- Learning and Teaching with Media, Institute of Education, Universität der Bundeswehr München, Neubiberg, Germany
Virtual Reality (VR) and photogrammetry are emerging technologies that facilitate and shape the ongoing digital transformation of education. VR offers new opportunities for creating immersive and interactive educational experiences. Photogrammetry enables new ways to create lifelike educational virtual environments and is becoming an alternative to manual 3D modeling with graphics software. The manner in which VR affects the authenticity of educational experiences has been addressed in previous educational and psychological research. Empirical papers have so far focused on the authenticity of educational VR environments created by 3D modeling. However, little is known about the authenticity of educational VR environments developed with photogrammetry. Given that VR provides rich multi-sensory experiences and interests can be stimulated by engaging contexts, educational VR environments also possess great potential to support interest development. What is still unknown regarding this topic are the beneficial characteristics of VR environments and the individual variables required to trigger and explain interest development. Consequently, we conducted an experiment following up on the mentioned authenticity and interest research questions in the context of higher education. A two-group between-subjects design was used and N = 64 educational science and psychology university students gathered information about a railroad bridge wearing a head-mounted display (HMD). The control group encountered an educational virtual environment created with 3D modeling. The intervention group was presented with the same educational virtual environment but the main object of the railroad bridge was generated by photogrammetry. Situational interest was measured in the pretest and the posttest; authenticity-related variables (i.e., presence and representation fidelity) were assessed in the posttest. Concerning authenticity, there were no significant group differences. Photogrammetry might thus not affect authenticity in educational contexts in which participants focus on gathering information. Regarding interest development, there were two main findings. First, interest in VR for learning increased from pretest to posttest, supporting that interest can be induced in VR. Second, a large share of posttest interest was explained by presence and pretest interest, highlighting the importance of these variables.
1. Introduction
Virtual Reality (VR) and photogrammetry are new technologies that will have a major impact on digital education in the coming years. In the following, we provide a brief introduction to these new technologies and highlight shortly their importance for education.
Virtual Reality (VR) refers to computer simulations and specific hardware that together convey a strong feeling of being physically present within an interactive digital environment (Radianti et al., 2020). Recently, head-mounted displays (HMDs) have become increasingly popular as VR hardware. HMDs are goggles that enable an immersive experience with sharp displays and track users’ movements in the physical world to project them into a digital space. Some HMDs require a connection to a computer to operate, while others can be used as a standalone device. Unlike other immersive VR devices, such as CAVE systems – dedicated rooms consisting of multiple screens and motion tracking systems – HMDs require less space and do not have to be permanently installed (Jensen and Konradsen, 2018). As this advantage is crucial for many educational settings, such as bringing VR to regular classrooms, this paper will focus on HMDs as VR devices.
Photogrammetry records real-world objects with light-wave based and other sensors for survey and evaluation purposes (American Society for Photogrammetry and Remote Sensing, 2022). As photogrammetry can be used to create digital models of real-world objects and landscapes (Historic England, 2017), it is becoming an alternative to 3D modeling for creating educational virtual environments. In 3D modeling, a designer creates objects manually using computer graphics software in a time-consuming process requiring extensive expertise (Erolin, 2019).
VR and photogrammetry are currently becoming more accessible as the required software and hardware become more affordable (Nebel et al., 2020; Pellas et al., 2020). Likewise, both technologies are also becoming easier to use: VR engines and asset stores frequently contain templates that facilitate scene set-up and implement basic user navigation. Photogrammetry software often includes detailed documentation and automated workflows, which foster the swift creation of 3D objects. As a result of the improved accessibility and higher convenience, an increased number of educational researchers and practitioners can make use of VR and photogrammetry to deliver or create content (Jensen and Konradsen, 2018; Nebel et al., 2020).
It is an open question whether innovative educational VR environments created by photogrammetry are perceived as more authentic than traditional educational VR environments created by 3D modeling. On the one hand, photogrammetry may raise environmental fidelity, that is, the “degree to which variables in the training environment resemble those in the real world” (Waller et al., 1998, p. 130), by providing photorealistic models. On the other hand, perceived authenticity may also depend on other factors, such as the quality of the interaction and the realism of the provided task (Witmer and Singer, 1998).
Given that educational VR environments provide rich multi-sensory experiences and interests can be stimulated by engaging contexts (Hidi and Renninger, 2006), VR can also be used to support interest development. Some empirical studies have been conducted on interest development in educational VR environments (e.g., Makransky et al., 2020; Petersen et al., 2020). Yet, much remains unknown about how interest is triggered by educational VR environments and which individual variables, such as pretest interest, and presence, contribute to this process.
At the beginning of this section, we will elaborate on creating content for educational VR environments with 3D modeling and photogrammetry. We will then address the mentioned research questions on authenticity and interest development.
1.1. Creating content for educational virtual reality environments with 3D modeling and photogrammetry
In the past, the creation of educational VR content was almost exclusively carried out using 3D modeling. In 3D modeling, designers perform tasks such as creating meshes, applying textures, adjusting light settings, and building animations using computer graphics software (such as Blender or Maya). This process is rather time-consuming and requires extensive expertise (Erolin, 2019). Content for VR applications is produced with this method usually by 3D designers who have completed professional training or education in the field of graphic design. With the wider distribution and better availability of photogrammetry software and hardware, the creation of VR content can now also be carried out with photogrammetry. Photogrammetric methods can use various sensors including photo cameras, drone cameras, laser-scanning, and geo-references (American Society for Photogrammetry and Remote Sensing, 2022). Data from one of these or multiple sources is then processed to a coherent 3D model relatively automatically by algorithms comparing the correspondence between the records (Historic England, 2017). Photogrammetry software, such as RealityCapture or AutoDesk, includes automated workflows and requires the user in easier projects to mainly clean and simplify the model until it fits the project’s requirements (Trebuňa et al., 2020). Content for VR applications can thus be more easily created using this method by users without specific professional training or prior education (Erolin, 2019).
Both types of content creation can be used to produce educational VR environments including single objects, buildings, virtual humans, and even full terrains. However, educational VR environments are currently mainly created with 3D modeling. Researchers and designers acquire suitable 3D objects created by others from asset stores or create these objects with a modeling software such as blender. Soon, photogrammetry will likely be used increasingly to develop models for educational VR environments. Asset stores will then include more objects created with photogrammetry, and researchers and designers will become capable of producing photogrammetric 3D models themselves. As mentioned, there are several differences between developing models for educational VR environments with 3D modeling and photogrammetry. Table 1 lists and contrasts these differences between both content creation methods that concern the creation process, as well as the structure, texture, lighting, and level of detail of the created 3D model.
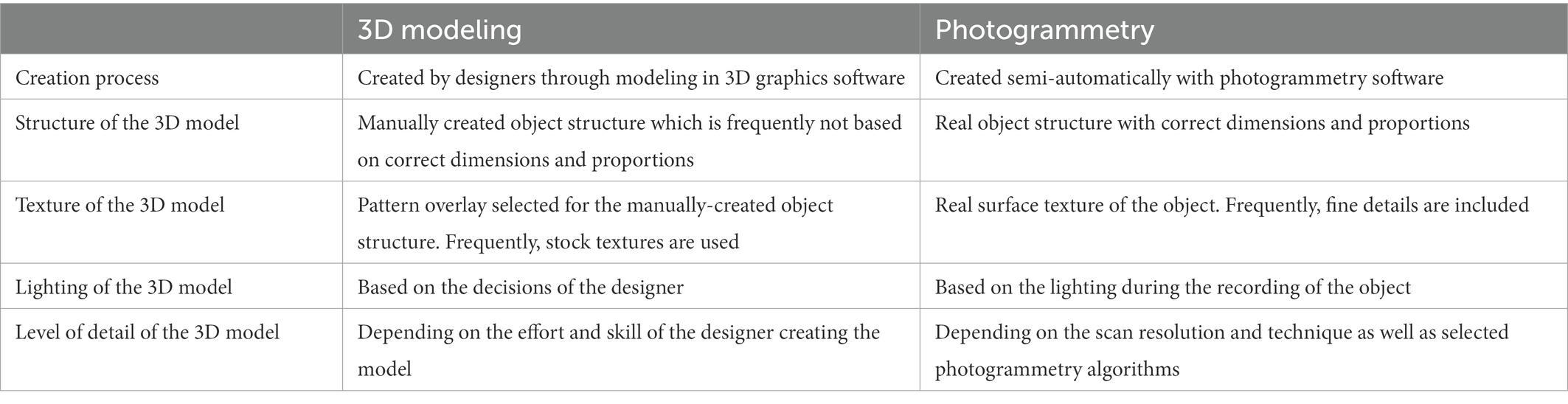
Table 1. Differences between creating educational virtual environments with 3D modeling and photogrammetry.
Despite its great potential, research on education in VR using the new technology of photogrammetry is still lacking (Nebel et al., 2020). Thus, this paper sets out to compare educational environments created with 3D modeling and photogrammetry. In doing this, the paper focuses on photogrammetry with a hybrid modeling approach that combines records from photos and laser-scanning (Historic England, 2017). Laser-scanning is a method in which a lidar generates multiple point clouds (i.e., x, y, z coordinates and other characteristics such as color) of a structure (Historic England, 2018). Following the mentioned hybrid modeling approach, the photogrammetry software then imports the photographs and laser scans and generates a coherent 3D model from them. The hybrid modeling approach was selected because it is particularly suitable for capturing large objects, and our study’s educational environment included a large railroad bridge as the main object and center of attention.
1.2. Increasing the authenticity of experiences through virtual reality
One goal that VR has had since its inception is undoubtedly to increase the authenticity of experiences. A classical example of one of the first VR systems illustrates this goal. The Sensorama created by VR pioneer Morton Heilig in 1961 was a VR movie theater that stimulated multiple senses not normally considered when watching movies (McLellan, 2008). Users were surrounded by displays and experienced smell and motion in realistic situations such as during helicopter flights (McLellan, 2008). This example shows that VR technology uses both software and hardware together to enhance authenticity. On the software side, authenticity is pursued in the realms of education by providing interactive, realistic simulations of didactically-selected systems, situations, and content (Witmer and Singer, 1998; Chernikova et al., 2020). On the hardware side, devices such as HMDs have immersive displays and intuitive user input that may evoke a sense of physicality and authenticity (Velev and Zlateva, 2017). Next, different types of variables used and examined in educational authenticity research will be distinguished from each other.
Two important authenticity-related variables include presence and representation fidelity (Dalgarno and Lee, 2010). Presence is the feeling of being within a virtual environment yet being physically located in another place (Witmer and Singer, 1998). This concept contains, according to a factor analysis by Schubert et al. (2001), the aspects of involvement, realness, and spatial presence. Involvement refers to focusing attentively on the environment, realness is a rating to what extent the environment is as realistic and believable as the real world, and spatial presence denotes feeling located physically in an environment (Schubert et al., 2001). Representation fidelity can be defined as the level of realism that the educational 3D environment with its included objects possesses and is affected by factors such as image and lighting quality (Dalgarno et al., 2002). Even though it has been argued that presence is a more subjective concept and representation fidelity is more objective, it has been theorized that both aspects affect each other (Lee et al., 2010; Makransky and Petersen, 2021).
There are three main reasons for developing educational VR environments with high authenticity. First, providing authentic educational virtual environments can promote important affective outcomes. In this respect, positive effects of authentic educational virtual environments have been reported for motivation (Makransky and Petersen, 2019), curiosity (Schutte, 2020), and interest (Makransky et al., 2020; Petersen et al., 2020). Second, authenticity-related variables are considered to be important mediators of learning (Lee et al., 2010; Makransky and Petersen, 2021). In this respect, empirical studies have shown that highly authentic educational virtual environments can evoke deeper cognitive processing but can also lead to negative side effects such as cognitive overload (Makransky et al., 2019; Škola et al., 2020). Third, situated learning approaches contend that educational virtual environments with high functional and physical authenticity increase the contextualization of what is experienced and facilitate knowledge acquisition (Renkl et al., 1996). In fact, a meta-analysis by Wu et al. (2020) reported that more declarative knowledge was acquired in educational VR environments on average than in regular lecture-based training. However, this meta-analysis also showed relatively comparable knowledge levels were acquired in HMD-based educational VR environments with higher authenticity and regular desktop-based VR environments with lower authenticity (Wu et al., 2020).
The literature provides several insights on whether VR created by 3D modeling or photogrammetry would be more authentic. According to Witmer and Singer (1998), experienced presence depends on multiple, mostly subjective judgments. These judgments include, amongst other things, the scene realism (i.e., the feeling that the stimuli are related to each other), the perceived level of control (i.e., the level of interactivity), and the meaningfulness (i.e., the personal value). However, Witmer and Singer (1998) also acknowledge that external factors such as image and HMD resolution affect these subjective judgments. Skulmowski et al. (2021) take a more technical stance and contend that the level of detail provided by the mesh, texture, and lighting of a 3D model affect its realism rating. In line with these theoretical reasons, authenticity-related variables should be higher in VR created by photogrammetry than in VR created by 3D modeling.
1.3. Interest development through virtual reality
Another goal that VR can pursue in educational contexts lies in supporting interest in new topics and content. In the following paragraphs, we will define interest and discuss its relevance for education before we describe major tenets of interest development theories.
Interest is an affective-cognitive construct focusing on specific objects or topics (Krapp, 2002) that evokes repeated engagement and increased persistence (Hidi and Renninger, 2006; Harackiewicz et al., 2016). This construct is essential for life-long learning (Krapp, 2002). For instance, associations of interest with attention (Bolkan and Griffin, 2018), learning strategies (Schiefele, 1991; McWhaw and Abrami, 2001), and academic achievement (Schiefele et al., 1992; Kpolovie et al., 2014) have been shown.
According to two popular interest development theories (Krapp, 2002; Hidi and Renninger, 2006), this construct evolves from a situational to an individual interest. Situational interest is a temporary state that can either fade away or last longer in the further course of interest development (Hidi, 1990). Individual interest, however, is more stable, lasts longer, and is firmly rooted in a subject’s personality (Renninger et al., 2002). The mentioned development process includes phases in which interest is first induced and then sustained over a longer period to transform qualitatively (Krapp, 2002; Hidi and Renninger, 2006). Value and feeling judgments, individual variables (e.g., cognitive prerequisites), and external variables (e.g., availability of engaging materials) play an important role and interact in the formation and sustainment of interest (Krapp, 2002; Hidi and Renninger, 2006). As this paper focuses on the early phase of interest development, we will now discuss the triggering of situational interest and variables associated with interest development in this phase.
Educational virtual environments can be an important trigger of situational interest (Hidi, 1990). In particular, educational environments that generate positive value and feeling judgments are thought to induce situational interest (Krapp, 2002; Hidi and Renninger, 2006). Multiple studies have supported this point, showing that meaningful educational environments foster the development of situational interest and related constructs in high school and college students (Cordova and Lepper, 1996; Verhagen et al., 2012; Siklander et al., 2017). Recently, these findings have also been substantiated for educational VR environments. Makransky et al. (2020) discovered in two experiments that taking part in laboratory VR simulations with HMDs increased interest in science topics.
In terms of variables associated with early interest development, the two popular theories mentioned (Krapp, 2002; Hidi and Renninger, 2006) argue that pre-existing individual interest and triggered situational interest are linked with the development of later situational interest in new content. Findings from studies on interest trajectories using shorter and longer digital simulations and inquiry environments supported this claim (Tapola et al., 2013; Chen et al., 2016; Rodríguez-Aflecht et al., 2018). As digital simulations and inquiry environments share many commonalities with educational VR environments (e.g., high interactivity and authenticity), we believe this finding should also hold true for educational VR environments. Furthermore, the variable presence could also be a predictor of early interest development. This could be the case because presence is assumed to be beneficial for many regulatory and affective aspects of self-directed learning (Krapp, 2002). In line with this reasoning, an experiment in which psychology students took part in educational VR simulations with high and low immersion wearing HMDs showed that the development of situational interest was affected by varying the degree of presence (Petersen et al., 2022).
1.4. Research questions and hypotheses
Against this background, we pose three research questions:
RQ1: To what extent do the authenticity-related variables involvement, realness, spatial presence, and representation fidelity differ in educational VR environments created by 3D modeling and photogrammetry?
We hypothesize that involvement (H1.1), realness (H1.2), spatial presence (H1.3), and representation fidelity (H1.4) are higher in the VR created by photogrammetry than in the VR created by 3D modeling.
RQ2: To what extent does interest develop in educational VR environments created with 3D modeling resp. photogrammetry?
We assume that interest in VR for learning (H2.1) and interest in bridges (H2.2) increase across both educational VR environments.
RQ3: To what extent can posttest situational interest be predicted by pretest situational interest, group (3D modeling resp. photogrammetry), presence, and representation fidelity?
We expect that posttest interest in VR for learning (H3.1) and posttest interest in bridges (H3.2) is predicted by pretest interest, group, presence, and representation fidelity.
2. Method
2.1. Participants
N = 64 educational sciences and psychology university students of a German university took part in the study. The sample consisted of 17.2% (n = 11) participants below the age of 21, 59.4% between 21 and 24 (n = 38), 20.3% (n = 13) above 24, and 3.1% (n = 2) participants with a missing age. 50% (n = 32) of the participants were freshmen, 39.1% (n = 25) sophomores, 6.2% (n = 4) juniors, and 1.6% (n = 1) seniors; 3.1% (n = 2) did not report their study year. A majority of 81.2% (n = 52) of the participants had none or very little VR experience, 14.1% (n = 9) had little experience with VR, and 4.7% (n = 3) did not provide an answer.
2.2. Study design, procedure, and randomization
The experiment employed a two-group between-subjects design with a pretest and a posttest. The pretest included questions on demographics and interest. Afterwards, participants took part in an electronic tutorial which made them familiar with the educational VR environment. The subsequent intervention phase focused on exploring a railroad bridge in VR. The control group (n = 33) encountered an educational virtual environment created with 3D modeling in this phase. The intervention group (n = 31), however, was presented with the same educational virtual environment in which the main object of the railroad bridge was generated by photogrammetry. Both groups are depicted in Figure 1 and are closer described in the remainder of this chapter. Participants were randomly assigned to one of the two described groups by drawing a ticket. Consequently, the experimenter administered the corresponding educational VR environment to them. The posttest assessed the experienced presence and representation fidelity and participants’ interest after taking part.
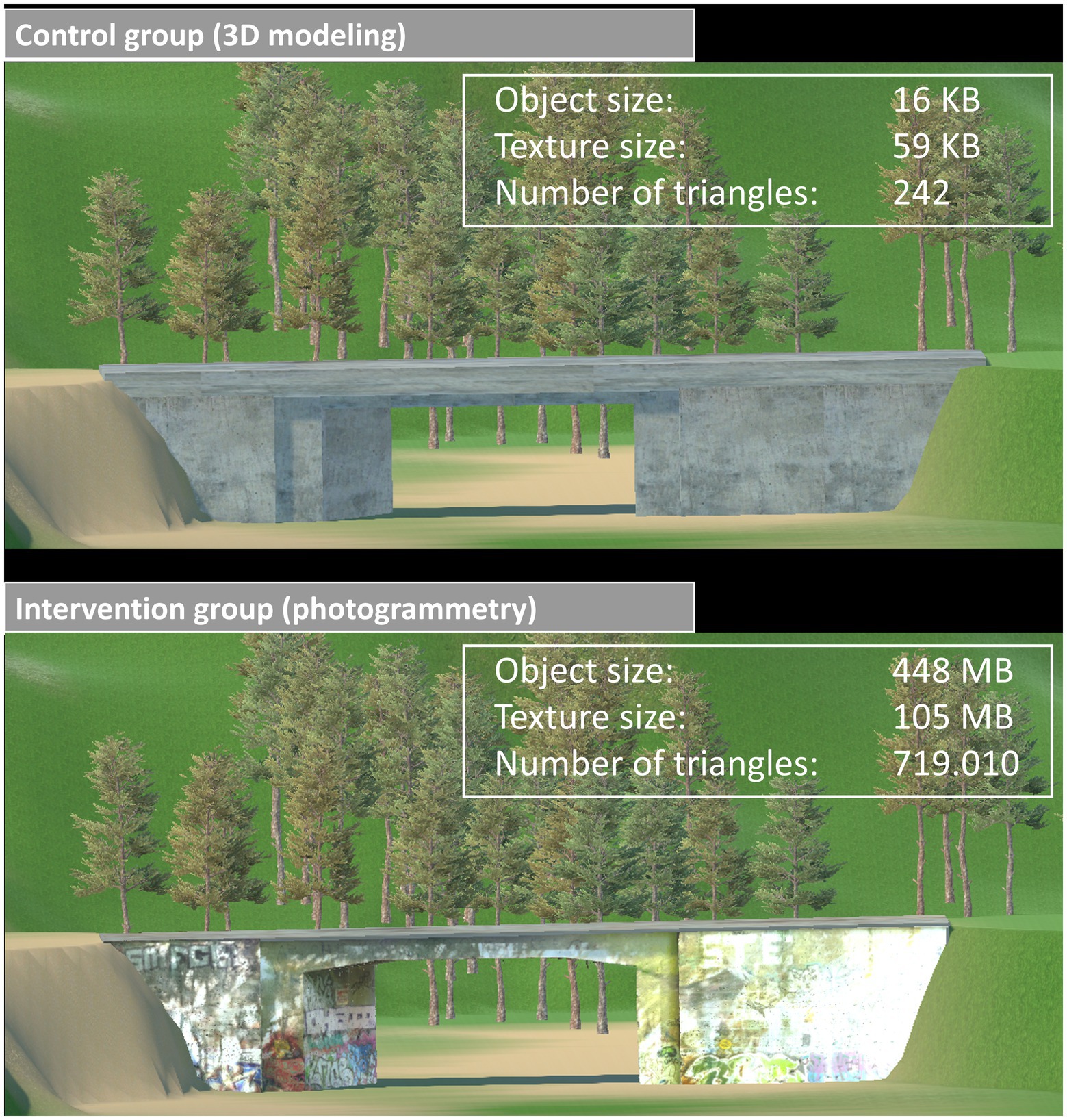
Figure 1. Overview of the 3D model contained in the control and intervention groups. The used 3D models had a relatively similar geometry and comparable dimensions, even though they differed on several other objective characteristics (please see the box). In the photogrammetry group, details such as graffiti were included and fine lines of the structure can be seen.
2.2.1. Educational standpoint and VR scenario
From an educational standpoint, the study can be considered a virtual field trip. A virtual field trip is “a journey taken without actually making a trip to the site” (Woerner, 1999, p. 5) that aims to reach predefined educational goals. The main educational goals the study pursued were sparking interest in a construction engineering topic, experiencing a concrete representation of a railroad bridge, and conveying basic knowledge about bridge construction and characteristics. Consistent with self-determination theory (Deci et al., 1991), two instructional design choices were made. The educational virtual environment emphasized free exploration to provide a sense of autonomy. Moreover, the educational virtual environment only conveyed basic knowledge to allow the participating education and psychology students to experience a sense of competence. Finally, the study draws on theories of multimedia education (Makransky and Petersen, 2021; Vogt, 2021), which emphasize that fidelity and instructional support (e.g., visualizations) can improve motivational and affective states.
The VR scenario depicted in Figure 2 focused on exploring a railroad bridge and gathering information about it. More specifically, participants received 7 min to teleport between eight different platforms freely. Depending on the active teleportation platform, participants could interact with one of two types of user interaction: video screens and visualizations. The video screens played films of PowerPoint slides with audio recordings that focussed on the bridge’s features and characteristics. The visualizations (such as blueprints) depicted the bridge’s features and characteristics graphically. With respect to content, it should be highlighted that some of the content was related to engineering (e.g., the blueprints) while other content had to do with the bridge itself (e.g., the environment). The scenario, video screens, visualizations and the content were created in collaboration with the civil engineering department and reviewed by them for correctness.
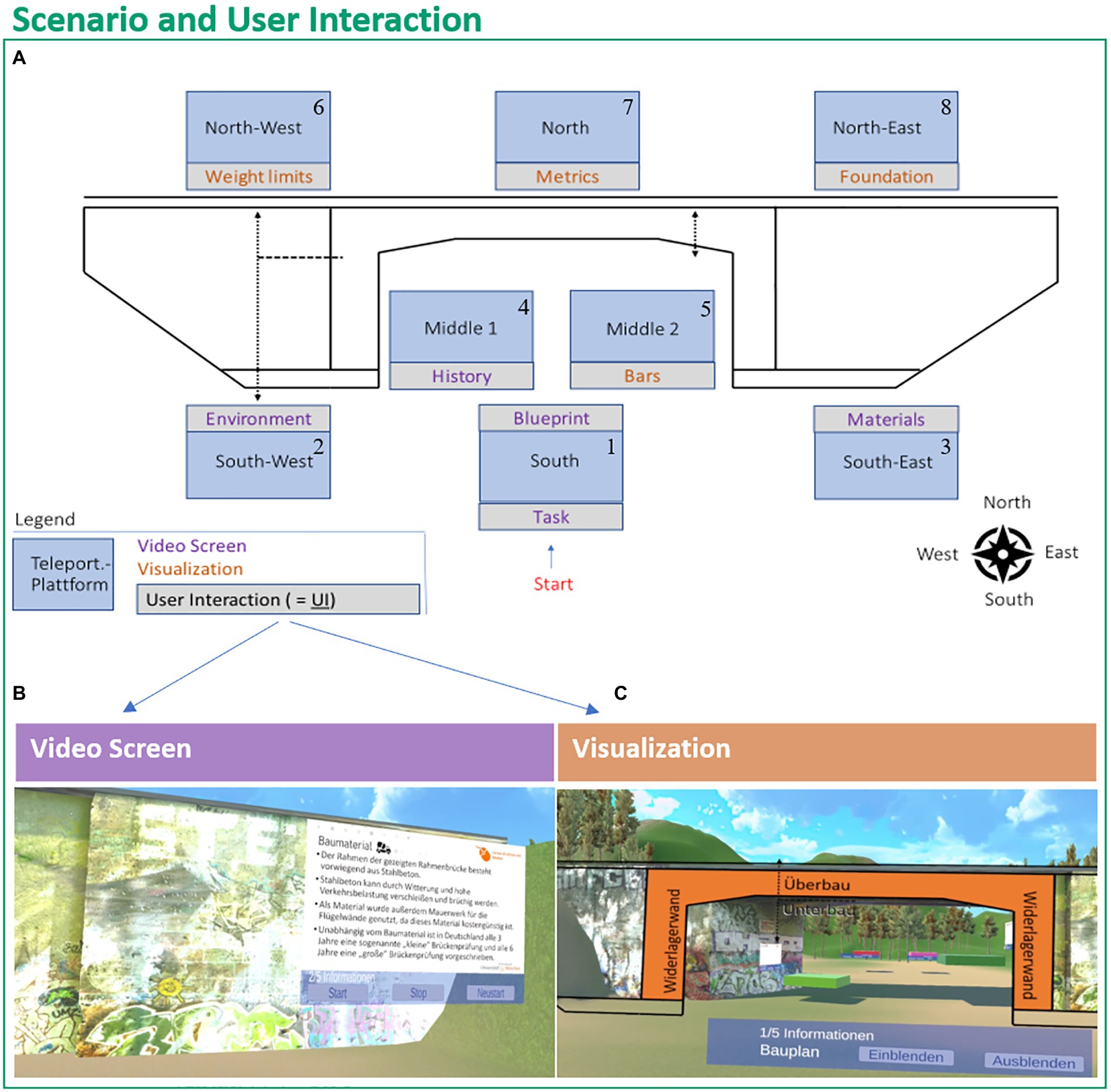
Figure 2. Scenario and user interaction included in the VR environment. Based on the scenario (A), participants started at the platform “South” and then teleported freely to seven other teleportation platforms in the order of their choosing. Video screens (B) and visualizations (C) were offered as user interaction at the teleportation platforms.
2.2.2. Tutorial, terrain, and contents in the VR
At the beginning of the experiment, participants took part in an electronic tutorial. This tutorial was situated in a neutral virtual environment and lasted about four minutes. As part of the tutorial, participants familiarized themselves with teleporting across the platforms and interacting with video screens and visualizations. This way, the tutorial prepared the participants well for the educational VR environment which took place afterwards.
Both educational VR environments used the same simple terrain. This terrain consisted of a valley with a dried-out river, hills, and conifers. The railroad bridge, which was varied in both groups, was located in the middle of this terrain and crossed the dried-out river. Through its large size and center position, the railroad bridge was the educational environment’s main object and center of attention.
Next, we will discuss how both of the created educational VR environments depicted in Figure 1 differed from each other. In the control group, a regular 3D model of a railroad bridge was used. This 3D model was created by hand using the software blender (The Blender Foundation, 2021). The proportions and surface texture of the 3D model were loosely based on the real bridge. A neutral, grey texture that matched to the level of detail of the surrounding environment was applied to the railroad bridge. In the intervention group, a high-resolution photogrammetric model of a real railroad bridge was included. This model was recorded with the terrestrial laser scanner FARO FocusS (Faro Technologies, 2022) and then post-processed with the software RealityCapture (Epic Games, 2021). Thus, the model’s proportions and surface structure were very close to the original railroad bridge. The texture was created from photos of the original bridge also taken by the terrestrial laser scanner and included graffiti and realistic lighting. To hold both described groups as comparable as possible, the used 3D models had a relatively similar geometry and comparable dimensions. The mentioned texture difference between the two groups (i.e., the neutral texture in the 3D modeling group and the high-resolution texture in the photogrammetry group) was deliberately retained. Firstly, this decision magnified a particular feature of the two experimental groups that could have been detected in our comparison of authenticity (RQ1). Adding a high-resolution texture and details to the 3D modeling group would have decreased the expected differences between the 3D modeling group and the photogrammetry group we were investigating. Secondly, we opted for this decision because the described difference in texture detail could occur frequently when these two content creation methods are used in educational settings. Educational scientists and practitioners who create 3D models with graphics software will mostly use simple stock textures. This group of people typically does not have the skills and/or time to create high-resolution textures for 3D models that contain fine details and artwork themselves. It is unquestionable that educational scientists and practitioners with large budgets can obtain 3D models with fine details and artwork. The IT companies hired to do this either employ their own 3D modelers and art designers or purchase high-resolution 3D models from third parties. When educational scientists and practitioners use photogrammetry, matters are different. Educational scientists and practitioners can use photogrammetry to create 3D models with high resolution and detail without spending large sums of money and hiring contractors.
2.2.3. Creation and implementation of the educational VR environments
The described educational virtual environments and their terrain were created with the game engine Unity (Unity Technologies, 2020). Functions specific to VR, such as input and output via the HMDs, were developed using the XR Interaction Toolkit (Unity Technologies, 2021). Scripts for specific purposes and customizations of the educational virtual environment (e.g., creating the control flow of the educational VR environments), were written with the programming language C#.
The experiment was conducted in a small, dedicated VR laboratory. In this lab, the participants had sufficient space for walking around and turning their heads. Further, participants were observed by an experimenter who ensured their safety. While instrument data were collected using paper-and-pencil questionnaires, the tutorial and the educational environments ran on a gaming laptop. An HTC Vive (HTC, 2016) was used as a HMD.
2.3. Instruments
2.3.1. Interest
Interest was assessed with a 6-item scale (Rotgans and Schmidt, 2011). Two versions of this scale were created: One measuring interest in VR for learning and one tapping into interest in bridges. For brevity reasons, the first scale is also referred to as VR interest, while the second scale is called Bridge interest. Both scales were used in the pretest and the posttest. The described instrument utilized a Likert scale ranging from 1 (strongly disagree) to 5 (strongly agree). Lower values indicated lower interest, whereas higher values represented higher interest.
2.3.2. Presence
Presence was measured with a 13-item questionnaire (Schubert et al., 2001). This questionnaire comprised items on the involvement, realness, and spatial presence of the educational environment. It was administered directly after taking part in the VR and used a 7-point Likert scale reaching from −3 to 3 with varying anchors. Lower values stood for lower presence, higher values for higher presence.
2.3.3. Representation fidelity
Data on representation fidelity was collected with a 3-item instrument (Lee et al., 2010). This instrument focused mainly on the experienced realism of the 3D scene. Anchors of the instrument ranged from 1 (strongly disagree) to 5 (strongly agree).
3. Results
3.1. Descriptive statistics and manipulation checks
The descriptive statistics for the experiment are reported in Table 2. Manipulation checks using two-sided t-tests were conducted to screen for unwanted pretest group differences. Pretest interest in VR for learning and pretest interest in bridges did not differ significantly between the control and the intervention group, as intended [t(61) = 0.74, p = 0.461 resp. t(62) = 1.11, p = 0.270].
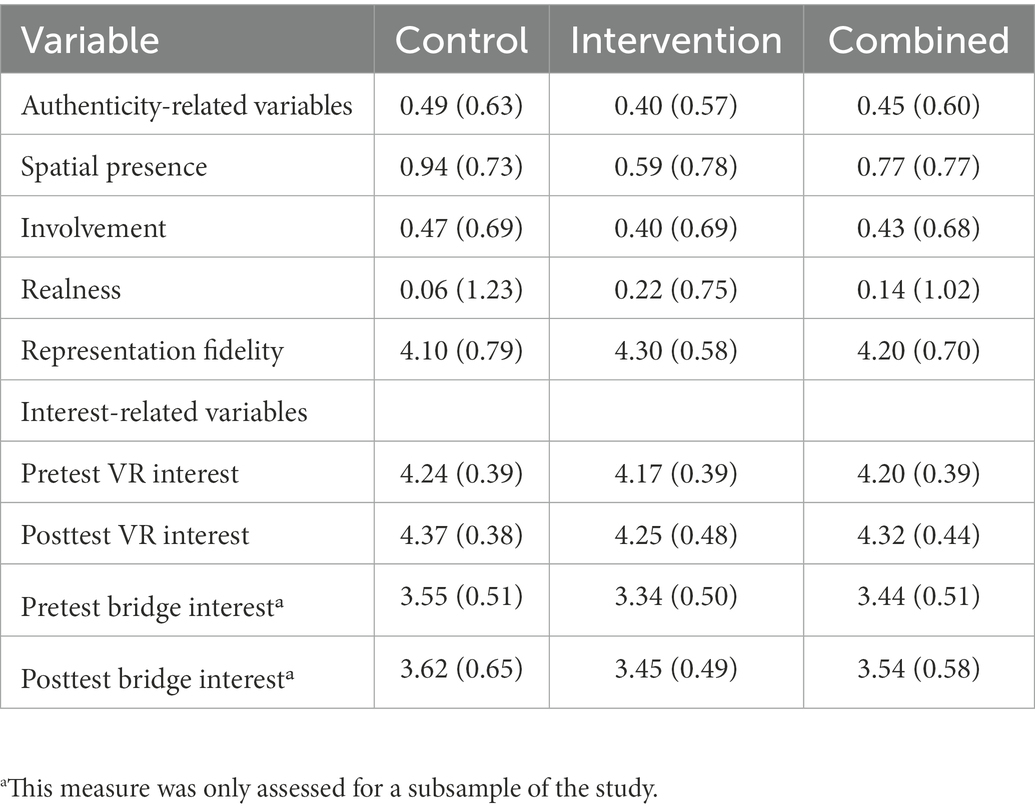
Table 2. Means (and SDs) for authenticity-related and interest-related variables in the control group, the intervention group, and combined for both groups.
3.2. Differences in authenticity-related variables in educational VR environments created by photogrammetry and through 3D modeling (RQ1)
To examine RQ1, one-sided independent samples t-tests of the authenticity-related variables involvement, realness, spatial presence, and representation fidelity split by the group were conducted. These analyses are visualized and reported in a boxplot in Figure 3. All four mentioned authenticity-related variables were not significantly greater in the intervention group than in the control group. Thus, the hypotheses H1.1-H.1.4 were not supported.
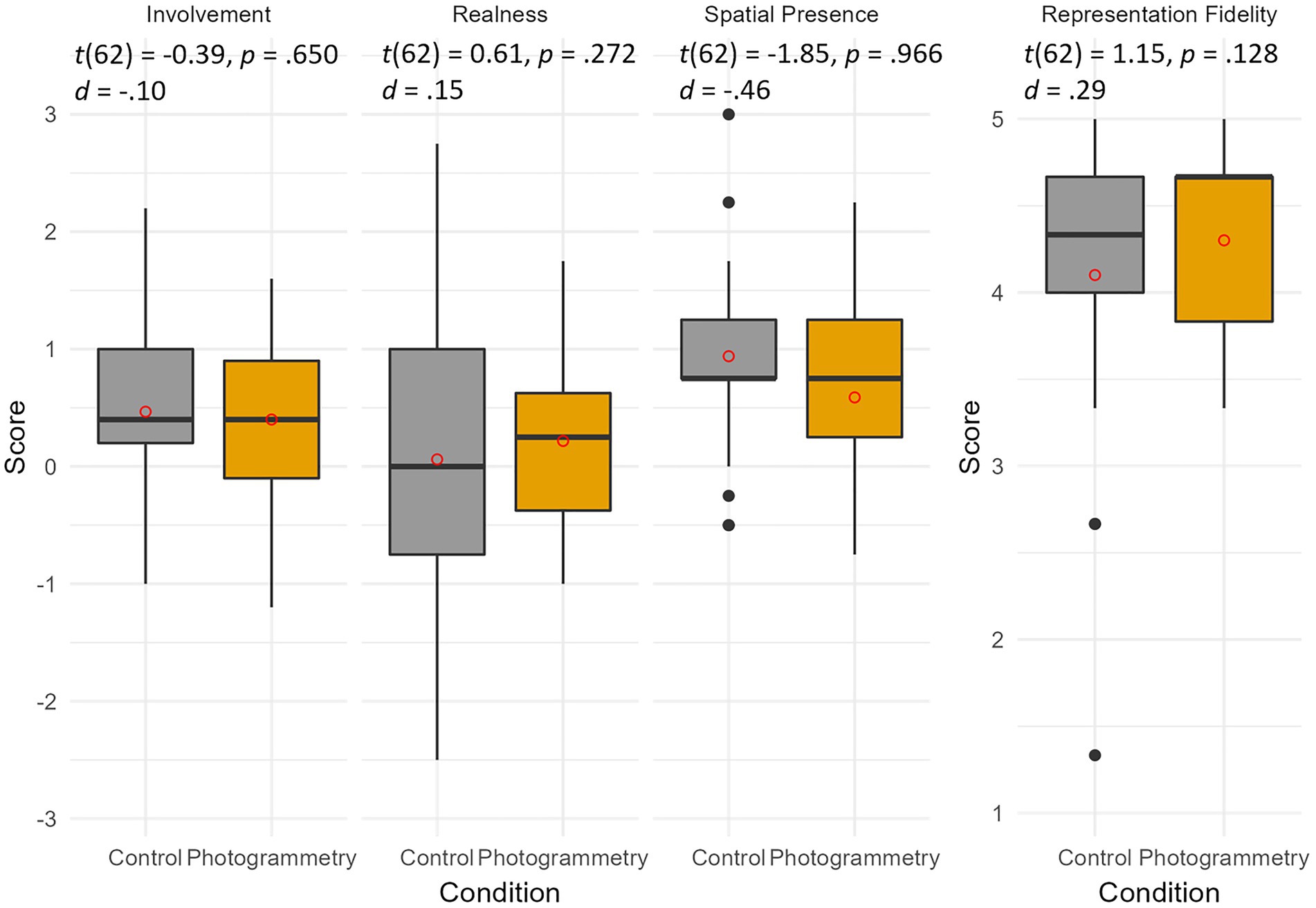
Figure 3. Group comparisons using one-sided independent t-test results of the authenticity-related variables. Involvement, realness, and spatial presence ranged from −3 to 3. Representation fidelity ranged from 1 to 5. The box of the boxplot visualizes the interquartile range, including the median, Q2 (the 25th percentile), and Q3(the 75th percentile). Whiskers of the boxplot show Q1 and Q4. The red circles represent the mean. Effect sizes are reported using Cohen’s d.
3.3. Interest development in educational VR environments (RQ2)
To investigate RQ2, one-sided dependent sample t-tests of the interest development from the pretest to the posttest were calculated. These analyses are reported and visualized in Figure 4. Interest in VR for learning increased from the pretest to the posttest in the full sample. Therefore, H2.1 was substantiated. However, bridge interest did not improve significantly from the pretest to the posttest in the full sample. Therefore, H2.2 was not supported. Additionally, explorative analyses were also conducted separately for the intervention group and the control group (see Figure 4). These explorative analyses rely on small subsamples and should not be overinterpreted. However, the explorative analyses provide an initial indication that interest increases did not differ strongly according to the group (resp. content creation method).
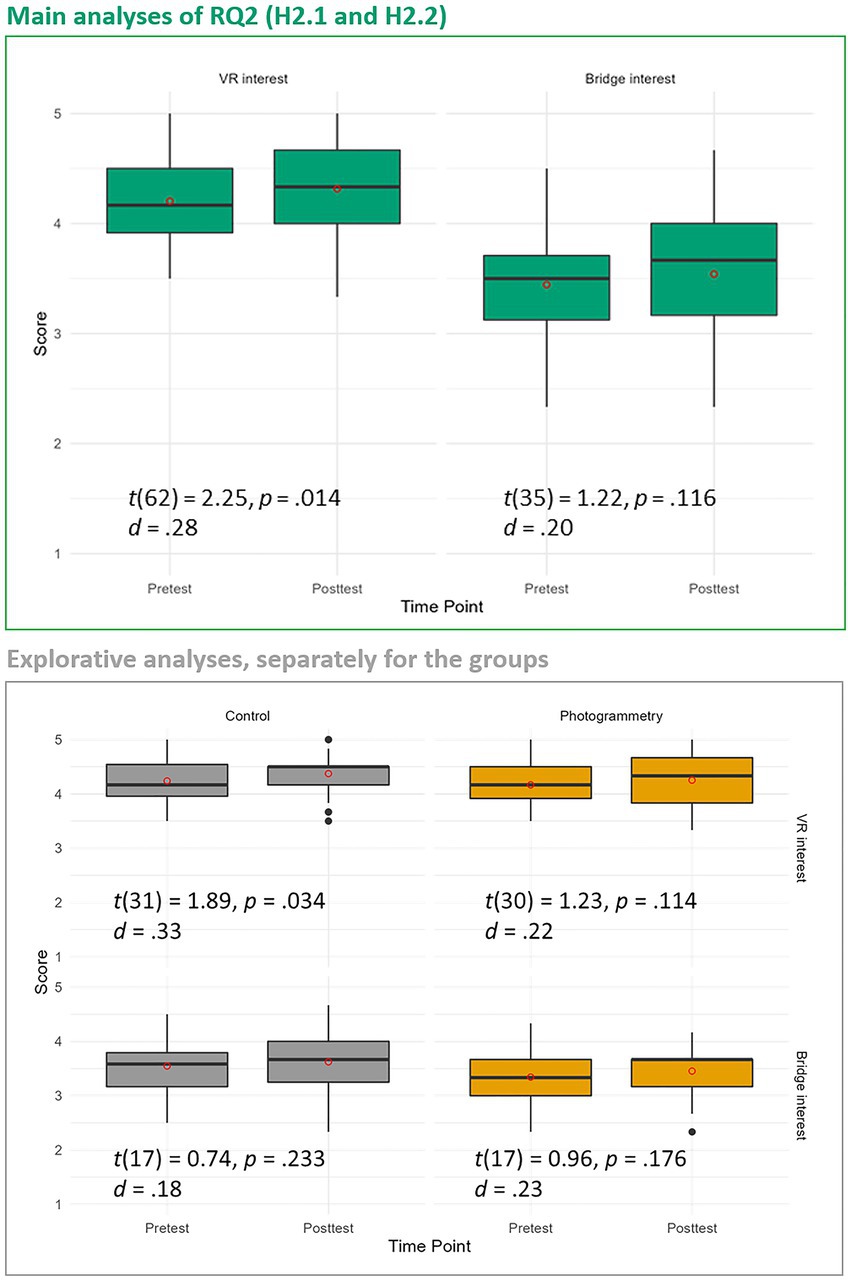
Figure 4. Boxplots of the interest scores and results of one-sided paired-samples t-tests. The results for the main analyses are based on the full sample. The explorative analyses are reported separately for the control and the photogrammetry group. Interest scores ranged from 1 to 5. The box of the boxplot visualizes the interquartile range, including the median, Q2 (the 25th percentile), and Q3 (the 75th percentile). Whiskers of the boxplot show Q1 and Q4. The red circle depicts the mean. Effect sizes are reported using Cohen’s d.
3.4. Variables explaining situational posttest interest (RQ3)
Before conducting regression analyses, we inspected intercorrelations between the interest-related and authenticity-related variables (see Table 3). Both posttest interest variables (interest in VR for learning and interest in bridges) correlated significantly with the corresponding pretest interest, the full presence score, the authenticity-related variables realness, and representation fidelity. Despite being smaller and sometimes not reaching significance, there were also associations between both posttest interest variables and involvement and spatial presence. Representation fidelity and the full presence score displayed a medium positive correlation (r = 0.32) with each other. Additionally, separate correlation analyses for the control group and the intervention group are available in Supplementary Tables 1, 2.
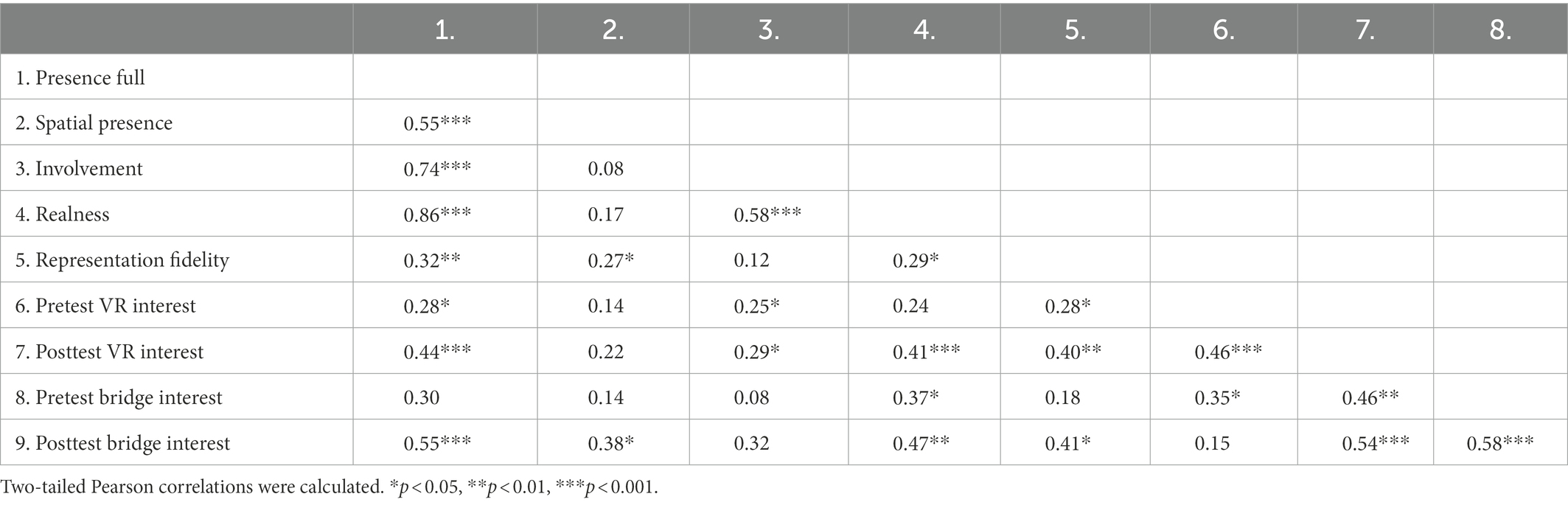
Table 3. Intercorrelations between authenticity-related and interest-related variables combined for both educational virtual environments.
3.4.1. Interest in VR for learning
A hierarchical regression for posttest interest in VR for learning as outcome was conducted and is presented in Table 4. Model V1 (R2 = 0.21), containing the pretest interest, was significant and explained a substantial amount of variance. Model V2 (R2 = 0.22), additionally including the group, did not explain significantly more variance than Model V1 [F(1, 60) = 1.20, p = 0.277, ΔR2 = 0.02]. In Model V3 (R2 = 0.31), presence was added as predictor which resulted in additional explained variance in comparison to Model V2 [F(1, 59) = 7.54, p = 0.008, ΔR2 = 0.09]. Model V4 (R2 = 0.36) included all mentioned predictors plus representation fidelity. Model V4 explained significantly more variance than Model V3 [F(1, 58) = 4.76, p = 0.033, ΔR2 = 0.05]. The overall model including pretest interest, group, presence and representational fidelity was significant. However, group was not a significant predictor of posttest interest in VR for learning. Consequently, H3.1 was partially substantiated.
3.4.2. Bridge interest
A hierarchical regression for posttest bridge interest as a dependent variable was carried out and is shown in Table 5. Model B1 (R2 = 0.34), including the pretest bridge interest, was significant and explained a substantial amount of posttest bridge interest. Model B2 (R2 = 0.34), which also contained the group, did not explain significantly more variance than Model B1 [F(1, 33) = 0.09, p = 0.761, ΔR2 = 0.00]. Model B3 (R2 = 0.49) included, as an additional variable, the full presence score and resulted in a raise of explained variance compared to Model B2 [F(1, 32) = 9.41, p = 0.004, ΔR2 = 0.15]. Model B4 (R2 = 0.52) consisted of all mentioned variables plus representation fidelity and did not increase explained variance compared to Model B3 [F(1, 31) = 1.48, p = 0.233, ΔR2 = 0.02]. Bridge interest was explained by pretest interest, and presence. Yet, group and representational fidelity were not significant predictors. Based on these results, H3.2 was partially substantiated.
4. Discussion
4.1. Authenticity in virtual reality created by 3D modeling and photogrammetry (RQ1)
This paper examined to what extent authenticity-related variables differ in educational VR environments created by 3D modeling and photogrammetry. Our results indicate that the three presence-related variables (involvement, spatial presence, and realness) and representational fidelity do not differ between educational environments created by using 3D modeling and photogrammetry. There are several possible explanations for our results. One explanation may be that subjective judgments play a particularly important role in creating the feeling of presence (Witmer and Singer, 1998). These subjective judgments might have been comparable in both groups and perhaps led to the reported equal presence and representation fidelity level. Another explanation for our findings could lie in the measurement of the authenticity-related variables. Our instruments did not distinguish between the multiple, varying objects contained in the 3D scene but measured perceived authenticity globally. Differences between the authenticity of the environment (i.e., the terrain) and the varied main object of interest (i.e., the railroad bridge) could therefore not be captured. It seems possible that especially in hybrid educational environments involving objects created with 3D modeling and photogrammetry, separate measurements of authenticity are necessary to reveal relationships between object characteristics and authenticity-related variables. A final explanation of our findings could be that while the authenticity of virtual environments might generally depend on the detail, texture, and lighting of 3D models (Skulmowski et al., 2021), this relationship may be reduced in hybrid educational virtual environments. Participants likely focused so strongly on gathering information in our educational virtual environment that they did not notice the objective differences in the detail, texture, and lighting created by the main object (i.e., the railroad bridge) included in the scene. Consequently, further research should more closely examine in which contexts and under what conditions, authenticity-related variables are determined by the detail, texture, and lighting of 3D objects.
4.2. Triggering of situational interest through virtual reality (RQ2)
Another focus of this paper was to investigate the triggering of situational interest through educational VR environments. In doing so, we measured two types of interest: interest in VR for learning and interest in bridges. Interest in VR for learning increased significantly from the pre- to the posttest in the full sample. This finding is in line with other studies’ results that regular technology-enhanced educational environments can induce situational interest and related constructs (Cordova and Lepper, 1996; Verhagen et al., 2012; Siklander et al., 2017). Moreover, this finding adds support to two studies that found that interest can be triggered within educational VR environments (Makransky et al., 2020). Interest in bridges rose slightly from the pre- to the posttest in the full sample but did not reach significance. The discovered non-significant interest growth for this type of interest was smaller than for interest in VR for learning. One possible explanation for this result could be that it may have been more difficult to induce interest in an engineering topic among the participating educational science and psychology students. This explanation is backed up by short debriefing talks, in which multiple participants commented that they had difficulty getting excited about engineering topics.
Our study also provides new insights into inducing interest in educational VR environments using 3D modeling and photogrammetry. The interest growths encountered in our explorative analyses (see Figure 2) did not differ strongly and coherently between these two content creation methods. A dive into the literature shows that this finding is not surprising. Interest development is determined – apart from individual predispositions – to a large extent by value and feeling judgments toward the content (Krapp, 2002; Hidi and Renninger, 2006). Probably, value and feeling judgments toward the content were relatively similar in both groups as both educational virtual environments possessed many shared features (e.g., the same content on the video screens and in the visualizations) except for the physical appearance of the main 3D object (i.e., the railroad bridge). It is an open question, to what extent other characteristics of educational virtual environments interact with interest development. In this regard, the match between the functions educational virtual environments provide and the situations they represent (Chernikova et al., 2020) could be an important characteristic. Functionally more realistic educational virtual environments could be associated with higher interest development, particularly because they could increase value and feeling judgments toward the content. Photogrammetry and VR could be used in tandem with realistic tasks and interaction possibilities to develop such educational virtual environments offering high physical and functional realism.
4.3. Variables related to developing situational interest (RQ3)
This paper also sought to gain insights into the variables related to developing situational interest in the context of VR. As the majority of results were similar for the outcomes of interest in VR for learning and interest in bridges, we will discuss these results together.
Our study showed that pretest situational interest was a predictor of posttest situational interest. This finding is consistent with theoretical reasoning put forward by interest development theories (Krapp, 2002; Hidi and Renninger, 2006) and aligns well with empirical findings on interest trajectories conducted for other technology-enhanced educational methods such as digital simulations and inquiry environments (Tapola et al., 2013; Chen et al., 2016; Rodríguez-Aflecht et al., 2018). The content creation method of the educational VR environment (3D modeling resp. photogrammetry) was, however, not a significant predictor of posttest situational interest. One explanation for this result could be – as pointed out in Section 4.2 which addressed a related topic – that both methods of content creation lead to comparable value and feeling judgments (Krapp, 2002; Hidi and Renninger, 2006). This could be particularly the case because both educational environments offered participants the same tasks and information despite their visual differences.
Authenticity-related variables like presence and their association with interest development were also examined. Presence explained substantial variance in posttest situational interest. This finding corresponds to an experiment by Petersen et al. (2022) on education in VR which found that varying degrees of immersion affected interest development. Our experiment goes beyond this experiment with two points. First, it used presence as a broader operationalization of authenticity than immersion. Second, our experiment showed that presence explained posttest interest over and above the variance accounted for by preexisting situational interest. To conclude, Petersen et al.’s (2022) and our experiment identify presence as a vital variable to consider when analyzing interest development in educational environments. This link between presence and interest development in VR can perhaps be best explained by the positive regulatory and affective aspects that may go along with the experience of presence (Krapp, 2002). More specifically, an increased feeling of presence can be accompanied by improved focus and positive affect states like flow. In our study, such positive regulatory and affective aspects may have led to improved interest development when experiencing higher levels of presence.
Our findings on associations with representation fidelity differ for the two interest outcomes. For interest in VR for learning, representation fidelity was a significant predictor. Moreover, the regression model including representation fidelity explained more variance than the model only including pretest interest, the group, and presence. This result indicates that representation fidelity can be used as an additional predictor of interest development in some contexts. For interest in bridges, representation fidelity was, however, not a significant predictor. One explanation of this result could be that representation fidelity does not affect interest development directly but is - in line with Makransky and Petersen (2021) - mainly an antecedent of presence. In support of this point, we discovered a positive correlation between representation fidelity and the full presence score (r = 0.32).
4.4. Limitations
One limitation of our study is the restricted generalizability of the reported findings on the content creation methods. To critically discuss this point, we would like to revisit our experimental manipulation briefly. In both experimental groups, the same terrain and environment created with 3D modeling was used. Between both experimental groups, the content creation method was varied and the railroad bridge as the main object of interest and center of attention was either created by 3D modeling or photogrammetry. This decision was primarily made to reach a high degree of experimental standardization across the groups. We believe it resulted in experimentally comparable bridge models which possessed few manifest differences in the mesh, texture, and lighting, but substantial objective differences in these characteristics (see Figure 2). In contrast, it could be argued that particular features like the graffiti contained in the photogrammetry group were seductive details, as defined as “interesting but irrelevant adjuncts” (Harp and Mayer, 1998, p. 414, p. 414). This point is relevant as seductive details could have potentially bolstered perceived authentiticy and interest development in the photogrammetry group. The fact that authenticity levels and interest increases in the control group and the photogrammetry group were relatively comparable suggests that seductive details did not play a crucial role in our study. Based on these considerations, we believe that our results can be generalized to new types of hybrid educational virtual environments that consist primarily of 3D modeled terrain and environments and also include one or a few objects created with photogrammetry. These hybrid educational environments are relatively comparable to the photogrammetry group studied in this paper.
Another limitation of our study is the relatively small sample of N = 64 participants. Developing this innovative study was intricate and required considerable man-hours in programming, graphics design, and creating photogrammetric models on top of regular research tasks. Likewise, conducting the study was time-consuming as all participants had to be tested in one-on-one settings in a VR laboratory that had to be set up each time. Due to the higher effort of developing and conducting the study, it cannot be expected that the study reaches the same number of participants as questionnaire studies. Nevertheless, a larger sample size would have been desirable for examining the described interest and authenticity research questions. This point is highlighted by the effect sizes our study reported and should be considered by future studies on authenticity and interest in VR.
Finally, it should be emphasized that the reported findings were obtained for educational VR environments displayed with HMDs. For this reason, generalizations to other VR technologies should be made only with caution. CAVE sytems, in which participants experience a VR in a dedicated room consisting of multiple screens, typically offer a higher resolution and/or wider viewing angles than HMDs. Therefore, CAVE systems could represent educational environments in more detail than HMDs, possibly evoking authenticity and interest differences between 3D modeling and photogrammetry as content creation methods.
5. Conclusion and outlook
We investigated authenticity and interest development in an educational VR environment. 3D modeling and photogrammetry were employed as content creation methods to develop two different educational virtual environments and then examined further. With respect to authenticity, we discovered that varying the content creation methods did not evoke a substantial difference. This finding implies that creating educational environments with 3D modeling may be sufficient for many educational contexts in which participants mainly focus on the task and have no need to note fine details of contained 3D objects. Regarding interest development, there were two main findings. First, we discovered that interest in VR for learning increased from the pretest to the posttest. This finding aligns well with other studies’ results and corroborates that educational virtual environments, including VR with HMDs, can trigger situational interest (Cordova and Lepper, 1996; Verhagen et al., 2012; Siklander et al., 2017; Makransky et al., 2020). Second, we examined the variables associated with interest development and identified presence as an important predictor that explained substantial amounts of variance. This finding is consistent with a result by Petersen et al. (2022) and can also be explained theoretically. Perhaps the positive regulatory and affective aspects that accompany presence (Krapp, 2002) also link this variable with higher interest development.
After carrying out the study, we are convinced that photogrammetry and 3D modeling both have unique benefits and drawbacks and are suitable for different use-cases of content creation in education. 3D modeling can be particularly suitable in contexts in which the user’s attention is not on the object itself but on learning. Researchers and practitioners, may, thus develop or acquire 3D modeled objects for such use-cases and devote time and effort to the content, tasks, and support of the participants. Photogrammetry, however, can be particularly suited to creating educational virtual environments in which fine details of objects have to be visualized. This technology also allows researchers and practitioners who have no experience with graphic design to easily create highly-detailed objects.
To wrap this paper up, we will now take a brief look at the future of VR and photogrammetry in education. VR enables users to make multi-sensory, highly-immersive experiences. Within the next few years, VR hard and software will likely make further progress and become more accessible. VR hardware, such as HMDs, will become more affordable, compact, and overall more practical and may thus enable a better integration within educational contexts. VR software, such as simulations and meta-verse applications, will become more authentic, interactive, and meaningful. We believe that VR will then become an important part of education, that may fulfill particular goals, such as providing participants with realistic contexts for improved problem-solving and transfer as well as sparking interest. Photogrammetry has been primarily part of surveying, but it will likely soon become more accessible and will be used in other fields. Since photogrammetry enables the automatic and time-efficient creation of 3D models, it can become an alternative to manual 3D modeling with graphics software. When creating educational virtual environments, photogrammetry can be used to create life-like objects and terrains. Our study illustrates that researchers and practitioners can already use the two new technologies, VR and photogrammetry, to create authentic and interesting educational virtual environments. This finding is noteworthy because the two technologies do not appear to have been used together in educational contexts before, even though they fit well together and complement each other.
Data availability statement
The raw data supporting the conclusions of this article will be made available by the authors, without undue reservation.
Ethics statement
The studies involving human participants were reviewed and approved by Ethics committee of Universität der Bundeswehr München. The patients/participants provided their written informed consent to participate in this study.
Author contributions
MF, DS, VE, and BE designed the experiment together. MF and DS programmed and created the educational environments and used stimuli. MF and BE pre-processed and analyzed the data. MF created the first draft of the manuscript. VE wrote individual sections of the abstract and theory. DS, VE, and BE provided feedback as well as critical revisions of the manuscript. All authors read and approved the final version of the manuscript for submission.
Funding
This research paper is funded by dtec.bw – Digitalization and Technology Research Center of the Bundeswehr [project RISK.twin]. We acknowledge financial support by Universität der Bundeswehr München.
Acknowledgments
We thank Johannes Wimmer for his advice and support in creating the concept of the educational environment. Moreover, we are grateful to Fabian Seitz for reviewing and correcting the materials of the educational virtual environment. Credit also goes to Maximilian Gründl who served as a consultant in creating the photogrammetric model of the railroad bridge. MF thanks Larissa Kaltefleiter for her advice and support.
Conflict of interest
The authors declare that the research was conducted in the absence of any commercial or financial relationships that could be construed as a potential conflict of interest.
Publisher’s note
All claims expressed in this article are solely those of the authors and do not necessarily represent those of their affiliated organizations, or those of the publisher, the editors and the reviewers. Any product that may be evaluated in this article, or claim that may be made by its manufacturer, is not guaranteed or endorsed by the publisher.
Supplementary material
The Supplementary material for this article can be found online at: https://www.frontiersin.org/articles/10.3389/feduc.2023.969966/full#supplementary-material
References
American Society for Photogrammetry and Remote Sensing (2022). “What is ASPRS? ” http://www.asprs.org/a/society/about.html.
Bolkan, S., and Griffin, D. J. (2018). Catch and hold: instructional interventions and their differential impact on student interest, attention, and autonomous motivation. Commun. Educ. 67, 269–286. doi: 10.1080/03634523.2018.1465193
Chen, J. A., Tutwiler, M. S., Metcalf, S. J., Kamarainen, A., Grotzer, T., and Dede, C. (2016). A multi-user virtual environment to support students' self-efficacy and interest in science. A latent growth model analysis. Learn. Instr. 41, 11–22. doi: 10.1016/j.learninstruc.2015.09.007
Chernikova, O., Heitzmann, N., Stadler, M., Holzberger, D., Seidel, T., and Fischer, F. (2020). Simulation-based learning in higher education: a meta-analysis. Rev. Educ. Res. 90, 499–541. doi: 10.3102/0034654320933544
Cordova, D. I., and Lepper, M. R. (1996). Intrinsic motivation and the process of learning: beneficial effects of contextualization, personalization, and choice. J. Educ. Psychol. 88, 715–730. doi: 10.1037/0022-0663.88.4.715
Dalgarno, B., Hedberg, J., and Harper, B. (2002). “The contribution of 3D environments to conceptual understanding” in Winds of change in the sea of learning: Proceedings of the 19th annual conference of the australasian society for computers in learning in tertiary education. ed. O. J. McKerrow (Auckland: UNITEC, Institute of Technology), 149–158.
Dalgarno, B., and Lee, M. J. W. (2010). What are the learning affordances of 3-D virtual environments? Br. J. Educ. Technol. 41, 10–32. doi: 10.1111/j.1467-8535.2009.01038.x
Deci, E. L., Vallerand, R. J., Pelletier, L. G., and Ryan, R. M. (1991). Motivation and education: the self-determination perspective. Educ. Psychol. 26, 325–346. doi: 10.1080/00461520.1991.9653137
Epic Games (2021). RealityCapture. Available at: https://www.capturingreality.com/
Erolin, C. (2019). “Interactive 3D digital models for anatomy and medical education,” in Biomedical visualization: Advances in experimental medicine and biology. ed. P. M. Rea (Cham: Springer), 1–16. doi: 10.1007/978-3-030-14227-8_1
Faro Technologies (2022). Available at: https://www.faro.com/
Harackiewicz, J. M., Smith, J. L., and Priniski, S. J. (2016). Interest matters: the importance of promoting interest in education. Policy Insights Behav. Brain Sci. 3, 220–227. doi: 10.1177/2372732216655542
Harp, S. F., and Mayer, R. E. (1998). How seductive details do their damage: a theory of cognitive interest in science learning. J. Educ. Psychol. 90, 414–434. doi: 10.1037/0022-0663.90.3.414
Hidi, S. (1990). Interest and its contribution as a mental resource for learning. Rev. Educ. Res. 60, 549–571. doi: 10.3102/00346543060004549
Hidi, S., and Renninger, K. A. (2006). The four-phase model of interest development. Educ. Psychol. 41, 111–127. doi: 10.1207/s15326985ep4102_4
Historic England (2017). Photogrammetric Applications for Cultural Heritage: Guidance for Good Practice. Swindon: Historic England.
Historic England (2018). 3D Laser Scanning for Heritage: Advice and Guidance on the Use of Laser Scanning in Archaeology and Architecture. Swindon: Historic England.
HTC (2016). VIVE. Available at: https://www.vive.com/us/support/vive/
Jensen, L., and Konradsen, F. (2018). A review of the use of virtual reality head-mounted displays in education and training. Educ. Inf. Technol. 23, 1515–1529. doi: 10.1007/s10639-017-9676-0
Kpolovie, P. J., Joe, A. I., and Okoto, T. (2014). Academic achievement prediction. Role of interest in learning and attitude towards school. Int. J. Humanit. Soc. Sci. Educat. 1, 73–100.
Krapp, A. (2002). Structural and dynamic aspects of interest development. Theoretical considerations from an ontogenetic perspective. Learn. Instr. 12, 383–409. doi: 10.1016/S0959-4752(01)00011-1
Lee, E.-L., Wong, K. W., and Fung, C. C. (2010). How does desktop virtual reality enhance learning outcomes? A structural equation modeling approach. Comput. Educ. 55, 1424–1442. doi: 10.1016/j.compedu.2010.06.006
Makransky, G., and Petersen, G. B. (2019). Investigating the process of learning with desktop virtual reality: a structural equation modelling approach. Comput. Educ. 134, 15–30. doi: 10.1016/j.compedu.2019.02.002
Makransky, G., and Petersen, G. B. (2021). The Cognitive Affective Model of Immersive Learning (CAMIL): a theoretical research-based model of learning in immersive virtual reality. Educ. Psychol. Rev. 33, 937–958. doi: 10.1007/s10648-020-09586-2
Makransky, G., Petersen, G. B., and Klingenberg, S. (2020). Can an immersive virtual reality simulation increase students’ interest and career aspirations in science? Br. J. Educ. Technol. 51, 2079–2097. doi: 10.1111/bjet.12954
Makransky, G., Terkildsen, T. S., and Mayer, R. E. (2019). Adding immersive virtual reality to a science lab simulation causes more presence but less learning. Learn. Instr. 60, 225–236. doi: 10.1016/j.learninstruc.2017.12.007
McLellan, H. (2008). “Virtual realities,” in Handbook of Research for Educational Communications and Technology: A Project of the Association for Educational Communications and Technology. ed. D. H. Jonassen (New York: Taylor & Francis), 461–498.
McWhaw, K., and Abrami, P. C. (2001). Student goal orientation and interest: effects on students' use of self-regulated learning strategies. Contemp. Educ. Psychol. 26, 311–329. doi: 10.1006/ceps.2000.1054
Nebel, S., Beege, M., Schneider, S., and Rey, G. D. (2020). A review of photogrammetry and photorealistic 3D models in education from a psychological perspective. Front. Educ. 5:144. doi: 10.3389/feduc.2020.00144
Pellas, N., Dengel, A., and Christopoulos, A. (2020). A scoping review of immersive virtual reality in STEM education. IEEE Trans. Learn. Technol. 13, 748–761. doi: 10.1109/TLT.2020.3019405
Petersen, G. B., Klingenberg, S., Mayer, R. E., and Makransky, G. (2020). The virtual field trip: investigating how to optimize immersive virtual learning in climate change education. Br. J. Educ. Technol. 51, 2099–2115. doi: 10.1111/bjet.12991
Petersen, G. B., Petkakis, G., and Makransky, G. (2022). A study of how immersion and interactivity drive VR learning. Comput. Educ. 179:104429. doi: 10.1016/j.compedu.2021.104429
Radianti, J., Majchrzak, T. A., Fromm, J., and Wohlgenannt, I. (2020). A systematic review of immersive virtual reality applications for higher education: design elements, lessons learned, and research agenda. Comput. Educ. 147:103778. doi: 10.1016/j.compedu.2019.103778
Renkl, A., Mandl, H., and Gruber, H. (1996). Inert knowledge: analyses and remedies. Educ. Psychol. 31, 115–121. doi: 10.1207/s15326985ep3102_3
Renninger, K., Ewen, L., and Lasher, A. (2002). Individual interest as context in expository text and mathematical word problems. Learn. Instr. 12, 467–490. doi: 10.1016/S0959-4752(01)00012-3
Rodríguez-Aflecht, G., Jaakkola, T., Pongsakdi, N., Hannula-Sormunen, M., Brezovszky, B., and Lehtinen, E. (2018). The development of situational interest during a digital mathematics game. J. Comput. Assist. Learn. 34, 259–268. doi: 10.1111/jcal.12239
Rotgans, J. I., and Schmidt, H. G. (2011). The role of teachers in facilitating situational interest in an active-learning classroom. Teach. Teach. Educ. 27, 37–42. doi: 10.1016/j.tate.2010.06.025
Schiefele, U. (1991). Interest, learning, and motivation. Educ. Psychol. 26, 299–323. doi: 10.1080/00461520.1991.9653136
Schiefele, U., Krapp, A., and Winteler, A. (1992). “Interest as a predictor of academic achievement: a meta-analysis of research” in The Role of Interest in Learning and Development. eds. K. A. Renninger, S. Hidi, and A. Krapp (Hillsdale: Erlbaum), 183–212.
Schubert, T., Friedmann, F., and Regenbrecht, H. (2001). The experience of presence. Factor analytic insights. Presence. 10, 266–281. doi: 10.1162/105474601300343603
Schutte, N. S. (2020). The impact of virtual reality on curiosity and other positive characteristics. Int. J. Hum. Comput. Interact. 36, 661–668. doi: 10.1080/10447318.2019.1676520
Siklander, P., Kangas, M., Ruhalahti, S., and Korva, S. (2017). “Exploring triggers for arousing interest in the online learning,” in INTED2017 Proceedings: 11th International Technology, Education and Development Conference, ed. L. Gómez Chova, A. López Martínez, and I. Candel Torres Valencia (IATED), 9081–9089.
Škola, F., Rizvić, S., Cozza, M., Barbieri, L., Bruno, F., Skarlatos, D., et al. (2020). Virtual reality with 360-video storytelling in cultural heritage: study of presence, engagement, and immersion. Sensors 20:5851. doi: 10.3390/s20205851
Skulmowski, A., Nebel, S., Remmele, M., and Rey, G. D. (2021). Is a preference for realism really naive after all? A cognitive model of learning with realistic visualizations. Educ. Psychol. Rev. 34, 649–675. doi: 10.1007/s10648-021-09638-1
Tapola, A., Veermans, M., and Niemivirta, M. (2013). Predictors and outcomes of situational interest during a science learning task. Instr. Sci. 41, 1047–1064. doi: 10.1007/s11251-013-9273-6
The Blender Foundation (2021). Blender. Available at: https://www.blender.org/
Trebuňa, P., Mizerák, M., Trojan, J., and Rosocha, L. (2020). 3D scanning as a modern technology for creating 3D models. Acta Technol. 6, 21–24. doi: 10.22306/atec.v6i1.74
Unity Technologies (2020). Unity engine. Available at: https://unity.com/
Unity Technologies (2021). XR Interaction Toolkit. Available at: https://docs.unity3d.com/Packages/com.unity.xr.interaction.toolkit@0.9/manual/index.html
Velev, D., and Zlateva, P. (2017). Virtual reality challenges in education and training. Int. J. Learn. Teach. 3, 33–37. doi: 10.18178/ijlt.3.1.33-37
Verhagen, T., Feldberg, F., van den Hooff, B., Meents, S., and Merikivi, J. (2012). Understanding users’ motivations to engage in virtual worlds: a multipurpose model and empirical testing. Comput. Hum. Behav. 28, 484–495. doi: 10.1016/j.chb.2011.10.020
Vogt, A. G. (2021). Fostering deep learning in immersive virtual reality: Interplay of learner’s characteristics with internal and external support. dissertation. Universität Ulm. Available at: https://oparu.uni-ulm.de/xmlui/bitstream/handle/123456789/44276/Dissertation_Vogt.pdf?sequence=1&isAllowed=y
Waller, D., Hunt, E., and Knapp, D. (1998). The transfer of spatial knowledge in virtual environment training. Presence 7, 129–143. doi: 10.1162/105474698565631
Witmer, B. G., and Singer, M. J. (1998). Measuring presence in virtual environments: a presence questionnaire. Presence 7, 225–240. doi: 10.1162/105474698565686
Woerner, J. J. (1999). “Virtual field trips in the earth science classroom,” in Proceeding of the annual conference of the Association for the Education of Teachers in Science, ed. P. A. Rubba, J. A. Rye, and P. F. Keig (Greenville, NC), 1232–1244.
Keywords: authenticity, interest, virtual reality, photogrammetry, 3D modeling, presence, educational virtual environment, laser scanning
Citation: Fink MC, Sosa D, Eisenlauer V and Ertl B (2023) Authenticity and interest in virtual reality: Findings from an experiment including educational virtual environments created with 3D modeling and photogrammetry. Front. Educ. 8:969966. doi: 10.3389/feduc.2023.969966
Edited by:
Gergana Vladova, University of Potsdam, GermanyReviewed by:
Fernando Moreu, University of New Mexico, United StatesMalte Rolf Teichmann, University of Potsdam, Germany
Copyright © 2023 Fink, Sosa, Eisenlauer and Ertl. This is an open-access article distributed under the terms of the Creative Commons Attribution License (CC BY). The use, distribution or reproduction in other forums is permitted, provided the original author(s) and the copyright owner(s) are credited and that the original publication in this journal is cited, in accordance with accepted academic practice. No use, distribution or reproduction is permitted which does not comply with these terms.
*Correspondence: Maximilian C. Fink, Maximilian.Fink@unibw.de