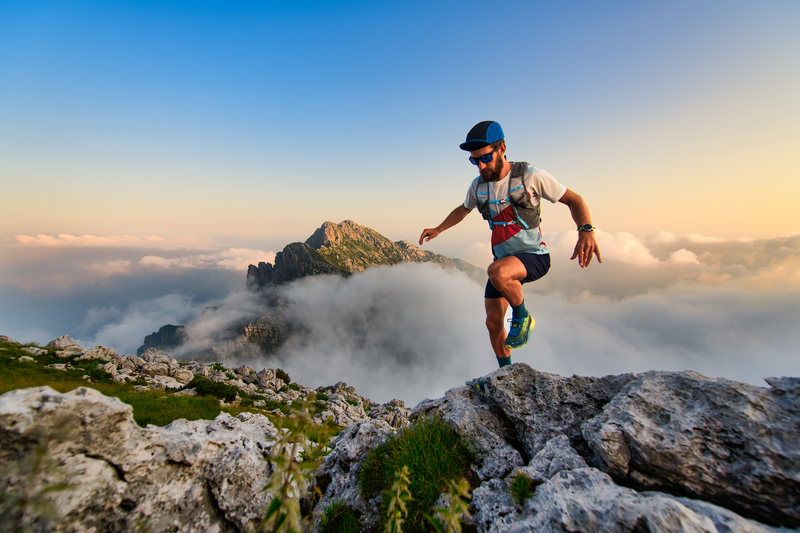
94% of researchers rate our articles as excellent or good
Learn more about the work of our research integrity team to safeguard the quality of each article we publish.
Find out more
METHODS article
Front. Educ. , 20 November 2023
Sec. Assessment, Testing and Applied Measurement
Volume 8 - 2023 | https://doi.org/10.3389/feduc.2023.1271335
This article is part of the Research Topic Insights in Assessment, Testing, and Applied Measurement: 2022 View all 21 articles
This study aimed to show the usefulness of Lawshe’s method (1975) in investigating the content validity of measurement instruments under the strategy of expert judgment. The research reviewed the historical use of Lawshe’s method in the social sciences and analyzed the main criticisms of this method using mathematical hypotheses. Subsequently, we experimented with an instrument designed to determine the pedagogical skills possessed by students undertaking initial teacher training in Chile. The results showed that in Lawshe’s proposal, it is essential to highlight the need to reconsider the sum of all Content Validity Ratio (CVR) indices for the calculation of the context validity index and not only the indices that exceed the critical Content Validity Index (CVI) given the greater power of discrimination presented by the latter alternative.
In research, especially in education, measurement instruments are valuable tools to generate and structure complex constructs and convert them into analyzable parameters (Juárez-Hernández and Tobón, 2018). Implementing these instruments entails using statistical techniques that allow their validity to be demonstrated in the measurement field. In this sense, scientific organizations recommend considering the following sources of validity: content, response processes, internal structure, relations with other variables, and evaluation consequences (Eignor, 2013; Plake and Wise, 2014). Measuring the correspondence between the content of the items and the evaluated content is very relevant for evaluating content validity.
The literature identifies two approaches for addressing the content analysis of a measurement instrument: the first is related to the methods based on expert judgment; the second is related to the methods derived from the application of measuring instruments (Pedrosa et al., 2014; Urrutia et al., 2014). These methods aim to collect evidence on two sources of validity: the definition of the items’ domain (representativeness) and the adequacy of the content (relevance). This study opted to focus on methods based on expert judgment.
According to Urrutia et al. (2014), before carrying out content analysis under the expert judgment strategy, the researcher must resolve two critical issues: first, determine what can be measured, and second, define the number and characteristics of the experts who will participate in evaluating the relevance of the instrument items. Moreover, the variability of the number of participants during the expert judgment, their suitability concerning the study’s objective, their work activity, and their geographical area of origin. However, the literature on measurement instruments indicates that when defining the number of experts for a panel, the researcher must predict the type of statistical analysis that will be carried out with the responses obtained in such a way that the number of selected participants is equal to or greater than the minimum number to the minimum number of judges for the statistical test to be valid (Tristán-López, 2008).
The importance of defining statistical parameters (measures) in statistics (Statistical Measure)lies in the fact that the qualitative assessment of items is not sufficient —to determine the degree of agreement of evaluators on the suitability of an item (Sireci, 1998).
In the search for indices to calculate inter-judge agreement, we reviewed different methods used in the social sciences to calculate content validity. In this review, the proposal of Lawshe (1975). This strategy considers a panel of judges with expertise in the construct of the instrument, and each specialist individually evaluates the items associated with said construct. Lawshe (1975) suggested that under sociological principles, the minimum inter-judge agreement should be 50%, and the use of two indices: the content validity ratio (CVR), which measures the agreement of the panelists on an item, and the CVI, which presents the average of the CVR that constitutes the final instrument. The CVR can be presented as follows:
where ne is the number of panelists in agreement and nne, is the number of panelists in disagreement.
A CVR index is considered acceptable depending on the level of agreement of the panelists regarding an item. Lawshe (1975) presented a table of the critical values of the CVR index according to the number of panelists. For the research exemplified below, it was necessary to find an index that applied to seven judges who participated in the process. For Lawshe, the critical CVR of a 7-member panel of experts should be equal to or greater than 0.75, a value that would allow the Interjudge agreement to be considered statistically valid.
Polit et al. (2007) suggested a critical CVR value equal to.78 for three or more panelists. It represents an advance concerning Lawshe, who proposed a critical CVR calculation with at least five panelists. In any case, the proposal by Polit et al. does not apply to the social sciences because, although it supports a smaller number of panelists, the recommended critical CVR is too low for panels of five or fewer experts, thus losing discriminant validity. Likewise, in panels with six or more members, it is objectionable for the critical CVR value to remain very close to that defined for three panelists, as it would contradict studies on the indirectly proportional relation between the number of rating experts on a panel and the critical value of CVR (Tristán-López, 2008; Wilson et al., 2012; Ayre and Scally, 2014).
Tristán-López (2008) pointed out that Lawshe’s formula does not apply to panels with fewer than five members and further generated a critical CVR table, revealing, unlike Lawshe, the statistical test he used (Chi-squared = χ2(α = 0.1, gl = 1)), while Lawshe had used the value of α =0. 05 as significance level without mentioning the test used, A question that, to date, represents the most significant criticism of the work of the latter author. Although Tristán-López’s proposal represents an advance concerning the previous ones, and the use of the statistic used in the critical CVR table for each number of evaluating experts is made explicit, the increase of the admissible error to the value 1 (α =0.1) is not justified.
As an effect of the above, we observe that the higher the value of α, the better the probability of calculating the critical values for a smaller number of panelists, which is beneficial from the point of view of reducing the number of panelists but not from the perspective of the error of permissible significance and, therefore, of the level of exigency of the proof. On the other hand, according to Ayre and Scally (2014), using the Tristan-López chi-square test is inadequate, considering that the data with which the RCV is calculated would have a binomial distribution. Consequently, the statistical modalization of Tristán-López (2008) to generate the critical CVR table is inappropriate.
Wilson et al. (2012) proposed a new critical CVR table using the normal approximation for a binomial distribution under the assumption of the central limit theorem, in which the rating expert’s answers do not interact with each other (each rating expert is an independent variable). The complexity of this interesting proposal for its application in social sciences lies in the fact that this approach is valid only for a considerable size of panelists, which corresponds to another of the assumptions of the central limit theorem and represents a great difficulty for the search for expert judges.
Ayre and Scally (2014) proposed a new critical CVR table using the exact binomial test (EBT). Unlike the chi-squared test used by Tristán-López (2008) and the normal approximation of Wilson et al. (2012), the EBT is an appropriate alternative to the model proposed by Lawshe for the following reasons. Lawshe proposed two response categories (essential and non-essential) such that the data distribution of each expert rater corresponds to a Bernoulli distribution with parameter p = 0.5. If, in addition, each expert rater’s responses are considered independent variables. The criteria of an exact binomial distribution are met, with parameter p = 0.05 and N, the number of expert raters.
Suppose we consider an instrument composed of “n” items evaluated by “N” panelists with a dichotomous scale (“essential” or “non-essential”). Then, modeling the problem statistically and finding the critical value of panelists in agreement that allows an item to be considered essential using the CVR cr index calculated using equation (1), we considered the null hypothesis.
where n e is the number of panelists in agreement, with a significance level equal to 0.05.
The null hypothesis is rejected if the probability of the “number of panelists in agreement is greater than the minimum required number of panelists in agreement with the essential modality ncr” is greater than 0.95.
where n cr is the minimum required number of panelists who agree with the “essential” modality.
Ayre and Scally (2014) calculated the critical values table for each number of panelists using STATA. Using the R program, we compared the three results (Lawshe, 1975; Tristán-López, 2008; Ayre and Scally, 2014).
As shown in Figure 1, the three methods for nine or fewer panelists coincide with the value calculated for critical CVR, but the values differ for ten or more panelists. Given that the method based on the binomial test is more precise than the chi-squared test, proposing an alternative to the CVR index would not change the decision-making under the exact binomial method. However, the Tristán-López (2008) method can be used as an alternative to calculating the critical CVR index when the number of panelists is equal to four (see Table 1).
Figure 1. Comparison of Critical CVR Between Chi-squared and Exact Binomial Methods The graph represents the critical values for the CVR index and their variation based on the number of panelists comparing the proposals of Lawshe (1975), Tristán-López (2008), and Ayre and Scally (2014).
Table 1. Comparison of the Critical Content Validity Ratio (CVR) according to proposals from different authors.
Baghestani et al. (2019) proposed a new method to calculate the critical CVR based on Bayesian statistics. They replaced the null hypothesis given in (1) with.
where p is the realization of a random variable X, whose distribution is unknown. The prior misinformation on the distribution of X was studied by Jeffreys (1935) and Berger (2013), who considered that the previous distribution of X is beta with parameters , denoted by . Given the above, the posterior density function of X is
where f(X; α, β) = dBeta(α, β) is the density function of the variable X, and g(Ne|X) = dbinom(p, N) is the density function of Ne given the variable X.
Thus, the posterior probability of hypothesis H0 is
At a significance level of , the null hypothesis H 0 is rejected if Then, the parameter Ne is determined as the minimum value to reject the null hypothesis considering the model of Berger (2013), with .
Figure 2 shows that with the method developed by Baghestani et al. (2019), the number of judges can be reduced while still obtaining acceptable critical CVR results. This fact is a significant advantage, given the difficulty in obtaining the required judges. However, this conclusion is only possible after reviewing the calculations carried out by Baghestani et al. (2019), which originated in discovering discrepancies regarding using the “pbinom” function of the program’s stats package.
Figure 2. Comparison of Critical CVR Between the Exact Binomial and Bayesian Methods The graph represents the critical values for the CVR index and their variation based on the number of panelists comparing the proposals of Ayre and Scally (2014) and Baghestani et al. (2019).
Compared with the exact binomial method, using Bayesian statistics to calculate the critical CVR helps reduce the number of panelists. This issue became evident after correcting the calculation error in Baghestani et al. (2019). In addition, these alternative shares the advantage of the chi-squared test method to calculate critical CVR (Tristán-López, 2008), where four panelists can evaluate an instrument.
Lawshe (1975) asserted that the CVI value is dependent on the number of panelists and that the following equation would represent it:
Where is the number of items whose CVR values exceed the critical CVR values, and is the value of the CVR index of the accepted item “j”: the number of questions that obtained a CVR greater than the critical CVR.
Lawshe’s criterion can be considered an exaggeration in the sense that, according to the Lawshe model, the value of the CVI of the complete instrument (with accepted and non-accepted items) will not exceed, in any case, the CVIaccepted (calculated from the average of the CVR values for each accepted article). For this reason, we consider the critical value CVI cr de Tilden et al. (1990), which suggests a value greater than 0.7 for the research outlined here.
However, unlike the CVR index, the critical CVI value, which allows accepting or rejecting the content validity of the total instrument, differs between authors. Tilden et al. (1990) suggested that this index is satisfactory, starting at 0.7, whereas Davis (1992) proposed a value of 0.8. The critical CVI value of 0.5 was proposed by Tristán-López (2008). The key question is which of the two indices proposed by Lawshe —CVR and CVI— provides better and more information on the content validity of an instrument? Gilbert and Prion (2016) pointed out that the choice of one index over another depends on the orientation of the study. Based on the above and given that the general objective of our study was to analyze inter-judge agreement against a set of items to interpret their theoretical perspective and improve the instrument, we opted to use the CVI index.
The index used to calculate the CVI (Lawshe, 1975), although less demanding because it considers only the accepted values of the CVR, allows the maintenance of a vision of the relevance and representativeness of the items, mainly because this stage was combined with the analysis of the validity of the metric characteristics of the instrument, called construct validity, where the reliability of the items and the unidimensionality of the factors formed by the items are statistically verified through confirmatory factor analysis (CFA) (Díaz Costa et al., 2015; Romero-Jeldres and Faouzi, 2018). Consequently, and based on the fact that the CVI calculated for the instrument was more significant than 0.7, it was decided to maintain the total of the items elaborated for each dimension of the theoretical construct to which they referred.
Romero-Jeldres and Faouzi (2018) demonstrated that inter-judge agreement is complex, given the cultural elements that mediate the object of study. In the case of pedagogical skills for the exercise of teaching, each country develops different socio-political constructs for their understanding and evaluation, for which an essential adjustment of the Chilean evaluators to the regulatory framework of that country has been observed, in comparison with German evaluators who have shown differences regarding the theoretical frameworks supporting their vision of pedagogical competences for professional practice.
In other words, the CFA considered all the instrument items to verify instrument dimensionality, analyzing the data through various indices, namely, the Joreskög coefficient (Joreskög’s rho), variance extracted index, factorial contribution, and chi-squared statistic divided by the degree of freedom. By combining the strategies for calculating the CVI with the confirmatory factor analysis (CFA) strategy, we reduced the bias of the item evaluators (Juárez-Hernández and Tobón, 2018; Ventura-León, 2019).
The present study was preceded by another research that defined the items associated with the construct to be measured (see variable operationalization Appendices A in Supplementary material) by Romero-Jeldres and Faouzi (2018), The conditions for performing a content validity analysis described by Guion (1977) were partially assured:
1. The domain content had to be rooted in behavior with a generally accepted meaning.
2. The domain content had to be relevant to the measurement objectives.
3. The domain content had to be adequately sampled.
4. Qualified evaluators must have agreed that the domain had been adequately sampled.
5. The content of the responses must have been reliably observed and evaluated.
Therefore, in addition to deciding the type of index to use for content validation, we defined the number of evaluators necessary to perform the validation. This question always turns out to be complex owing to the laboriousness of the task for the evaluators and the low recognition of this type of contribution to the academe. With these difficulties, recruiting a certain number of evaluators with expertise in the research topic is always a challenge.
For this purpose, we adopted expert judgment, defined as an informed opinion of people with experience in the subject and recognized as qualified experts able to provide information, evidence, judgments, and assessments (Cabero et al., 2013). Several expert judgment methods are available, varying in whether the evaluation is done individually or in a group. In all cases, the research problem determines the profile of the specialists. Therefore, defining the attributes of the possible expert persons is a prerequisite, considering as basic requirements having a background, experience, and disposition toward the topic, as well as being willing to review their initial judgment in the development of the study (López-Gómez (2018); Moreno López et al., 2022).
For the present study, we accounted for two additional criteria: the specificity of the content of the object of study and available resources. Akins et al. (2005) suggested that small panels must have at least seven experts to maintain the representation of the information obtained. However, to maintain fairness at the national level, we opted to identify 14 evaluators who met the study criteria in the regions where the sample was collected. We contacted the experts personally and then by email to provide them with all the information and documents needed for the evaluation. The recruitment of the participants was sustained until eight qualified panelists confirmed their willingness to participate. The characterization of the group of experts was highly relevant to the categorical development of the instrument. Table 2 shows the attributes considered.
Based on the Bayesian statistical strategy Baghestani et al., 2019, we calculated the CVR index for each instrument item to define their relevance concerning the purposes of our study. We calculated the CVI for the instrument using Lawshe’s model. This value was compared with the critical value Tilden et al. (1990) defined. This criterion was chosen for the convenience of maintaining all the items. For this purpose, the following equation was used:
where n is the total number of items.
According to Pedrosa et al. (2014), the CVR calculation represents a problem when half of the experts indicate an item as relevant and the other half as irrelevant. Concerning this criticism, we showed that having a certain number of experts makes it possible to define a critical value of CVR = 0, with which it is possible to affirm that even a value close to 0 can be admissible under certain conditions.
About the content validation of the items, we affirm that based on the CVR index of the Lawshe (1975) method and using Tables 1, 56 of the 71 items exceeded 0.71. The calculated CVI value, including all instrument items, was 0.77, which is considered acceptable by Tilden et al. (1990), according to their CVI critical value proposition of 0.7. To verify that from a certain number of experts, a critical value of CVR = 0 can be defined, we implement the following:
Using Table 1 and Equation (1), we found that CVR cr was a decreasing positive function concerning N; thus, critical CVR must be strictly positive for any n e greater than n ne . Equation (4) was obtained using the following (Armitage et al., 2008; Wilson et al., 2012; Ayre and Scally, 2014).
N is extensive, , and the variable z has a standard normal distribution.
Thus, we could formulate the following:
Subsequently, when , where is a fixed integer value greater than 0, the value of CVR approaches 0 as N approaches infinity. Therefore, an item with a CVR value close to 0 would be acceptable if there are many panelists.
The concept of content validity has undergone multiple transformations, but its essence has remained stable since its origin. Pedrosa et al. (2014) reviewed the conceptions developed about content validity, going through perspectives that considered it only for one type of test and others that considered it the basis of construct validity.
Although Lawshe’s (1975) method for analyzing the content validity of a measurement instrument presents numerous advantages over alternatives for these purposes (Divayana et al., 2020), it needs more critical values for the reliability of the CVR calculation and the acceptance of the CVI. Thus, researchers using this method have been compelled to decide between several proposals from different authors.
The present work opted for the method of Baghestani et al. (2019) as an appropriate proposal for variables of unknown distribution. Meanwhile, and about Lawshe’s proposal, it is essential to highlight the need to reconsider the sum of all the CVR indices for calculating the CVI—and not only the indices that exceed the critical CVR—given the greater power of discrimination presented by this last alternative.
We also noted the need to consider mixed methods to provide greater veracity to the content validation process. In this sense, it is helpful to add spaces to the analysis matrix offered to experts to enable them to provide contributions and insights regarding the questions (Urrutia et al., 2014). This fact allows the collection of a broader spectrum of information beyond pertinence and relevance. This type of opening makes it possible to interpret the experts’ responses and understand their frame of reference for their responses, both at the theoretical and representational levels.
Concerning the possible sources of interpretation error (Pedrosa et al., 2014), it is arguable to consider the CVR index equal to 0 as a difficulty when half of the experts indicate an item as relevant and the other half as irrelevant. Lawshe identified the need for an agreement of at least 50%, with which the previously exposed problem is overcome. In addition, we demonstrated the possibility of considering the critical value of CVR = 0 when the number of experts tends toward infinity, allowing for straightforward interpretation.
Although different authors justify statistical indices, such as Lawshe’s CVR, there is a strong tendency to use other strategies that complement these indices to circumvent their limitations. In this sense, it is currently suggested that “once the relevant items have been defined, they should be applied to a set of participants in order to apply the GT [Generalization Theory] to their answers (...), so that it would be possible to quantify the effect of possible sources of error” (Pedrosa et al., 2014, p. 15).
The original contributions presented in the study are included in the article/Supplementary material, further inquiries can be directed to the corresponding author.
MR: Conceptualization, Formal analysis, Funding acquisition, Investigation, Methodology, Project administration, Resources, Supervision, Validation, Writing – original draft, Writing – review & editing. ED: Conceptualization, Investigation, Methodology, Validation, Writing – review & editing. TF: Conceptualization, Data curation, Investigation, Methodology, Validation, Writing – review & editing, Software.
The author(s) declare that no financial support was received for the research, authorship, and/or publication of this article.
The results of this publication have been possible thanks to the financing awarded by DIUMCE of the Metropolitan University of Educational Sciences through the PMI-EXA-PNNI 01-17 Project.
The authors declare that the research was conducted in the absence of any commercial or financial relationships that could be construed as a potential conflict of interest.
All claims expressed in this article are solely those of the authors and do not necessarily represent those of their affiliated organizations, or those of the publisher, the editors and the reviewers. Any product that may be evaluated in this article, or claim that may be made by its manufacturer, is not guaranteed or endorsed by the publisher.
The Supplementary material for this article can be found online at: https://www.frontiersin.org/articles/10.3389/feduc.2023.1271335/full#supplementary-material
Akins, R. B., Tolson, H., and Cole, B. R. (2005). Stability of response characteristics of a Delphi panel: application of bootstrap data expansion. BMC Med. Res. Methodol. 5, 1–12. doi: 10.1186/1471-2288-5-37
Armitage, P, Berry, J., and Matthews, N.S. Statistical methods in medical research. New York: John Wiley & Sons (2008).
Ayre, C., and Scally, A. J. (2014). Critical values for Lawshe's content validity ratio. Meas. Eval. Couns. Dev. 47, 79–86. doi: 10.1177/0748175613513808
Baghestani, A. R., Ahmadi, A., and Meshkat, M. (2019). Bayesian critical values for Lawshe's content validity ratio. Meas. Eval. Couns. Dev. 52, 69–73. doi: 10.1080/07481756.2017.1308227
Berger, J O. Statistical decision theory and Bayesian analysis. Berlin: Springer Science & Business Media (2013).
Cabero, J., del Carmen, M., and Llorente, F. (2013). La aplicación del juicio de experto como técnica de evaluación de las tecnologías de la información y comunicación (TIC). Revista Eduweb 7, 11–22.
Davis, L. (1992). Instrument review: getting the most from a panel of experts. Appl. Nurs. Res. 5, 194–197. doi: 10.1016/S0897-1897(05)80008-4
Díaz Costa, E., Fernández-Cano, A., Faouzi, T., and Henríquez, C. (2015). Validación del constructo subyacente en una escala de evaluación del impacto de la investigación educativa sobre la práctica docente mediante análisis factorial confirmatorio. Revista de Investigación Educativa 33, 47–63. doi: 10.6018/rie.33.1.193521
Divayana, D. G. H., Suyasa, P. W. A., and Adiarta, A. (2020). Content validity determination of the countenance-tri kaya parisudha model evaluation instruments using Lawshe’s CVR formula. J. Phys. Conf. Ser. 1516:012047. doi: 10.1088/1742-6596/1516/1/012047
Eignor, D. R. (2013). “The standards for educational and psychological testing,” in APA handbook of testing and assessment in psychology, Vol. 1. Test theory and testing and assessment in industrial and organizational psychology. Eds. K. F. Geisinger, B. A. Bracken, J. F. Carlson, J.-I. C. Hansen, N. R. Kuncel, S. P. Reise, and M. C. Rodriguez (American Psychological Association), 245–250.
Gilbert, G. E., and Prion, S. (2016). Making sense of methods and measurement: Lawshe’s content validity index. Clin. Simulation Nursing 12, 530–531.
Jeffreys, H. (1935). Some tests of significance treated by the theory of probability. Math. Proc. Camb. Philos. Soc. 31, 203–222. doi: 10.1017/S030500410001330X
Juárez-Hernández, L. G., and Tobón, S. (2018). Análisis de los elementos implícitos en la validación de contenido de un instrumento de investigación. Revista Espacios 39.
Lawshe, C. H. (1975). A quantitative approach to content validity. Pers. Psychol. 28, 563–575. doi: 10.1111/j.1744-6570.1975.tb01393.x
Moreno López, N. M., and Monroy González, J. D. (2022). Validez de contenido por juicio de expertos del inventario de respuestas de afrontamiento CRI-A dirigido a víctimas del conflicto armado colombiano. Cátedra Villarreal-Psicología, 1. Recuperado a partir de. Available at: https://revistas.unfv.edu.pe/CVFP/article/view/137810.3389/feduc.2023.1271335
López-Gómez, E. (2018). El método Delphi en la investigación actual en educación: Una revisión teórica y metodológica. Educación XXI 1, 17–40.
Pedrosa, I., Suárez-Álvarez, J., and García-Cueto, E. (2014). Evidencias sobre la validez de contenido: Avances teóricos y métodos para su estimación. Revista Acción Psicológica 10, 3–20. doi: 10.5944/ap.10.2.11820
Plake, B. S., and Wise, L. L. (2014). "what is the role and importance of the revised AERA, APA, NCME standards for educational and psychological testing?" educational. Measurement 33, 4–12. doi: 10.1111/emip.12045
Polit, D. F., Tatano, C. B., and Owen, S. (2007). Is the CVI an acceptable indicator of content validity? Appraisal and recommendations. Res. Nurs. Health 30, 459–467. doi: 10.1002/nur.20199
Romero-Jeldres, M., and Faouzi, T. (2018). Validación de un modelo de competencias pedagógicas para docentes de Educación Media Técnica. Educación y Educadores 21, 114–132. doi: 10.5294/edu.2018.21.1.6
Sireci, S. G. (1998). The construct of content validity. Soc. Indic. Res. 45, 83–117. doi: 10.1023/A:1006985528729
Tilden, V. P., Nelson, C., and May, B. A. (1990). Use of qualitative methods to enhance content validity. Nurs. Res. 39, 172–175. doi: 10.1097/00006199-199005000-00015
Tristán-López, A. (2008). Modificación al modelo de Lawshe para el dictamen cuantitativo de la validez de contenido de un instrumento objetivo. Avances en medición 6, 37–48.
Urrutia, E., Marcela, S. B., Araya, M. G., Núñez,, and Camus, M. M. (2014). Métodos óptimos para determinar validez de contenido. Educación Médica Superior 28, 547–558.
Ventura-León, J. (2019). De regreso a la validez basada en el contenido. Adicciones 34, 323–326. doi: 10.20882/adicciones.1213
Keywords: instrument development, content validity index, content validity ratio, panel of experts, Bayesian methods
Citation: Romero Jeldres M, Díaz Costa E and Faouzi Nadim T (2023) A review of Lawshe’s method for calculating content validity in the social sciences. Front. Educ. 8:1271335. doi: 10.3389/feduc.2023.1271335
Received: 02 August 2023; Accepted: 31 October 2023;
Published: 20 November 2023.
Edited by:
Gavin T. L. Brown, The University of Auckland, New ZealandReviewed by:
Dennis Arias-Chávez, Continental University, PeruCopyright © 2023 Romero Jeldres, Díaz Costa and Faouzi Nadim. This is an open-access article distributed under the terms of the Creative Commons Attribution License (CC BY). The use, distribution or reproduction in other forums is permitted, provided the original author(s) and the copyright owner(s) are credited and that the original publication in this journal is cited, in accordance with accepted academic practice. No use, distribution or reproduction is permitted which does not comply with these terms.
*Correspondence: Marcela Romero Jeldres, bWFyY2VsYS5yb21lcm9AdW1jZS5jbA==
Disclaimer: All claims expressed in this article are solely those of the authors and do not necessarily represent those of their affiliated organizations, or those of the publisher, the editors and the reviewers. Any product that may be evaluated in this article or claim that may be made by its manufacturer is not guaranteed or endorsed by the publisher.
Research integrity at Frontiers
Learn more about the work of our research integrity team to safeguard the quality of each article we publish.