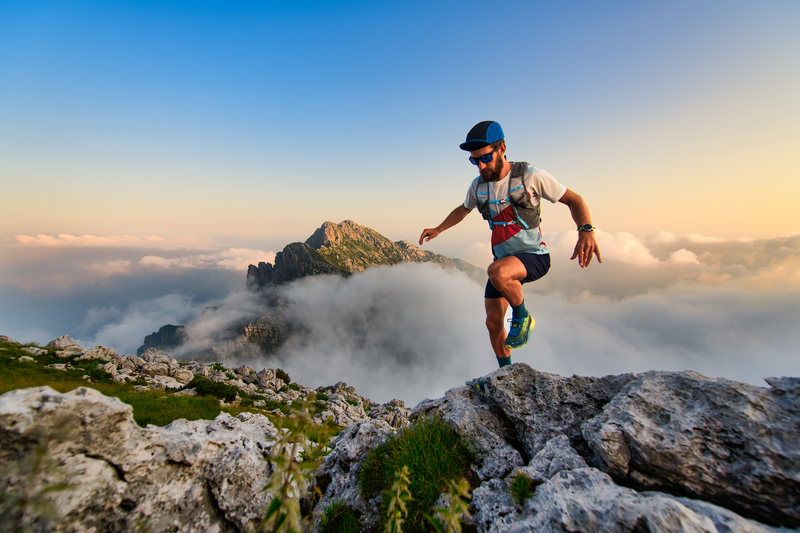
95% of researchers rate our articles as excellent or good
Learn more about the work of our research integrity team to safeguard the quality of each article we publish.
Find out more
ORIGINAL RESEARCH article
Front. Educ. , 19 October 2023
Sec. Assessment, Testing and Applied Measurement
Volume 8 - 2023 | https://doi.org/10.3389/feduc.2023.1281431
In the Dynamic Model of Educational Effectiveness, classroom-level effectiveness factors are assessed through observations or aggregated students’ ratings. The current study is aimed at developing and validating a complementary teacher self-report instrument of effective practices at classroom level (the DMEE-Class-T). The new instrument showed concurrent validity with the CSS-T. Dimensionality of both instruments was examined using the bifactor exploratory structural equation modeling (ESEM) framework, testing for alternative factorial representations of the data collected in a large-scale study conducted in all Luxembourgish elementary schools. For both instruments, the bifactor-ESEM and ESEM models showed excellent fitting indices and parameters, but the ESEM solution was retained as the best model for parsimony purpose. These results suggest that the eight types of practices assessed through the DMEE-Class-T could be considered as distinct facets of effective teaching practices while taking items cross-loadings into account. Implications for research and teachers’ professional development are discussed.
According to several authors (Scheerens, 2016; Sánchez-Cabrero et al., 2021; Charalambous and Praetorius, 2023), the Dynamic Model of Educational Effectiveness (DMEE, Creemers and Kyriakides, 2008; Kyriakides et al., 2020) is one of the reference models that encompassed the most relevant theoretical and empirical findings in the field of educational effectiveness. DMEE is a comprehensive and general theoretical framework based on over 40 years of research conducted as part of the Educational Effectiveness Research. Quantitative syntheses and numerous empirical studies in different countries have provided strong support to the main assumptions of the model (see Kyriakides and Panayiotou, 2023 for a review).
The first distinctive feature of the DMEE is that it aims to explain different types of students’ outcomes (cognitive, affective, psychomotor, metacognitive), which are therefore not limited to the traditional measures of competence in mathematics, reading or science. The second feature of the model is its multi-level nature. The output variables are explained not only by factors at student level, but also by factors at class level, school level and system (or regional) level. Finally, the third feature of the model is that it considers the factors at the different levels not only quantitatively, generally in terms of frequency or duration, but also qualitatively in terms of focus (degree of specificity of the tasks or practices), stage (point in the educational process at which the tasks or practices are proposed), quality (direct impact of the tasks or practices on the targeted learning or behaviour) and differentiation (adaptation of the tasks or practices to different groups of pupils). Contextual factors at the level of the system (or its regional entities) refer to the policy advocated in terms of education and teaching, but also to the value placed by society on learning and the school institution. At this macro level, it is also a question of describing national (or regional) policies relating to the professional development of teachers, the evaluation of schools and teachers, and the development of school quality. School-level factors have direct effects on student outcomes, but also indirect effects by influencing classroom-level factors, in particular teaching practices. Specifically, the model refers, on the one hand, to the policies defined by the school with regard to teaching practices (quantity, quality, learning opportunities offered) and the quality of the learning environment (management of student behaviour outside the classroom, collaboration between teachers, partnerships with other players, provision of sufficient resources for students and teachers) and, on the other hand, to the evaluation of these policies within the school. According to the DMEE, there are eight factors of effectiveness defined at classroom level: (1) orientation practices, (2) structuring practices, (3) questioning techniques, (4) teaching-modelling practices, (5) exercising, (6) management of time, (7) making the classroom a learning environment and (8) assessment practices (Creemers and Kyriakides, 2008; Kyriakides et al., 2018). Orientation practices refer to how teachers engage students in understanding the purpose behind a learning activity to make the lesson content more meaningful to them. Structuring practices are practices used to present and structure course materials and lessons parts (i.e., overviews, roadmap, indicating transitions, emphasizing key concepts, summarizing). Questioning techniques refer to posing a variety of questions (about product but also process) at appropriate level of difficulty and to adequately dealing with students’ answers. Questioning can be a valuable tool for assessing students’ comprehension, assisting them in clarifying their thoughts, and articulating their understanding to develop a sense of mastery. Teaching-modelling practices refer to self-regulated learning. Effective teachers encourage students to use specific strategies or to develop their own problem-solving strategies. Exercising refers to opportunities for practice and application. Management of time focuses on the amount of time used for on-task behavior. The aim is to minimize the time lost because of classroom disruptions or organizational issues. Making the classroom a learning environment refers to a classroom climate which encourages interactions between teachers and students, and between students, and which minimizes students’ disruptive behaviors. Assessment practices mainly refer to the use of formative assessment practices. Teachers are expected to use appropriate techniques to gather data regarding students’ learning, to identify individual needs, and assess their own practices. It is important to note that it is the combination of these eight factors and their quantitative and qualitative aspects that defines the quality of the teaching provided. In other words, it is not enough for these practices to be frequent; they must also be implemented with quality.
Importantly, in empirical studies testing the DMEE, data at classroom level are in general collected by external observers and/or through questionnaires administered to students which are then aggregated at class level. Teachers are asked to fill in the school-level questionnaire but never a classroom-level questionnaire about their practices. In a context other than research or formal teacher assessment, self-reported measures could increase teachers’ willingness to engage in self-reflective assessment of the real impact of their teaching practices. This is of particular importance in school-wide multi-tiered systems of support frameworks where teachers are supposed to use effective instructional and behavioral practices before addressing struggling students to more intensive support services.
In complement to the existing instruments developed in reference to the DMEE, the present study aims at developing and validating a teacher questionnaire to measure classroom level factors.
Improving teaching practices has been on the agenda of most education systems in industrialised countries for two decades now (OECD, 2003, 2007; Levin, 2011; Kraft and Gilmour, 2017; Brown and Malin, 2022). In this context, more and more attention is being paid to the assessment and improvement of teachers’ practices. According to Reddy et al. (2021), teacher evaluation is designed to provide teachers with formative feedback that could help to identify professional development needs. In the USA, multi-method teacher evaluation systems combining administrators’ and students’ ratings of teachers, classroom observations, and student competency growth measures are already in place, even if they are not without measurement bias (see for example the sampling effect limiting the accuracy of observer ratings, Clausen, 2002; Göllner et al., 2021).
Teacher input is, in general, not included in multi-method teacher evaluation systems or in studies about instructional quality because of common self-report method issues, such as social desirability (Little et al., 2009; Van de Vijver and He, 2014). This issue often occurs when respondents are asked to indicate the importance of an instructional strategy on a Likert scale of importance. One notable exception is the Teaching and Learning International Survey (TALIS) conducted by the OECD about teachers, school leaders and their learning environment. In this recurrent international large-scale survey, instructional quality is measured through teachers’ self-report. To minimize the issue of social desirability, TALIS uses frequency response scales (Ainley and Carstens, 2018). The questionnaire asks respondents to use a frequency scale to indicate how often a particular instructional strategy occurs during lessons. According to the authors, this choice of response scale means that teachers’ self-reports on selected instructional practices no longer represent the quality of these instructional practices but the frequency of their occurrence, which reduces social desirability.
Numerous studies have however examined the correlation between teachers’ self-reports on their teaching and classrooms observations or students’ ratings (Desimone et al., 2010; Wagner et al., 2016; Fauth et al., 2020; Lazarides and Schiefele, 2021; Wiggs et al., 2023). According to Fauth et al. (2020), there are two consistent findings: (1) overall, the correlations are low, and (2) the highest correlations are observed among classroom management strategies, rather than other strategies such as cognitive activation or student support. As an illustration, we summarize here the recent study of Wiggs et al. (2023) who analysed the convergence between teachers’ self-reports of teaching practices and school administrators’ observation ratings using the Classroom Strategies Assessment System (CSAS, Reddy and Dudek, 2014). The CSAS has been designed for teachers and school administrators to concurrently evaluate teachers’ use of evidence-based instructional practices and behavior management strategies. The authors compared data collected through the CSAS Observer form (CSS-O) and those from the CSAS Teacher form (CSS-T), both including the same rating scales. Observers and teachers are first asked to rate how often teachers use specific strategies on a 7-point Likert scale (1 = never, 7 = always used) and then rate how often the teachers should have used each strategy. An item discrepancy score is then calculated by making the difference between the observed/reported frequency of use and the recommended frequency of use. These discrepancy scores were used for the comparison between observers’ and teachers’ ratings. Results showed that cross-informant correlations were statistically significant, but low to moderate. To partially explain small correlations, the authors explained that the CSS-O ratings were generated in real time during lesson while CSS-T ratings were completed by the teachers some time after the lesson. During this self-reflection exercise, teachers were maybe more critical about certain strategies they had hoped to improve on facing the administrator.
Despite the low correlation between teacher self-report of instructional quality and other sources of information, it however can be seen as a valuable and complementary source of data for decision making post teacher evaluation, and maybe more an interesting tool for professional development (Koziol and Burns, 1986; Wiggs et al., 2023). The conversations between administrators and teachers based on multi-method teacher evaluation systems should lead to better specify need-based teacher training and to focus on practices whose effectiveness has been demonstrated by research.
In this respect, the present study aims at developing a teacher questionnaire to assess the eight classroom-level factors of the DMEE, complementary to the students’ questionnaire already used for this purpose. Another objective is to evaluate the concurrent validity of this new teacher self-report instrument with the CSS-T instrument.
In November and December 2021, the 5,905 teachers at state elementary schools in Luxembourg were asked to take part in a national consultation commissioned by the National Observatory for School Quality and funded by the Ministry of Education and Youth. The study was approved by the Ethics Review Panel of the University of Luxembourg. Every teacher working in a basic school received an e-mail inviting them to take part in the consultation, together with a unique access code giving them access to one of the six versions of the questionnaire designed to cover a range of aspects of the profession. The six versions of the questionnaire included both common and specific items, which explains why not all teachers responded to all the items. Participation in the consultation was anonymous and not compulsory. A total of 1825 teachers logged on to the Qualtrics electronic platform that hosted the questionnaires, but a significant number of them (825) did not reach the end of the questionnaire. Of these 825 teachers, only 16% had a questionnaire completion rate of 50% or more. We nevertheless decided to analyse all available data. The analytical dataset comprises 1,418 teachers, with 80.2% being female, and an average teaching experience of 14.6 years. In terms of teaching levels,1 23.3% are cycle 1 teachers, 25.6% are cycle 2 teachers, 22.8% are cycle 3 teachers, 19.6% are cycle 4 teachers, and 8.7% of the teachers work across multiple teaching levels.
Scale development followed the suggestions made by DeVellis (2012). A pool of items was drawn up based on original items and items taken from or adapted from existing scales (Creemers and Kyriakides, 2012; Kyriakides et al., 2013; Roy et al., 2013). Items used in the present study were administered in French and were pretested with 88 teachers during a pilot study in May 2021. For each of the eight theoretical factors of the DMEE-Class-T, we tried to include items measuring the quantitative and qualitative dimensions of evaluation (frequency, focus, stage, quality and differentiation), but this was not possible for each factor, as it is the case in studies only using the student questionnaire (e.g., Kyriakides et al., 2012; Panayiotou et al., 2014; Vanlaar et al., 2016; Teodorović et al., 2022). Importantly, it has to be noted that the structural validity of the 8-factor assumption and their five dimensions of evaluation has been investigated, and only partially confirmed, in a few studies relying on observation data (Dimosthenous et al., 2020), on students’ data (Panayiotou et al., 2014), or on a combination of both types of data (e.g., Azigwe et al., 2016; Kyriakides et al., 2018). In these studies, Confirmatory Factor Analyses (CFA) were conducted separately for each factor to assess the extent to which data emerging from different dimensions of evaluation can be used to measure each factor. Factor scores were then calculated and used in subsequent analyses, but at no point was the existence of the theoretical factors verified within a single CFA first-order measurement model (see Anderson and Gerbing, 1988). Moreover, in some studies aimed at defining stages of effective teaching (e.g., Kyriakides et al., 2013), students’ data were analyzed using the Item Response Theory and the Rasch model in particular. Authors showed that the teaching behaviors measured by the student questionnaire was reducible to a common unidimensional scale. In the light of these results, it seems there is still a need to investigate the structural validity of the classroom-level factors and their dimensions, as defined by the DMEE. This is even truer for the new self-report instrument that is discussed here.
Descriptive statistics for the 58 items of the DMEE-Class-T are given in Supplementary Table S1 in the online supplements. All items were rated using a seven-point scale (1 = never, 2 = rarely, 3 = sometimes, 4 = regularly, 5 = frequently, 6 = very frequently, 7 = systematically).
Orientation refers to teaching behaviours aimed at explaining to students or questioning them about the reasons for which a particular activity or lesson or series of lessons occur. It was measured with 8 items.
Structuring refers to teaching behaviours aimed at explaining the structure of the lesson, with or without links to previous lessons. It was measured with 9 items.
Questioning refers to questioning techniques (i.e., raising different types of questions at appropriate difficulty level, giving time for students to respond, dealing with student responses). It was measured with 7 items.
Modeling refers to teaching behaviours aimed at helping students acquire learning strategies and procedures, or solve problematic situations. It was measured with 6 items.
Application activities refer to activities intended to help students understand what has been taught during the lesson. It was measured with 8 items.
Learning environment refers to all actions aimed at ensuring a positive classroom climate and creating a well-organized and accommodating environment for learning. It was measured with 6 items.
Assessment refers to teaching behaviours aimed at identifying the students’ learning needs, as well as at evaluating their own practice. It was measured with 11 items.
Management of time refers to actions aimed at prioritizing academic instruction and allocating available time to curriculum-related activities and at maximizing student engagement rates. It was measured with 3 items, but exploratory analyses showed that the Cronbach alpha (0.29) was unacceptable. Unfortunately, these items were not included in the subsequent analyses.
Reddy et al. (2015) developed a self-assessment instrument for teaching practices and managing student behaviour in the classroom (the Classroom Strategies Scales-Teacher Form, CSS-T). The CSS-T is designed to measure how often teachers use specific strategies that have been shown to be effective in research. According to the authors, it is possible to distinguish eight sub-dimensions. In terms of teaching strategies, the four factors are: (1) Student Focused Learning and Engagement (7 items), (2) Instructional Delivery (7 items), (3) Promotes Student Thinking (6 items) and (4) Academic Performance Feedback (6 items). In terms of behaviour management strategies, the four factors are: (1) Praise (5 items), (2) Corrective Feedback (6 items), (3) Prevention management (5 items) and (4) Directives/transitions (7 items). Descriptive statistics for the 49 CSS-T items are given in Supplementary Table S2 in the online supplements. All items were rated using a seven-point scale (1 = never, 2 = rarely, 3 = sometimes, 4 = regularly, 5 = frequently, 6 = very frequently, 7 = systematically).
To test the factor structure of DMEE-Class-T questionnaire and the CSS-T questionnaire, the bifactor exploratory structural equation modeling (Morin et al., 2016, 2020) has been used. By contrasting competing models, this analytical framework takes into account two potential sources of psychometric multidimensionality that are commonly observed in multidimensional measures. First, the framework accounts for the simultaneous presence of hierarchically organized constructs by offering a direct and explicit estimate of a global construct (referred to as G-factor), while considering specific factors (referred to as S-factors) that capture the distinct characteristics associated with each individual subscale, beyond what is accounted for by the overarching factor. Secondly, the framework also addresses the inherent imperfections in the indicators used to measure each construct by permitting the estimation of cross-loadings among all factors that represent the global construct. Five alternative models have been considered in the present study for both instruments. We first tested a one-factor model. Then we tested the usual independent cluster model confirmatory factor analysis (ICM-CFA) requiring that each item is defined by only one latent factor. By fixing all cross-loadings at zero, correlations between latent factors are artificially inflated (Morin et al., 2020). In exploratory structural equation modeling (ESEM, Asparouhov and Muthén, 2009), a target rotation is used to freely estimate cross-loadings between non-target constructs and imperfect items. Compared with ICM-CFA, ESEM provides more accurate estimates of factor correlations (Asparouhov and Muthén, 2009; Morin et al., 2016). If factors are well-defined by large target factor loadings in the ESEM solution, the factor correlations matrix is examined. If there is a substantial difference in the size of factor correlations between CFA and ESEM, the latter model is preferred as it provides more exact estimates (Asparouhov et al., 2015). If differences between factor correlations are not substantial, then CFA is preferred according to the parsimony principle. Moreover, if there are numerous large cross-loadings in the ESEM model, the existence of an unmodeled general factor is suggested, which can be tested with a bifactor representation. The bifactor CFA (Reise, 2012) and the bifactor ESEM (Morin et al., 2016) assume the existence of a global factor accounting for the shared variance by all items and specific group factors explaining the residual variance beyond that global factor. In the bifactor CFA, orthogonality is assumed between the general factor and the specific factors. In the bifactor ESEM, the relations between non-target constructs and items are considered. Importantly, while bifactor models usually tend to show better goodness-of-fit indices (Bonifay et al., 2017), model specification must be anchored in theory (Morin et al., 2020).
All analyzes were conducted using the robust weighted least squares mean and variance adjusted (WLSMV) estimator as implemented in Mplus 8.3 (Muthén and Muthén, 2012-2019) to respect the ordinal level of measurement of Likert-type items. The WLSMV estimator permit that all available information is used (missing values are handled using pairwise present) and has been found to outperform maximum-likelihood estimation methods for ordered-categorical items with asymmetric thresholds (Finney and DiStefano, 2013). As teachers are nested in schools, we took into account the hierarchical nature of the data using the Mplus design-based adjustment implemented by the TYPE = COMPLEX function (Asparouhov, 2005).
The adequacy of all models was assessed using several indices (CFI, TLI, RMSEA, and SRMR) as well as typical interpretation guidelines (Hu and Bentler, 1999). CFI and TLI above 0.90 and 0.95 as well as RMSEA and SRMR below 0.08 and 0.06 were considered as reflecting adequate and excellent fit, respectively. The relative changes in CFI, TLI, RMSEA were examined to compare the nested measurement invariance models and a decrease of 0.010 or higher in CFI and TLI or an increase of 0.015 or higher in RMSEA was considered as indicating a lack of invariance or a lack of similarity (Cheung and Rensvold, 2002; Chen, 2007).
To assess reliability, we reported the McDonald’s model-based composite reliability (CR) index. CR values above 0.50 are considered as acceptable (Perreira et al., 2018). For bifactor models, we also computed the omega (ω) and omega hierarchical (ωH) indices (Rodriguez et al., 2016). Omega represents the proportion of variability in the overall score explained by both the general factor (G-factor) and the specific factors (S-factors), while omega hierarchical gives the proportion of variance in the total score that can be attributed to the G-factor only. To determine that using a total score is justified, ωH is divided by ω to get the Explained Common Variance (ECV). Using the total score is justified when ECV exceeds 0.75, as recommended by Reise et al. (2013).
To examine the concurrent validity of the DMEE-Class-T, Pearson’s product–moment correlations were computed between the new scale dimensions and the CSS-T dimensions. As suggested by Cohen (1988), correlations between 0.10 and 0.30 were considered as small, those between 0.31 and 0.50 as medium, and those over 0.51 as large.
We began by testing the one-factor, ICM-CFA, and ESEM representations for both instruments. Fit indices are provided in Table 1 while factors correlations and standardized estimates are reported in Supplementary Tables S3–S8 in the Supplementary material.
Concerning the DMEE-Class-T, CFI, TLI, RMSEA and SRMR indices did not reach the recommended cut-offs for the one-factor model and the ICM-CFA model while the ESEM solution showed excellent fit indices. Factor correlations were considerably reduced in the correlated factors ESEM solution (|r| = 0.001 to 0.547, M|r| = 0.266) relative to the correlated factors CFA solution (|r| = 0.502 to 0.903, M|r| = 0.748). When inspecting the standardized estimates for the ESEM model, we however noticed that some target loadings related to items assessing the differentiation facet of several factors were particularly weak or even opposed to the other items of the target dimension (items 8, 16, 17, 38, 43, and 44). Moreover, these items also had strong non-target cross-loadings. This observation led us to consider the existence of an additional differentiation factor beside the seven other factors. We tested this second version of the DMEE-Class-T with 8 sub-dimensions and fit indices were excellent for the ICM-CFA solution and much better for the ESEM solution (ΔCFI = +0.045; ΔTLI = +0.034; ΔRMSEA = −0.015; ΔSRMR = −0.038). Factor correlations (see Supplementary Table S3) were again considerably reduced in the correlated factors ESEM solution (|r| = 0.025 to 0.511, M|r| = 0.265) relative to the correlated factors CFA solution (|r| = 0.366 to 0.901, M|r| = 0.624). Thus, goodness-of-fit information and factor correlations converge in supporting the correlated factors ESEM solution with 8 sub-dimensions. This solution was contrasted with its bifactor counterpart, as 201 statistically significant cross-loadings (of which 19 over 0.300) were observed in the ESEM solution. Fit indices for the bifactor ESEM solution (Table 1) were also excellent and just slightly better than for the ESEM solution (ΔCFI = +0.002; ΔTLI = +0.003; ΔRMSEA = −0.001; ΔSRMR = −0.003). As increases in CFI/TLI and decreases in RMSEA/SRMR between the bifactor-ESEM solution and the ESEM solution are below the recommended cut-offs, it was decided to retain the more parsimonious ESEM model as the best factorial representation of our data concerning the DMEE-Class-T. Standardized estimates are reported in Supplementary Tables S5 and S6, respectively for the ICM-CFA, the ESEM and the bifactor ESEM solutions. The correlated factors ESEM (Orientation: |λ| = 0.525 to 0.913, M|λ| = 0.691; Structuration: |λ| = 0.105 to 0.553, M|λ| = 0.324; Questioning: |λ| = 0.023 to 0.760, M|λ| = 0.358; Modeling: |λ| = 0.158 to 0.643, M|λ| = 0.346; Application: |λ| = 0.285 to 0.779, M|λ| = 0.530; Learning environment: |λ| = 0.333 to 0.572, M|λ| = 0.474; Assessment: |λ| = 0.156 to 0.837, M|λ| = 0.544; Differentiation: |λ| = 0.621 to 0.765, M|λ| = 0.679) resulted in at least moderately well-defined factors for all dimensions of effective teaching practices.
Concerning the CSS-T questionnaire, CFI, TLI, RMSEA and SRMR indices (Table 1) were acceptable for the one-factor model, excellent for the ICM-CFA model, and yet better for the ESEM solution (ΔCFI = +0.031; ΔTLI = +0.030; ΔRMSEA = −0.018; ΔSRMR = −0.029 in comparison to the ICM-CFA solution). Factor correlations (see Supplementary Table S3) were moreover considerably reduced in the correlated factors ESEM solution (|r| = 0.010 to 0.593, M|r| = 0.335) relative to the correlated factors CFA solution (|r| = 0.651 to 0.923, M|r| = 0.799). The ESEM solution was considered as better than the ICM-CFA solution. Due to the presence of 181 statistically significant cross-loadings (of which 18 over 0.300) in the ESEM solution, we tested a bifactor ESEM representation. Fit indices for this model (see Table 1) were also excellent but just slightly better than for the ESEM solution (ΔCFI = +0.001; ΔTLI = +0.001; ΔRMSEA = −0.001; ΔSRMR = −0.002). As increases in CFI/TLI and decreases in RMSEA/SRMR between the bifactor-ESEM solution and the ESEM solution are below the recommended cut-offs, it was decided to retain the more parsimonious ESEM model as the best factorial representation of our data concerning the CSS-T instrument. The correlated factors ESEM were relatively well-defined (Student focused learning and engagement: |λ| = 0.171 to 0.857, M|λ| = 0.438; Instructional delivery: |λ| = 0.285 to 0.912, M|λ| = 0.522; Promotes student thinking: |λ| = 0.381 to 0.712, M|λ| = 0.531; Academic performance feedback: |λ| = 0.201 to 0.725, M|λ| = 0.451; Praise: |λ| = 0.275 to 0.611, M|λ| = 0.406; Corrective feedback: |λ| = 0.216 to 0.956, M|λ| = 0.511; Directives/transitions: |λ| = 0.304 to 0.659, M|λ| = 0.499) but it was not the case for the Prevention management factor which was poorly defined (|λ| = 0.032 to 0.286, M|λ| = 0.138).
Reliability indicators (Table 2) show that most subscales had adequate levels (>0.500) of McDonald’s composite reliability (CR) except for the Prevention management factor in the CSS-T.
Pearson’s correlation between the factor scores derived from the ESEM solutions for the DMEE-Class-T and the CSS-T are reported in Table 3. Remarkably, almost all correlations are statistically significant, but at low or moderate levels. In other words, the eight factors assessed through the DMEE-Class-T instrument could be seen as a good predictors of other measures of effective teaching practices assessed through the CSS-T questionnaire, supporting the concurrent validity of the new instrument.
To study instructional quality, generic, subject-specific or hybrid frameworks have been developed (Charalambous and Litke, 2018; Senden et al., 2021). In the present study, a generic questionnaire (DMEE-Class-T) assessing the use of effective teaching practices has been developed for teachers to complement the existing observation instruments and student questionnaire aimed at measuring the classroom level of the Dynamic Model of Educational Effectiveness. The concurrent validity of the DMEE-Class-T was assessed and confirmed in reference to another self-reported instrument (CSS-T; Reddy et al., 2015) about effective teaching practices. Based on theory and previous work, a CFA with 8 correlated factors was expected for both instruments (with different factors however), but the very strong factor correlations in the ICM-CFA models suggested discriminant validity issues. For both instruments, we tested several competing factorial models using the bifactor exploratory structural equation modeling framework (Morin et al., 2016, 2020). The ESEM model showed better fit indices than the CFA model. For parsimony reason, the ESEM solution was retained against its bifactor counterpart. Therefore, the ESEM solution was considered as the best representation of data for the DMEE-Class-T and the CSS-T in the present study. These findings confirmed that scale items may not be flawless indicators of the factors they are intended to measure. Consequently, it is suggested to systematically compare CFA and ESEM models in order to obtain a more accurate representation of the data. These findings are in line with other theories and studies rejecting the unidimensionality vision of the instructional quality and distinguishing several groups of effective teaching practices (i.e., Seidel and Shavelson, 2007; Klieme et al., 2009).
The use of the eight factor scores calculated from the DMEE-Class-T and those calculated from the CSS-T (with the exception of the Prevention management scale) may have relevance for people interested in identifying specific effective teaching practices that are insufficiently used or those whose quality needs to be improved. This is true for people who assess teachers with formative purpose, but also to give a place to the teachers in this process by including an input on their practices from their point of view. In this sense, as suggested by Reddy et al. (2015), the DMEE-Class-T or the CSS-T could usefully be integrated into multimethod systems of support for enhancing professional development, offering constructive feedback and support, and guiding teachers’ professional growth,. Maybe more importantly, we believe that such self-reported assessments of effective teaching practices offer valuable insights into how teachers perceive their own teaching methods, fostering self-awareness, and helping them identify areas for improvement, particularly in the context of multi-tiered systems of support where the instructional quality of the tier-1 intervention with all students is critical.
Despite the relevance of our findings, the present study has several limitations. First, it should be remembered that the two instruments examined are self-reporting measurement instruments, which are known to potentially produce biased data, particularly because of social desirability. We have tried to limit this bias by guaranteeing the anonymity of the answers given by the teachers, but we cannot exclude it. Secondly, for the CSS-T, we did not follow the authors’ recommendations, which suggest constructing discrepancy scores by asking teachers to rate their use of the practices but also the frequency they should ideally have used. Thirdly, country specificities and demographic characteristics of Luxembourgish teachers are relatively unique. It is plausible that our findings are not generalizable to other education systems and further research is needed for confirmation or invalidation.
The DMEE-Class-T was developed to be a useful complementary instrument in reference to the already existing instruments developed to measure the variables within each level defined in the Dynamic Model of Educational Effectiveness. The findings need to be confirmed by further research applying the same factorial analysis framework, conducting invariance testing, and comparing self-reported data to observational data. It would also be interesting to assess the predictive validity of such self-reported use of effective teaching practices on student achievement and to examine if the instrument could be used as a tool to measure and enhance teachers’ professional development.
The raw data supporting the conclusions of this article will be made available by the authors, without undue reservation.
The studies involving humans were approved by Ethics Review Panel of the University of Luxembourg. The studies were conducted in accordance with the local legislation and institutional requirements. The participants provided their written informed consent to participate in this study.
CD: Writing – original draft, Writing – review & editing.
The author(s) declare financial support was received for the research, authorship, and/or publication of this article. This study was commissioned by the National Observatory for School Quality and funded by the Ministry of Education and Youth.
The author declares that the research was conducted in the absence of any commercial or financial relationships that could be construed as a potential conflict of interest.
All claims expressed in this article are solely those of the authors and do not necessarily represent those of their affiliated organizations, or those of the publisher, the editors and the reviewers. Any product that may be evaluated in this article, or claim that may be made by its manufacturer, is not guaranteed or endorsed by the publisher.
The Supplementary material for this article can be found online at: https://www.frontiersin.org/articles/10.3389/feduc.2023.1281431/full#supplementary-material
1. ^There are 4 two-year learning cycles (C1, C2, C3, C4) in the Luxembourgish elementary school system.
Ainley, J., and Carstens, R. (2018). Teaching and Learning International Survey (TALIS) 2018 Conceptual Framework, OECD Education Working Papers, No. 187, OECD Publishing, Paris. doi: 10.1787/799337c2-en
Anderson, J. C., and Gerbing, D. W. (1988). Structural equation modeling in practice: a review and recommended two-step approach. Psychol. Bull. 103, 411–423. doi: 10.1037/0033-2909.103.3.411
Asparouhov, T. (2005). Sampling weights in latent variable modeling. Structural Equation Modeling: A Multidisciplinary Journal, 12(3), 411–434. doi: 10.1207/s15328007sem1203_4
Asparouhov, T., and Muthén, B. (2009). Exploratory structural equation modeling. Struct. Equ. Model. 16, 397–438. doi: 10.1080/10705510903008204
Asparouhov, T., Muthen, B., and Morin, A. J. S. (2015). Bayesian structural equation modeling with cross-loadings and residual covariances. J. Manag. 41, 1561–1577. doi: 10.1177/0149206315591075
Azigwe, J. B., Kyriakides, L., Panayiotou, A., and Creemers, B. P. M. (2016). The impact of effective teaching characteristics in promoting student achievement in Ghana. Int. J. Educ. Dev. 51, 51–61. doi: 10.1016/j.ijedudev.2016.07.004
Bonifay, W., Lane, S. P., and Reise, S. P. (2017). Three concerns with applying a bifactor model as a structure of psychopathology. Clin. Psychol. Sci. 5, 184–186. doi: 10.1177/2167702616657069
Brown, C., and Malin, J. (2022). The emerald handbook of evidence-informed practice in education. Emerald Publishing Limited.
Charalambous, C. Y., and Litke, E. (2018). Studying instructional quality by using a content-specific lens: the case of the mathematical quality of instruction framework. ZDM 50, 445–460. doi: 10.1007/s11858-018-0913-9
Charalambous, C., and Praetorius, A.-K. (2023). “Theorizing teaching: synthesizing expert opinion to identify the next steps” in Theorizing teaching: Current status and open issues. eds. A.-K. Praetorius and C. Charalambous (Cham: Springer).
Chen, F. F. (2007). Sensitivity of goodness of fit indexes to lack of measurement invariance. Struct. Equ. Model. 14, 464–504. doi: 10.1080/10705510701301834
Cheung, G. W., and Rensvold, R. B. (2002). Evaluating goodness-of-fit indexes for testing measurement invariance. Struct. Equ. Model. 9, 233–255. doi: 10.1207/S15328007SEM0902_5
Clausen, M. (2002). Qualität von Unterricht: Eine Frage der Perspektive? [Quality of instruction as a question of perspective?]. Waxmann.
Cohen, J. (1988). Statistical power analysis for the behavioral sciences, 2nd Edn. Oxfordshire: Erlbaum.
Creemers, B., and Kyriakides, L. (2008). The dynamics of educational effectiveness: A contribution to policy, practice, and theory in contemporary schools. Routledge.
Creemers, B., and Kyriakides, L. (2012). Improving quality in education. Dynamic approaches to school improvement. Routledge.
Desimone, L. M., Smith, T. M., and Frisvold, D. E. (2010). Survey measures of classroom instruction: comparing student and teacher reports. Educ. Policy 24, 267–329. doi: 10.1177/0895904808330173
DeVellis, R. F. (2012). Scale development: Theory and applications (3rd ed.). Thousand Oaks, CA: Sage.
Dimosthenous, A., Kyriakides, L., and Panayiotou, A. (2020). Short- and long-term effects of the home learning environment and teachers on student achievement in mathematics: a longitudinal study. Sch. Eff. Sch. Improv. 31, 50–79. doi: 10.1080/09243453.2019.1642212
Fauth, B., Göllner, R., Lenske, G., Praetorius, A. K., and Wagner, W. (2020). Who sees what? Zeitschrift für Pädagogik 66, 138–155.
Finney, S. J., and DiStefano, C. (2013). “Non-normal and categorical data in structural equation modeling” in Structural equation modeling: A second course. eds. G. R. Hancock and R. O. Mueller. 2nd ed (Charlotte, North Carolina: Information Age Publishing), 439–492.
Göllner, R., Fauth, B., and Wagner, W. (2021). “Student ratings of teaching quality dimensions: empirical findings and future directions”, in Student feedback on teaching in schools. eds. W. Rollett, H. Bijlsma, and S. Röhl (Switzerland, AG: Springer).
Hu, L.-T., and Bentler, P. M. (1999). Cutoff criteria for fit indexes in covariance structure analysis: conventional criteria versus new alternatives. Struct. Equ. Model. Multidiscip. J. 6, 1–55. doi: 10.1080/10705519909540118
Klieme, E., Pauli, C., and Reusser, K. (2009). “The pythagoras study: investigating effects of teaching and learning in Swiss and German mathematics classrooms” in The power of video studies in investigating teaching and learning in the classroom. eds. T. Janik and T. Seider (Munster: Waxmann), 137–160.
Koziol, S. M., and Burns, P. (1986). Teachers’ accuracy in self-reporting about instructional practices using a focused self-report inventory. J. Educ. Res. 79, 205–209. doi: 10.1080/00220671.1986.10885678
Kraft, M. A., and Gilmour, A. F. (2017). Revisiting the widget effect: teacher evaluation reforms and the distribution of teacher effectiveness. Educ. Res. 46, 234–249. doi: 10.3102/0013189X17718797
Kyriakides, L., Archambault, I., and Janosz, M. (2013). Searching for stages of effective teaching: a study testing the validity of the dynamic model in Canada. J. Classr. Interact. 48, 11–24.
Kyriakides, L., Creemers, B., and Panatyiotou, A. (2012). Report of the data analysis of the student questionnaire used to measure teacher factors: Across and within country results. Available at: https://websites.ucy.ac.cy/esf/documents/Analysis_of_Data/Analysis_of_Student_Questionnaire/D12b_Report_student_questionnaire_analysis.pdf (Accessed 5 July 2023).
Kyriakides, L., Creemers, B., and Panayiotou, A. (2018). Using educational effectiveness research to promote quality of teaching: the contribution of the dynamic model. ZDM 50, 381–393. doi: 10.1007/s11858-018-0919-3
Kyriakides, L., Creemers, B., Panayiotou, A., and Charalambous, E. (2020). Quality and equity in education. Revisiting theory and research on educational effectiveness and improvement. ProQuest Ebook Central.
Kyriakides, L., and Panayiotou, A. (2023). “Using educational effectiveness research for promoting quality of teaching: the dynamic approach to teacher and school improvement” in Effective teaching around the world. eds. R. Maulana, M. Helms-Lorenz, and R. M. Klassen (Cham: Springer).
Lazarides, R., and Schiefele, U. (2021). The relative strength of relations between different facets of teacher motivation and core dimensions of teaching quality in mathematics. Learn. Instr. 76:101489. doi: 10.1016/j.learninstruc.2021.101489
Levin, B. (2011). Mobilising research knowledge in education. Lond. Rev. Educ. 9, 15–26. doi: 10.1080/14748460.2011.550431
Little, O., Goe, L., and Bell, C. (2009). A practical guide to evaluating teacher effectiveness. Washington, DC: National Comprehensive Center for Teacher Quality.
Morin, A. J. S., Arens, A. K., and Marsh, H. W. (2016). A bifactor exploratory structural equation modeling framework for the identification of distinct sources of construct-relevant psychometric multidimensionality. Struct. Equ. Model. 23, 116–139. doi: 10.1080/10705511.2014.961800
Morin, A. J. S., Myers, N. D., and Lee, S. (2020). “Modern factor analytic techniques: Bifactor models, exploratory structural equation modeling (ESEM) and bifactor-ESEM” in Handbook of sport psychology. eds. G. Tenenbaum and R. C. Eklund. 4th ed (Hoboken, New Jersey: Wiley), 1044–1073.
Muthén, L. K., and Muthén, B. O. (2012-2019). Mplus user’s guide: Statistical analysis with latent variables (8.3). Los Angeles, CA: Muthén & Muthén.
Panayiotou, A., Kyriakides, L., Creemers, B. P. M., McMahon, L., Vanlaar, G., Pfeifer, M., et al. (2014). Teacher behavior and student outcomes: results of a European study. Educ. Assess. Eval. Account. 26, 73–93. doi: 10.1007/s11092-013-9182-x
Perreira, T. A., Morin, A. J., Hebert, M., Gillet, N., Houle, S. A., and Berta, W. (2018). The short form of the workplace affective commitment multidimensional questionnaire (WACMQ-S): a bifactor-ESEM approach among healthcare professionals. J. Vocat. Behav. 106, 62–83. doi: 10.1016/j.jvb.2017.12.004
Reddy, L. A., and Dudek, C. M. (2014). Teacher progress monitoring of instructional and behavioral management practices: an evidence-based approach to improving classroom practices. Int. J. Sch. Educ. Psychol. 2, 71–84. doi: 10.1080/21683603.2013.876951
Reddy, L. A., Dudek, C. M., Fabiano, G. A., and Peters, S. (2015). Measuring teacher self-report on classroom practices: construct validity and reliability of the classroom strategies scale-teacher form. Sch. Psychol. Q. 30, 513–533. doi: 10.1037/spq0000110
Reddy, L. A., Hua, A. N., Dudek, C. M., Kettler, R., Arnold-Berkovits, I., Lekwa, A., et al. (2021). The relationship between school administrator and teacher ratings of classroom practices and student achievement in high-poverty schools. Assess. Eff. Interv. 46, 87–98. doi: 10.1177/1534508419862863
Reise, S. P. (2012). The rediscovery of Bifactor measurement models. Multivar. Behav. Res. 47, 667–696. doi: 10.1080/00273171.2012.715555
Reise, S. P., Bonifay, W. E., and Haviland, M. G. (2013). Scoring and modeling psychological measures in the presence of multidimensionality. J. Pers. Assess. 95, 129–140. doi: 10.1080/00223891.2012.725437
Rodriguez, A., Reise, S. P., and Haviland, M. G. (2016). Applying bifactor statistical indices in the evaluation of psychological measures. J. Pers. Assess. 98, 223–237. doi: 10.1080/00223891.2015.1089249
Roy, A., Guay, F., and Valois, P. (2013). Teaching to address diverse learning needs: development and validation of a differentiated instruction scale. Int. J. Incl. Educ. 17, 1186–1204. doi: 10.1080/13603116.2012.743604
Sánchez-Cabrero, R., Estrada-Chichón, J. L., Abad-Mancheño, A., and Mañoso-Pacheco, L. (2021). Models on teaching effectiveness in current scientific literature. Educ. Sci. 11:409. doi: 10.3390/educsci11080409
Scheerens, J. (2016). Educational effectiveness and ineffectiveness: A critical review of the Knowledge Base. Dordrecht: Springer.
Seidel, T., and Shavelson, R. J. (2007). Teaching effectiveness research in the past decade: the role of theory and research design in disentangling meta-analysis results. Rev. Educ. Res. 77, 454–499. doi: 10.3102/0034654307310317
Senden, B., Nilsen, T., and Blömeke, S. (2021). “Instructional quality: a review of conceptualizations, measurement approaches, and research findings” in Ways of analyzing teaching quality. eds. M. Blikstad-Balas, K. Klette, and M. Tengberg. Red ed (Scandinavian University Press), 140–172.
Teodorović, J., Milin, V., Bodroža, B., Ivana, D., Derić, I. D., Vujačić, M., et al. (2022). Testing the dynamic model of educational effectiveness: the impact of teacher factors on interest and achievement in mathematics and biology in Serbia. Sch. Eff. Sch. Improv. 33, 51–85. doi: 10.1080/09243453.2021.1942076
Van de Vijver, F., and He, J. (2014). Report on social desirability, midpoint and extreme responding in TALIS 2013. OECD Education Working Papers, No. 107. OECD Publishing, Paris.
Vanlaar, G., Kyriakides, L., Panayiotou, A., Vandecandelaere, M., McMahon, L., De Fraine, B., et al. (2016). Do the teacher and school factors of the dynamic model affect high- and low-achieving student groups to the same extent? A cross-country study. Res. Pap. Educ. 31, 183–211. doi: 10.1080/02671522.2015.1027724
Wagner, W., Göllner, R., Werth, S., Voss, T., Schmitz, B., and Trautwein, U. (2016). Student and teacher ratings of instructional quality: consistency of ratings over time, agreement, and predictive power. J. Educ. Psychol. 108, 705–721. doi: 10.1037/edu0000075
Keywords: teacher assessment, classroom practices, evaluation, professional development, self-report, Dynamic Model of Educational Effectiveness, classroom strategies assessment system
Citation: Dierendonck C (2023) Measuring the classroom level of the Dynamic Model of Educational Effectiveness through teacher self-report: development and validation of a new instrument. Front. Educ. 8:1281431. doi: 10.3389/feduc.2023.1281431
Received: 22 August 2023; Accepted: 29 September 2023;
Published: 19 October 2023.
Edited by:
Aldo Bazán-Ramírez, Universidad Nacional José María Arguedas, PeruReviewed by:
Walter Capa, National University Federico Villareal, PeruCopyright © 2023 Dierendonck. This is an open-access article distributed under the terms of the Creative Commons Attribution License (CC BY). The use, distribution or reproduction in other forums is permitted, provided the original author(s) and the copyright owner(s) are credited and that the original publication in this journal is cited, in accordance with accepted academic practice. No use, distribution or reproduction is permitted which does not comply with these terms.
*Correspondence: Christophe Dierendonck, Y2hyaXN0b3BoZS5kaWVyZW5kb25ja0B1bmkubHU=
Disclaimer: All claims expressed in this article are solely those of the authors and do not necessarily represent those of their affiliated organizations, or those of the publisher, the editors and the reviewers. Any product that may be evaluated in this article or claim that may be made by its manufacturer is not guaranteed or endorsed by the publisher.
Research integrity at Frontiers
Learn more about the work of our research integrity team to safeguard the quality of each article we publish.