- 1Department of Human and Social Sciences, University of Aosta Valley, Aosta, Italy
- 2Interuniversity Department of Regional and Urban Studies and Planning, University of Turin, Turin, Italy
Introduction: Academic satisfaction (AS) is considered by researchers and educators as a key variable to explain both academic success and the main problems of university students. AS is a complex construct affected by a range of factors, both internal and external to the individual. The aim of this study was to analyze the influence of certain individual (motivation and self-regulated learning), social (relationships with other students and with the instructor) and organizational (course organization and class attendance mode) variables on AS during the Covid-19 emergency, when face-to-face learning was replaced with online learning environments.
Methods: We hypothesized a model in which AS would be directly influenced by the social and organizational variables, and indirectly influenced by the social, organizational, and individual variables via the mediation of perceived learning. The study sample comprised 104 students (83.7% female) with a mean age of 26.6 years (SD = 9.8), enrolled on bachelor’s or master’s degree at a Northern Italian University. Participants filled out an online questionnaire, which they were instructed to complete with reference to a single course that they had taken during the second semester of the 2020–21 academic year.
Results: The hypothesized model, tested using a structural equation modelling technique for observed variables, offered an acceptable fit for the data [χ2(3) = 7.569, p = 0.0558; RMSEA = 0.121; CFI = 0.974; SRMR = 0.012]. Perceived learning (R2 = 0.454), was influenced by self-regulated learning (β = 0.243, p < 0.01) and course organization (β = 0.453, p < 0.001); AS (R2 = 0.857) was influenced by relationship with the instructor (β = 0.613, p < 0.001) and course organization (β = 0.221, p < 0.001), as well as by the mediating variable, perceived learning (β = 0.227, p < 0.001). The indirect effects of self-regulated learning and course organization on AS were statistically significant but of low magnitude.
Conclusion: These outcomes point up the active role of students in terms of self-regulated learning and perceived learning and the key role of course organization and the student-instructor relationship in promoting students’ AS during the Covid-19 pandemic, when the learning environment changed abruptly. These findings can usefully inform the work of instructors and instructional designers, including in non-emergency contexts.
1. Introduction
In recent times, the technological revolution has brought about great changes in many aspects of human life, including in the field of education. Specifically, in the domain of higher education, the demand for distance learning has been increasing exponentially, and internet-based education has seen rapid development in universities the world over (Bawaneh, 2021).
Then, the COVID-19 pandemic has added further impetus to the development of distance learning: at the beginning of 2020, when the World Health Organization (WHO) declared the pandemic a public health emergency, the subsequent lockdowns to prevent the spread of infection led to close schools and universities, canceling all face-to-face teaching activities at all levels of education (Wang et al., 2021). According to the International Association of Universities (IAU, 2020), the pandemic-related suspension of school and university classes affected over 1.5 billion students throughout the world. At that point, the only way to keep academic activities going was to implement distance education. Thus, online learning replaced the traditional classroom environment, becoming a major mode of instructional delivery for a considerable period of time (Jaradat and Ajlouni, 2020; Keržič et al., 2021). This unplanned and brusque shift turned distance education into a high-profile emergent research topic, because it offered an unprecedented opportunity to study the impact of remote learning on students, and also to investigate how it might be improved (including with a view to future emergencies) (Koka et al., 2023).
The consensus in the literature is that the learning environment is a significant determinant of students’ learning processes and outcomes (Eom and Ashill, 2016). The COVID-19 pandemic forced a transition from traditional classroom learning to online learning, disrupting the “traditional learning environment” and giving rise to a new “virtual learning environment.” Within this rapidly changing learning scenario, educators and researchers have raised many concerns about students’ learning experience, such as: Are university students satisfied with their distance learning experience? How do they perceive their learning in a virtual environment? Is their academic satisfaction influenced by individual variables? How do instructors influence their students’ learning? What role is played by their relationship with the other students? And how important is the organization and design of the courses? This study aimed to contribute to answering these questions by exploring what variables influenced university students’ academic satisfaction during the COVID-19 emergency period.
1.1. Background
In higher education, there is increasing interest in academic satisfaction (AS), which is viewed by researchers and educators as a key variable to explain both academic success and the main problems of university students (Joo et al., 2011; Flores Kanter et al., 2017; Morelli et al., 2023a). AS may be defined as students’ positive judgment regarding their learning experience at university (Kuo et al., 2014). AS is thus considered a critical variable for understanding students’ academic experience: on the one hand, the literature shows that high academic satisfaction is associated with academic success, positive learning outcomes, commitment to continuing one’s studies, and lower levels of stress and dysfunctional behavior throughout one’s academic career (Eom et al., 2006; Tessema et al., 2012; Wang et al., 2013; Jaradat and Ajlouni, 2020) on the other hand, low academic satisfaction can result in a higher risk of drop-out, especially in distance education settings, where this phenomenon is already more common (Kuo et al., 2014; Biasi et al., 2018; Jaradat and Ajlouni, 2020; Morelli et al., 2021). Indeed, students who are more satisfied with their academic experience will be more likely to reinvest their resources in their study activities, while those with less AS will redirect their resources toward other activities that may be more satisfying for them (Kong and Yan, 2014). For all of these reasons, AS is viewed as crucial to students’ university careers. Existing research confirms the importance of studying AS and analyzing the factors that promote it, in order to ensure effective learning experiences, including in the growing field of online learning (Flores Kanter et al., 2017; Jaradat and Ajlouni, 2020).
To date, studies that have compared the academic satisfaction of students attending traditional university classroom settings with that of online university students have reported conflicting findings. While according to some studies, university students report a high level of satisfaction with online learning environments (Larson and Sung, 2009; Owston et al., 2013; Eom and Ashill, 2016; Al Awamleh, 2019), other studies have identified medium or low levels of academic satisfaction with this approach (Cole, 2016; Amir et al., 2020; Young and Bruce, 2020; Bawaneh, 2021). Eom and Ashill (2016) reported that, in their study, approximately 50% of students were highly satisfied with their online experience. Similarly, Al Awamleh (2019) found that students generally reported being satisfied (83%) with a blended program and online learning environments. Research conducted by Larson and Sung (2009), who compared students’ satisfaction with face-to-face (F2F), blended, and online learning formats, showed that mode of delivery did not affect student satisfaction, and that the students were satisfied with both the blended and online versions of the class. In addition, Owston et al. (2013) found that high achieving students were the most satisfied with blended courses, preferring a blended delivery mode over traditional F2F courses. On the other hand, Bawaneh (2021), in a study conducted during the COVID-19 pandemic, reported that the average level of students’ academic satisfaction with respect to distance learning was only medium. Young and Bruce (2020) conducted a study comparing students’ satisfaction depending on different delivery modes: F2F, synchronous online, asynchronous online, and hybrid. Their results showed that participants preferred F2F delivery over any of the three types of online delivery. Similarly, Cole (2016) also reported that students appeared more satisfied with F2F courses than online courses. In a study by Amir et al. (2020), 62% of students disagreed that distance learning gave similar levels of learning satisfaction compared to F2F learning, implying that the majority of students experienced lower levels of satisfaction when engaging in remote learning.
Students’ AS is based on a complex judgment that is influenced by several other variables besides class attendance (F2F vs. online) (Morelli et al., 2021). The literature identifies different antecedents related to students’ satisfaction with distance learning, including motivation (Eom et al., 2006; Wang et al., 2013), self-regulated learning (Wang et al., 2013; Eom and Ashill, 2016 Li and Yang, 2021), social presence and peer interaction (Cole et al., 2014; Eom and Ashill, 2016; Richardson et al., 2017; Jaradat and Ajlouni, 2020; Lane et al., 2021; Lim and Richardson, 2021), instructor-student interaction (Joo et al., 2011; Cole et al., 2014; Kuo et al., 2014; Cole, 2016; Eom and Ashill, 2016; Martin et al., 2018; Lane et al., 2021; Lim and Richardson, 2021), and course design (Bernard et al., 2009; Eom and Ashill, 2016; Lane et al., 2021).
Motivation is the process that initiates, guides, and maintains people’s goal-oriented behaviors (Ryan and Deci, 2000). Previous studies have shown that high levels of motivation are related to academic success and student satisfaction (Eom et al., 2006; Wang et al., 2013). On the contrary, a lack of motivation is related to a higher risk of drop-out (Biasi et al., 2018; Rabin et al., 2020; Morelli et al., 2023b). Although motivation is often viewed as a single construct, the literature distinguishes between intrinsic motivation and extrinsic motivation. Intrinsic motivation may be defined as engagement with a task due to the personal desire to challenge oneself, gain new experiences, acquire new knowledge, have fun, or follow one’s personal interests or values. On the other hand, extrinsic motivation may be defined as the regulation of one’s own behavior depending on rewards, punishments, or pressures from external context (Ryan and Deci, 2000). The different types of motivation are associated with different experiences and outcomes: in the online learning context, intrinsic motivation is associated with better learning outcomes (Eom and Ashill, 2016), whereas extrinsic motivation is considered a barrier to student satisfaction and is related to the risk of drop-out (Rabin et al., 2020). Lesserd and Puhl (2021) found that one of students’ most frequent concerns with respect to distance learning during the COVID-19 pandemic was that they might lose their motivation to participate in academic activities.
Another variable that can positively influence academic satisfaction and learning performance in online learning environments is students’ ability to self-regulate their learning (Wang et al., 2013; Rabin et al., 2019). Self-regulation is the process whereby learners transform their thoughts, feelings, and behaviors into useful strategies aimed at accomplishing their goals (Zimmerman, 2000). On the other hand, learners who are unable to regulate their learning process may experience dissatisfaction. In the context of learning, self-regulated learning (SRL) is defined as the ability of students to: (a) define their academic goals, (b) implement effective strategies to achieve their goals, (c) monitor their performance to understand if they are making progress, (d) adapt their physical and social context to suit their goals, (e) efficiently manage time, (f) self-assessing the methods they employ, (g) correctly attributing causes to specific outcomes and (h) adapting their strategies and methods to future challenges (Zimmerman, 2002). When students use SRL strategies they are more likely to function well academically, have positive learning outcomes, be more satisfied, and feel more motivated to continue to improve their learning methods (Zimmerman, 2000; Richardson et al., 2012). According to Wang et al. (2013), by using more effective learning strategies, students increase their levels of motivation to engage with online courses, which leads to higher levels of academic satisfaction and better performance. However, contrary to earlier research, a study by Eom and Ashill (2016) failed to establish a positive link between SRL and students’ satisfaction with distance learning.
In the context of distance education, one of the main differences compared to traditional education concerns social interaction. The lack of informal interaction with both instructors and classmates is one of the greatest barriers to online learning (Furlonger and Gencic, 2014; Amir et al., 2020; Rabin et al., 2020). In a study by Young and Bruce (2020), in which different delivery methods (F2F/online) were compared, students reported that the traditional F2F approach provides a stronger sense of social presence and classroom interaction, and that although online learning provides social interaction with classmates and instructors, it does not enable interpersonal connections that are as fulfilling as those afforded by face-to-face (F2F) learning settings. Furthermore, Lesserd and Puhl (2021) showed that during the COVID-19 pandemic, the majority of students engaged in less frequent communications and received less support from instructors. Nevertheless, the literature has shown that in both face-to-face and online learning environments, social interactions are a primary predictor of student satisfaction (Cole et al., 2014; Grieve et al., 2016; Richardson et al., 2017; Jaradat and Ajlouni, 2020; Lim and Richardson, 2021).
In the context of distance education, several studies have shown that student-instructor interaction is the strongest predictor of student satisfaction and learning outcomes, and one of the most important factors contributing to effective online teaching (Joo et al., 2011; Kuo et al., 2014; Cole, 2016; Eom and Ashill, 2016; Martin et al., 2018; Lim and Richardson, 2021). The instructor is considered a “facilitator” of student learning: the relationship with the instructor is crucial to improve student engagement and learning, especially when instructors are supportive, answering students’ questions, providing feedback, and encouraging students to become more engaged in their courses (Martin et al., 2018).
While the key role of positive interaction with instructors is thus well recognized, on the other hand, the literature reports conflicting outcomes concerning the impacts of student–student interaction in online learning settings. Moretti et al. (2018) emphasize the importance of collaborating with peers in the academic context: given that students are “developing subjects,” working with peers can positively influence academic outcomes and enhance relational skills, benefiting both students’ academic careers and their professional futures. Lane et al. (2021) showed that in blended learning, peer collaborative learning and group work are positively associated with student satisfaction. However, Zhang et al. (2020) distinguish between teaching presence (in terms of students’ perceptions of the quality of student-instructor interaction and individual feedback) and social presence (in terms of students’ perceptions of the quality of student–student interaction). Several studies have reported that social presence and social support have a positive effect on academic satisfaction (Cole et al., 2014; Grieve et al., 2016; Richardson et al., 2017; Jaradat and Ajlouni, 2020), and on student wellbeing (Cattelino et al., 2021). On the contrary, other studies have suggested that student–student interactions do not have a significant influence on student learning outcomes or satisfaction: both Kuo et al. (2014) and Joo et al. (2011) reported that in online courses, student–instructor interaction is a significant predictor of student satisfaction but student–student interaction is not.
Finally, with regard to organizational variables, several studies have pointed up the importance of course design and organization on students’ experience of distance learning. According to Moore (1991) and Zhang et al. (2020), course design refers to a clear explanation, before the course begins, of the learning’s educational objectives, course structure, teaching strategies, and evaluation methods. Eom and Ashill (2016) found that well-defined course design has a significant relationship with both student satisfaction and learning outcomes. Similarly, Lane et al. (2021) also demonstrated that the design and organization of online content play a key part in students’ satisfaction and engagement with the course. According to Han and Geng (2023), when students receive adequate instruction, clear goals, and helpful study materials, it enables them to study more effectively.
Therefore, as just outlined, the construct of academic satisfaction is a complex one and is influenced by numerous other variables. In an effort to develop a theoretical model that could systematically organize the relations among these variables, Lent (2004) proposed the Social Cognitive Model of Academic Satisfaction. According to this model, AS is directly affected by students’ perceptions of how they are progressing towards their goals, which in turn mediates the effects of self-efficacy, expected outcomes, and environmental support on AS (Flores Kanter et al., 2017). In essence, one of the innovative contributions of this model is its consideration of the active role of the individual student: in other words, students do not simply passively absorb external influences, but rather their subjective perceptions also contribute to their academic satisfaction (Zalazar-Jaime et al., 2021). Then, students’ perceived learning outcomes may act as a mediator between personal, social, and organizational variables and AS. Indeed, the literature offers evidence that students’ perceived learning is used as an indicator of the quality of online learning (Eom et al., 2006) and that it is affected by intrinsic motivation (Eom and Ashill, 2016), student-instructor interaction (Eom et al., 2006; Arbaugh, 2008; Martin et al., 2018; Lim and Richardson, 2021), social presence (Arbaugh, 2008; Richardson et al., 2017; Lim and Richardson, 2021; Wang et al., 2021), and well-defined course design (Eom and Ashill, 2016; Han and Geng, 2023).
Finally, as suggested by Lent (2004), the influences on AS need to be investigated from a multivariate perspective that takes into account the complex interaction of multiple factors. However, studies that have examined the drivers of AS using a multivariate approach are still scarce, especially in the Italian context. Given this complex scenario, and in light of the literature just reviewed, the aim of this study was to analyze the influence of selected individual (motivation and self-regulated learning), social (instructor-student and student–student interaction), and organizational (course organization and class attendance) variables on university students’ AS, as well as the mediating role of perceived learning, during a period marked by restrictions due to the COVID-19 pandemic. We designed our model (see Figure 1) to test the following hypotheses:
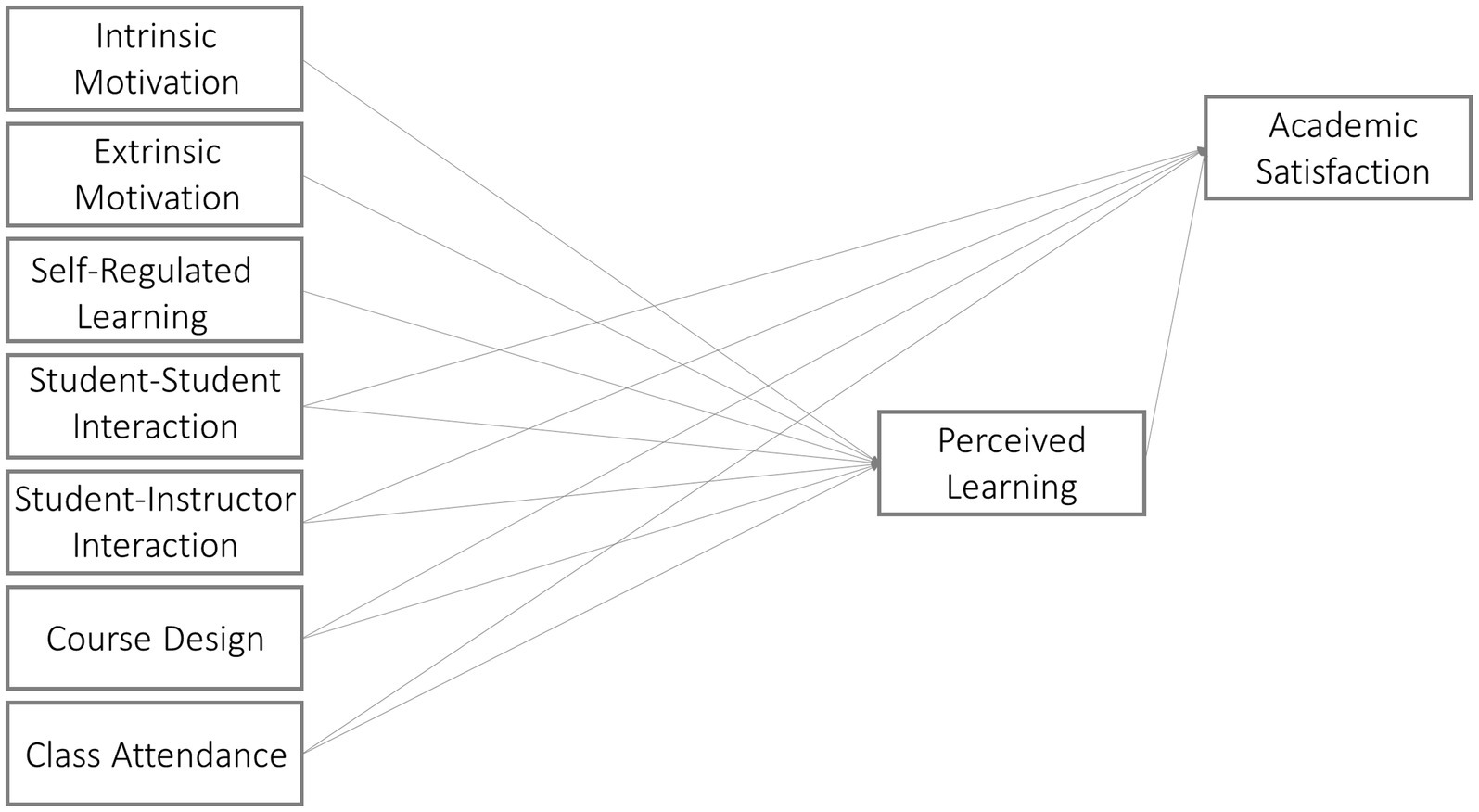
Figure 1. Theoretical model linking academic satisfaction with personal, social, and organizational variables via the mediation of perceived learning.
H1: Intrinsic motivation, extrinsic motivation, and self-regulated learning have an indirect effect on students’ academic satisfaction (AS) via the mediation of perceived learning.
H2: Student-student interaction and student-instructor interaction have a direct effect on AS.
H3: Student-student interaction and student-instructor interaction have an indirect effect on AS via the mediation of perceived learning.
H4: Course organization and class attendance (completely online/other modes) have a direct effect on AS.
H5: Course organization and class attendance (completely online/other modes) have an indirect effect on AS via the mediation of perceived learning.
2. Method
2.1. Participants
A total of 104 university students from at a Northern Italian University took part in the study, of whom 87 (83.7%) were female. Students had a mean age of 26.6 (SD = 9.8) years; 46.2% were enrolled in the degree course in Psychology (bachelor’s degree), 12.5% in the degree course in Education Sciences for Primary School (master’s degree), 11.5% in the course in Political Science (bachelor’s degree), 11.5% in the Economics degree course (bachelor’s degree), 8.7% in the Economics degree course (master’s degree), and 9.6% in the Languages and Communication course (bachelor’s degree). The research team contacted the students by email, providing information about the study, an informed consent form, and a link to the online questionnaire. All participants voluntarily signed the informed consent form, authorizing us to use the data collected for the purposes of the study. The study was approved by the ethic committee of the Department of Human and Social Sciences, University of Aosta Valley, Italy (2021-UNVACLE-0014654).
2.2. Procedure and measures
Participants were asked to complete an online questionnaire in which they were asked to respond to the questions by choosing a single course they had taken during the second semester of the 2020–21 academic year, for which they had attended at least 50% of the classes offered. The questionnaire comprised a set of scales selected to measure the different variables under study. Our examination of the factorial structure of the scales is reported in the Appendix.
2.2.1. Motivation
We measured intrinsic and extrinsic motivation using the two subscales of the Motivated Strategies for Learning Questionnaire (MSLQ, Pintrich et al., 1991), each comprising four items. The eight items were first independently translated by two members of the research team who are fluent in English. A consensus version was then agreed upon and back-translated by a native English speaker. Examples of items are: “In a class like this, I prefer course material that arouses my curiosity, even if it is difficult to learn”; “Getting a good grade in this class is the most satisfying thing for me right now.” Participants were asked to rate the extent to which each statement was true for them on a Likert scale ranging from 1 (not at all) to 7 (very much). Based on a bidimensional exploratory structural model (see Appendix), two items from the intrinsic motivation scale were excluded. The Cronbach’s alpha coefficients for the scales were 0.64 (intrinsic) and 0.71 (extrinsic).
2.2.2. Self-regulated learning
We measured self-regulated learning using the 12-item subscale of the Italian adaptation of the MSLQ (Moretti et al., 2018). A sample item is: “I ask myself questions to make sure I understand the material I have been studying in this class.” Again the items were rated on a Likert scale ranging from 1 (not at all true) to 7 (very much true) and Cronbach’s alpha coefficient was 0.77.
2.2.3. Student–student and student-instructor interactions
We assessed the participants’ interactions among students using the relative 7-item subscale of the Italian adaptation of the MSLQ (Moretti et al., 2018). A sample item is: “When I can’t understand the material in this course, I ask another student in this class for help.” To examine the interactions between students and instructors, we adopted the 6-item Good Teaching subscale of the Italian version of the Student Course Experience Questionnaire (Barattucci and Zuffo, 2012). A sample item is: “My instructor made a real effort to understand the difficulties I might be having with my work.” The items concerning student–student relations were rated on a 7-point scale ranging from 1 (not at all true) to 7 (very much true); the items concerning student-instructor relations were rated on a 5-point scale ranging from 1 (strongly disagree) to 5 (strongly agree). Cronbach’s alpha coefficients were 0.82 and 0.92, respectively.
2.2.4. Organizational aspects
The four items used to assess course organization were drawn from Bassi et al. (2017). A sample item is: “At the beginning of the course, aims and topics were clearly outlined.” Participants rated the items on a 5-point Likert scale ranging from 1 (strongly disagree) to 5 (strongly agree). Cronbach’s alpha coefficient was 0.78.
Class attendance was treated as a dummy variable, with a value of 1 assigned to “completely online attendance” and a value of 0 assigned to “other modes of attendance.”
2.2.5. Outcome variables
The items measuring the outcome variables have been developed specifically for the study. We assessed perceived learning using the following item: “In your opinion, to what extent did you successfully learn the contents presented during this course?” on a scale ranging from 1 (not at all) to 5 (to a great extent). AS was measured using: four items concerning satisfaction with course content, relationships with classmates, relationships with instructors, and the overall course experience, to be rated on a scale ranging from 1 (completely unsatisfied) to 10 (completely satisfied); two further items assessed the respondent’s willingness to recommend the course and its instructor to other students (on a 4-point rating scale ranging from 1 (definitely not) to 4 (definitely yes), then converted to the range 1–10 for the purposes of the statistical analyses). Confirmatory Factor Analysis (CFA) produced a non-statistically significant loading for the item that assessed satisfaction regarding the relationships with classmates, which was therefore removed from the subsequent analyses. For the final set of five items, Cronbach’s alpha coefficient was 0.92.
2.3. Data analysis
We tested our research hypotheses using a structural equations model for observed variables (path analysis). This analysis was performed after controlling for the factorial structure of each scale, as detailed in the Appendix. There were no missing data for any of the study variables.
Path analysis coefficients were estimated by means of a robust maximum likelihood estimator (MLMV). For the estimation of the indirect effects, the bootstrap procedure (Hayes, 2018) with 1,000 samples was used. We assessed the model’s goodness of fit based on the following fit indexes: root-mean-square error of approximation (RMSEA), standardized root-mean-square residual (SRMR), and comparative fit index (CFI) (Schermelleh-Engel et al., 2003). Values greater than 0.95 for CFI, and under 0.05 for RMSEA and SRMR are taken to indicate excellent fit (Hu and Bentler, 1999; Schermelleh-Engel et al., 2003). We relied especially upon SRMR and CFI, because the literature cautions against the use of RMSEA in case of models with few degrees of freedom and a small sample size, as in the present study. Under such conditions, RMSEA often incorrectly indicates poor fit (Kenny et al., 2015).
We used Spss software (version 27, IBM SPSS Statistics, IBM Corporation) to perform the descriptive analyses and totally the scores for each of the scales; we drew on Mplus (version 8, Muthén and Muthén, 1998–2017) to conduct the factor analyses and path analysis.
3. Results
3.1. Descriptive statistics
The descriptive statistics and bivariate relationships between the variables included in the path analysis are reported in Table 1. Scores for motivation, self-regulated learning, interaction with the instructor, interaction with other students, course organization, and AS were computed as the mean of the scores on the pertinent subscales.
As shown in the table, around 69% of the participants chose to evaluate a course that they attended completely online (class attendance). Students’ perceptions of the extent to which they had succeeded in learning the contents of the course were generally positive (M = 4.07, with respect to a range from 1 to 5) and their level of overall satisfaction with the course was high (M = 8.25, with respect to a range from 1 to 10).
As shown in Table 1, the two types of motivation displayed different patterns of correlations: Intrinsic motivation was weakly correlated with self-regulated learning and interaction with other students, while extrinsic motivation was weakly correlated with interaction with instructor, perceived learning, and AS. Self-regulated learning was correlated with intrinsic motivation, interaction with other students, course organization, and the two outcome variables of perceived learning and academic satisfaction. Interaction with other students was only correlated with intrinsic motivation and self-regulated learning. Interaction with the instructor was very strongly correlated with academic satisfaction and course organization, and strongly correlated with perceived learning; the relationship with extrinsic motivation was low. Course organization on the other hand was strongly correlated with the two outcome variables and interaction with the instructor, and weakly correlated with self-regulated learning. Class attendance was not correlated with any of the other variables. The two outcome variables, perceived learning and academic satisfaction, were highly correlated with one another and displayed extremely similar patterns of relationships with the other study variables: more specifically, moderate to high correlations with the individual variables of extrinsic motivation and self-regulated learning, as well as with interaction with the instructor and course organization.
3.2. Path model
The theoretical model, when tested using a structural equation model for observed variables, was found to offer an acceptable fit for the data (χ2(3) = 7.569, p = 0.0558; RMSEA = 0.121; CFI = 0.974; SRMR 0.012). The variance explained by the model was high (R2 = 0.454 for perceived learning and R2 = 0.857 for AS). The standardized path coefficients are reported in Figure 2 and described in the following.
H1: Intrinsic motivation, extrinsic motivation, and self-regulated learning have an indirect effect on students’ academic satisfaction (AS) via the mediation of perceived learning.
As shown in Figure 2, the standardized coefficients relating intrinsic and extrinsic motivation to perceived learning did not attain statistical significance (β = 0.028, p > 0.05 and β = 0.097, p > 0.05, respectively). The standardized coefficient for self-regulated learning was statistically significant (β = 0.243, p < 0.01). Hence, we only tested the indirect influence of self-regulated learning on AS, obtaining a statistically significant coefficient of low magnitude (standardized estimate = 0.055; bootstrap 95% CI = 0.012–0.110). H1 was thus partially supported.
H2: Student-student interaction and student-instructor interaction have a direct effect on AS.
The standardized coefficient for student–student interaction did not attain significance (β = −0.068, p > 0.05). The standardized coefficient for student-instructor interaction was high and statistically significant (β = 0.613, p < 0.001). H2 was therefore partially supported.
H3: Student-student interaction and student-instructor interaction have an indirect effect on AS via the mediation of perceived learning.
The indirect effects of student–student interaction and student-instructor interaction on AS were not tested, because their relationship with the mediator variable, perceived learning, was not statistically significant (β = 0.013, p > 0.05 for student–student interaction and β = 0.118, p > 0.05 for student-instructor interaction). Therefore, H3 was not supported.
H4: Course organization and class attendance (completely online/other modes) have a direct effect on AS.
The standardized coefficient for course organization was statistically significant (β = 0.221, p < 0.005). The standardized coefficient for the mode of class attendance did not attain statistical significance (β = 0.004, p > 0.05). Hence, H4 was partially supported.
H5: Course organization and class attendance (completely online/other modes) have an indirect effect on AS via the mediation of perceived learning.
The relationship between course organization and perceived learning was statistically significant (β = 0.453, p < 0.001) but the relationship between class attendance and perceived learning was not (β = −0.136, p > 0.05). Therefore, we only tested the indirect effect of course organization on AS, obtaining a statistically significant coefficient of low magnitude (standardized value of 0.103, bootstrap 95% CI =0.041–0.168). H5 was therefore partially supported.
As a control analysis, the model was re-estimated with the addition of biological sex and age as predictors of perceived learning and AS. The outcomes were very similar to those reported above, in terms of both the global fit indices (χ2(3) = 7.511, p = 0.0573; RMSEA = 0.120; CFI = 0.976; SRMR = 0.009) and the path coefficients (not reported).
4. Discussion and conclusions
The aim of the current study was to analyze the influence of selected individual (motivation and self-regulated learning), social (instructor-student and student–student interaction), and organizational (course design and class attendance) variables on university students’ AS, as well as the mediating role of perceived learning, during a period marked by restrictions due to the COVID-19 pandemic.
The outcomes are in line with those of other studies that have shown academic satisfaction (AS) to be an indicator of quality in higher education (Flores Kanter et al., 2017; Jaradat and Ajlouni, 2020; Koka et al., 2023; Morelli et al., 2023a), while also offering some novel insights. Academic satisfaction, as proposed by Lent (2004), is a construct influenced by many factors, both internal and external to the individual, and this study further contributes to the literature on this topic by examining the effects of a set of personal, social, and organizational variables on AS, and specifically in the context of a particular emergency; during the 2020-2021 academic year, most classes were delivered online, on the exceptional grounds of the pandemic, but they had been designed for face-to face delivery and not for distance education. Hence, we set out to explore what factors contributed to the students’ satisfaction with their academic learning experience under these extraordinary circumstances.
We found that, during the pandemic, student-instructor interaction, perceived learning, and course organization were particularly strongly linked with AS. Furthermore, self-regulated learning and course organization had an indirect influence on students’ academic satisfaction via the mediaton of perceived learning (as shown in Figure 2). These findings underscore the importance of simultaneously analyzing individual, social, and organizational variables when investigating AS.
More specifically, in relation to the individual variables, only self-regulated learning affected perceived learning, whereas both intrinsic and extrinsic motivation were not significant predictors. The key role of self-regulation in the learning process is well known (Zimmerman, 2002; Robbins et al., 2004; Rabin et al., 2019): attending university is an important developmental task for young adults, and the COVID-19 pandemic, with the consequent abrupt shift from face-to-face to online teaching, made this experience even more challenging. It is precisely during challenges and in the face of novelty that self-regulation plays a central role; it may thus have overridden the effects of other variables, such as motivation, which appear to be significant in research carried out in non-emergency periods (Morelli et al., 2023b). Perceived learning in its turn was found to promotes higher AS, as proposed by Zalazar-Jaime et al. (2021).
With regards to social variables, the findings of the present study confirmed the positive effects of student-instructor interaction on academic satisfaction in the online environment (Cole et al., 2014; Cole, 2016; Miao et al., 2022): during the COVID-19 pandemic, the presence of instructors in online or blended environments who were perceived to be more supportive, responsive, ready to provide feedback, and encouraging was strongly associated with greaer students’ academic satisfaction. On the contrary, we found no relationship between student–student interaction and AS. This finding is consistent with some previous studies that also reported no significant effect of student–student interaction on student’s academic satisfaction (Joo et al., 2011; Kuo et al., 2014). However, there is a lack of consensus in the literature concerning the role of this predictor of AS in distance learning settings; the reasons why relationships among students are associated with greater satisfaction (Morelli et al., 2023a) and lower intention to drop-out (Cattelino et al., 2021; Morelli et al., 2023b) in face-to-face teaching environments, but possibly not in online or blended settings, remains to be further explored.
Finally, as far as organizational variables are concerned, course organization is closely related to both academic satisfaction and perceived learning. This result is in line with previous studies that found course structure to have a significant bearing on students’ satisfaction and learning outcomes (Eom et al., 2006; Eom and Ashill, 2016; Lane et al., 2021). More than ever during the COVID-19 pandemic, students experienced uncertainty about their academic career. In this unexpected situation, which risked compromising the learning process of many young people, clearly explained learning and educational objectives, course structure, teaching, and evaluation strategies helped students to be more satisfied with their experience and more confident in their learning outcomes. Good course organization likely offers certainty about content, timing, and evaluation and this allows students to better monitor their learning goals, thereby enhancing their perceived learning. Similarly, clear feedback on how their learning is progressing is reassuring to students and therefore, also affects their satisfaction with their academic experience.
Overall, our findings highlight the pivotal role of student-instructor interaction, course organization, and perceived learning in directly promoting the AS, and the role of self-regulated learning and course organization in indirectly promoting the AS via the mediation of the perceived learning. In sum, high self-regulation in learning and good relationships with instructors during the pandemic period, backed up by good course organization, all enhanced students’ academic satisfaction, which is one of the best indicators of educational success.
Furthermore, students’ relationship with the instructor and course organization (which is, in any case, a variable linked with the capability of the instructor), played a greater part in determining a positive academic experience than the class delivery (online/F2F). This confirms the decisive role of the instructor, who is not a mere transmitter of knowledge, but rather an active and crucial party in the student’s learning process.
Another particularly interesting finding of this study is the fact that perceived learning mediates between multiple personal and organizational variables and academic satisfaction. This outcome represents an additional contribution to the literature on AS, as it confirms for online learning what Lent (2004) had already understood for face-to-face learning, namely that AS is not only influenced by external factors, but also by personal perceptions. Individuals are not mere assimilators of external stimuli, but rather their subjective perceptions play an active role in shaping their satisfaction with their academic experience by mediating the impact of the outside factors at play. Hence, perceived learning is key to optimizing academic satisfaction in online learning environments also.
Therefore, alongside social interaction with instructors, which is a leading predictor of student satisfaction (Cole et al., 2014; Grieve et al., 2016; Richardson et al., 2017; Jaradat and Ajlouni, 2020; Lim and Richardson, 2021), perceived learning and course organization also contributed heavily to enhancing academic satisfaction during the pandemic period.
New lines of inquiry may usefully focus on the design of different kinds of online or blended learning environments, based for example on problem-based learning, project-based learning, or knowledge building (e.g., Saputra et al., 2019; Tan et al., 2021; Cacciamani et al., 2023), and intended to foster interaction among students and between students and instructors – with a view to assessing how such learning trajectories may best be organized to promote student success and academic satisfaction.
This study presents some limitations. First of all, our sample was small and drawn from only one Italian university, meaning that it was not representative of the entire student population. In addition, the study was conducted at a university in Northern Italy, an area where the first outbreaks of COVID-19 were registered and where the risk of contagion was higher than in other regions of the country. This situation and the policies that it engendered may constitute a unique context that requires us to be cautious in generalizing the results. Furthermore, perceived learning was measured using a single item; future studies might usefully draw on more robust operationalizations of this construct. Finally, the cross-sectional nature of the study imposes still further caution in generalizing the results.
Despite these limitations, our findings provide some important implications and recommendations for online instructors and instructional designers, which can be also relevant to non-emergency situations. First, student-instructor interaction is essential to the successful learning experience and students’ academic satisfaction, and for this reason, it is very important to implement strategies that could encourage interaction during online or blended courses also; the online environment should be configured as a meeting tool where the students can work collaboratively and engage in effective interaction with istructors about course topics, and not simply as a platform for depositing and exchanging materials. To this end, it is crucial to support instructors, who often only use strategies designed for face-to-face teaching, by providing them with targeted professional development opportunities.
A second key aspect to address in order to develop a more positive academic satisfaction is perceived learning, which is promoted by self-regulation and organizational variables. To this end, it might be of value to provide students with tools for self-assessing their learning progress, such as exercises and self-assessment questions on course content. Furthermore, it is really important that information about how the course is organized should be accessible, clear, and communicated to the students in a timely manner: in fact, these aspects concerns the organizational and regulatory context in which the learning process takes place and makes a key contribution to students’ satisfaction with their academic experience. Specifically, effective course organization facilitates anticipation and control, in that students know in advance what the course contents and timeframe are going to be and are therefore better positioned to self-regulate their learning activity. All these aspects proved to be particularly crucial in the context of online courses delivered during the pandemic period, but they are equally fundamental in non-emergency contexts and in face-to-face learning settings.
Data availability statement
The raw data supporting the conclusions of this article will be made available by the authors, without undue reservation.
Ethics statement
The study was approved by the ethic committee of the Department of Human and Social Sciences, University of Aosta Valley, Italy (2021-UNVACLE-0014654). The participants provided their informed consent to participate in this study.
Author contributions
ST: Conceptualization, Data curation, Formal analysis, Funding acquisition, Investigation, Methodology, Project administration, Supervision, Writing – original draft, Writing – review & editing. AM: Data curation, Writing – original draft, Writing – review & editing. FB: Writing – review & editing. SC: Conceptualization, Formal analysis, Methodology, Writing – original draft, Writing – review & editing. TG: Writing – review & editing. VP: Writing – review & editing. AP: Funding acquisition, Project administration, Writing – review & editing. GT: Writing – review & editing. EC: Conceptualization, Data curation, Funding acquisition, Investigation, Methodology, Supervision, Writing – original draft, Writing – review & editing.
Funding
The author(s) declare financial support was received for the research, authorship, and/or publication of this article. This study was funded by a local grant from the University of Aosta Valley (TES20PRA.SHS).
Conflict of interest
The authors declare that the research was conducted in the absence of any commercial or financial relationships that could be construed as a potential conflict of interest.
The author(s) declared that they were an editorial board member of Frontiers, at the time of submission. This had no impact on the peer review process and the final decision.
Publisher’s note
All claims expressed in this article are solely those of the authors and do not necessarily represent those of their affiliated organizations, or those of the publisher, the editors and the reviewers. Any product that may be evaluated in this article, or claim that may be made by its manufacturer, is not guaranteed or endorsed by the publisher.
Supplementary material
The Supplementary material for this article can be found online at: https://www.frontiersin.org/articles/10.3389/feduc.2023.1268585/full#supplementary-material
References
Al Awamleh, A. (2019). Students satisfaction on blended learning in School of Sport Sciences. Ann. Appl. Sport Sci. 8:e803. doi: 10.29252/aassjournal.803
Amir, L. R., Tanti, I., Maharani, D. A., Wimardhani, Y. S., Julia, V., Sulijaya, B., et al. (2020). Student perspective of classroom and distance learning during COVID-19 pandemic in the undergraduate dental study program Universitas Indonesia. BMC Med. Educ. 20:392. doi: 10.1186/s12909-020-02312-0
Arbaugh, J. B. (2008). Does the community of inquiry framework predict outcomes in online MBA courses? Int. Rev. Res. Open Dist. Learn. 9, 1–21. doi: 10.19173/irrodl.v9i2.490
Barattucci, M., and Zuffo, R. G. (2012). Measuring learning environment perceptions: validation of the Italian version of the approaches to studying inventory and the student course experience questionnaire. TPM - Test. Psychom. Methodol. Appl. Psychol. 19:1. doi: 10.4473/TPM19.1.2
Bassi, F., Clerci, R., and Aquario, D. (2017). Students’ evaluation of teaching at a large Italian university: measurement scale validation. Electron. J. Appl. Stat. Anal. 10, 93–117. doi: 10.1285/i20705948v10n1p93
Bawaneh, A. K. (2021). The satisfaction level of undergraduate science students towards using e-learning and virtual classes in exceptional condition covid-19 crisis. Turk. Online J. Distance Educ. 22, 52–65. doi: 10.17718/tojde.849882
Bernard, R. M., Abrami, P. C., Borokhovski, E., Wade, C. A., Tamim, R. M., Surkes, M. A., et al. (2009). A metaanalysis of three types of interaction treatments in distance education. Rev. Educ. Res. 79, 1243–1289. doi: 10.3102/0034654309333844
Biasi, V., De Vincenzo, C., and Patrizi, N. (2018). Strategie cognitive per l’autoregolazione dell’apprendimento e motivazione allo studio. ECPS J. 17, 139–159. doi: 10.7358/ecps-2018-017-bias
Cacciamani, S., Perrucci, V., Khanlari, A., and Balboni, G. (2023). Sense of community and peer feedback in a blended university course. Educ. Inf. Technol., 1–13. doi: 10.1007/s10639-023-11982-4
Cattelino, E., Chirumbolo, A., Baiocco, R., Calandri, E., and Morelli, M. (2021). School achievement and depressive symptoms in adolescence: the role of self-efficacy and peer relationships at school. Child Psychiatry Hum. Dev. 52, 571–578. doi: 10.1007/s10578-020-01043-z
Cole, A. W. (2016). Testing the impact of student preference for face-to-face communication on online course satisfaction. West. J. Commun. 80, 619–637. doi: 10.1080/10570314.2016.1186824
Cole, M. T., Shelley, D. J., and Swartz, L. B. (2014). Online instruction, E-learning, and student satisfaction: A three-year study. Int. Rev. Res. Open Dist. Learn. 15, 111–131. doi: 10.19173/irrodl.v15i6.1748
Eom, S., and Ashill, N. (2016). The determinants of students’ perceived learning outcomes and satisfaction in university online education: an update. Decis. Sci. J. Innov. Educ. 14, 185–215. doi: 10.1111/dsji.12097
Eom, S. B., Ashill, N., and Wen, H. J. (2006). The determinants of students’ perceived learning outcome and satisfaction in university online education: an empirical investigation. Decis. Sci. J. Innov. Educ. 4, 215–235. doi: 10.1111/j.1540-4609.2006.00114.x
Flores Kanter, P. E., Losano, C., Moretti, L. S., and Medrano, L. A. (2017). Empirical evidence for a socio-cognitive model of academic satisfaction: a review and meta-analysis approach. Psychol. Educ. 54, 1–25.
Furlonger, B., and Gencic, E. (2014). Comparing satisfaction, life-stress, coping and academic performance of counseling students in on-campus and distance education learning environments. Aust. J. Guid. Couns. 24, 76–89. doi: 10.1017/jgc.2014.2
Grieve, R., Padgett, C. R., and Mofitt, R. L. (2016). Assignments 2.0: the role of social presence and computer attitudes in student preferences for online versus offline marking. Internet High. Educ. 28, 8–16. doi: 10.1016/j.iheduc.2015.08.002
Han, J., and Geng, X. (2023). University students’ approaches to online learning technologies: the roles of perceived support, affect/emotion and self-efficacy in technology-enhanced learning. Comput. Educ. 194:104695. doi: 10.1016/j.compedu.2022.104695
Hayes, A. F. (2018). Introduction to mediation, moderation, and conditional process analysis: A regression-based approach. New York, NY: Guilford publications.
Hu, L. T., and Bentler, P. M. (1999). Cutoff criteria for fit indexes in covariance structure analysis: Conven-tional criteria versus new alternatives. Struct. Equ. Model. 6, 1–55. doi: 10.1080/10705519909540118
IAU (2020). COVID-19: Higher education challenges and responses International Association of Universities Available at: https://www.iau-aiu.net/COVID-19-Higher-Education-challenges-and-responses.
Jaradat, S. A., and Ajlouni, A. O. (2020). Social presence and self-efficacy in relation to student satisfaction in online learning setting: a predictive study. Int. J. Educ. Pract. 8, 759–773. doi: 10.18488/journal.61.2020.84.759.773
Joo, Y. J., Lim, K. Y., and Kim, E. K. (2011). Online university students’ satisfaction and persistence: examining perceived level of presence, usefulness and ease of use as predictors in a structural model. Comput. Educ. 57, 1654–1664. doi: 10.1016/j.compedu.2011.02.008
Kenny, D. A., Kaniskan, B., and McCoach, D. B. (2015). The performance of RMSEA in models with small degrees of freedom. Sociol. Methods Res. 44, 486–507. doi: 10.1177/0049124114543236
Keržič, D., Alex, J. K., Pamela Balbontín Alvarado, R., Da Silva Bezerra, D. D. S., Cheraghi, M., Dobrowolska, B., et al. (2021). Academic student satisfaction and perceived performance in the e-learning environment during the COVID-19 pandemic: evidence across ten countries. PLoS One 16:e0258807. doi: 10.1371/journal.pone.0258807
Koka, F., Kılıç, S., and Dadandı, İ. (2023). Attitudes towards distance education and academic life satisfaction: the mediation role of academic self-efficacy and moderator role of gender. Technol. Knowl. Learn. 23, 1–22. doi: 10.1007/s10758-023-09645-x
Kong, H., and Yan, Q. (2014). The relationship between learning satisfaction and career competencies. Int. J. Hosp. Manag. 41, 133–139. doi: 10.1016/j.ijhm.2014.05.013
Kuo, Y. C., Walker, A. E., Schroder, K. E. E., and Belland, B. R. (2014). Interaction, internet self-efficacy, and self-regulated learning as predictors of student satisfaction in online education courses. Internet High. Educ. 20, 35–50. doi: 10.1016/j.iheduc.2013.10.001
Lane, S., Hoang, J. G., Leighton, J., and Rissanen, A. (2021). Engagement and satisfaction: mixed-method analysis of blended learning in the sciences can. J. Sci. Math. Technol. Educ. 21, 100–122. doi: 10.1007/s42330-021-00139-5
Larson, D. K., and Sung, C. H. (2009). Comparing student performance: online versus blended versus face-to-face. JALN 13, 31–42. doi: 10.24059/olj.v13i1.1675
Lent, R. (2004). Toward a unifying theoretical and practical perspective on well-being and psychosocial adjustment. J. Couns. Psychol. 51, 482–509. doi: 10.1037/0022-0167.51.4.482
Lesserd, L. M., and Puhl, R. M. (2021). Adolescent academic worries amid COVID-19 and perspectives on pandemic-related changes in teacher and peer relations. School Psychol. 36, 285–292. doi: 10.1037/spq0000443
Li, L., and Yang, S. (2021). Exploring the influence of teacher-student interaction on university students’ self-efficacy in the flipped classroom. J. Educ. Learn. 10, 84–90. doi: 10.5539/jel.v10n2p84
Lim, J., and Richardson, J. (2021). Predictive effects of undergraduate students’ perceptions of social, cognitive, and teaching presence on affective learning outcomes according to disciplines. Comput. Educ. 161:104063. doi: 10.1016/j.compedu.2020.104063
Martin, F., Wang, C., and Ma, L. (2018). Teacher–student interaction, student–student interaction and social presence: their impacts on learning engagement in online learning environments. Internet High. Educ. 37, 52–65. doi: 10.1016/j.iheduc.2018.01.003
Miao, J., Chang, J., and Sadaf, A. (2022). Student perception of helpfulness of facilitation strategies that enhance instructor presence, connectedness, engagement and learning in online courses. J. Genet. Psychol. 6, 514–526.
Moore, M. G. (1991). Editorial: distance education theory. Am. J. Dist. Educ. 5, 1–6. doi: 10.1080/08923649109526758
Morelli, M., Baiocco, R., Cacciamani, S., Chirumbolo, A., Perrucci, V., and Cattelino, E. (2023a). Self-efficacy, motivation and academic satisfaction: the moderating role of friendship at the university. Psicothema 35, 238–247. doi: 10.7334/psicothema2022.254
Morelli, M., Chirumbolo, A., Baiocco, R., and Cattelino, E. (2021). Academic failure: individual, organizational, and social factors. Psicol. Educ. 27, 167–175. doi: 10.5093/psed2021a8
Morelli, M., Chirumbolo, A., Baiocco, R., and Cattelino, E. (2023b). Self-regulated learning self-efficacy, motivation, and intention to drop-out: the moderating role of friendships at university. Curr. Psychol. 42, 15589–15599. doi: 10.1007/s12144-022-02834-4
Moretti, G., Giuliani, A., and Morini, A. (2018). Accrescere la consapevolezza degli studenti come soggetti in formazione: utilizzo e validazione del Motivated Strategies for Learning Questionnaire in Italia. Italian J. Educ. Res. 21, 115–132.
Owston, R., York, D., and Murtha, S. (2013). Student perceptions and achievement in a university blended learning strategic initiative. Internet High. Educ. 18, 38–46. doi: 10.1016/j.iheduc.2012.12.003
Pintrich, P. R., Smith, D. A. F., Garcia, T., and McKeachie, W. J. (1991). A manual for the use of the motivated strategies for learning questionnaire (MSLQ). Ann Arbor, MI: National Center for Research to Improve Postsecondary Teaching and Learning.
Rabin, E., Kalman, Y. M., and Kalz, M. (2019). Predicting learner-centered MOOC outcomes: satisfaction and intention-fulfillment. Int. J. Educ. Technol. High. Educ. 16:14. doi: 10.1186/s41239-019-0144-3
Rabin, E., Kalman, Y. M., and Kalz, M. (2020). What are the barriers to learners’ satisfaction in MOOCs and what predicts them? The role of age, intention, self-regulation, self-efficacy and motivation. Australas. J. Educ. Technol. 36, 119–131. doi: 10.14742/ajet.5919
Raykov, T., and Marcoulides, G. A. (2016). Scale reliability evaluation under multiple assumption violations. Struct. Equ. Model. Multidiscip. J. 23, 302–313. doi: 10.1080/10705511.2014.938597
Richardson, M., Abraham, C., and Bond, R. (2012). Psychological correlates of university students' academic performance: a systematic review and meta-analysis. Psychol. Bull. 138, 353–387. doi: 10.1037/a0026838
Richardson, J. C., Maeda, Y., Lv, J., and Caskurlu, S. (2017). Social presence in relation to students’ satisfaction and learning in the online environment: A meta-analysis. Comput. Hum. Behav. 71, 402–417. doi: 10.1016/j.chb.2017.02.001
Robbins, S. B., Lauver, K., Le, H., Davis, D., Langley, R., and Carlstrom, A. (2004). Do psychosocial and study skill factors predict college outcomes? A meta-analysis. Psychol. Bull. 130, 261–288. doi: 10.1037/0033-2909.130.2.261
Ryan, R., and Deci, E. (2000). Self-determination theory and the facilitation of intrinsic motivation, social development, and well-being. Am. Psychol. 55, 68–78. doi: 10.1037/0003-066X.55.1.68
Saputra, M. D., Joyoatmojo, S., Wardani, D. K., and Sangka, K. B. (2019). Developing critical-thinking skills through the collaboration of jigsaw model with problem-based learning model. Int. J. Instr. 12, 1077–1094. doi: 10.29333/iji.2019.12169a
Schermelleh-Engel, K., Moosbrugger, H., and Müller, H. (2003). Evaluating the fit of structural equation models: tests of significance and descriptive goodness-of-fit measures. Methods Psychol. Res. Online 8, 23–74.
Tan, S. C., Chan, C., Bielaczyc, K., Ma, L., Scardamalia, M., and Bereiter, C. (2021). Knowledge building: aligning education with needs for knowledge creation in the digital age. Educ. Technol. Res. Dev. 69, 2243–2266. doi: 10.1007/s11423-020-09914-x
Tessema, M. T., Ready, K., and Yu, W. C. (2012). Factors affecting college students’ satisfaction with major curriculum: evidence from nine years of data. Int. J. Humanit. Soc. Sci. 2, 34–44.
Wang, R., Han, J., Liu, C., and Xu, H. (2021). How do University students’ perceptions of the Instructor’s role influence their learning outcomes and satisfaction in cloud-based virtual classrooms during the COVID-19 pandemic? Front. Psychol. 12:e627443. doi: 10.3389/fpsyg.2021.627443
Wang, C.-H., Shannon, D. M., and Ross, M. E. (2013). Students’ characteristics, self-regulated learning, technology self-efficacy, and course outcomes in online learning. Distance Educ. 34, 302–323. doi: 10.1080/01587919.2013.835779
Young, S., and Bruce, M. A. (2020). Student and faculty satisfaction: can distance course delivery measure up to face-to-face courses? Educ. Res. Theory Pract. 31, 36–48.
Zalazar-Jaime, M. F., Moretti, L. S., Garcìa-Batista, Z. E., and Medrano, L. A. (2021). Evaluation of an academic satisfaction model in E-learning education context. Interact. Learn. Environ. 31, 1–11. doi: 10.1080/10494820.2021.1979047
Zhang, J., Addae, H., Bakeman, M., Boyraz, M., Flaherty, P. T., Habich, M., et al. (2020). Management students’ perceptions of online teaching quality. E-J. Bus. Educ. Scholar. Teach. 14, 33–52.
Zimmerman, B. J. (2000). “Attainment of self-regulation: A social cognitive perspective” in Handbook of self-regulation. eds. M. Boekaerts, P. R. Pintrich, and M. Zeidner (San Diego, CA: Academic Press), 13–39.
Keywords: academic satisfaction, perceived learning, distance learning, self-regulated learning, course organization, student-instructor interaction, COVID-19
Citation: Testa S, Macagno A, Bertolino F, Cacciamani S, Grange T, Perrucci V, Piu A, Timpano G and Cattelino E (2023) Academic satisfaction among university students during the COVID-19 pandemic: the influence of individual, social, and organizational variables. Front. Educ. 8:1268585. doi: 10.3389/feduc.2023.1268585
Edited by:
Caterina Fiorilli, Libera Università Maria SS. Assunta University, ItalyReviewed by:
Luciano Romano, European University of Rome, ItalyFrancesco Sulla, University of Foggia, Italy
Copyright © 2023 Testa, Macagno, Bertolino, Cacciamani, Grange, Perrucci, Piu, Timpano and Cattelino. This is an open-access article distributed under the terms of the Creative Commons Attribution License (CC BY). The use, distribution or reproduction in other forums is permitted, provided the original author(s) and the copyright owner(s) are credited and that the original publication in this journal is cited, in accordance with accepted academic practice. No use, distribution or reproduction is permitted which does not comply with these terms.
*Correspondence: Stefano Cacciamani, cy5jYWNjaWFtYW5pQHVuaXZkYS5pdA==