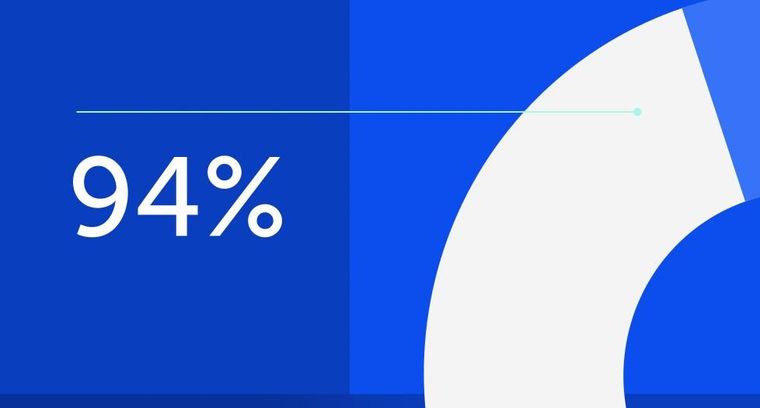
94% of researchers rate our articles as excellent or good
Learn more about the work of our research integrity team to safeguard the quality of each article we publish.
Find out more
REVIEW article
Front. Educ., 23 November 2023
Sec. Digital Education
Volume 8 - 2023 | https://doi.org/10.3389/feduc.2023.1290287
The practical teaching or training of enzymatic proteolysis can prove challenging because of the lengthy duration of the process, the complexity of identifying short amino acid sequences, the high cost of the enzymes, and the need to use very specific equipment. There are several freely-available online tools that, despite being employed by scientists to help identify bioactive peptides, are not commonly used for teaching and training activities. This work summarises the most common protein and peptide databases along with other tools that allow one to simulate enzymatic hydrolysis of a given protein and to study the structure, physicochemical properties, bioactivity, toxicity, allergenicity, and even the bitterness of the resulting peptides. Overall, in silico tools can be used during the teaching and training of chemical engineers as innovative alternatives to conventional laboratory work and theoretical classes.
Proteins are large macromolecules made up of amino acids. They are the building blocks of life and are needed by all living things to function properly. In humans, for example, proteins are the basis of body structures, act as messengers, maintain the proper pH, transport and store nutrients, provide energy, and are responsible for most biochemical reactions. At the industrial level, proteins are used in many areas. For example, proteins are one of the key ingredients in many human foods and most animal feeds, amino acids are common agricultural products, and enzymes (proteins) are common ingredients in the food industry (e.g., baked goods and drinks); they are also widely used during the production of pulp, paper, and leather.
For most commercial protein applications, it is important to retain the protein structure given that their activity is structure dependent. However, other protein applications and properties are enhanced after the protein structure of the protein is disrupted. For example, the cleavage of proteins might result in the release of bioactive fragments or might improve the techno-functional properties of proteins with poor functionality (Vogelsang-O’dwyer et al., 2022). Bioactive peptides are short amino acid sequences that have biological effects when they are released from their parent proteins. Most of the bioactive peptides reported to date are of between 2 and 20 amino acids in length and several have been shown to have different modes of action and to exert more than one form of bioactivity (Ulug et al., 2021). Enzymatic hydrolysis is the most widely used technology to produce bioactive peptides, usually from food sources. This process is carried out under controlled conditions (ideally under the optimal ones for the enzyme being used) and is generally preferred to microbial fermentation because of its high specificity. To obtain quality products with high bioactivity, it is essential to understand the enzyme cleavage sites and the specificity of the bioactive peptides as well as to apply adequate process control. This knowledge is key for chemical engineers and food engineers working in the field of bioactive peptide generation. The use of enzymes in industrial processes is covered by different subjects comprising most chemical engineering curricula, for example, Bioreactors, Reactor Design, Reaction Kinetics, Biochemical Engineering, and Chemistry. The use of in silico strategies, meaning strategies conducted via computer simulations, has become increasingly important in the field and has been used to predict toxic (Gupta et al., 2013), antiviral (Charoenkwan et al., 2020), cell penetrating (Gautam et al., 2013), antihypertensive (Kumar et al., 2015), and antidiabetic (Lafarga et al., 2014) peptides, amongst other bioactivities. Moreover, being able to predict the cleavage of a given protein by means of a computer is also useful for estimating the potential bioavailability of amino acids upon gastrointestinal digestion (Sayd et al., 2018). Because of their potential to save both time and money, in silico tools have gained increasing importance in the scientific literature. The practical teaching or training on enzymatic proteolysis can be challenging because of the long duration of the process, the complexity of identifying short amino acid sequences, the high cost of enzymes, and the need to use of very specific equipment including liquid chromatographs and mass spectrometers. For these reasons, at most universities, the teaching of enzymatic proteolysis is generally theoretical and training in this topic is rare. The use of computer simulations in training is also beneficial as they give the students the time necessary to understand the concept (Rodrigues et al., 2010).
Over the last decade, several freely available online tools have been developed that are now being implemented by scientists all around the world. Such tools can also be used to teach and facilitate the understanding of proteins, bioactive peptides, and proteolysis. The results have a strong graphic impact and allow the rapid simulation of reactions and processes that would otherwise be impossible in a training environment. Despite being common in scientific environments, in silico tools are not yet widely implemented in teaching and training activities. For this reason, the goal of our review is to summarise the main online tools that can potentially be used in educational and training environments although they are currently only being used by scientists to simulate enzymatic hydrolysis and the release of bioactive peptides. The most comprehensive protein and bioactive peptide databases will be explained together with various tools that enable enzymatic proteolysis to be estimated and understood. Other tools that predict bioactivity, toxicity, or allergenicity will also be discussed suggesting, with tips on their use in science lessons. These tools could be introduced into various Undergraduate and Masters’ Degrees, including Chemistry, Chemical Engineering, Food Science, and Biotechnology. A recent work revealed that chemical engineering students were very satisfied with simulator-based learning approaches (Borreguero et al., 2019; Roman et al., 2020). Furthermore, professors teaching a range of subjects (for example, Organic Chemistry, Biochemistry, Bioreactors, Bioprocesses, Food Engineering, and Biotechnology), could benefit from these easy-to-use and freely available tools.
The UniProt database, available at https://www.uniprot.org, is a collection of protein sequences covering more than 120 million proteins (Bateman, 2019). This database is the result of a collaboration between the European Bioinformatics Institute, the SIB Swiss Institute of Bioinformatics, and the Protein Information Resource. It counts on the participation of over 100 people who oversee database curation and software development, and who give support. This tool could be used by students to search for protein sequences (and much more) using the central “Find your protein” search bar; it also supports advanced searches that allow keywords and taxonomies, etc. to be included (Figure 1). We searched for bovine serum albumin (P02769, ALBU_BOVIN) and were immediately directed to a vast amount of information including publications related to the protein, its amino acid sequence, 3D structure, subcellular location, potential allergenic properties, features, and function in the body (Figure 2). This information is very useful for students and facilitates access to scientific information on any given protein. The tool also searches for related proteins, which are either obtained from the same organism (e.g., bovine fibrinogen) or a related one (e.g., porcine serum albumin).
Figure 2. (A) Function and (B) cellular location of bovine serum albumin. Data accessed from UniProt, available at https://www.uniprot.org on 30/04/2023.
UniProt includes other tools, for example, BLAST, which stands for Basic Local Alignment Search Tool, which finds regions of local similarity between protein sequences (Zaru and Orchard, 2023). In the case of BSA (ALBU_BOVIN), the software was able to identify many similar sequences including sheep serum albumin (ALBU_SHEEP, 92.4% similarity), porcine serum albumin (ALBU_PIG, 79.9% similarity), cat serum albumin (ALBU_CAT, 78.5% similarity), and dog serum albumin (ALBU_CANLF, 76.6% similarity). The similarity between the amino acid sequence of bovine and human serum albumin (ALBU_HUMAN) was 76.6%. This tool allows the user, in this case students, to estimate the release of bioactive peptides from a given protein source based on the similarity of the protein to a known source of bioactive peptides. This is useful when estimating the differences that can occur in the final product if the raw material (the protein source) is changed to a similar one. This tool can be complemented with the Align tool, available at https://www.uniprot.org/align/, which allows two or more protein sequences to be aligned and to observe where the main difference occur (Figure 3). This option can identify which peptides will be different if the protein source is changed, offering a possible key to a process in which not all the peptides generated are of interest.
Figure 3. Comparison between bovine and human serum albumin using the Align tool at https://www.uniprot.org/align.
The Peptide Search option (available at UniProt) is also very useful because it allows one to search for a known bioactive peptide from the proteins available in the database. For example, the tripeptides IPP and VPP are known to attenuate the development of hypertension and may also have beneficial effects on vascular function (Turpeinen et al., 2009). Both peptides were identified for the first time from fermented milk proteins although they are present in other protein sequences as well. We used the Peptide Search option to look for protein that include the IPP sequence. The software was able to identify almost 1,000,000 proteins that contain this peptide sequence, suggesting that its production might not be limited to milk proteins. As an example, Peptide Search identified the IPP sequence in the proteins FOGA_ASPRC, FRA17_FRAAN, and LHY_PETHY, which are found in Aspergillus ruber, Fragaria ananassa (strawberry), and Petunia hybrida.
The Research Collaboratory for Structural Bioinformatics Protein Data Bank (RCSB PDB) is available at http://rcsb.org/ (Rose et al., 2016). The webpage includes public data with no usage limitations and offers several tools for structure query, analysis, and visualisation. In terms of analysis, the webpage offers different tools including: (i) Pairwise Structure Alignment, which allows one to calculate pairwise structure alignments using different methods, (ii) Structure Quality, which shows a slider graphic that compares important global quality indicators for a given structure, and (iii) Symmetry Resources, which include exploratory tools that display global, local, and helical symmetry amongst subunits. Users can search using the top menu bar by introducing the name of the protein or the source organism, or even build complex search combinations with the “Advanced Search” interface. The platform also allows one to search for scientific publications related to a given protein and access and download valuable information. As an example, we searched for bovine serum albumin (BSA), which is often used as a protein standard in laboratory experiments. BSA is also a rich source of bioactive peptides (Lafarga et al., 2017) and a relatively small protein, thus facilitating its study. The protein is classified in RCSB PDB as a transport protein included as part of the Bos taurus organism. Besides the protein’s amino acid sequence, this online tool enables one to access the literature related to the protein and the experimental data available on it, including its structure validation, a 3D structural representation, and ligand interactions, amongst other useful information (Figure 4). The RCSB PDB is a simple tool that can be used by students to access amino acid sequences when carrying out in silico simulations, as described in the following sections. Furthermore, RCSB PDB includes educational resources including training courses, guides, and other free resources to promote the exploration of the world of proteins (e.g., colouring books, 3D printing models, paper models, flyers, and posters).
Figure 4. (A) 3D structure, (B) ligand interaction, and (C) amino acid sequence of bovine serum albumin. Data accessed from RCSB PDB at https://www.rcsb.org on 30/04/2023.
There are other protein databases that contain thousands of sequences. These include PDBe (Protein Data Bank in Europe) available at https://www.ebi.ac.uk/pdbe/and wwPDB (Worldwide Protein Data Bank), available at https://www.wwpdb.org. The goal of the latter keep data and metadata for biological macromolecules freely accessible to promote basic and applied research and education. Other online protein databases include the NIH protein database of the National Library of Medicine (USA), available at https://www.ncbi.nlm.nih.gov/protein, where protein sequences and different tools can be accessed. All these protein databases, together with the ones mentioned above, allow many different processes to be simulated; these would otherwise be extremely complex to study in a laboratory. For example, working online allows one to simulated processes using raw materials in a location where they are uncommon or not unavailable raw materials are used in a location where they are uncommon or are not available.
Expasy is the bioinformatics resource portal of the SIB Swiss Institute of Bioinformatics. It is a comprehensive portal that provides access to over 160 databases and online software tools to support different scientific activities including proteomics and medical chemistry (Duvaud et al., 2021). The following section discusses several useful tools that are available via the Expasy portal. PeptideCutter1 predicts potential cleavage sites cleaved by proteases in a given protein sequence. This can be accessed either via an amino acid sequence or an identified protein such as ALBU_BOVIN. The software allows one to select an enzyme from a list of various commercially available proteases and returns the query sequence with the possible cleavage sites, as shown in Figure 5A. The tool includes a summary of the cleavage sites for the different enzymes selected. These options offer great potential for use in teaching activities, especially since the cleavage sites of a specific enzyme are key to obtaining the desired peptide fractions. Furthermore, these tools allow one to observe the differences between enzymes as a graphic presentation. For example, for the enzyme Thermolysin (EC 3.4.24.27), the Expasy portal reads “Thermolysin preferentially cleaves sites with bulky and aromatic residues (Ile, Leu, Val, Met, Phe) in position P1’. Cleavage is favoured with aromatic sites in position P1 but hindered with acidic residues in position P1. Pro blocks when located in position P2’ but not when found in position P1” (see Figure 5B). PeptideCutter can be used together with PeptideMass, also developed by the SIB Swiss Institute of Bioinformatics and available at https://www.expasy.org/resources/peptidemass. This tool enables one to cleave a protein sequence with a chosen enzyme and computes the masses and amino acid sequences of the generated peptides. The user can select a protein sequence by either introducing the entire amino acid sequence or entering the UniProt protein identifier ID (e.g., ALBU_BOVIN). The user can select different options including the protease being tested, the maximum number of missed cleavages permitted, ot the size of the peptides shown, as one might be interested in either small or big peptides, but not both (Figure 6).
Figure 5. (A) Estimation via PeptideCutter of the cleavage sites of bovine serum albumin using thermolysin. (B) Schematic representation of enzyme-substrate complex with eight binding sites according to Schechter and Berger (1968).
Figure 6. Peptides released after the in silico cleavage of bovine serum albumin using thermolysin. Estimation carried out using PeptideMass, available at https://www.expasy.org/resources/peptidemass.
DeepDigest predicts protein cleavage using deep learning (Yang et al., 2021). It was designed to discriminate between correct and incorrect peptide identification in analytical determination but can also be used to help select proteases, not just for shotgun proteomic experiments but also for the release of bioactive peptides. In addition, the RPG Rapid Peptides Generator is software dedicated to predicting the cleavage sites of different enzymes on a given protein. It is a python tool that follows the standards for software development with continuous Gitlab integration.2 One interesting aspect of this tool is that the hydrolysis can be carried out either in concurrent mode, assuming that several enzymes are available at the same time in the reactor, or in sequential mode, where the protein will be digested by the different enzymes one by one. Both methods are commonly used in the scientific literature (Lu et al., 2010; Lafarga et al., 2016). The option of selecting a concurrent mode is not available in other tools. The RPG Rapid Peptides Generator currently incorporates 43 different enzymes (and chemicals) including bromelain (EC 3.4.22.33) and papain (EC 3.4.22.2), which are not available in the Expasy PeptideCutter. In addition, there are some other tools that allow the release of bioactive peptides from a given protein to be estimated; usually, these tools are included in a protein or peptide database such as those described in the following section.
Research interest in bioactive peptides has increased exponentially over the last two decades. This has led to a vast number of bioactive sequences being discovered, mainly from food sources and food co-products. These sequences are stored in bioactive peptide databases, which are powerful sources of information that aid in the selection of raw materials, proteases, and hydrolysates to obtain bioactive peptides. In terms of training, these databases enable the students to observe the differences in the amino acid sequences between different bioactivity groups (e.g., antihypertensive, antioxidant, or antidiabetic peptides). There are generic databases, which include information on peptides with different bioactivities and from a wide range of raw materials, and others that are more specific and focus on just one type of peptide (e.g., antimicrobial peptides) or a specific parent protein (e.g., peptides derived from milk proteins). Some of the more widely used peptide databases are listed below.
This database focuses on peptides that are encrypted within dairy proteins. Dairy proteins are one of the main sources of peptides because milk, apart from basic nutrients, provides proteins that are degraded by native proteases into peptides that have biological activity. The MBPDB database, which is available online at https://mbpdb.nws.oregonstate.edu, was created from hundreds of published scientific papers that identified peptides with in vitro and in vivo biological activity following the hydrolysis of milk proteins (Nielsen et al., 2017). The database includes an MBPDB Search option, where the user can include any amino acid sequence and the software returns a list of publications where that peptide was used together with the main outcomes of each study, particularly the proven bioactivity. As an example, after searching for the tri-peptide IPP, which is a known bioactive sequence, the software returned the list of publications shown in Table 1. The webpage is easy to navigate and provides useful information on bioactive peptides. However, it is limited to peptides derived from milk proteins.
DFBP is freely available at http://www.cqudfbp.net. It consists of a database containing over 6,200 peptides isolated from different sources (Qin et al., 2022). In this database, the bioactive peptides are divided into 31 bioactivities, which include inhibitors of the enzymes ACE-I (EC 3.4.15.1), renin (EC 3.4.23.15), DPP-IV (EC 3.4.14.5), α-amylase (EC 3.2.1.1), and β-glucosidase (EC 3.2.1.20) as well as antioxidant, antimicrobial, antithrombotic, and antiviral peptides. The most abundant peptides are ACE inhibitors (N = 1,961), antioxidants (N = 1,032), and antimicrobial peptides (N = 476). DFBP is more than just a list of bioactive peptides as every peptide in the database is characterised by 30 different attributes including their main physicochemical and functional properties, as well as by their stability and toxicity. It also includes a special section containing those peptides that have two or more proven bioactivities, known as multifunctional peptides. When searching for the IPP peptide, the software identifies it as a multifunctional peptide with ACE-inhibitory and antihypertensive properties. Along with the peptide’s main physicochemical properties (e.g., its theoretical mass, net charge, isoelectric point, GRAVY, hydrophilic residue ration, and length), the software returns information on its predicted bitterness and a list of different proteins in which IPP has been identified. In this case, more than 1,400 proteins that include IPP in their amino acid sequence are available on the database. The list is extensive, including proteins from animal, microbial, and plant sources. Finally, the tool returns a table with cross-references so that the one can see the results for this same peptide in a different database such as the above-mentioned MBPDB, or in BIOPEP (described below).
In addition to the peptide database, DFBP has a range of prediction and calculation tools that include HotSpot Search,3 which can be used to search for specific sequences in a list of proteins or group of proteins selected by the user, and the EHP-Tool,4 which is used to simulate the enzyme hydrolysis of proteins. We hydrolysed bovine serum albumin using papain and the software returned a list of all the potential peptides generated, highlighting those that are already available in their database as bioactive peptides. Other tools include the BPP-Tool,5 which can be used to predict the bitterness of a given amino acid sequence, the Peptide Calculator,6 which estimates the different useful properties of the peptides, and AASD-Tool,7 which is to obtain the peptide descriptors. These can be then used for molecular modelling and bioinformatics prediction.
The BIOPEP-UWM database, formerly known as BIOPEP, is probably the most popular peptide database. It focuses especially on bioactive peptides derived from foods and contains over 4,600 bioactive peptide sequences and more than 750 proteins (although the database is being continuously updated). It was developed by the Chair of Food Biochemistry at Warmia and Mazury University in Poland and is freely available at https://biochemia.uwm.edu.pl/en/biopep-uwm-2/ (Minkiewicz et al., 2019).
The database is divided into four sections: proteins, bioactive peptides, allergenic proteins, and sensory peptides. When searching for the tripeptide IPP in the bioactive peptides section of BIOPEP-UWM, the system returned 32 peptide sequences that include not only IPP but also other longer bioactive peptides in which the IPP sequence is encrypted (e.g., AIPP, IAIPP, MAIPPKK). Focusing just on IPP, the database identified four bioactivities for this peptide including α-amylase inhibition (ID 10286), β-glucosidase inhibition (ID 10311), anti-inflammatory properties (ID 9537), and ACE-inhibitory properties (ID 3522), together with the scientific publications that support their bioactivity. In terms of proteins, the tool allows one to search for useful information on the more than 750 proteins registered, including their chemical mass, number of amino acid residues, sequence of amino acids, and references for the scientific publications in which the protein was used. In addition, BIOPEP-UWM contains a list of over 130 allergenic proteins with their epitopes. In terms of sensory peptides and amino acids, BIOPEP-UWM includes a database with information on their taste and bioactivity data, amongst other useful details (Iwaniak et al., 2016).
One of the most interesting aspects of BIOPEP-UWM is the “Analysis” area, where the user can perform various calculations and estimations on a selected protein. These includes calculating profiles of potential biological activity, which are lists of known bioactive peptides contained within a protein sequence. We calculated the profile of potential ACE-inhibitory activity for bovine serum albumin (ID 1729) and obtained a list with all the ACE-inhibitors that are contained within it, together with their position in the protein (Figure 7). These include, amongst other, ALKAWSVAR, RL, RY, LY, VF, LVL, LPP, and AY. Another interesting option provided in the “Analysis” area is the calculation of the quantitative A and B values. The A parameter refers to the occurrence frequency of bioactive fragments in the protein chain, calculated as the number of fragments with a given activity in the protein divided by the number of amino acid residues in the protein chain. In turn, the B parameter refers to the potential biological activity of the protein fragments; this is calculated using the number of repetitions of bioactive fragments in a protein, their half-maximal activity, their half-maximal inhibition, and the total number of amino acid residues.
Figure 7. Analysis of the simulated thermolysin hydrolysate of bovine serum albumin using BIOPEP-UWM.
BIOPEP-UWM can also be used to simulate the release of bioactive fragments from a given parent protein. This is carried out in the “Enzyme(s) Action” section. The software allows you to use one of the protein sequences already available in the database or to introduce a new protein for analysis. Several proteases are already included and up to three different enzymes can be used simultaneously. Figure 6 shows the simulated hydrolysis of bovine serum albumin using thermolysin. The software calculates the potential cleavage bonds and gives the user two options. Firstly, the user can identify which peptides (from those that have been predicted) have shown bioactivity in the past, independently of their source or bioactivity type. In this case, the software identified 48 bioactive peptides (only the first 10 are shown) which have a variety of bioactivities; these include inhibitors of the enzymes DPP-III, ACE, renin, α-glucosidase, and DPP-IV, immunostimulant peptides, and antioxidant peptides. The second option provided by BIOPEP-UWM is to calculate several parameters that have been developed to facilitate protein comparison. These include W, which refers to the relative frequency of fragments released for a given activity by the selected enzyme (in this case thermolysin) and AE, which refers to the frequency of fragments released for a given bioactivity by the selected enzyme (Iwaniak et al., 2020). The calculation of other parameters, such as BE and V, involves the use of their EC50 or IC50 values, respectively, and therefore might not be available for many of the peptides. All these options could be used by students to compare different proteins, proteases, and protease combinations to identify which raw material, and which enzyme, might induce the release of a greater number of bioactive peptides.
PepDraw is available at https://www2.tulane.edu/~biochem/WW/PepDraw/. It was developed by the Wimley Lab at Tulane University (LA, United States). This tool can be used to draw the primary structure of a peptide and calculate certain theoretical properties, including its isoelectric point, net charge, hydrophobicity, and mass, amongst other parameters. The isoelectric point of a protein is the key parameter that needs to be calculated to recover proteins by precipitation at the industrial scale. Estimating this parameter online allows students to design processes and/or estimate protein recoveries in silico. The images created can be downloaded in png format at high quality. As an example, the 2D structure of the peptide VGTRCCTKPESERMPCTEDY is shown; this, was predicted to be released during the hydrolysis of bovine serum albumin using thermolysin in the previous section (Figure 8A). The tool is available free of charge for academic use.
Figure 8. (A) 2D and (B) 3D representation of the peptide VGTRCCTKPESERMPCTEDY using PepDraw and PEP-FOLD, respectively.
PEP-FOLD is available at https://bioserv.rpbs.univ-paris-diderot.fr/services/PEP-FOLD3/. It is a de novo approach that predicts the structure of a peptide from its amino acid sequence (Lamiable et al., 2016). Using this tool, it is also possible to generate native-like conformations of peptides interacting with a protein when the interaction site is known in advance. The process takes a few minutes and returns 3D images as well as useful information on the peptide sequence. As an example, the 3D structure of the peptide VGTRCCTKPESERMPCTEDY is shown; this was predicted to be released during the hydrolysis of bovine serum albumin using thermolysin (Figure 8B). PEP-FOLD is limited to amino acid sequences between 5 and 50 residues long, which is enough for teaching enzymatic proteolysis and various topics related to bioactive peptides, given that most of them are much shorter than 50 residues in length.
Several useful tools for the teaching and training of enzymatic proteolysis have been developed by the SIB Swiss Institute of Bioinformatics, all of which are available at the Expasy portal. Expasy Compute pI/MW8 is a tool that allows one to calculate the isoelectric point and the molecular weight of a protein sequence. This information is useful for developing protein isolation processes based on isoelectric precipitation (Villaró et al., 2023) or for estimating the approximate region where a protein may be found in an electrophoresis gel. ProtParam,9 computes several physicochemical parameters for a given amino acid sequence including its molecular weight, amino acid composition, extinction coefficient, aliphatic index, or grand average of hydropathicity (GRAVY), amongst others. For bovine serum albumin, the software returned its molecular weight (69190.28), atomic composition (C3068H4821N815O926S39), total number of atoms (9669), estimated half-life (10–30 h), instability index (40.33, unstable), aliphatic index (77.59), GRAVY (−0.434), and amino acid composition (7.9% Ala, 4.3% Arg, 2.3% Asn, 6.6% Asp., 5.6% Cys, 3.3% Gln, 9.7% Glu, 2.8% Gly, 2.8% His, 2.5% Ile, 10.7% Leu, 9.9% Lys, 0.8% Met, 5.0% Phe, 4.6% Pro, 5.3% Ser, 5.6% Thr, 0.5% Trp, 3.5% Tyr, and 6.3% Val). The tool also provided a summary of the total number of negatively charged (Asp + Glu) and positively charged (Arg + Lys) residues: 99 and 86, respectively.
PeptideRanker, available at http://distilldeep.ucd.ie/PeptideRanker/, gives a score for a given peptide (or list of peptides) that predicts the probability of it being bioactive (Mooney et al., 2012). PeptideRanker was trained using different peptide databases mainly antimicrobial peptide databases; these included approximately 19,000 unique peptide sequences. It includes two independent neural network predictors for short and long peptides (larger than 20 amino acids); these predict those peptides that are more likely to be bioactive from amongst a set of peptides. The software is available free of charge for academic use and has been effectively used to aid in the identification of bioactive peptides from different food sources (Lafarga et al., 2015; de Fátima Garcia et al., 2020).
Peptides released during food digestion do not pose a risk to human health. However, certain peptides are toxic and their oral administration at high doses (or even at low concentrations via intravenous administration) might be dangerous. For this reason, several online tools have been developed to estimate the potential toxicity of a peptide sequence. ToxIBTL, freely accessible at https://server.wei-group.net/ToxIBTL/, is based on novel deep learning frameworks (Wei et al., 2022). ToxinPred, available free of charge at http://crdd.osdd.net/raghava/toxinpred/, was developed to predict potentially toxic peptides using a dataset of over 1,800 toxic peptides shorter than 35 residues in length (Gupta et al., 2013). This software is based on models using machine learning techniques and a quantitative matrix using of the different peptide properties related to toxicity. A third online software tool for predicting toxicity is CSM-TOXIN, which is available at https://biosig.lab.uq.edu.au/csm_toxin/. The software relies solely on the primary protein structure and encodes the sequence information using deep learning techniques (Morozov et al., 2023). It is important to point out that, although these tools enable one to estimate potential toxicity, the safety of a peptide must be validated in vitro and in vivo prior to being administered to humans. This tool allows students to estimate which enzymes are more suitable for producing or avoiding the release of toxic peptides from a given protein source. Furthmore, students can identify (when designing the process) which proteins might pose a potential toxicity risk.
Proteins and peptides must be evaluated for their allergenic potential prior to being commercialised because both pose a risk of inducing allergic responses. In this regard, there are various online tools that can be used during the training and education of chemical engineers. These include (i) AllerCatPro, available at https://allercatpro.bii.a-star.edu.sg, which predicts potential allergenicity based on the similarity of the 3D structure of proteins and their amino acid sequences (Maurer-Stroh et al., 2019), (ii) AlgPred, available at http://crdd.osdd.net/raghava/algpred/, which is based on support vector machine methods using the amino acid and dipeptide composition (Saha and Raghava, 2006), and (iii) Allermatch,10 which predicts allergenicity following the recommendations given in the Codex Alimentarius and the FAO/WHO Expert consultation on the allergenicity of foods (Fiers et al., 2004). In addition, ChAIPred, which is available at https://webs.iiitd.edu.in/raghava/chalpred/, can be used to estimate the potential allergenicity of chemical compounds that are not proteins or peptides (Sharma et al., 2021). These tools can be used for training and educational activities and during the initial screening of allergens. However, potential allergenicity must be further validated as the results given by these online tools are only estimates and predictions.
The present paper provides a summary of several protein/peptide databases, as well as various online tools that are easy-to-use and freely available. These can be used to predict the enzymatic cleavage of proteins and the potential bioactivity, allergenicity, bioaccessibility, and other useful characteristics of proteolytic processes. Such tools could be used during training activities for chemical engineers, food scientists, biotechnologists, or chemists. Amongst the advantages of using these tools during training is the fact that they allow one to observe what happens during the process and to obtain data that would not be possible with conventional technical training. This has been recently discussed in another work, in which algorithms and design equations were suggested to allow results that chemical engineering students would otherwise find hard to identify and lead to a superficial understanding of the problem (Roman et al., 2020). Their use could be evaluated by collecting post-session student feedback and by evaluating what was learned during the training, for example, via oral presentations or a written exam. Assessing not only the academic performance of students but also their learning experience would be a useful way of evaluating the teaching and learning experience. Moreover, simulating proteolysis online would allow students to continue the simulations and estimations at home with no need for laboratory reagents and equipment.
SV-C: Conceptualization, Visualization, Writing – original draft. TL: Conceptualization, Funding acquisition, Visualization, Writing – original draft.
The author(s) declare financial support was received for the research, authorship, and/or publication of this article. This work forms part of the CLEAN·AIR (TED2021-131511A-I00) and SOLAR·FOODS (PID2022-136292OB-I00) projects, both funded by the Spanish Ministry of Science and Innovation - MCIN/AEI/10.13039/501100011033 and the European Union NextGenerationEU/PRTR. It was also funded by the BLUE·FUTURE (PCM_00083) project, overseen by the Regional Government of Andalusia and the European Union NextGenerationEU/PRTR. TL would like to thank PPIT-UAL, Junta de Andalucía-FEDER 2021-2027 (Programme 54.A) and the Ramon y Cajal Programme (RYC2021-031061-I) funded by MCIN/AEI/10.13039/501100011033 and the European Union NextGenerationEU/PRTR.
The authors declare that the research was conducted in the absence of any commercial or financial relationships that could be construed as a potential conflict of interest.
All claims expressed in this article are solely those of the authors and do not necessarily represent those of their affiliated organizations, or those of the publisher, the editors and the reviewers. Any product that may be evaluated in this article, or claim that may be made by its manufacturer, is not guaranteed or endorsed by the publisher.
1. ^https://web.expasy.org/peptide_cutter/
2. ^https://gitlab.pasteur.fr/nmaillet/rpg
3. ^http://www.cqudfbp.net/blast/data_input.jsp
4. ^http://www.cqudfbp.net/enzymes/hydrolysis_tools/dataInput.jsp
5. ^http://www.cqudfbp.net/bitterPrediction/tools/DataInput.jsp
6. ^http://www.cqudfbp.net/peptide_calculator/data_input.jsp
7. ^ http://www.cqudfbp.net/uploadanddownload/upload.jsp
8. ^https://web.expasy.org/compute_pi/
Adams, C., Sawh, F., Green-Johnson, J. M., Jones Taggart, H., and Strap, J. L. (2020). Characterization of casein-derived peptide bioactivity: differential effects on angiotensin-converting enzyme inhibition and cytokine and nitric oxide production. J. Dairy Sci. 103, 5805–5815. doi: 10.3168/JDS.2019-17976
Bateman, A. (2019). UniProt: a worldwide hub of protein knowledge. Nucleic Acids Res. 47, D506–D515. doi: 10.1093/NAR/GKY1049
Borreguero, A. M., Valverde, J. L., García-Vargas, J. M., and Sánchez-Silva, L. (2019). Simulator-based learning in the teaching of chemical engineering. Comput. Appl. Eng. Educ. 27, 1267–1276. doi: 10.1002/CAE.22150
Chakrabarti, S., Liao, W., Davidge, S. T., and Wu, J. (2017). Milk-derived tripeptides IPP (Ile-pro-pro) and VPP (Val-pro-pro) differentially modulate angiotensin II effects on vascular smooth muscle cells. J. Funct. Foods 30, 151–158. doi: 10.1016/J.JFF.2016.12.022
Chakrabarti, S., and Wu, J. (2015). Milk-derived tripeptides IPP (Ile-pro-pro) and VPP (Val-pro-pro) promote adipocyte differentiation and inhibit inflammation in 3T3-F442A cells. PLoS One 10:e0117492. doi: 10.1371/JOURNAL.PONE.0117492
Charoenkwan, P., Anuwongcharoen, N., Nantasenamat, C., Hasan, M. M., and Shoombuatong, W. (2020). In silico approaches for the prediction and analysis of antiviral peptides: a review. Curr. Pharm. Des. 27, 2180–2188. doi: 10.2174/1381612826666201102105827
de Fátima Garcia, B., de Barros, M., and de Souza Rocha, T. (2020). Bioactive peptides from beans with the potential to decrease the risk of developing noncommunicable chronic diseases. Crit Rev Food Sci Nutr 61, 2003–2021. doi: 10.1080/10408398.2020.1768047
Donkor, O. N., Henriksson, A., Singh, T. K., Vasiljevic, T., and Shah, N. P. (2007). ACE-inhibitory activity of probiotic yoghurt. Int. Dairy J. 17, 1321–1331. doi: 10.1016/J.IDAIRYJ.2007.02.009
Duvaud, S., Gabella, C., Lisacek, F., Stockinger, H., Ioannidis, V., and Durinx, C. (2021). Expasy, the Swiss bioinformatics resource portal, as designed by its users. Nucleic Acids Res. 49, W216–W227. doi: 10.1093/NAR/GKAB225
Fiers, M. W. E. J., Kleter, G. A., Nijland, H., Peijnenburg, A. A. C. M., Nap, J. P., and van Ham, R. C. H. J. (2004). Allermatch™, a webtool for the prediction of potential allergenicity according to current FAO/WHO codex alimentarius guidelines. BMC Bioinformatics 5, 1–6. doi: 10.1186/1471-2105-5-133/TABLES/2
Gautam, A., Chaudhary, K., Kumar, R., Sharma, A., Kapoor, P., Tyagi, A., et al. (2013). In silico approaches for designing highly effective cell penetrating peptides. J. Transl. Med. 11:74. doi: 10.1186/1479-5876-11-74/FIGURES/6
Gupta, S., Kapoor, P., Chaudhary, K., Gautam, A., Kumar, R., and Raghava, G. P. S. (2013). In silico approach for predicting toxicity of peptides and proteins. PLoS One 8:e73957. doi: 10.1371/JOURNAL.PONE.0073957
Huttunen, M. M., Pekkinen, M., Ahlström, M. E. B., and Lamberg-Allardt, C. J. E. (2007). Effects of bioactive peptides isoleucine-proline-proline (IPP), valine-proline-proline (VPP) and leucine-lysine-proline (LKP) on gene expression of osteoblasts differentiated from human mesenchymal stem cells. Br. J. Nutr. 98, 780–788. doi: 10.1017/S0007114507744434
Iwaniak, A., Minkiewicz, P., Darewicz, M., Sieniawski, K., and Starowicz, P. (2016). BIOPEP database of sensory peptides and amino acids. Food Res. Int. 85, 155–161. doi: 10.1016/J.FOODRES.2016.04.031
Iwaniak, A., Minkiewicz, P., Pliszka, M., Mogut, D., and Darewicz, M. (2020). Characteristics of biopeptides released in silico from collagens using quantitative parameters. Foods 9:965. doi: 10.3390/FOODS9070965
Jäkälä, P., Turpeinen, A. M., Rajakari, K., Korpela, R., and Vapaatalo, H. (2010). Biological effects of casein-derived tripeptide powders are not affected by fermentation process. Int. Dairy J. 20, 366–370. doi: 10.1016/J.IDAIRYJ.2009.11.017
Jing, P., Qian, B., He, Y., Zhao, X., Zhang, J., Zhao, D., et al. (2014). Screening milk-derived antihypertensive peptides using quantitative structure activity relationship (QSAR) modelling and in vitro/in vivo studies on their bioactivity. Int. Dairy J. 35, 95–101. doi: 10.1016/J.IDAIRYJ.2013.10.009
Kumar, R., Chaudhary, K., Chauhan, J. S., Nagpal, G., Kumar, R., Sharma, M., et al. (2015). An in silico platform for predicting, screening and designing of antihypertensive peptides. Nat. Publ. Group 5:12512. doi: 10.1038/srep12512
Lafarga, T., Aluko, R. E., Rai, D. K., O’Connor, P., and Hayes, M. (2016). Identification of bioactive peptides from a papain hydrolysate of bovine serum albumin and assessment of an antihypertensive effect in spontaneously hypertensive rats. Food Res. Int. 81, 91–99. doi: 10.1016/j.foodres.2016.01.007
Lafarga, T., Álvarez, C., and Hayes, M. (2017). Bioactive peptides derived from bovine and porcine co-products: a review. J. Food Biochem. 41:e12418. doi: 10.1111/jfbc.12418
Lafarga, T., O’Connor, P., and Hayes, M. (2014). Identification of novel dipeptidyl peptidase-IV and angiotensin-I-converting enzyme inhibitory peptides from meat proteins using in silico analysis. Peptides 59, 53–62. doi: 10.1016/j.peptides.2014.07.005
Lafarga, T., O’Connor, P., and Hayes, M. (2015). In silico methods to identify meat-derived prolyl endopeptidase inhibitors. Food Chem. 175, 337–343. doi: 10.1016/j.foodchem.2014.11.150
Lamiable, A., Thevenet, P., Rey, J., Vavrusa, M., Derreumaux, P., and Tuffery, P. (2016). PEP-FOLD3: faster de novo structure prediction for linear peptides in solution and in complex. Nucleic Acids Res. 44, W449–W454. doi: 10.1093/NAR/GKW329
Lu, J., Ren, D. F., Xue, Y. L., Sawano, Y., Miyakawa, T., and Tanokura, M. (2010). Isolation of an antihypertensive peptide from alcalase digest of spirulina platensis. J. Agric. Food Chem. 58, 7166–7171. doi: 10.1021/jf100193f
Maurer-Stroh, S., Krutz, N. L., Kern, P. S., Gunalan, V., Nguyen, M. N., Limviphuvadh, V., et al. (2019). AllerCatPro—prediction of protein allergenicity potential from the protein sequence. Bioinformatics 35, 3020–3027. doi: 10.1093/BIOINFORMATICS/BTZ029
Minkiewicz, P., Iwaniak, A., and Darewicz, M. (2019). BIOPEP-UWM database of bioactive peptides: current opportunities. Int. J. Mol. Sci. 20:5978. doi: 10.3390/IJMS20235978
Mooney, C., Haslam, N. J., Pollastri, G., and Shields, D. C. (2012). Towards the improved discovery and Design of Functional Peptides: common features of diverse classes permit generalized prediction of bioactivity. PLoS One 7:e45012. doi: 10.1371/JOURNAL.PONE.0045012
Morozov, V., Rodrigues, C. H. M., and Ascher, D. B. (2023). CSM-Toxin: a web-server for predicting protein toxicity. Pharmaceutics 15:431. doi: 10.3390/PHARMACEUTICS15020431/S1
Nakamura, Y., Yamamoto, N., Sakai, K., Okubo, A., Yamazaki, S., and Takano, T. (1995). Purification and characterization of angiotensin I-converting enzyme inhibitors from sour Milk. J. Dairy Sci. 78, 777–783. doi: 10.3168/JDS.S0022-0302(95)76689-9
Narva, M., Collin, N., Jauhiainen, T., Vapaatalo, J., and Korpela, R. (2004). Effects of Lactobacillus helveticus fermented milk and its bioactive peptides on bone parameters in spontaneously hypertensive rats. Milchwissenschaft 59, 359–363.
Nielsen, S. D., Beverly, R. L., Qu, Y., and Dallas, D. C. (2017). Milk bioactive peptide database: a comprehensive database of milk protein-derived bioactive peptides and novel visualization. Food Chem. 232, 673–682. doi: 10.1016/J.FOODCHEM.2017.04.056
Okamoto, K., Kawamura, S., Tagawa, M., Mizuta, T., Zahid, H. M., and Nabika, T. (2020). Production of an antihypertensive peptide from milk by the brown rot fungus Neolentinus lepideus. Eur. Food Res. Technol. 246, 1773–1782. doi: 10.1007/S00217-020-03530-Y/TABLES/3
Qin, D., Bo, W., Zheng, X., Hao, Y., Li, B., Zheng, J., et al. (2022). DFBP: a comprehensive database of food-derived bioactive peptides for peptidomics research. Bioinformatics 38, 3275–3280. doi: 10.1093/BIOINFORMATICS/BTAC323
Rodrigues, R., Soares, R. P., and Secchi, A. R. (2010). Teaching chemical engineering using EMSO simulator. Comput. Appl. Eng. Educ. 18, 607–618. doi: 10.1002/cae.20255
Roman, C., Delgado, M. A., and García-Morales, M. (2020). Using process simulators in chemical engineering education: is it possible to minimize the “black box” effect? Comput. Appl. Eng. Educ. 28, 1369–1385. doi: 10.1002/cae.22307
Rose, P., Prlic, A., Altunkaya, A., Bi, C., Bradley, A., Christie, C., et al. (2016). The RCSB protein data bank: integrative view of protein, gene and 3D structural information. Nucleic Acids Res. 45, D271–D281. doi: 10.1093/nar/gkw1000
Saha, S., and Raghava, G. P. S. (2006). AlgPred: prediction of allergenic proteins and mapping of IgE epitopes. Nucleic Acids Res. 34, W202–W209. doi: 10.1093/NAR/GKL343
Sayd, T., Dufour, C., Chambon, C., Buffière, C., Remond, D., and Santé-Lhoutellier, V. (2018). Combined in vivo and in silico approaches for predicting the release of bioactive peptides from meat digestion. Food Chem. 249, 111–118. doi: 10.1016/J.FOODCHEM.2018.01.013
Schechter, I., and Berger, A. (1968). On the active site of proteases. III. Mapping the active site of papain; specific peptide inhibitors of papain. Biochem. Biophys. Res. Commun. 32, 898–902. doi: 10.1016/0006-291X(68)90326-4
Sharma, N., Patiyal, S., Dhall, A., Devi, N. L., and Raghava, G. P. S. (2021). ChAlPred: a web server for prediction of allergenicity of chemical compounds. Comput. Biol. Med. 136:104746. doi: 10.1016/J.COMPBIOMED.2021.104746
Tagliazucchi, D., Shamsia, S., and Conte, A. (2016). Release of angiotensin converting enzyme-inhibitory peptides during in vitro gastro-intestinal digestion of camel milk. Int. Dairy J. 56, 119–128. doi: 10.1016/J.IDAIRYJ.2016.01.009
Turpeinen, A. M., Kumpu, M., Rönnback, M., Seppo, L., Kautiainen, H., Jauhiainen, T., et al. (2009). Antihypertensive and cholesterol-lowering effects of a spread containing bioactive peptides IPP and VPP and plant sterols. J. Funct. Foods 1, 260–265. doi: 10.1016/J.JFF.2009.03.001
Ulug, S. K., Jahandideh, F., and Wu, J. (2021). Novel technologies for the production of bioactive peptides. Trends Food Sci. Technol. 108, 27–39. doi: 10.1016/J.TIFS.2020.12.002
Villaró, S., Jiménez-Márquez, S., Musari, E., Bermejo, R., and Lafarga, T. (2023). Production of enzymatic hydrolysates with in vitro antioxidant, antihypertensive, and antidiabetic properties from proteins derived from Arthrospira platensis. Food Res. Int. 163:112270. doi: 10.1016/J.FOODRES.2022.112270
Vogelsang-O’dwyer, M., Sahin, A. W., Arendt, E. K., and Zannini, E. (2022). Enzymatic hydrolysis of pulse proteins as a tool to improve techno-functional properties. Foods 11:1307. doi: 10.3390/FOODS11091307
Wei, L., Ye, X., Sakurai, T., Mu, Z., and Wei, L. (2022). ToxIBTL: prediction of peptide toxicity based on information bottleneck and transfer learning. Bioinformatics 38, 1514–1524. doi: 10.1093/BIOINFORMATICS/BTAC006
Yamada, A., Sakurai, T., Ochi, D., Mitsuyama, E., Yamauchi, K., and Abe, F. (2015). Antihypertensive effect of the bovine casein-derived peptide met-Lys-pro. Food Chem. 172, 441–446. doi: 10.1016/J.FOODCHEM.2014.09.098
Yang, J., Gao, Z., Ren, X., Sheng, J., Xu, P., Chang, C., et al. (2021). DeepDigest: prediction of protein proteolytic digestion with deep learning. Anal. Chem. 93, 6094–6103. doi: 10.1021/ACS.ANALCHEM.0C04704/SUPPL_FILE/AC0C04704_SI_001.PDF
Keywords: education, bioactive peptides, enzymes, BIOPEP, databases, proteins
Citation: Villaró-Cos S and Lafarga T (2023) Online tools to support teaching and training activities in chemical engineering: enzymatic proteolysis. Front. Educ. 8:1290287. doi: 10.3389/feduc.2023.1290287
Received: 20 September 2023; Accepted: 13 November 2023;
Published: 23 November 2023.
Edited by:
Krishna Prasad Rajan, Royal Commission for Jubail and Yanbu, Saudi ArabiaReviewed by:
Aravinthan Gopanna, Royal Commission for Jubail and Yanbu, Saudi ArabiaCopyright © 2023 Villaró-Cos and Lafarga. This is an open-access article distributed under the terms of the Creative Commons Attribution License (CC BY). The use, distribution or reproduction in other forums is permitted, provided the original author(s) and the copyright owner(s) are credited and that the original publication in this journal is cited, in accordance with accepted academic practice. No use, distribution or reproduction is permitted which does not comply with these terms.
*Correspondence: Tomas Lafarga, dG9tYXMubGFmYXJnYUB1YWwuZXM=
Disclaimer: All claims expressed in this article are solely those of the authors and do not necessarily represent those of their affiliated organizations, or those of the publisher, the editors and the reviewers. Any product that may be evaluated in this article or claim that may be made by its manufacturer is not guaranteed or endorsed by the publisher.
Research integrity at Frontiers
Learn more about the work of our research integrity team to safeguard the quality of each article we publish.