carry out UIC activities (Taboada, 2004; García-Galván, 2018). The third dimension, Incentives, refers to the retribution received by academic T-Rs for carrying out UIC (Antonelli, 2008); these include economic, social, professional or personal rewards. The Institutional section refers to the norms of UIC. This area is divided into two dimensions: (a) Formal Institutions and (b) Institutional Change. The Formal Institutions dimension refers to the inclusion of UIC in the curriculum, documentation, and statutes of university (North, 1990; Hodgson, 2007; Attard et al., 2021). The Institutional Change dimension refers to document management, as well as training, participation and T-Rs’ proposals for the improvement of norms and public policies related to UIC (North, 1990). Figure 1 shows the theoretical model with the domains and dimensions of T-Rs perception of scientific-technological collaboration between universities and industry.
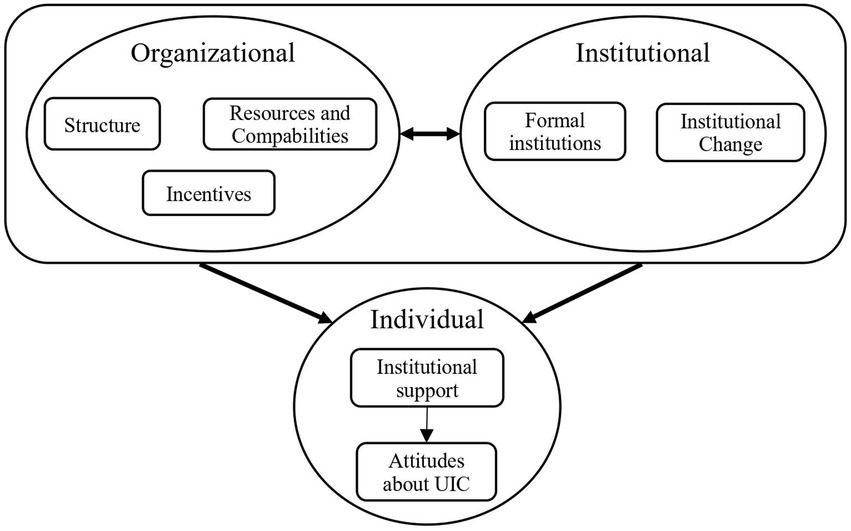
Figure 1. Theoretical model with the domains and dimensions of T-Rs perception of scientific-technological UIC.
According to Morales (2019), organizations can be considered as the operational base of institutions. Likewise, in the process of function of the organizations, they provide feedback to the institutions. For their part, the institutions support the normative bases of the organizations, while the organizations provide inputs for the subsequent development of the institutions. Consequently, if the organizational and institutional aspects are adequately addressed, providing the necessary support for the UIC activities, the TRs present a better attitude towards the UIC.
In particular, the CP-COOPTEC has six subscales to measure the attitude of researchers towards scientific-technological collaboration between universities and industry. The first subscale, which refers to the Cognitive component of the attitude towards UIC, measures the T-Rs’ thoughts about it (Rokeach, 1968). The second subscale, which refers to the Affective component, measures the T-Rs’ affect and beliefs (Rokeach, 1968). The third subscale, which refers to the Perception about institutional supports component, measure the perception of T-Rs about the interest of UIC (Casalet and Casas, 1998; Etzkowitz, 1998; Alcántara et al., 2006; Bajo, 2006; García-Galván, 2013; López-Leyva, 2014). The fourth subscale, which refers to the Perception of the infrastructure and management component, measures the aspects related to the performance of universities and research centers as organizations (Hodgson, 2006, 2007). The fifth subscale, which refers to the Perception of the value of contributions component, measures the benefits of carrying out UIC for universities and research centers, the productive sector and society in general (Antonelli, 2008). Finally, the sixth subscale, which refers to the Perception of the institutional framework component, measure the aspects related to the formal and informal norms that exist in universities and research centers with respect to the UIC (North, 1990; Aoki, 2007; Gandlgruber, 2007; Hodgson, 2007). Among the main advantages of the CP-COOPTEC we can mention: (a) it has evidence of validity of the design and content, based on theoretical foundation and expert judgment; (b) as well as some evidence of validity of the internal structure aspect; and (c) there are results of its application in qualitative and quantitative exploratory studies of UIC (Morales, 2019; Morales and Rodríguez, 2021; Pérez-Morán et al., 2021).
Based on the literature review, it can be said that there are few studies that measure the perception, motivation or attitude of T-Rs towards scientific-technological UIC. In this regard, Morales (2019) mentions that it is important to measure the attitude of T-Rs towards scientific-technological collaboration because it is a factor that affects the effectiveness and impact of knowledge transfer between universities and other sectors. Although, the six subscales of the (EA-COOPTEC) to measure the attitude of researchers towards scientific-technological collaboration between universities and the productive sector are considered a methodological advance within the theoretical approach of the ICI, it is necessary to obtain evidence of construct validity through more robust methods that adhere to the recommendations and guidelines of emblematic organizations and authors (Messick, 1989, 1995; American Educational Research Association, American Psychological Association, and National Council on Measurement in Education, 2014). Therefore, the purpose of this study is to analyze the psychometric properties of the EA-COOPTEC to measure the attitude of researchers for scientific-technological collaboration between university and industry, in order to obtain evidence of construct validity of the internal structure aspect and invariance, and thereby propose a valid and reliable revised version that can be used by other researchers to conduct predictive and comparative studies at the state level.
2. Methods
2.1. Participants
A total of 184 Teacher-Researchers (T-Rs) selected by non-probabilistic purposive sampling participated. The selection criteria for the participants in the study were that they had to (1) work as T-Rs in a higher education institution or research center in the state of Baja California, and (2) belong to the National System of Researchers (SNI, by acronyms in Spanish) of the National Council of Science and Technology (Conacyt, by acronyms in Spanish) of Mexico. After a database cleaning process, 179 cases were analyzed (129 [72%] men and 50 [28%] women). The sample of T-Rs participants represents 21.3% of the researchers in the SNI 2017 census in the state of Baja California (N = 841; CONACyT, 2017), that is, one-fifth of the population under study. Participants had a mean age of 36 to 40 years, 21 belonged to the age group 35 years or younger, 39 to the age group 36 to 40 years, 31 to the age group 41 to 45 years, 14 to the age group 46 to 50 years, and 74 were older than 50 years. It is important to mention that the gender distribution of the T-Rs participating in the study is relatively similar with the information at the national participation level in the SNI in Mexico reported by CONACyT (2021) in the General Report on the State of Science, Technology and Innovation. Mexico 2019. In 2013 the participation of women in the SNI in Mexico was 37.01% (6,869 of 18,555), in 2015 was 35.79% (8,346 of 23,316), in 2017 was 36.72% (9,982 of 27,186), and, in 2019 was 37.61% (11,489 of 30,548).
2.2. Instrument
For the purposes of the present study, the EA-COOPTEC designed by Morales (2019) and adjusted by Pérez-Morán et al. (2021) was used. This instrument measures the attitude of the subjects in relation to the linkage activities between UIC and is composed of six self-report scales of 46 items with Likert-type response options ranging from 1 (strongly disagree) to 4 (strongly agree). The six subscales are organized in three domains: Individual (subscale 1, 2, 3, and 5), Organizational (subscale 4), and Institutional (subscale 6). Previous studies (Morales and Rodríguez, 2021) reported an adequate overall Cronbach’s Alpha coefficient index (α = 0.92), and an overall Rho Alpha and McDonald Omega index with excellent values (overall ρ = 0.91, overall ω = 0.94, respectively) (see Table 1).
2.3. Procedure
An invitation was sent to their institutional e-mail address, in which they were informed about the general purpose of the study, their voluntary participation and the anonymity of their responses. A link to answer the CP-COOPTEC was also attached. If the T-Rs had any questions or concerns about the nature of the study, they had the possibility of contacting the investigators through the institutional e-mail address. From the data collected, a database cleaning was performed, following the recommendations of Hair et al. (2019), and Tabachnick and Fidell (2018). No missing data or erroneous values were found. Cases with outlier scores were identified and eliminated through a visual analysis of a box- and-whisker plot. Five outlier cases (overall index scores less than 95) were found, so the database was reduced to 179 cases (129 males and 50 females).
2.4. Data analysis
This section was organized in several stages. In the first stage, descriptive statistics were obtained, mean and standard deviation values were calculated, both for the items and for the general index of the EA-COOPTEC. Likewise, the assumptions of normality, linearity, sample adequacy and reliability were verified. Normality was analyzed by means of the Kolmogorov–Smirnov test with Lilliefors correction, as well as by obtaining the kurtosis and skewness values of the scores. Regarding the acceptance criteria, for the Kolmogorov–Smirnov test a value of less than 0.05 (p > 0.05) (Dallal and Wilkinson, 1986). Kurtosis and skewness had to be between the values of −1 to 1 (−1 < p > 1) according to the recommendations of Hair et al. (2019). For the calculation of reliability indices of the scale, Cronbach’s test (α) was used. Given that the usefulness of Cronbach’s Alpha test has been a matter of debate in recent decades (see McNeish, 2018; Raykov and Marcoulides, 2019), it was decided to accompany it with the standardized ordinal Rho’s Alpha (ρ) and McDonald’s Omega coefficient (ω). The cut-off criteria were α ≥ 0.70, ρ ≥ 0.70 (Hair et al., 2019) and ω ≥ 0.80 (Nájera-Catalán, 2019). For its part, for the sample adequacy measure, it was determined through Bartlett’s test of sphericity and the Kaiser-Meyer-Olkin coefficient (KMO). The cutoff criteria for acceptance were: a value of p ≤0.50 for Bartlett’s test, and a value ≥0.70 for the KMO coefficient (Hill, 2011; Hair et al., 2019).
In the second stage, a series of analyses were carried out to obtain evidence of construct validity of the internal structure aspect. For the dimensionality analysis, with the support of three specialists in the subject of UIC was proposed a four-factor model EFA was applied from a deductive approach. A new revised version of the instrument was adjusted and proposed, consisting of four factors: Community benefits (k = 15), Personal benefits (k = 13), Institutional responsibility (k = 14), and Regulation and normativity (k = 4). For this, a varimax extraction method was applied (Tabachnick and Fidell, 2018). Model fit was also evaluated according to the suggestions of Hu and Bentler (1999); given the sample size (N = <250), the fit indices and their criteria were: Comparative Fit Index (CFI) ≥ 0.90, Tucker-Lewis Index (TLI) ≥ 0.90, Standardized RMSR ≤0.08 and RMSEA ≤0.08. Because that the four-factor model proposed explains only 45% of the total variance, which is less than the recommendation by specialists (Tabachnick and Fidell, 2018) and did not show evidence of good fit (CFI = 0.74; TLI = 0.72; GFI = 0.70; NFI = 0.60; RMSEA = 0.08; SRMR = 0.08), it was decided to adjust the items by subscale: Community benefits (k = 10), Personal benefits (k = 7), Institutional responsibility (k = 9), and Regulation and normativity (k = 3). The option of eliminating problematic items was explored to improve the statistical fit of the model based on modification indices, standardized factor loadings, and error variances. Once a model that met the established fit criteria was obtained, the analysis of the internal structure of the scale was complemented with a maximum likelihood estimation model for the AFC following the suggestions of Hu and Bentler (1999) for the evaluation of model fit.
In the third stage, an MFCMG was applied to test the factorial invariance of the adjusted version of the scale (EA-COOPTEC v0.1) according to the gender and age variables. For the gender variable, the groups were divided into men and women. Likewise, for the age variable, two large age groups were considered: T-Rs under 45 years of age and T-Rs 45 years of age or older. For the measurement of invariance, the recommendations of Jöreskog and Sörbom (1979) and Vandenberg and Lance (2000) were followed. The four-factor model, product of the previous stage, was taken as a basis and a sequential constraint procedure for nested models was used. The criterion for acceptance of invariance was that the chi-square difference (Δχ2) between the nested models was not significant (p > 0.05). Because the calculation of χ2 is sensitive to sample size (Vandenberg and Lance, 2000; Cheung and Rensvold, 2002) the analysis was accompanied with CFI and RMSEA indices. The criteria for these indices were: a CFI difference (ΔCFI) between models less than −0.01 and an RMSEA close to or less than 0.08.
3. Results
3.1. Descriptive analysis
The mean score for the EA-COOPTEC v0.1 index was 85.98 (SD = 11.31). Also, the means for each of the subscales were 2.70 (SD = 0.53) for the first, 2.98 (SD = 0.41) for the second, 2.25 (SD = 0.51) for the third and 3.37 (SD = 0.0) for the fourth. The means of the item scores presented values ranging from 2.23 (SD = 0.84) to 3.55 (SD = 0.61). These results indicate that the majority of the T-Rs respondents answered the items by marking the response options Agree and Strongly agree. Regarding the internal consistency of the EA-COOPTEC v0.1, acceptable internal reliability indices were obtained. The overall Cronbach’s Alpha coefficient presented a value of 0.91. Likewise, the standardized ordinal Rho Alpha test obtained acceptable results (overall ρ global = 0.91). Similarly, the McDonald Omega index presented excellent values (ω global = 0.93). The results of the scale were acceptable, given that they presented scores ranging from moderate to excellent. Based on these results, the assumption of reliability of the internal structure of the overall scale is accepted (see Table 2).
The assumptions of normality and linearity were verified. As evidence of normality, low values of kurtosis (0.84) and skewness (−0.04) were obtained. In addition, the results of the Kolmogorov- Smirnov normality test with Lilliefors correction showed a value lower than the established criterion (p > 0.50, gl = 182), so it is considered that the data are normally distributed. For the linearity assumption, a Q-Q plot was visually evaluated and it was found that the data behave linearly (see Figure 2).
The results obtained indicate a good measure of sample adequacy in the EA-COOPTEC v0.1. Bartlett’s test of sphericity yielded a significant result (p < 0.00), suggesting the presence of sample adequacy among the variables. Likewise, the KMO coefficient presented an index greater than the cut-off criterion (KMO = 0.86). The above results allow the application of multivariate analysis, such as the EFA and CFA to obtain a theoretical model underlying the EA-COOPTEC v0.1 (Tabachnick and Fidell, 2018).
Moreover, the findings show high means for factors 1 (Community benefits), 2 (Personal benefits) and 4 (Regulation and normativity). This suggests that the T-R respondents have a positive perception of the Community and Personal benefits of engaging in UIC activities, as well as the importance of regulation and standardization of these activities. With respect to the results of factor 3 (Institutional responsibility), the mean for the response of the items presents the lowest value of all the factors (M = 2.23, SD = 0.84). This suggests that, in the universities and research centers where the subjects work: (a) there is little economic, material and human support to carry out UIC; (b) few training activities or courses are provided; and (c) the institutional plan does not include activities related to UIC.
On the other hand, the correlation coefficients between factors 1, 2 with factor 4 present positive and moderate values (r between 0.34 and 0.35), in particular, factors 1 and 2, which show a strong correlation coefficient (r = 0.56), suggesting that the perception of Community benefits and the perception of Personal benefits are closely related. However, factor 3 presents low correlation with factors 2 (r = 0.15) and 4 (r = −0.01) suggests that the support and interest perceived by universities and research centers does not have an impact on the motivation and perception of the Personal benefits of this activities, as well as the perception of the importance of generating and implementing manuals, protocols, public policies and peer reviews to improve UIC, respectively.
3.2. Factor structure
The EFA of the adjusted version of the four-factor model with 29 items of the EA-COOPTEC v0.1 explained 51% of the total variance. In general, the items presented good factor loadings. The standardized factor loadings for the revised model presented an acceptable correlation between items: for the first factor these ranged between 0.32 (Q_CB.03) and 0.68 (Q_CB.06); for the second, between 0.33 (Q_PB.03) and 0.53 (Q_PB.02); for the third, between 0.46 (Q_IR.04) and 0.61 (Q_IR.08); and for the fourth, between 0.47 (Q_RN.03) and 0.53 (Q_RN.01). The CFA of the adjusted version of the four-factor model met the criteria for adequate fit indices (χ2 = 558.08, gl = 366, p < 0.00, CFI = 0.92, TLI = 0.91, GFI = 0.82, NFI = 0.81, RMSEA = 0.05 [95% CI = 0.04, 0.06], SRMR = 0.06). Table 3 shows the comparison between the fit parameters of the revised four-factor model of the EA-COOPTEC with 46 items and the revised four-factor model of the EA-COOPTEC v0.1 with 29 items.

Table 3. Comparison between the fit parameters of the four-factor model of the EA-COOPTEC with 46 items and the revised four-factor model of the EA-COOPTEC v0.1 with 29 items.
The structure of the model is described as follows: the first factor, Community benefits of universities-industry collaboration, is made up of 10 items and describes the perception that T-Rs have about to the enhancement of the individual, social, developmental and linkage aspects that these activities bring to the region in which they take place. The second factor, Personal benefits, is made up of seven items and refers to the improvement of the academic and collaborative dimensions for the T-Rs, as well as a description of their motivation and stance towards the institutions involved in these activities. The third factor, Institutional responsibility, is made up of nine items that explain the support, training and regulatory planning obligations that have to generate UIC. Finally, the fourth factor, Regulation and normativity, is made up of three items that measure the documentation activities in universities and research centers, government management and the peer review process necessary for these activities. Table 4 shows the distribution of the domains and items of the adjusted scale (EA-COOPTEC v0.1).
3.3. Factorial invariance
An MFCMG was applied to examine the evidence of factorial invariance between T-Rs men and women in the four-factor model of the EA-COOPTEC v0.1. First, the factor structure of the model was evaluated to be the same for men and women; according to the results of the analysis, it was found to have a moderate fit according to the criteria established a-priori (χ2 = 1164.9; gl = 732; p < 0.00; CFI = 0.841; RMSEA = 0.081 [95% CI = 0.072, 0.090]). Similarly, the values obtained in the fit indices allow the acceptance of factorial invariance of the weak model (Δχ2 = 16.36; p = 0.90; ΔCFI = −0.003), of the strong model (Δχ2 = 31.28; p = 0.18; ΔCFI = −0.002) and of the strict model (Δχ2 = 22.872; p = 0.78; ΔCFI = −0.002). These results suggest that factor loadings, intercepts, and residuals are the same for both groups. The fit indices and values of the different nested models can be seen in Table 5.
We also examined the evidence of factorial invariance between T-Rs with an age equal to or less than 45 years and those older than 45 years. The factor structure of the model was the same for T-Rs with an age equal to or less than 45 years and those older than 45 years; according to the results of the analysis, the model was found to have a moderate fit according to the criteria established a-priori (χ2 = 1069.4; gl = 732; p < 0.00; CFI = 0.873; RMSEA = 0.072 [95% CI = 0.062, 0.081]). The values obtained in the fit indices allow the acceptance of factorial invariance of the weak model (Δχ2 = 29.966; p = 0.22; ΔCFI = −0.002), so it is considered that the factor loadings of the model are equal for both groups. As for the strong invariance model, the results do not provide evidence to support that the intercepts are equal for both groups (Δχ2 = 38.500; p = 0.041; ΔCFI = −0.005). The recommendations of Byrne et al. (1989) on parameter release for obtaining a partial invariance model were followed, so the intercept of item Q_PB.05 (“I sympathize with teacher-researchers engaged in universities-industry collaboration”) was released, which allowed us to accept a strong partial invariance model (Δχ2 = 32.887; p = 0.10; ΔCFI = −0.003). However, the values for the acceptance of the strict invariance model were not adequate (Δχ2 = 78.192; p = 3.5e-6; ΔCFI = −0.018) for acceptance.
4. Discussion
This study constitutes an advance in the field of measuring University-Industry Collaboration (UIC) from the perspective of researchers. By way of discussion, achievements and limitations are presented and contrasted with precedents concerning the study and measurement of UIC. First, it can be said that the psychometric properties of the EA-COOPTEC v0.1 meet the quality criteria of linearity, normality and reliability, as well as sample adequacy and construct validity of the internal structure and invariance for gender and age. In particular, based on the results of the EFA and the recommendations of UIC specialists, it can be argued that the six-factor version of the EA-COOPTEC is not the best organization of the items and therefore of representing the construct. For it, an inductive-deductive approach analysis was conducted in order to find an optimal model solution. As a result, a revised four-factor model was proposed that demonstrated adequate goodness-of-fit for the sample of T-R participants. With this, it is confirmed that the attitude of researchers for scientific-technological collaboration between universities and industry underlying the EA-COOPTEC v0.1 is a multidimensional construct, this finding is consistent with how several scholars conceptualize it (Etzkowitz, 1998; Etzkowitz et al., 2000; Bodas et al., 2013; Wanda, 2015).
Compared to other studies (López-Martínez et al., 2007; Boardman and Ponomariov, 2009; Giuliani et al., 2010; Morales, 2019; Cudic et al., 2021; Da Silva and Sartori, 2022), only López-Martínez et al. (2007) and Morales (2019) applied instruments similar to the EA-COOPTEC to measure UIC from the researchers’ perspective. However, López-Martínez et al. (2007) reported no evidence of validity or reliability. For his part, Morales (2019) obtained evidence of validity of the design and content of the instrument used, as well as some evidence of validity of the internal structure aspect; in particular, he reported a Cronbach’s Alpha of 0.92. In this sense, the results of the internal consistency analyses of the improved version of the EA-COOPTEC v0.1 of the present study (Cronbach’s Alpha = 0.91, ordinal Rho Alpha test = 0.91 and McDonald’s Omega index = 0.94) coincide relatively with that reported by Morales (2019). Despite this, it is important to highlight that there are few studies with which the results of the present study can be compared, especially where the measurement of a similar construct is addressed and evidence of internal structure and invariance is shown.
Second, the results support the evidence of factorial invariance on configurational, strong and strict models for gender and factorial invariance on configurational and strong models (with the exception of item Q_PB.05 “I sympathize with teacher-researchers engaged in universities-industry collaboration”) for the age in a sample of Mexicans T-Rs. Thus, indicating that participants conceptualize the universities-industry collaboration construct in the same way regardless of gender or age. Therefore, valid comparisons of mean scores of the EA-COOPTEC v0.1 subjects can be made, and it is not necessary to use normative scores for different groups according to gender or age. Also, it is hypothesized that these results may be different in a sample of T-Rs belonging to universities and research centers with a greater focus on research and development activities than those focused on teaching.
Regarding gender and UIC, no studies were found that apply methods for obtaining evidence of factorial invariance between genders; when this relationship between variables is addressed, it is commonly assessed whether there is a difference between men and women in the patterns of participation in these activities (Bozeman et al., 2013; Tartari and Salter, 2015). The same is true with respect to the age variable, no studies of invariance as a function of age were found; likewise, most of these investigations operationalize age differently (Bozeman et al., 2013). For example, Boardman and Ponomariov (2009) and Giuliani et al. (2010) conducted studies to explore UIC or make comparisons between different groups of participants. Boardman and Ponomariov (2009) drew the data for their analyzes from a survey of US university scientists. The dependent variables analyzed were nine and the explanatory variables were 15, including age and sex. In their findings, they report that male T-Rs carry out more activities related to the UIC. In particular, these authors mention that women are hired in the academic world in increasing numbers only recently. Likewise, they identified that the activities related to the UIC in which to be an older T-R is relevant are the generation of patents and the co-authorship of research articles with industry personnel. Giuliani et al. (2010) collected their data through a survey applied in person. This survey included aspects related to the background of the researcher and his personal collaborations with other academic researchers and with the industry. Based on their results, they reported that women are more likely than male T-Rs to carry out UIC-related activities. Also, in their study they found that younger T-Rs are more likely to form U-I bonds than their older colleagues. However, none of the mentioned studies reported evidence of confiability, validity and factorial invariance of their instruments.
Although this study constitutes a contribution to the measurement of scientific-technological cooperation between university and industry, some limitations should be considered: first, since the EA-COOPTEC v0.1 is a self-report scale, it is necessary to conduct social desirability studies of it; second, although the sample represents 21. 3% of the researchers in the 2017 SNI census in the state of Baja California (N = 841; CONACyT, 2017), it was taken from a specific geographic area, which implies that generalization of the findings to other regions or at the national level should be done with caution; third, the participation of women in the study is relatively similar, but lower than the trend in 2019 (37.61%) at the national level of participation in the SNI in Mexico reported by CONACyT (2021) in the latest General Report on the State of Science, Technology and Innovation. Mexico 2019; fourth, the factor Regulation and Normality, is composed of three items barely meeting the minimum acceptable range of items (Streiner, 1994); fifth, no information was collected from other indicators used to measure different types of UIC activities (Gardner et al., 2010; Seppo and Lilles, 2012) that provide evidence of concurrent, discriminant and predictive validity; and sixth, the criterion of strict invariance as a function of age is not met.
5. Conclusion
In conclusion, the EA-COOPTEC v0.1 presents adequate psychometric properties of reliability and internal structure aspect validity to measure the attitude and perception of T-Rs towards University-Industry Collaboration (UIC). The results support the hypothesis of the multifactorial nature of the measurement of T-Rs’ attitude towards UIC. From the theoretical point of view, with the four-factor model of the instrument (Community Benefits, Personal Benefits, Institutional Responsibility, and Regulation and Normativity), it contributes to the delimitation of the construct supported by Integrated Contemporary Institutionalism (ICI; Taboada, 2004; García-Galván, 2008), which provides a theoretical approach for the analysis of UIC from the T-Rs’ perspective.
Also, evidence of factorial invariance indicates that participants conceptualize the construct in the same way regardless of gender or age. The results support evidence of factorial invariance for the configurational, strong and strict models for the gender variable and factorial invariance for the configurational, strong partial models for the age variable, indicating that participants conceptualize the construct in the same way regardless of gender or age. This is particularly valuable in the study of UIC, since there are no scales designed for its exploration from the T-Rs’ perspective that report this type of measurement invariance and with it the possibility of conducting comparative studies.
Finally, the results of this study contribute to the creation of robust indicators for the measurement of scientific and technological cooperation between university and industry from the T-Rs’ perspective. The EA-COOPTEC v0. 1 is the only instrument at present that has evidence of reliability, validity, and invariance at the state level in Mexico, and that has a robust theoretical frame of reference from which to analyze and predict UIC outcomes: increasing competitiveness among industry (Burrone, 2005), promoting theoretical and methodological advancement in the field of science, fostering the capitalization of knowledge (Etzkowitz, 1998, 2003), and turning universities and research centers into an important actor for economic development (García-Galván, 2018).
For future research, it is recommended: (a) to conduct social desirability studies of the EA-COOPTEC v0. 1; (b) expand the sample to be representative by age strata and other variables of interest so that the results can be generalized to other states, at the national level, and in other countries in the region with a similar science and technology system; (c) expand the sample of participating female researchers to reduce possible biases when comparing with the male sample; (d) increase, based on the recommendations of UIC specialists and solid theoretical foundations, the number of items of the Regulation and Normality factor so that the construct faithfully represents the reality to be measured; (e) accompany the application of the EA-COOPTEC v0. 1 with the measurement of other variables in order to provide evidence of concurrent, discriminant and predictive validity, especially those variables associated with institutional factors, human factors, linkage factors, framework factors, intangible product indicators and tangible product indicators (Cudic et al., 2021); and (f) Conduct a study based on the Bayesian Mindsponge Framework (BMF) to consolidate the conceptualization, construction and fit the theoretical model underlying EA-COOPTEC v0. 1 and improve estimation with small sample sizes used for the study of UIC in science and technology systems worldwide (Nguyen et al., 2022).
Data availability statement
The raw data supporting the conclusions of this article will be made available by the authors, without undue reservation.
Ethics statement
The study procedure was supervised and approved by a committee of researchers from the Autonomous University of Baja California. For the application of the questionnaire, the voluntary participation of research professors was requested, who were previously informed about the objectives and procedures of the study and were informed of the confidentiality, safeguarding, and use of the results for research purposes. The application of the survey did not represent any risk to the physical or occupational integrity of the participants.
Author contributions
JP-M contributed to the idea of research, its conceptualization, implementation, and methodology. He was in charge of writing the manuscript. He also contributed to the analysis and interpretation of data, and to the revision of the English version and writing in Frontiers format. MM-P contributed to implementation of the methodology. He also contributed to the analysis and interpretation of data, and to the revision of the first version and writing in Frontiers format. BB-B directed the analysis and interpretation of data, contributed to the conceptualization of the research, and to the writing of the manuscript. He was also in charge of the revision of the English version and the writing in Frontiers format. JC-G collaborated in the analysis and interpretation of data, supported the search for additional bibliographic information, and reviewed the style of the article. All authors contributed to the article and approved the submitted version.
Acknowledgments
A special acknowledgment to the Teachers–Researchers (T-Rs) who participated in the project, and our thanks to professor Rodolfo García Galván for his support and advice in the area of Institutionalism and Technoscience.
Conflict of interest
The authors declare that the research was conducted in the absence of any commercial or financial relationships that could be construed as a potential conflict of interest.
Publisher’s note
All claims expressed in this article are solely those of the authors and do not necessarily represent those of their affiliated organizations, or those of the publisher, the editors and the reviewers. Any product that may be evaluated in this article, or claim that may be made by its manufacturer, is not guaranteed or endorsed by the publisher.
References
Agrawal, A. (2001). University to industry knowledge transfer: literature review and unanswered questions. Int. J. Manag. Rev. 3, 285–302. doi: 10.1111/1468-2370.00069
Alcántara, V. M., Arcos, J. L., and Mungaray, A. (2006). Vinculación y posicionamiento de la Universidad Autónoma de Baja California con su entorno social y productivo [linkage and positioning of the Autonomous University of Baja California with its social and productive environment]. Baja California: Universidad Autónoma de Baja California/Asociación Nacional de Universidades e Instituciones de Educación Superior.
American Educational Research Association, American Psychological Association, and National Council on Measurement in Education (2014). Standards for educational and psychological testing. Washington: American Educational Research Association.
Ankrah, S., and AL-Tabbaa, O. (2015). Universities-industry collaboration: a systematic review. Scand. J. Manag. 31, 387–408. doi: 10.1016/j.scaman.2015.02.003
Antonelli, C. (2008). The new economics of the university: a knowledge governance approach. J. Technol. Transfer. 33, 1–22. doi: 10.1007/s10961-007-9064-9
Aoki, M. (2007). Endogenizing institutions and institutional changes. J. Inst. Econ. 3, 1–31. doi: 10.1017/S1744137406000531
Attard, C., Berger, N., and Mackenzie, E. (2021). The positive influence of inquiry-based learning teacher professional learning and industry partnerships on student engagement with STEM. Front. Educ. 6:693221. doi: 10.3389/feduc.2021.693221
Bajo, A. (2006). Vinculación e innovación en la región noroeste de México. Culiacán: Universidad Autónoma de Sinaloa.
Boardman, P. C., and Ponomariov, B. L. (2009). University researchers working with private companies. Technovation 29, 142–153. doi: 10.1016/j.technovation.2008.03.008
Bodas, I. M., Argou, R., and Mirra, E. (2013). University–industry collaboration and innovation in emergent and mature industries in new industrialized countries. Res. Policy 42, 443–453. doi: 10.1016/j.respol.2012.06.006
Bozeman, B., Fay, D., and Slade, C. (2013). Research collaboration in universities and academic entrepreneurship: the-state-of-the-art. J. Technol. Transfer. 38, 1–67. doi: 10.1007/s10961-012-9281-8
Burrone, E. (2005). Intellectual property rights and innovation in SMEs in OECD countries. J. Intellect. Prop. Rights 10, 34–43.
Byrne, B., Shavelson, R., and Muthén, B. (1989). Testing for the equivalence of factor covariance and mean structures: the issue of partial measurement invariance. Psychol. Bull. 105, 456–466. doi: 10.1037/0033-2909.105.3.456
Casalet, M., and Casas, R. (1998). Un diagnóstico sobre la vinculación Universidad-Empresa [A diagnosis of University-Business linkage]. Baja California: Asociación Nacional de Universidades e Instituciones de Educación Superior.
Cheung, G. W., and Rensvold, R. B. (2002). Evaluating goodness-of-fit indexes for testing measurement invariance. Struct. Equ. Model. 9, 233–255. doi: 10.1207/S15328007SEM0902_5
CONACyT (2017). Informe general del estado de la ciencia, la tecnología y la innovación. México, 2017 [General report on the state of science, technology and innovation. Mexico, 2017]. Available at: https://www.siicyt.gob.mx/index.php/estadisticas/informe-general/informe-general-2017/4813-informe-general-2017/file. (Accessed March 8, 2022).
CONACyT (2021). Informe general del estado de la ciencia, la tecnología y la innovación. México, 2019 [General report on the state of science, technology and innovation. Mexico, 2019]. Available at: https://www.siicyt.gob.mx/index.php/estadisticas/informe-general/informe-general-2019/4948-informe-general-2019/file. (Accessed March 8, 2022).
Cudic, B., Alešnik, P., and Hazemali, D. (2021). Factors impacting university–industry collaboration in European countries. J. Innov. Entrepreneursh. 11, 1–24. doi: 10.1186/s13731-022-00226-3
Da Silva, J. A., and Sartori, R. (2022). Motivations and barriers of university-industry cooperation: a comparison between Brazil and Ireland. J. Technol. Manag. Innov. 17, 49–58. doi: 10.4067/S0718-27242022000200049
Dallal, G., and Wilkinson, L. (1986). An analytic approximation to the distribution of lilliefors’s test statistic for normality. Am. Stat. 40, 294–296. doi: 10.1080/00031305.1986.10475419
Edquist, C. (1997). Systems of innovation. Technologies, institutions and organizations. London: Routledge.
Etzkowitz, H. (1998). The norms of entrepreneurial science: cognitive effects of the new university-industry linkages. Res. Policy 27, 823–833. doi: 10.1016/S0048-7333(98)00093-6
Etzkowitz, H. (2003). Innovation in innovation: the triple helix of university–industry–government relations. Soc. Sci. Inf. 42, 293–337. doi: 10.1177/05390184030423002
Etzkowitz, H., and Leydesdorff, L. (1995). The triple helix - university-industry-government relations: a Laboratory for Knowledge Based Economic Development. EASST Rev. 14, 14–19.
Etzkowitz, H., Webster, A., Gebhardt, C., and Cantisano, B. R. (2000). The future of the university and the university of the future: evolution of ivory tower to entrepreneurial paradigm. Res. Policy 29, 313–330. doi: 10.1016/S0048-7333(99)00069-4
Freeman, C. (1995). The “National System of innovation” in historical perspective. Camb. J. Econ. 19, 5–24. doi: 10.1093/oxfordjournals.cje.a035309
Gandlgruber, B. (2007). Coordinación, instituciones y empresas [Coordination, institutions and companies]. [doctoral thesis]. [Ciudad de Mexico]: Universidad Autónoma Metropolitana.
García-Galván, R. (2008). Análisis teórico de la transferencia de conocimientos universidad-empresa mediante la colaboración [theoretical analysis of university-industry knowledge transfer through collaboration]. Revista Economía: Teoría y práctica 29, 51–86. doi: 10.24275/ETYPUAM/NE/292008/Garcia
García-Galván, R. (2011). Revisión de los elementos teórico-conceptuales en torno a la cooperación interfirma e interorganizacional [review of the theoretical and conceptual elements of inter-firm and inter-organizational cooperation]. Análisis Económico 26, 185–208.
García-Galván, R. (2013). “¿El conocimiento universitario puede promover el desarrollo industrial? Percepción sobre las promesas de la biotecnología en México [Can university knowledge promote industrial development? Perception of the promise of biotechnology in Mexico]” in Conocimiento para el crecimiento económico [Knowledge for economic growth] cords. eds. E. Gaona, D. Velázquez, and J. S. Hernández (Pachuca, Hidalgo: Universidad Autónoma del Estado de Hidalgo), 87–103.
García-Galván, R. (2018). Cooperación tecnocientífica universidad-empresa e interfirma: Análisis teórico integrado desde la perspectiva de la economía institucional contemporánea [university-industry and interfirm technoscientific cooperation: integrated theoretical analysis from the perspective of contemporary institutional economics]. Ciencia y Universidad. Revista de economía 37, 5–30.
Gardner, P. L., Fong, A. Y., and Huang, R. L. (2010). Measuring the impact of knowledge transfer from public research organisations: a comparison of metrics used around the world. Int. J. Learn. Intellect. Cap. 7, 318–327. doi: 10.1504/IJLIC.2010.034371
Gibbons, M., Limoges, C., Nowotny, H., Schwartzman, S., Scott, P., and Trow, M. (1997). La nueva producción del conocimiento [the new production of knowledge]. Barcelona: Ediciones Pomares-Corredor.
Giuliani, E., Morrison, A., Pietrobelli, C., and Rabellotti, R. (2010). Who are the researchers that are collaborating with industry? An analysis of the wine sectors in Chile, South Africa and Italy. Res. Policy 39, 748–761. doi: 10.1016/j.respol.2010.03.007
Hair, J., Black, W., Babin, B., and Anderson, R. (2019). Multivariate Data Analysis. Boston: Cengage Learning.
Hill, B. D. (2011). The sequential Kaiser-Meyer-Olkin procedure as an alternative for determining the number of factors in common-factor analysis: A Monte Carlo simulation. [doctoral thesis]. [Oklahoma]: Oklahoma State University.
Hodgson, G. M. (2006). What are institutions? J. Econ. Issues 40, 1–25. doi: 10.1080/00213624.2006.11506879
Hodgson, G. M. (2007). Economía institucional y evolutiva [institutional and evolutionary economics]. Mexico city Universidad Autónoma Metropolitana.
Hu, L. T., and Bentler, P. M. (1999). Cutoff criteria for fit indexes in covariance structure analysis: conventional criteria versus new alternatives. Struct. Equ. Model. 6, 1–55. doi: 10.1080/10705519909540118
Jöreskog, K. G., and Sörbom, D. (1979). Advances in factor analysis and structural equation models. Washington: ABT Books.
Leydesdorff, L., and Etzkowitz, H. (1996). Emergence of a triple helix of university—industry—government relations. Sci. Public Policy 23, 279–286. doi: 10.1093/spp/23.5.279
López-Leyva, S. (2014). La vinculación de la ciencia y la tecnología con el sector productivo: Una perspectiva económica y social [linking science and technology with the productive sector: An economic and social perspective]. Culiacán: Universidad Autónoma de Sinaloa.
López-Martínez, R. E., Medellín, E. A., Scanlon, A. P., and Solleiro, J. L. (2007). Motivations and obstacles to university industry cooperation (UIC): a Mexican case. R&D Manag. 24, 017–030. doi: 10.1111/j.1467-9310.1994.tb00844.x
Lundvall, B. A. (1992). “National Systems of innovation: towards a theory of innovation and interactive learning” in The learning economy and the economics of Hope. ed. B. A. Lundvall (London: Anthem Press), 85–106.
Lundvall, B. A. (2004). The economics of knowledge and learning. Res. Technol. Innov. Manag. Policy 8, 21–42. doi: 10.1016/S0737-1071(04)08002-3
McNeish, D. (2018). Thanks coefficient alpha, we’ll take it from here. Psychol. Methods 23, 412–433. doi: 10.1037/met0000144
Messick, S. (1989). “Validity” in Educational measurement. ed. R. L. Linn (New York: American Council on education/Macmillan), 13–103.
Messick, S. (1995). Validity of psychological assessment: validation of inferences from persons’ responses and performances as scientific inquiry into score meaning. Am. Psychol. 50, 741–749. doi: 10.1037/0003-066X.50.9.741
Morales, M. (2019). Cooperación tecnocientífica IES-sector Productivo desde la perspectiva del cambio institucional. Evidencias de Baja California Technoscientific cooperation between HEI and the productive sector from the perspective of institutional change. Evidence from Baja California]. doctoral thesis [Ensenada (Baja California)]: Universidad Autónoma de Baja California.
Morales, M., and García-Galván, R. (2019). La colaboración universidad-empresa: una perspectiva teórica y política para México [University-industry collaboration: a theoretical and policy perspective for Mexico]. Revista Educación y Desarrollo 51, 77–88.
Morales, M., and Rodríguez, J. C. (2021). “Percepción de los Investigadores de Baja California sobre la Cooperación Tecnocientífica que se realiza en las Instituciones de Educación Superior [Perception of Baja California Researchers on the Technoscientific Cooperation carried out in Higher Education Institutions],” in Cooperación de las organizaciones del conocimiento con el entorno productivo y social de Baja California [cooperation of knowledge organizations with the productive and social environment of Baja California], coords. R. García-Galván, J. C. Rodríguez, and A. A. Chaparro (Baja California Qartuppi), 28–48.
Muscio, A., and Vallanti, G. (2014). Perceived obstacles to university–industry collaboration: results from a qualitative survey of Italian academic departments. Ind. Innov. 21, 410–429. doi: 10.1080/13662716.2014.969935
Nájera-Catalán, H. E. (2019). Reliability, population classification and weighting in multidimensional poverty measurement: a Monte Carlo study. Soc. Indic. Res. 142, 887–910. doi: 10.1007/s11205-018-1950-z
Nguyen, M. H., La, V. P., Le, T. T., and Vuong, Q. H. (2022). Introduction to Bayesian Mindsponge framework analytics: an innovative method for social and psychological research. MethodsX 9:101808. doi: 10.1016/j.mex.2022.101808
North, D. (1990). Institutions, institutional change and economic performance. Cambridge: Cambridge University Press.
Pérez-Morán, J. C., Morales, M., and Bernal-Baldenebro, B. (2021). Reporte técnico: Propiedades psicométricas de un cuestionario para medir cooperación tecno-científica entre universidades y sector productivo [Technical report: Psychometric properties of a questionnaire to measure techno-scientific cooperation between universities and the productive sector]. Red Impulsora en Metodología de Evaluación Diagnóstica e Innovación Educativa (mimeo).
Raykov, T., and Marcoulides, G. (2019). Thanks coefficient alpha, we still need you! Educ. Psychol. Meas. 79, 200–210. doi: 10.1177/0013164417725127
Rokeach, M. (1968). A theory of organization and change within value-attitude systems. J. Soc. Issues 24, 13–33. doi: 10.1111/j.1540-4560.1968.tb01466.x
Santoro, M. D. (2000). Success breeds success: the linkage between relationship intensity and tangible outcomes in industry–university collaborative ventures. J. High Technol. Ranage. Res. 11, 255–273. doi: 10.1016/S1047-8310(00)00032-8
Santoro, M. D., and Chakrabarti, A. K. (2002). Firm size and technology centrality in industry–university interactions. Res. Policy 31, 1163–1180. doi: 10.1016/S0048-7333(01)00190-1
Seppo, M., and Lilles, A. (2012). Indicators measuring university-industry cooperation. Discuss. Estonian Econ. Policy 20:1.
Smit, R., Robin, N., and De Toffol, C. (2020). Explaining secondary students’ career intentions for technology and engineering jobs using an expectancy-value model. Front. Educ. 5:39. doi: 10.3389/feduc.2020.00039
Streiner, D. L. (1994). Figuring out factors: the use and misuse of factor analysis. Can. J. Psychiatry 39, 135–140. doi: 10.1177/070674379403900303
Taboada, E. L. (2004). ¿Qué hay detrás de la decisión de cooperar tecnológicamente? Propuesta teórica integradora Para explicar la cooperación tecnológica inter-firma [what is behind the decision to cooperate technologically? An integrative theoretical proposal to explain inter-firm technological cooperation]. [doctoral thesis]. [Ciudad de Mexico]: Universidad Autónoma Metropolitana.
Tartari, V., and Salter, A. (2015). The engagement gap: exploring gender differences in university-industry collaboration activities. Res. Policy 44, 1176–1191. doi: 10.1016/j.respol.2015.01.014
Teece, D. J., Pisano, G., and Shuen, A. (1998). Dynamic capabilities and strategic management. Strateg. Manag. J. 18, 509–533. doi: 10.1002/(SICI)1097-0266(199708)18:7%3C509::AID-SMJ882%3E3.0.CO;2-Z
Vandenberg, R. J., and Lance, C. E. (2000). A review and synthesis of the measurement invariance literature: suggestions, practices, and recommendations for organizational research. Organ. Res. Methods 3, 4–70. doi: 10.1177/2F109442810031002
Vertova, G. (2014). The state and National Systems of innovation: A sympathetic critique. Levy economics institute, Working Paper No. 823. University of Bergamo.
Keywords: universities-industry collaboration, higher eduacation, academic teachers-researchers, invariance, confirmatory factor analysis
Citation: Pérez-Morán JC, Morales-Páez M, Bernal-Baldenebro B and Cano-Gutiérrez JC (2023) Psychometric properties and invariance of the scale to measure attitude of researchers for university-industry collaboration. Front. Educ. 8:971367. doi: 10.3389/feduc.2023.971367
Edited by:
Minh-Hoang Nguyen, Phenikaa University, VietnamReviewed by:
Amina Muazzam, Lahore College for Women University, PakistanSyed Rizwan Ali, Bahria University, Pakistan
Tri Le, Ritsumeikan Asia Pacific University, Japan
Copyright © 2023 Pérez-Morán, Morales-Páez, Bernal-Baldenebro and Cano-Gutiérrez. This is an open-access article distributed under the terms of the Creative Commons Attribution License (CC BY). The use, distribution or reproduction in other forums is permitted, provided the original author(s) and the copyright owner(s) are credited and that the original publication in this journal is cited, in accordance with accepted academic practice. No use, distribution or reproduction is permitted which does not comply with these terms.
*Correspondence: Julio César Cano-Gutiérrez, jcano@uabc.edu.mx