- 1Faculty of Education, Beit Berl College, Kfar Saba, Israel
- 2IPN - Leibniz Institute for Science and Mathematics Education, Kiel, Germany
- 3Department of Learning, Teaching and Curriculum, College of Education and Human Development, University of Missouri, Columbia, MO, United States
- 4Department of Science and Technology Education, Ben Gurion University of the Negev, Beer Sheva, Israel
Editorial on the Research Topic
Investigating complex phenomena: bridging between systems thinking and modeling in science education
1. Introduction
Complexity is all around us, from sub-atomic particles to distant galaxies. Modern technology and knowledge provide us with incredible abilities to investigate the complexity of systems of various kinds, such as human societies, biological ecosystems, chemical reactions, and physical interactions between energy and matter. In recent years, complexity has become prominent in most human local and global challenges, such as climate change, pandemic outbreaks, and sustainable energy sources. Understanding the complexity of phenomena is essential for scientific reasoning and sense-making, problem-solving in STEM, and technological tools development. Complexity pushes us beyond the dichotomic determinism of black-and-white classification and simple, straightforward solutions. It allows us to examine systems from different perspectives, explore alternative explanations, and keep an open mind while investigating phenomena—all of which lays at the heart of good critical thinking.
Certain key competencies are crucial for engaging constructively and responsibly with today's complexity challenges, including systems thinking and modeling. For instance, competency in systems thinking competency is suggested as one of the eight key competencies for sustainability (UNESCO, 2017). Most conceptualizations of systems thinking in science education encompass the ability of “system modeling” (e.g., Schuler et al., 2018; Mambrey et al., 2020), emphasizing the importance of modeling as a scientific practice for investigating and understanding complex phenomena (Passmore et al., 2017). Systems thinking and modeling are important competencies that provide students with essential tools when investigating complex phenomena and solving complex real-world problems. A recent literature review and bibliometric analysis reported a sharp increase in the number of studies about systems thinking in STEM education since 2016 (Bielik et al., 2023). However, most of the identified studies focused on higher education, while only a few focused on teachers or elementary students. There was also an increase in systems thinking studies, particularly on how the use of digital tools and modeling support system thinking competencies. This trend in studies about systems thinking and modeling in STEM education indicates a growing interest of the research community in these issues and the relevance of it for the complexity of today's challenges and problems.
Modeling is a key process of understanding complex phenomena as systems (Godfrey-Smith, 2006; Leonelli, 2007). Hence, it can be assumed that explicit knowledge about systems and system characteristics, such as metacognitive level awareness of how and why phenomena are conceptualized as systems, is beneficial for developing an initial system model and for deducing hypotheses related to specific structures or processes of the system (Verhoeff et al., 2008). Therefore, it can be further assumed that systems thinking and modeling are critical for science education in particular, and for STEM education in general when investigating complex phenomena. However, this mutually supportive relationship between systems thinking and modeling has not yet been deeply investigated in science education.
This Research Topic aims to advance current research focusing on bridging systems thinking and modeling when investigating complex phenomena in science education. It includes a set of studies that provide science education researchers, practitioners, and decision-makers with in-depth analyses and insightful findings that can promote our understanding of how to improve teaching and learning about complex phenomena and how to support students' systems thinking and modeling when engaging with complex phenomena in their science classrooms.
2. Theoretical background
2.1. Systems thinking in science education
In science education, systems thinking is generally defined as an approach to understand, explain, and interpret complex and dynamic phenomena, a learning strategy that explicitly considers system characteristics to explain and predict natural phenomena (Verhoeff et al., 2018). Systems thinking can be defined as the ability to recognize, describe, and model a complex phenomenon in its structure, behavior, and function as a system, including the metacognitive awareness about systems and system characteristics (Verhoeff et al., 2008; Riess and Mischo, 2010). Systems thinking is widely acknowledged as an important goal in science education that is necessary for “developing coherent understanding of complex biological processes and phenomena” (Verhoeff et al., 2018, p. 1). Three generally agreed-upon central systems thinking skills are proposed in the literature: identifying system organization, analyzing system behavior, and system modeling (Ben-Zvi-Assaraf and Orion, 2010; Mehren et al., 2018; Schuler et al., 2018; Mambrey et al., 2020).
Recent studies suggest three effective strategies for fostering systems thinking skills: modeling, cross-level reasoning, and use of systems language. For example, Rachmatullah and Wiebe (2022) found that computational modeling significantly improved middle school students' understanding of food web concepts and systems thinking. Düsing et al. (2019) found that when students were presented with integrative cases, where all levels of organization are considered through matter and energy transfers, they developed their cross-level reasoning ability. According to Krist et al. (2019), thinking across levels allows students to explain and make predictions about phenomena, and implicitly support mechanistic reasoning. Other studies showed that exposure to systems language helps students deconstruct a phenomenon to its characteristics and support the discussion on how patterns emerge from the interactions among system components (Gilissen et al., 2021; Nguyen and Santagata, 2021; Momsen et al., 2022).
2.2. Models and modeling in science education
Models are defined as epistemic tools for investigating and making sense of phenomena (Knuuttila, 2011). The developed model has to be evaluated for internal consistency and adequate representation of what was observed (Frigg and Hartmann, 2017). The model should allow the modeler to deduce predictions about how the system should behave under certain conditions by mentally or materially manipulating the model (Giere et al., 2006). These predictions can be tested by conducting empirical investigations. If the predictions turn out to be false, it is likely that the model is not accurate and should be rejected or revised and retested in an iterative cyclic process (Göhner and Krell, 2020).
Modeling competency is the ability to engage in the process of developing and using models for reasoning in science (Nicolaou and Constantinou, 2014; Upmeier zu Belzen et al., 2019). Hence, modeling is mostly defined as a procedural and epistemological competency (Upmeier zu Belzen et al., 2019). There is a wide consensus that developing modeling competency is an important goal of science education (Passmore et al., 2014; Chiu and Lin, 2019). When achieving modeling competency, students are expected to understand scientific concepts better, develop an appreciation of the nature of science, and advance in their mastery of the scientific process (Gilbert and Justi, 2016). Modeling has been identified as a key competency for investigating complex phenomena and developing hypothetical explanations and reasoning abilities (Passmore et al., 2017; Zangori et al., 2017). However, most studies in science education propose that students and teachers struggle with understanding models as hypothetical entities and research tools but rather hold representational views on models (Krell and Krüger, 2016; Gouvea and Passmore, 2017). When engaging in modeling for reasoning, one major challenge is the need for prior experiences and conceptual understanding of the investigated phenomenon on which a model can be built (Ruppert et al., 2017; Göhner et al., 2022).
2.3. Bridging between systems thinking and modeling when investigating complex phenomena
Systems thinking is conceptualized as a specific form of knowledge organization that allows a coherent understanding of complex phenomena (Verhoeff et al., 2018), while modeling is seen as a procedural and epistemological competency (Upmeier zu Belzen et al., 2019). Passmore et al. (2017) emphasize that the essence of modeling is to figure out “the behavior of systems in the natural and designed world” (p. 113). Developing system models is important for achieving advanced systems thinking (Hung, 2008; Verhoeff et al., 2008; Gilissen et al., 2020). For example, Hung (2008) showed that digital system modeling supported university students' systems thinking. Generally, computer modeling is suggested to be a powerful tool to support systems thinking by highlighting the central components of a system, making systems mechanisms tangible, easily running simulations to examine possible emerging outcomes, and helping students to grasp complex relationships within a system (Damelin et al., 2017; Bielik et al., 2021; Nguyen and Santagata, 2021).
From a cognitive psychology perspective, systems thinking and modeling are connected by their shared reliance on the concept of mental models. Mental models are internal cognitive representations of ideas, events, objects, or systems, which humans draw upon when generating external representations. These mental models result from an internal modeling process that includes constructing new information upon existing knowledge to build a stable model (Johnson-Laird, 2004). Goldstone and Wilensky (2008) describe the connection between modeling and complex phenomena, noting that developing a model of a situation requires to ground the interpretation of its components and extract a general principle from the situation. For the model to work, the mechanisms through which system components interact must be modeled. Godfrey-Smith (2006) describes the strategy of modeling (in biology) as gaining an understanding of a complex real-world phenomenon through investigating a simpler, hypothetical system (i.e., a model) that resembles it in selected aspects.
3. The contributions to this Research Topic
Several contributions to this Research Topic focus primarily on systems thinking when exploring complex phenomena (e.g., Bielik et al.; Sabel et al.; Tamir et al.). For example, Tamir et al. explored high school students' conceptualization of complexity while designing, assembling, and testing a nanosatellite. Findings show that the broader the participants' involvement was, the greater the progress they experienced in their systems thinking. Participants who stayed focused on a single subsystem of the nanosatellite did not show progress, while participants who involved themselves with several subsystems exhibited a more meaningful progress. The challenge of a multidimensional lens in students' understanding of complex systems was also found by Sabel et al., who pointed to students' difficulty in considering both natural and societal aspects of systems. They investigated undergraduate students' engagement in systems thinking and modeling using causal maps, focusing on identifying the factors that undergraduate students prioritize when considering causal relationships within an ecosystem. Although humans and human-related factors were included in the assignment picture, few students included human-related causes and effects in their causal maps or in the answers to the questions following the causal maps.
Other contributions in this Research Topic focus mostly on students' reasoning with models when exploring complex phenomena (e.g., Eidin et al.; Engelschalt et al.; Ryan et al.). For instance, Ryan et al. explored how computational tools mediated middle school students' mechanistic reasoning. They report that, as students interacted with the computational tools, their mechanistic reasoning about their models increased in complexity. Eidin et al. also expand research in a similar vein, exploring how different kinds of computational modeling experiences support secondary students' development of complex causal reasoning structures. The authors suggest “a system dynamics approach has the potential to encourage a more complex causal scheme of the phenomenon which the static equilibrium model was unable to support” (Eidin et al., p. 16). Engelschalt et al. explored the role of abductive reasoning when undergraduate students modeled complex systems. Their study participants used components of abductive reasoning in constructing models of biological systems, but using those components did not necessarily lead to generating scientific explanations. The authors suggest that individual may need an “interplay between abductive reasoning and systems thinking skills such as cross-level reasoning” (Engelschalt et al., p. 13) in which individuals may need to develop both systems thinking skills and cross level reasoning to consider causal relationships across system time and space.
Using computational models when exploring complex phenomena was another aspect investigated in several contributions in this Research Topic (e.g., Eidin et al.; Langbeheim et al.). These studies provided students and teachers with opportunities to engage with different modeling tools such as NetLogo (Langbeheim et al.) and SageModeler (Eidin et al.). For example, Langbeheim et al. found that participatory computational simulation can support 9th grade students' explanations of crowd evacuation counterintuitive required behavior provided students having prior opportunity to engage with a participatory simulation in a different context.
Finally, several publications explored the connection between systems thinking and modeling when exploring complex phenomena (e.g., Ke et al.; Lankers et al.; Peretz et al.). For example, Peretz et al. investigated the effect of an interdisciplinary online course on the development of pre- and in-service science and engineering teachers' systems thinking and modeling competency. Based on the findings of their qualitative case study, the authors propose that teachers need scaffolding to gain systems-related ontological knowledge (e.g., understanding systems language) before being able to apply this knowledge—as previously suggested to foster competency development in science education in general (e.g., Krell et al., 2023). Miller and Yoon developed modeling units on biological concepts (e.g., gene regulation) for students. They investigated how students' understanding of biological models influenced their understanding of complex systems. A regression analysis proposed that growth in students' meta-modeling knowledge predicts growth in complex systems understanding. For instance, a better understanding of the purpose of models provided students “with strategies to interpret data generated from multiple runs and to develop explanations of the system” (Miller and Yoon, p. 13–14). Similarly, students developed more elaborate theories about the investigated phenomena when understanding the dynamic and changeable nature of models because this led to more model manipulations.
4. Summary
Systems thinking and modeling are two intertwined competencies that support students when investigating complex phenomena. On the one hand, modeling is a procedural and epistemological competency that allows to develop and evaluate systems (Passmore et al., 2017). It is significantly supported by a coherent understanding of the respective phenomenon (Ruppert et al., 2017). On the other hand, systems thinking is viewed as a content-related competency (Verhoeff et al., 2018), which encompasses the ability of system modeling (Mambrey et al., 2020). However, there is still much to be researched about how these two competencies are empirically interconnected and how they interact when engaging students and science teachers when investigating complex phenomena.
In Figure 1, we present a model for the system modeling process, as reflected in the literature and the contributions to this Research Topic. In this model, a system model is developed, evaluated, and revised to represent and make sense of selected relevant parts of the investigated complex phenomenon. The system model comprises of system characteristics (e.g., structures, processes, interactions, boundaries, and emergent states), represented by different shapes and arrows in Figure 1. This model demonstrates the connection between systems thinking and modeling when investigating complex phenomena.
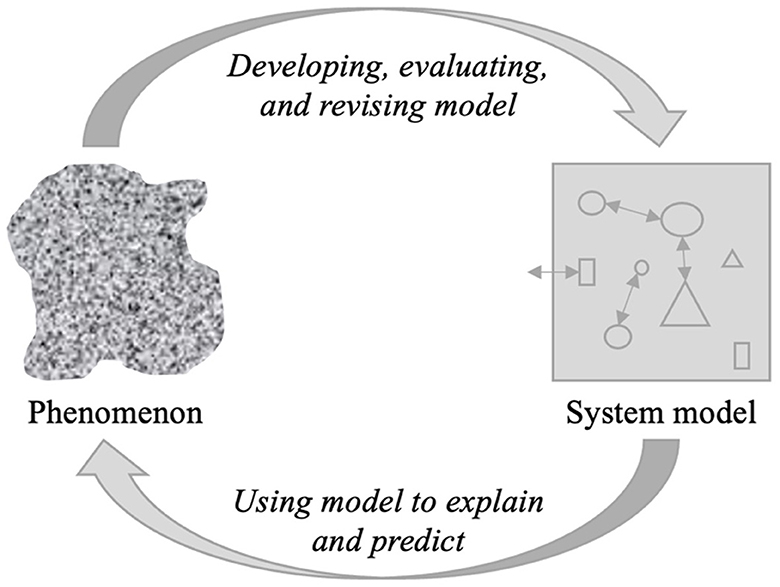
Figure 1. Idealized model of the cyclic and iterative system modeling process (based on Giere et al., 2006 and Göhner and Krell, 2020). A system model is developed, evaluated, and revised to represent selected relevant parts of the investigated complex phenomenon. The system model comprises of system characteristics (structures, processes, interactions, boundaries, and emergent states) represented by different shapes and arrows.
In summary, this Research Topic provides a collection of contributions focusing on modeling, systems thinking, and the connections between them when investigating complex phenomena. The contributions range from middle school to undergraduate students and pre- and in-service teachers, focusing on all science and engineering disciplines. We hope these contributions will further advance the understanding and promote the discussion among science education researchers, practitioners, and decision-makers regarding how to support teachers and students when engaging with complex phenomena.
Author contributions
TB: Conceptualization, Writing—original draft. MK: Conceptualization, Writing—original draft. LZ: Conceptualization, Writing—original draft. OB: Conceptualization, Writing—original draft.
Funding
The author(s) declare that no financial support was received for the research, authorship, and/or publication of this article.
Conflict of interest
The authors declare that the research was conducted in the absence of any commercial or financial relationships that could be construed as a potential conflict of interest.
Publisher's note
All claims expressed in this article are solely those of the authors and do not necessarily represent those of their affiliated organizations, or those of the publisher, the editors and the reviewers. Any product that may be evaluated in this article, or claim that may be made by its manufacturer, is not guaranteed or endorsed by the publisher.
References
Ben-Zvi-Assaraf, O., and Orion, N. (2010). Four case studies, six years later: Developing system thinking skills in junior high school and sustaining them over time. J. Res. Sci. Teach. 47, 1253–1280. doi: 10.1002/tea.20383
Bielik, T., Dalen, I., Krell, M., and Ben-Zvi Assaraf, O. (2023). Characterising the literature on the teaching and learning of systems thinking and complexity in STEM education: a bibliometric analysis and research synthesis. J. STEM Educ. 6, 199–231. doi: 10.1007/s41979-023-00087-9
Bielik, T., Stephens, L., McIntyre, C., Damelien, D., and Krajcik, J. (2021). Supporting student system modelling practice through curriculum and technology design. J. Sci. Educ. Technol. 31, 217–231. doi: 10.1007/s10956-021-09943-y
Chiu, M. H., and Lin, J. W. (2019). Modeling competence in science education. Discipl. Interdisc. Sci. Educ. Res. 12, 1–11. doi: 10.1186/s43031-019-0012-y
Damelin, D., Krajcik, J., Mcintyre, C., and Bielik, T. (2017). Students making systems models. Sci. Scope 40, 78–82. doi: 10.2505/4/ss17_040_05_78
Düsing, K., Asshoff, R., and Hammann, M. (2019). Students' conceptions of the carbon cycle: identifying and interrelating components of the carbon cycle and tracing carbon atoms across the levels of biological organisation. J. Biol. Educ. 53, 110–125. doi: 10.1080/00219266.2018.1447002
Frigg, R., and Hartmann, S. (2017). Models in Science. Stanford, CA: Stanford Encyclopedia of Philosophy.
Giere, R. N., Bickle, J., and Mauldin, R. F. (2006). Understanding Scientific Reasoning. Fort Worth, TX, USA: Wadsworth Publishing Company.
Gilbert, J., and Justi, R. (2016). Modelling-Based Teaching in Science Education. Cham: Springer. doi: 10.1007/978-3-319-29039-3
Gilissen, M., Knippels, M.-C., and van Joolingen, W. (2020). Bringing systems thinking into the classroom. Int. J. Sci. Educ. 42, 1253–1280. doi: 10.1080/09500693.2020.1755741
Gilissen, M. G., Knippels, M. C. P., and van Joolingen, W. R. (2021). Fostering students' understanding of complex biological systems. CBE—Life Sci. Educ. 20, ar37. doi: 10.1187/cbe.20-05-0088
Godfrey-Smith, P. (2006). The strategy of model-based science. Biol. Philos. 21, 725–740. doi: 10.1007/s10539-006-9054-6
Göhner, M., Bielik, T., and Krell, M. (2022). Investigating the dimensions of the modeling competence among pre-service science teachers. J. Res. Sci. Teach. 59, 1354–1387. doi: 10.1002/tea.21759
Göhner, M., and Krell, M. (2020). Preservice science teachers' strategies in scientific reasoning: The case of modeling. Res. Sci. Educ. 52, 395–414. doi: 10.1007/s11165-020-09945-7
Goldstone, R., and Wilensky, U. (2008). Promoting transfer by grounding complex systems principles. J. Learn. Sci. 17, 465–516. doi: 10.1080/10508400802394898
Gouvea, J., and Passmore, C. (2017). ‘Models of' versus ‘Models for'. Sci. Educ. 26, 49–63. doi: 10.1007/s11191-017-9884-4
Hung, W. (2008). Enhancing systems-thinking skills with modelling. Br. J. Educ. Technol. 39, 1099–1120. doi: 10.1111/j.1467-8535.2007.00791.x
Johnson-Laird, P. (2004). “The history of mental models,” in Psychology of Reasoning, eds. K. Manktelow and M. C. Chung (New York, NY: Psychology Press), 179–212.
Knuuttila, T. (2011). Modelling and representing: An artefactual approach to model-based representation. Stud. History Philos. Sci. A 42, 262–271. doi: 10.1016/j.shpsa.2010.11.034
Krell, M., Khan, S., Vergara, C., Cofré, H., Mathesius, S., and Krüger, D. (2023). Pre-service science teachers' scientific reasoning competencies: analysing the impact of contributing factors. Res. Sci. Educ. 53, 59–79. doi: 10.1007/s11165-022-10045-x
Krell, M., and Krüger, D. (2016). Testing models. J. Biol. Educ. 50, 160–173. doi: 10.1080/00219266.2015.1028570
Krist, C., Schwarz, C. V., and Reiser, B. J. (2019). Identifying essential epistemic heuristics for guiding mechanistic reasoning in science learning. J. Learn. Sci. 28, 160–205. doi: 10.1080/10508406.2018.1510404
Leonelli, S. (2007). “What is in a model?” in Modeling biology: Structures, behavior, evolution, eds. M. Laubichler and G. Müller (London: The MIT Press), 15–35.
Mambrey, S., Timm, J., Landskron, J. J., and Schmiemann, P. (2020). The impact of system specifics on systems thinking. J. Res. Sci. Teach. 57, 1632–1651. doi: 10.1002/tea.21649
Mehren, R., Rempfler, A., Buchholz, J., Hartig, J., and Ulrich-Riedhammer, E. (2018). System competence modelling. J. Res. Sci. Teach. 55, 685–711. doi: 10.1002/tea.21436
Momsen, J., Speth, E. B., Wyse, S., and Long, T. (2022). Using systems and systems thinking to unify biology education. CBE—Life Sci. Educ. 21, 1–11. doi: 10.1187/cbe.21-05-0118
Nguyen, H., and Santagata, R. (2021). Impact of computer modeling on learning and teaching systems thinking. J. Res. Sci. Teach. 58, 661–688. doi: 10.1002/tea.21674
Nicolaou, C., and Constantinou, C. (2014). Assessment of the modeling competence. Educ. Res. Rev. 13, 52–73. doi: 10.1016/j.edurev.2014.10.001
Passmore, C., Gouvea, J., and Giere, R. (2014). “Models in science and in learning science,” in International Handbook of Research in History, Philosophy and Science Teaching, ed. M. Matthews (Cham: Springer), 1171–1202. doi: 10.1007/978-94-007-7654-8_36
Passmore, C., Schwarz, C., and Mankowski, J. (2017). “Developing and using models,” in Helping Students Make Sense of the World Using Next Generation Science and Engineering Practices, eds. C. Schwarz, C. Passmore and B. Reiser (Arlington VA: NSTA Press), 109–134.
Rachmatullah, A., and Wiebe, E. N. (2022). Building a computational model of food webs: Impacts on middle school students' computational and systems thinking skills. J. Res. Sci. Teach. 59, 585–618. doi: 10.1002/tea.21738
Riess, W., and Mischo, C. (2010). Promoting systems thinking through biology lessons. Int. J. Sci. Educ. 32, 705–725. doi: 10.1080/09500690902769946
Ruppert, J., Duncan, R. G., and Chinn, C. A. (2017). Disentangling the role of domain-specific knowledge in student modeling. Res. Sci. Educ. 49, 921–948. doi: 10.1007/s11165-017-9656-9
Schuler, S., Fanta, D., Rosenkraenzer, F., and Riess, W. (2018). Systems thinking within the scope of education for sustainable development (ESD). J. Geogr. High. Educ. 42, 192–204. doi: 10.1080/03098265.2017.1339264
UNESCO (2017). Education for sustainable development goals: Learning objectives. UNESCO. doi: 10.54675/CGBA9153
Upmeier zu Belzen, A., Krüger, D., and van Driel, J. H. (2019). Towards a Competence-Based View on Models and Modeling in Science Education. Berlin: Springer. doi: 10.1007/978-3-030-30255-9
Verhoeff, R., Knippels, M.-C., Gilissen, M., and Boersma, K. (2018). The theoretical nature of systems thinking. Front. Educ. 3, 518. doi: 10.3389/feduc.2018.00040
Verhoeff, R., Waarlo, A., and Boersma, K. (2008). Systems modelling and the development of coherent understanding of cell biology. Int. J. Sci. Educ. 30, 543–568. doi: 10.1080/09500690701237780
Keywords: systems thinking, complexity, modeling, science education, science technology engineering mathematics (STEM)
Citation: Bielik T, Krell M, Zangori L and Ben Zvi Assaraf O (2023) Editorial: Investigating complex phenomena: bridging between systems thinking and modeling in science education. Front. Educ. 8:1308241. doi: 10.3389/feduc.2023.1308241
Received: 06 October 2023; Accepted: 17 October 2023;
Published: 27 October 2023.
Edited and reviewed by: Lianghuo Fan, East China Normal University, China
Copyright © 2023 Bielik, Krell, Zangori and Ben Zvi Assaraf. This is an open-access article distributed under the terms of the Creative Commons Attribution License (CC BY). The use, distribution or reproduction in other forums is permitted, provided the original author(s) and the copyright owner(s) are credited and that the original publication in this journal is cited, in accordance with accepted academic practice. No use, distribution or reproduction is permitted which does not comply with these terms.
*Correspondence: Tom Bielik, dG9tLmJpZWxpa0BiZWl0YmVybC5hYy5pbA==