- 1Institute of Child Development, University of Minnesota, Minneapolis, MN, United States
- 2Minnesota Population Center, University of Minnesota, Minneapolis, MN, United States
Introduction: We examined (1) differences in academic performance by structural and intermediary determinants of health, (2) associations between duration of college enrollment during the first year of the Coronavirus disease 2019 (COVID-19) pandemic, and (3) their two-way interactions on academic performance using data from two cohorts of freshmen undergraduate students.
Methods: Participants reported their demographic information, and their structural and intermediary determinants. The university reported academic performance at each semester. Mixed effects models tested repeated measures outcomes (i.e., grade point average (GPA) and courses where the grade received was D, F, Not passing, or Withdrawn (DFNW courses) and generalized linear models tested likelihood of graduation.
Results: More unfavorable structural and intermediary determinants were associated with an increased likelihood of receiving a DFNW grade (b = 0.33 and b = 0.40, p’s < 0.05, respectively). Greater unfavorable intermediary determinants were associated with a lower GPA (b = –0.07, p = 0.159). There were significant interactions between intermediary determinants of health and enrollment duration during COVID-19 for the likelihood of DFNW grades (b = –0.89, p = 0.008) and graduation (b = 1.31, p = 0.025), such that Cohort 1 fared worse than Cohort 2.
Discussion: The findings indicate that for those with more unfavorable intermediary determinants, there may have been an initial “shock” of the pandemic that stabilized over time.
1. Introduction
In response to increasing awareness of the unequal distribution of poor outcomes in health and well-being along characteristics like race, ethnicity, income, and sexual orientation, the World Health Organization’s (WHO) Commission on Social Determinants of Health, published a framework for conceptualizing the effects of social determinants of health and health inequities (Solar and Irwin, 2010). The WHO framework separates social determinants of health into structural determinants of health and health inequities and intermediary determinants of health. The framework suggests that differing socioeconomic and political contexts result in the stratification of individuals by factors such as income, education, race, gender, and occupation (Solar and Irwin, 2010). The WHO considers these macro-level factors (e.g., socioeconomic and political contexts) that result in stratification of individuals to be structural determinants of health and health inequities. While structural determinants are the root causes of health inequities, intermediary determinants are the conditions in which people are born, grow, live, work and age (e.g., neighborhood contexts, psychosocial stressors). Structural determinants give rise to stratification, which then lead to differential distribution of intermediary determinants. Structural stratifiers and intermediary determinants are often related to academic performance (Frazier et al., 2019), although academic outcomes are not the focus of the WHO framework. For example, if laws and policies (structural determinants) discriminate against a certain racial/ethnic minority (a structural stratifier), this can result in fewer educational opportunities or less income for that group relative to other groups. Consequently, members of that group may tend to live in lower-income neighborhoods and experience more stress and adversities and face more discrimination in their daily lives (i.e., unfavorable intermediary determinants) compared to members of other groups, which then disproportionately affect their health (i.e., health inequities due to structural determinants). Intermediary determinants are therefore the more proximate causes of poor outcomes that can explain the link between structural determinants of health and health inequities in academic performance, health, and well-being (Solar and Irwin, 2010).
Despite well-established research demonstrating risk factors for poor academic performance, minimal research has longitudinally examined risk factors for poor academic performance using a social determinants of health perspective. Thus, in the present study, we examine how structural stratifiers of health inequities and intermediary determinants of health (hereafter called structural stratifiers and intermediary determinants) contribute to academic performance in college students over time. Moreover, the ongoing Coronavirus disease 2019 (COVID-19) pandemic may be exacerbating the effects of structural stratifiers (Perry et al., 2021) and intermediary (Hoyt et al., 2021) determinants, especially when classes were remote during the first year of the pandemic. As such, we examined differential associations between structural stratifiers and intermediary determinants versus academic performance by time enrolled in college during the pandemic using two cohorts of students (see Figure 1 for conceptual model).
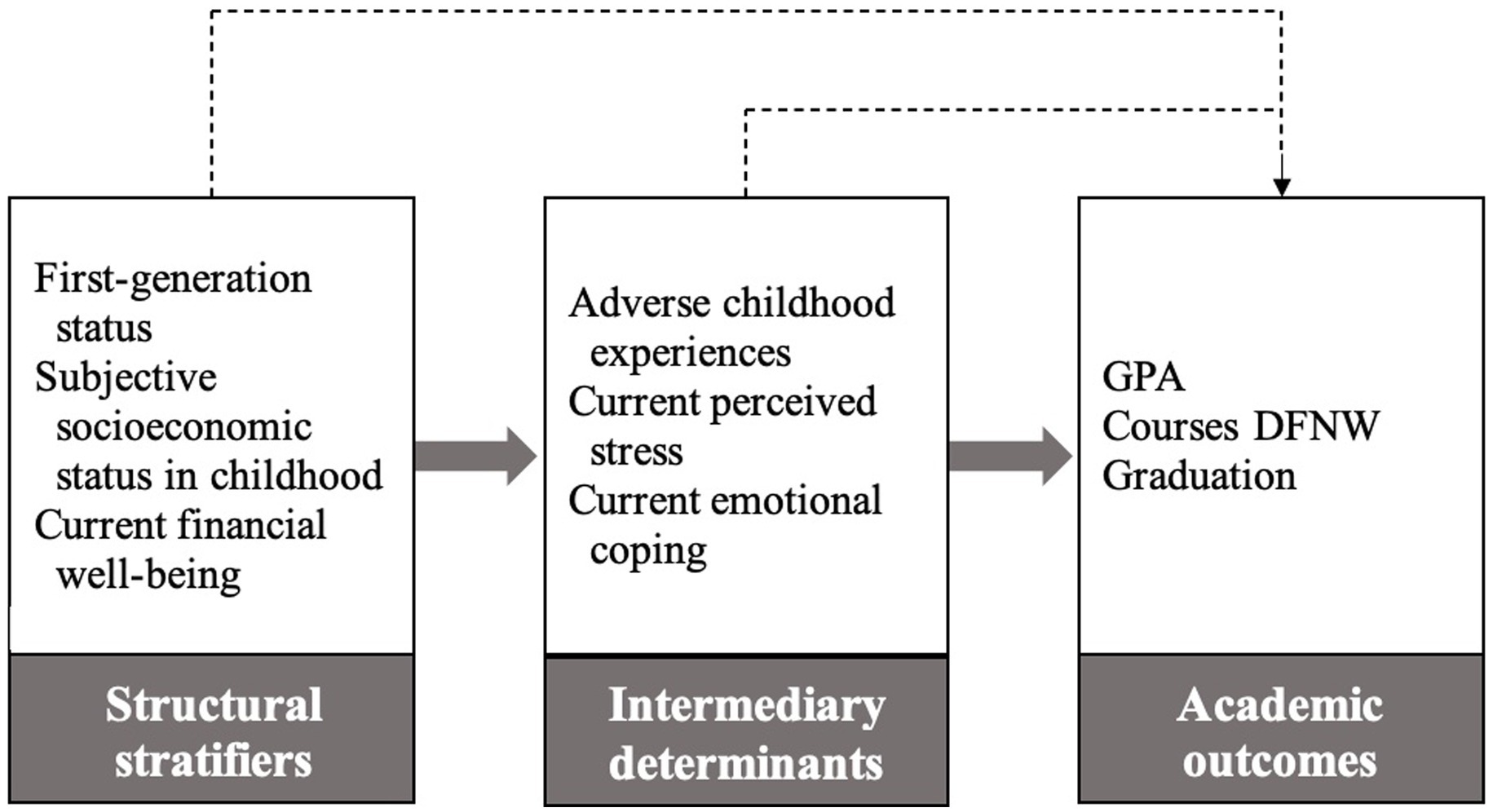
Figure 1. Conceptual figure (based on the broader WHO framework) of the associations between structural stratifiers, intermediary determinants of health, and academic outcomes tested in the current study.
There are a variety of factors that put students at risk for poor academic performance (Richardson et al., 2012). One meta-analysis showed that personality factors, academic motivation, learning strategies, stressors, and demographic characteristics (e.g., socioeconomic status (SES), first-generation status) are all associated with academic performance (Richardson et al., 2012). Some of these established risk factors can also be conceptualized as structural stratifiers and intermediary determinants. At the structural stratifier level, extant literature demonstrates that lower income and first-generation status are both associated with lower college performance (Chen, 2005). Intermediary determinants, such as adverse childhood experiences (ACEs), stress, and coping skills also have robust associations with academic performance. For instance, students with high levels of ACEs (e.g., Karatekin and Hill, 2019), often have lower grade-point-averages and are less likely to graduate (Pan et al., 2020). Psychological stress, which college students often report as the most impactful factor impeding their academic performance (American College Health Association, 2020), and maladaptive coping styles, are also associated with lower grades in college (Struthers et al., 2000; Vizoso et al., 2018).
Evidence suggests that structural stratifiers and intermediary determinants associated with increased risk for poor outcomes tend to co-occur. For example, students from lower socioeconomic backgrounds are also more likely to be first-generation college students (Bui, 2002), and both of these structural stratifiers link to more stressful life events (Hatch and Dohrenwend, 2007; Jenkins et al., 2013). In general, there are robust associations between income and education (both structural stratifiers leading to health inequities) and adversity, perceived stress, and perceived social support (all intermediary determinants of health). Given that risk factors also have a cumulative impact on poor outcomes across development (Evans et al., 2013), analyzing structural stratifiers and intermediary risk factors together may not only provide additional insight into which college students are most at risk of poor academic performance but also highlight the need for upstream solutions that target the root causes of academic inequities.
In March 2020, the WHO declared COVID-19 to be a global pandemic. To decrease the morbidity and mortality associated with COVID-19, college and university campuses closed and transitioned to remote learning for the majority of 2020, resulting in major disruptions to the normative structure of education (e.g., canceled classes, online courses, and altered course grading). Although COVID-19 infection rates are continuously changing, and the recent up-take of the vaccine has allowed for a return to many in-person classes and activities on campus (Junge et al., 2022), recent evidence highlights that the associations between structural stratifiers and intermediary determinants and academic performance in college students may be more pronounced during the pandemic. For example, Rodríguez-Planas (2022) found differential links to academic performance within usually high-performing college students depending on income (i.e., a structural stratifier of health inequities). Specifically, the grades and earned credits in Spring 2020 of top-performing low-income students decreased more so than those of their top-performing high-income peers. This trend may reflect the additional resources higher-income and non-first-generation college students have access to when overcoming challenges associated with the pandemic and remote learning. The pandemic has also caused great stress, placing additional strain on coping resources (i.e., intermediary determinants), with research suggesting that perceived stress among college students has increased over roughly the first year of the pandemic from pre-pandemic levels (i.e., Charles et al., 2021). Given the exacerbating effect of the pandemic on structural stratifiers and intermediary determinants, particularly during the first year of the pandemic, identifying how these risk factors accumulate to impact academic performance warrants further investigation.
Using data from students at a public, Midwestern US university, the aims of the current study were: (1) to examine differences in academic performance [i.e., grade point average (GPA), courses taken that did not count toward degree progress (DFNW courses), and likelihood of graduating within 4 years] by structural stratifiers and intermediary determinants over 4 years of enrollment, (2) to investigate whether academic performance differs between two cohorts of students that were enrolled in college for different durations during the first year of the COVID-19 pandemic, and (3) to examine the interactive effect of structural stratifiers and intermediary determinants and pandemic enrollment duration on academic performance. We expected that structural stratifiers and intermediary determinants would be associated with lower academic performance across each outcome (Aim 1). We further expected that being enrolled in college for a longer duration during the pandemic (i.e., Cohort 2) would be associated with lower academic performance (Aim 2). Lastly, we anticipated that the associations between structural stratifiers [i.e., first-generation status, financial well-being, and subjective socioeconomic status (SES)] and intermediary (i.e., perceived stress, ACEs, and emotional coping) determinants would be stronger for the cohort enrolled in college for a longer duration of time during the pandemic (i.e., Cohort 2).
2. Method
2.1. Participants
Two cohorts of freshmen undergraduate students were recruited from a large, public university in an urban, metropolitan area in [State; blinded for review] in Fall 2016 (Cohort 1: N = 160) and Fall 2017 (Cohort 2: N = 181). Participants were recruited through psychology courses that provided them with research credit for participation in the study. Despite being recruited from psychology courses, only ~10% of the sample were majoring in psychology (a liberal arts major at the university). Undergraduate colleges represented included liberal arts majors (46.6%) business majors (32.5%), biological science majors (11.2%), education and human development majors (7.8%), and other (1.9%). This distribution aligns with total undergraduate enrollment by college at [blinded for review; Institutional Data and Research], with the exception of enrollment in ‘other’ colleges. The enrollment distribution is as follows: liberal arts (41.33%), business (10.38%), biological sciences (7.62%), education and human development (7.55%), and other (33.12%).
Only students that were first-semester freshmen that had never attended another university were included in this study (Cohort 1: N = 103; Cohort 2: N = 165). As one of the substantive interests of the present investigation is the effect of the COVID-19 pandemic on trajectories of academic performance, only students that remained enrolled in the university during the Spring 2020 semester were included in the analysis. The full sample of first-semester freshmen is described in more detail elsewhere (blinded for review). The retained sample did not differ significantly from the complete sample in terms of age, gender, race, ethnicity, or GPA. However, significantly more participants were removed from Cohort 1 than from Cohort 2 (χ2 = 26.095; df = 1; p < 0.001). The final sample included a total of 223 students across Cohort 1 (N = 70; 67.1% female, 32.9% male; Mage = 18.20, SD = 0.44, Range = 18–20) and Cohort 2 (N = 153; 73.2% female, 26.8% male; Mage = 18.33, SD = 0.51, Range = 18–20). All students in Cohort 2 that were enrolled in college during the Spring 2020 semester remained enrolled until the end of the study period (i.e., until Spring 2021). The racial and gender composition of each cohort is presented in Table 1.
Pandemic restrictions began at [the university, blinded for review] around March 15, 2020. At this time, all in-person classes transitioned to online learning. Courses were taught synchronously on Zoom to the extent possible. Remote learning continued until Fall of 2021 (i.e., after both cohorts were expected to graduate). From the Spring 2020 semester to the Spring 2021 semester, the following grading policy exceptions were put in place: courses could be switched to/taken as Satisfactory/Not Satisfactory (S/N; equivalent to a C- or better) rather than an A-F grading scale. Courses that were taken as S/N during this time counted toward undergraduate program requirements and did not count toward credit limits on S/N classes. Courses taken as S/N did not earn any GPA points. This aligns with policies put in place across institutions throughout the US. The most implemented education-related COVID-19 policies included a switch to online only instruction from Spring 2020 to Spring 2021, with a return to in-person or hybrid instruction in beginning Fall 2022, and alterations to grading by allowing students to take courses as pass or fail (the equivalent to S/N at institution [blinded for review]) (Olneck-Brown, 2021).
2.2. Procedures
The current study was approved by the Institutional Review Board (IRB). Following enrollment into the study, participants provided consent. Next, participants completed a survey with a series of questionnaires through Qualtrics, a secure online survey tool. Upon completion, participants were provided with mental health resources to compensate for the sensitive quality of the survey questions. All participants received research credit.
2.3. Measures
2.3.1. Demographic information
Age was calculated from date of birth. Participants self-identified their race, ethnicity, and gender.
2.3.2. ACT/SAT scores
The university’s Office of Undergraduate Education provided each participant’s standardized test scores from admissions applications upon enrollment into the study. Because most participants had an American College Test (ACT) score, we used ACT scores or calculated an ACT score equivalent. Specifically, for the eight participants that did have an ACT score, we converted the Scholastic Aptitude Test (SAT) scores into equivalent ACT scores.1
2.3.3. Structural stratifiers of health and health inequities
2.3.3.1. First generation status
During the admissions process, participants self-identified whether they were first generation status (i.e., neither parent nor guardian obtained a 4-year college degree). Upon enrollment into the study, the university Office of Undergraduate Admissions provided each participant’s first-generation college student status.
2.3.3.2. Subjective SES during childhood
Subjective socioeconomic status (SES) was assessed with one item (Adler et al., 2000). Participants were shown a ladder, with steps numbered from 1 to 10, and instructed to think of the ladder as a representation of where people stand in society. The top of the ladder represented people with higher standing (e.g., the best off, most money, most education, and best jobs), whereas the bottom of the ladder represented those that were the worst off (e.g., least money, least education, worst or no job). Participants indicated the rung of the ladder that best represented their family’s standing while they were growing up (i.e., ages 0–18).
2.3.3.3. Financial well-being during freshman year
The InCharge Financial Distress/Financial Well-Being Scale (Prawitz et al., 2006) is an 8-item measure that was used to assess the current level of distress regarding one’s finances. Possible scores range from “overwhelming financial distress” (8) to “no financial distress” (80), as the scores for individual items were rated from 1 to 10. The measure had good internal consistency (α = 0.90) in the present study.
2.3.4. Intermediary determinants of health
2.3.4.1. Adverse childhood experiences (ACES)
Adverse childhood experiences (ACEs) were assessed with 31 questions that assessed maltreatment, household dysfunction, separation from caregivers, peer and sibling victimization, discrimination, community violence exposure, property victimization, bad accident or illness of someone close, and social isolation (Karatekin and Hill, 2019). Participants responded yes or no to each item and responses were summed, with a maximum score of 31. The composite measure has shown good reliability and validity for use with college students, including acceptable test–retest reliability and concurrent and convergent validity (Karatekin and Hill, 2019; Gresham and Karatekin, 2022). Internal consistency in the present sample was good (α = 0.82).
2.3.4.2. Perceived stress during freshman year
The Perceived Stress Scale (Cohen et al., 1983) is a 10-item measure used in the present study to assess how often respondents perceived situations in their life as stressful over the past month. Items were rated on a 5-point Likert scale from “never” (1) to “very often” (5) and were summed, with higher scores indicating higher levels of stress. The measure had good internal consistency in the present study (α = 0.90).
2.3.4.3. Emotional coping during freshman year
Coping was assessed using the emotional coping subscale of the Coping Inventory for Stressful Situations-Short Form scale (Cohan et al., 2006). The seven items were rated on a 5-point Likert scale from “not at all” (1) to “very much” (5) and summed, with higher scores corresponding to a higher level of emotion-oriented coping, or higher engagement in strategies like self-blame, blaming others, and emotion containment. The measure has good validity for use with undergraduate students and had high internal consistency in the present study (α = 0.87).
2.3.5. Academic outcomes
All academic outcomes were obtained from the university’s Office of Undergraduate Education.
2.3.5.1. GPA
Cumulative grade point average (GPA) was obtained each semester for the 4 years following enrollment in the study (i.e., Fall 2016 for Cohort 1, Fall 2017 for Cohort 2), for a total of eight semesters. Semesters were coded from “0” to “7,” regardless of the chronological timing of the semester (i.e., Fall 2016 was “0” for Cohort One, whereas Fall 2017 was “0” for Cohort Two).
2.3.5.2. DFNW courses
Courses that did not count toward degree progress were reported each semester for the 4 years after study enrollment (DFNW courses). This consisted of courses where the grade received was “D,” “F,” or “Not-passing,” as well as any courses from which the student Withdrew (DFNW). The value coded for each semester was “yes” (1) or “no” (0).
2.3.5.3. Graduation
Whether or not a student graduated was reported each semester for 4 years following enrollment in the university. At the end of the four-year follow-up period, whether a student graduated was coded dichotomously as “yes” (1) or “no” (0).
2.4. Data analysis
Data and analytic code for this study will be made available upon request. The analyses for the present study were post-hoc, as the pandemic could not have been foreseen when the study started in 2016, and thus were not pre-registered. All analyses were conducted in R version 4.2.0. (R Core Team, 2023). Data were examined for outliers, missing values, and deviations from normality. Internal reliability of each scale was assessed, with α greater than 0.70 generally indicating acceptable reliability (Gliem and Gliem, 2003). We present the descriptive statistics of participant demographic information and key study variables in Table 1. Relationships among study variables were evaluated with bivariate Pearson’s correlations. Structural stratifiers and intermediary determinants were examined for collinearity. Chi-square tests and t-tests examined differences between cohorts on key study variables.
Weighted composite variables were created from multiple indicators of structural stratifiers (i.e., first-generation college student status, subjective SES, and financial wellbeing) and intermediary determinants (ACEs, perceived stress, and emotional coping) and combined into their own respective variables. Principal components analysis (PCA) is a useful tool for creating weighted composite variables (Song et al., 2013) which allow for each item to be weighted proportional to its relevance for the underlying construct. We conducted PCA with varimax rotation was conducted to extract sample weights for each indicator variable detailed above (i.e., one for each composite variable). The PCA models were restricted to load onto a single factor. Each indicator variable was then multiplied by the weight derived from the PCA. The indicators were summed, with higher scores indicating higher risk.
2.4.1. GPA
Mixed effects modeling was used to estimate within- and between-person differences in GPA trajectories over 4 years. As linear mixed effects models assume that both the outcome and residuals are normally distributed, generalized linear mixed effects modeling (GLMEM) with a gamma distribution and a linear link was specified due to the skew in GPA (Peralta et al., 2018). The intra-class correlation (ICC) was evaluated using the baseline model to determine the appropriateness of a mixed effects model, with a value >0.10 indicating a mixed effects model should be used (Musca et al., 2011).
2.4.2. DFNW courses
Mixed effects modeling was also used to estimate within- and between-person differences in DFNW courses taken over time. GLMEM with a binomial link was used due to the dichotomous nature of the outcome (Peralta et al., 2018). Like GPA, the ICC was evaluated to determine the appropriateness of a mixed effects model.
2.4.3. Graduation
Binary logistic regression was used for models predicting graduation in 4 years. Analyses were performed using the R statistics package version 3.6.2 (R Core Team, 2021).
Model assumptions for each outcome were tested and met. All analyses controlled for gender. Race (racism) and ethnicity (cultural racism) are meaningful structural stratifiers but were excluded from the analyses due to limited variability. For the repeated measures outcomes (i.e., GPA and courses DFNW), a series of four mixed-effects models were specified. First, a baseline model including the intercept and the random and fixed effect of time was specified (Model 0). Structural stratifiers and intermediary determinants were then added to the model to examine whether they predicted academic performance (Aim 1, Model 1). To test whether the duration of enrollment in college during the pandemic was associated with academic performance, cohort (i.e., whether one belonged to Cohort 1 or Cohort 2) was added to the model (Aim 2, Model 2). Finally, two-way interactions between structural stratifiers and intermediary determinants and cohort were added to the model to examine whether the associations between structural stratifiers and intermediary determinants and academic performance were exacerbated by the pandemic (Aim 3, Model 3). Models predicting the likelihood of graduating in 4 years proceeded in the same iterative manner described above, without the effect of time.
Models were evaluated based on Akaike Information Criterion (AIC) and analysis of variance was used to compare nested models. For binary outcomes (i.e., courses DFNW and graduation), unstandardized beta coefficients and odds ratios were reported to describe the magnitude of the findings. R package lme4 version 1.1–27.1 was used to perform the analyses (Bates et al., 2014). Simple slope analyses were performed to probe significant interactions, with +/−1 SD serving as high and low values of the moderator (i.e., structural stratifiers or intermediary determinants).
3. Results
Descriptive statistics and correlations among study variables are presented in Tables 1 2, respectively. Structural stratifiers and intermediary determinants were only moderately correlated (r = 0.43, p < 0.05). Thus, structural stratifiers and intermediary determinants were included in the same model. Cohort 1 and Cohort 2 did not significantly differ by age, race, ethnicity, gender, SAT/ACT scores, GPA, courses DFNW, graduation, or on the indicators and composite variables for structural stratifiers and intermediary determinants.
3.1. GPA
To determine whether structural stratifiers and intermediary determinants, duration of enrollment during the COVID-19 pandemic, and their interactions, predicted GPA over time, a series of generalized linear mixed effects models were performed. A spaghetti plot of individual- and mean-level GPA by cohort is presented in Figure 2. The AIC, parameter estimates, and p-values from a series of mixed effects models are presented in Table 3. There was a large amount of between-person variability (ICC > 0.90), suggesting a mixed effects model was appropriate. There was a relatively shallow slope (b = 0.001), indicating GPA is relatively stable over time. Thus, a reduced model was tested, eliminating the random effect of time. A model comparison between the baseline and reduced models indicated that the fit worsened with the exclusion of the random effect of time (p < 0.05), so the random effect of time was retained. The fixed effect of the intercept indicated that the average GPA during the first semester of college was relatively high (b = 3.55, p < 0.001). The fixed effect of time was not significant (b = 0.01, p = 0.426), meaning student GPA scores did not significantly change over time. Unfavorable intermediary determinants during freshman year significantly predicted lower GPA over time (b = −0.07, p = 0.014), but unfavorable structural stratifiers were not a significant predictor (b = −0.04, p = 0.159). The main effect of cohort was not significant (b = −0.06, p = 0.513), suggesting the duration of college enrollment in relation to the pandemic was not associated with changes in GPA. It is important to note that the AIC increased when cohort (M.2) was added to the model. However, the ΔAIC was <2 and may not indicate significantly better fit. The interactions between cohort and structural stratifiers (b = −0.001, p = 0.905) and intermediary determinants (b = −0.0004, p = 0.953) were also not significant (presented in Figures 3A, 4A, respectively). The AIC increased significantly when interactions between structural stratifiers/intermediary determinants and cohort (M.3) were added to the model, suggesting that M.2 was more parsimonious.
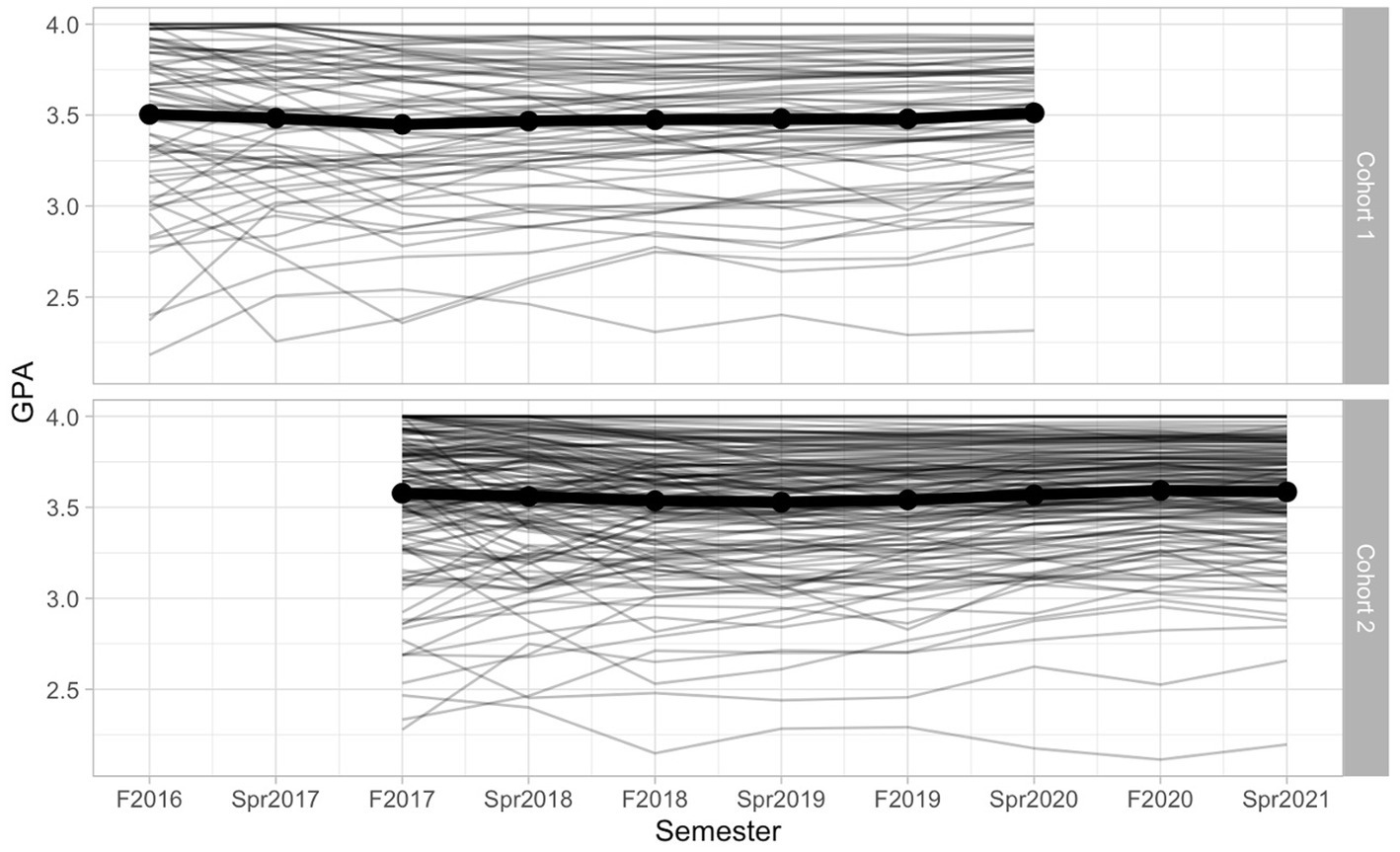
Figure 2. Spaghetti plot of individual (gray lines) and mean (thick, black lines) trajectories of GPA over time faceted by cohort.
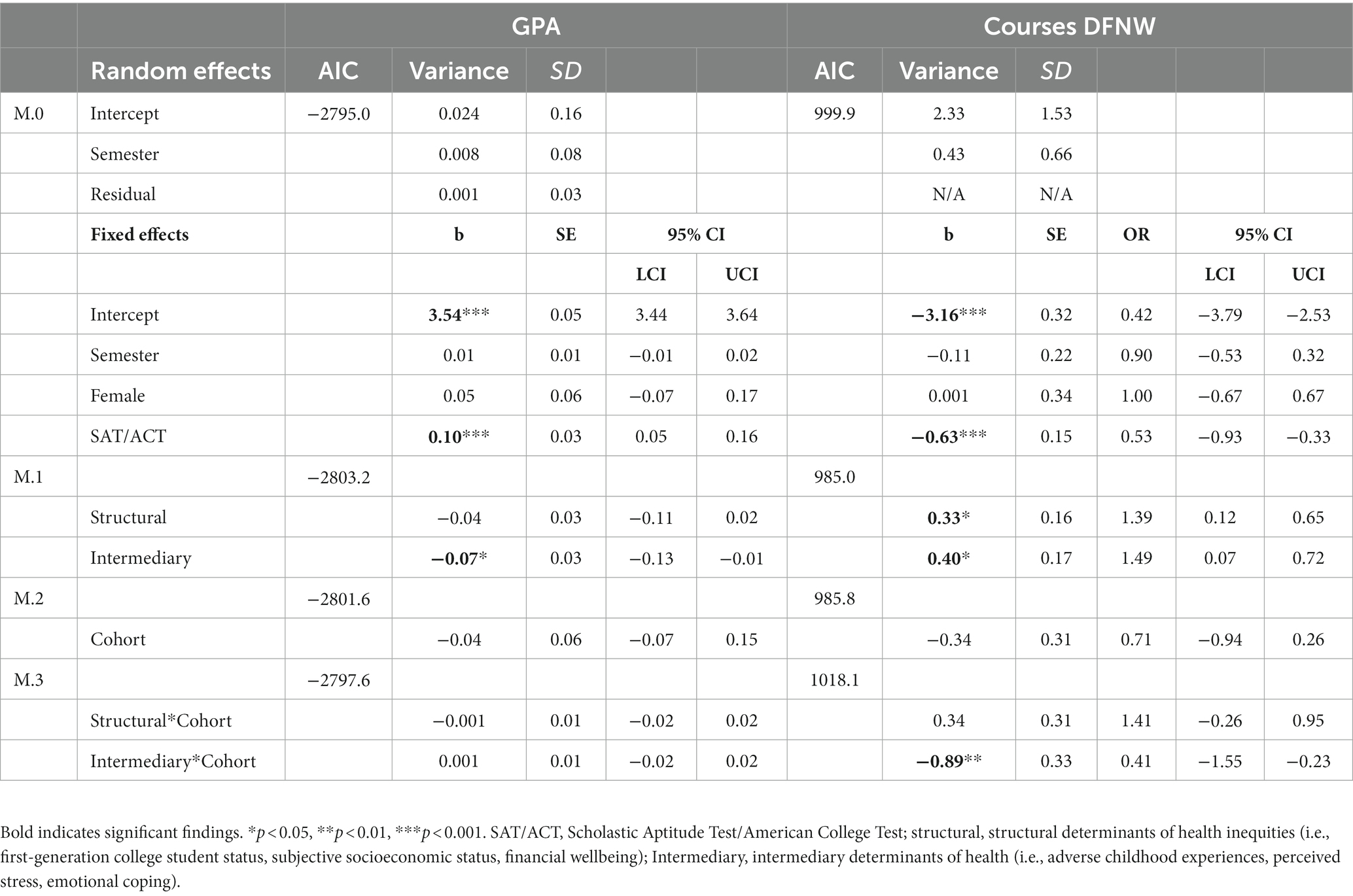
Table 3. Results from a series of generalized linear mixed effects models predicting GPA and likelihood of taking courses DFNW over time.
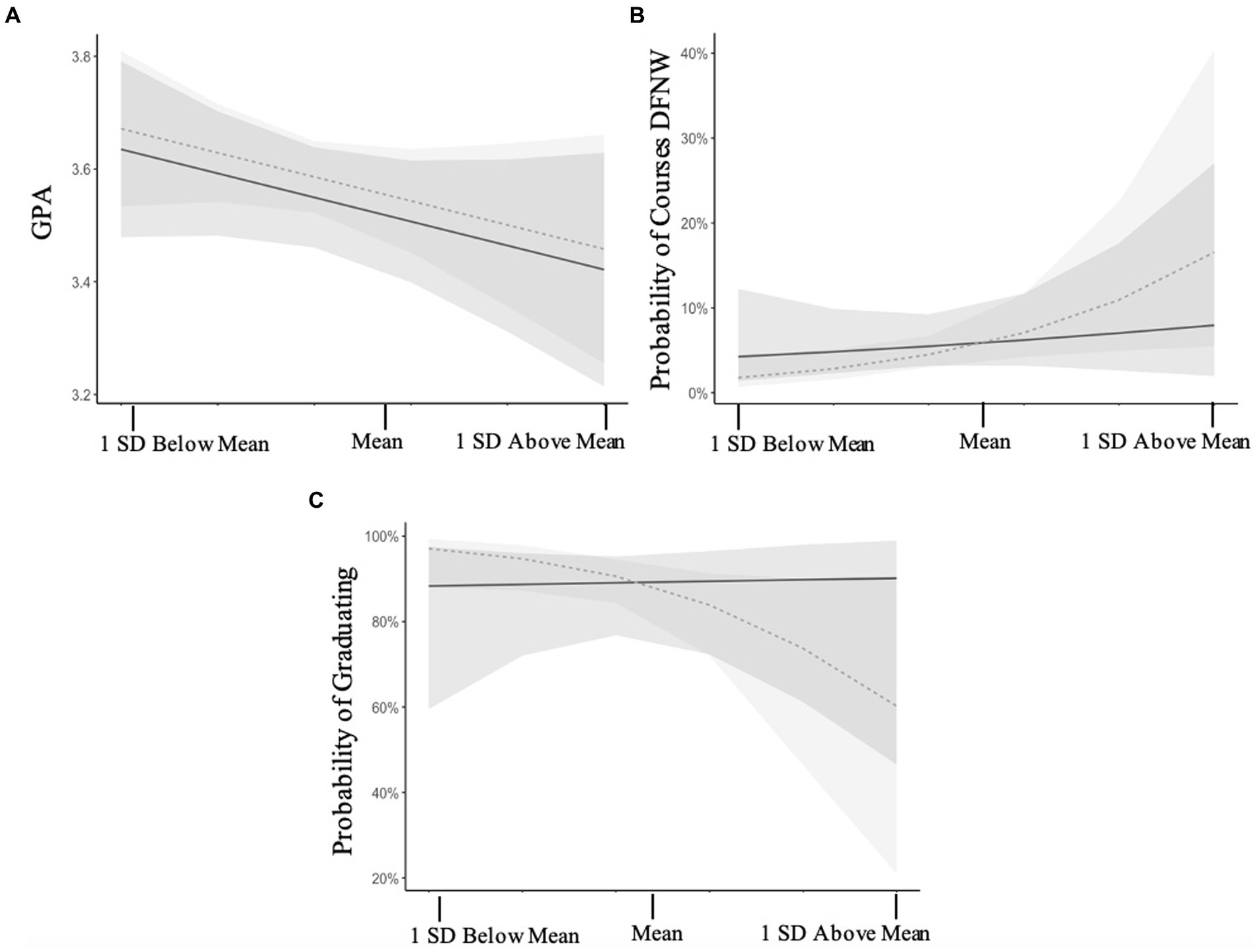
Figure 3. Plot of interactions between risky structural determinants of health inequities (i.e., first-generation status, subjective SES and financial wellbeing) and cohort (i.e., duration of enrollment during the COVID-19 pandemic; dotted line = Cohort 1; solid line = Cohort 2) for (A) linear models predicting GPA, and logit models predicting (B) likelihood of receiving a DFNW grade and (C) likelihood of graduating within four years.
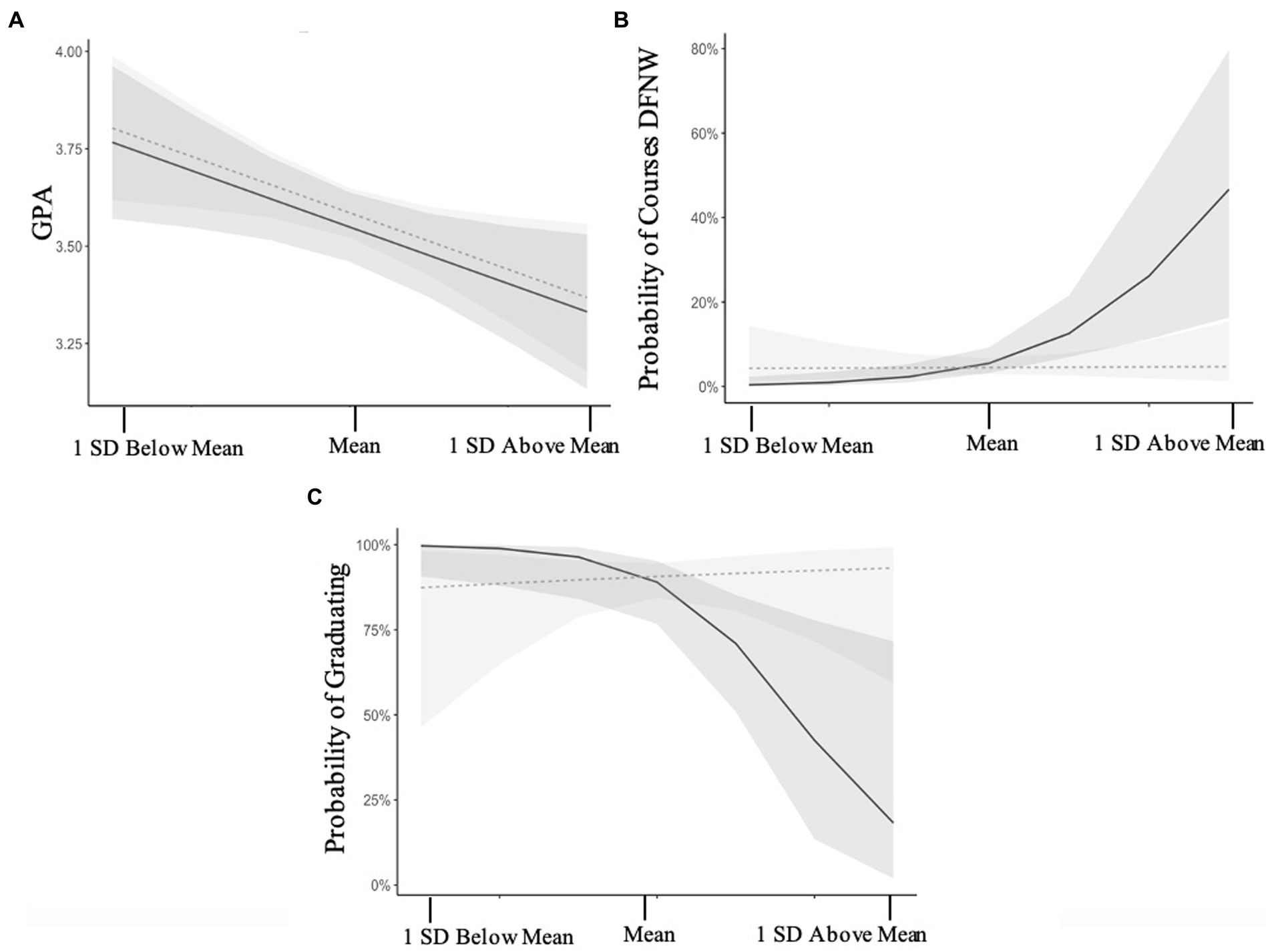
Figure 4. Plot of interactions between risky intermediary determinants of health (i.e., ACES, perceived stress, and emotional coping) and cohort (i.e., duration of enrollment during the COVID-19 pandemic; solid line = Cohort 1; dotted line = Cohort 2) for linear models predicting (A) GPA, and logit models predicting (B) likelihood of receiving a DFNW grade and (C) likelihood of graduating within 4 years.
3.2. Courses DFNW
Generalized linear mixed effects modeling tested whether DFNW courses were predicted by structural stratifiers and intermediary determinants and duration of enrollment during COVID-19, and their two-way interactions. Table 3 displays the AIC, parameter estimates, and p-values from a series of mixed effects models. Mixed effects modeling was deemed appropriate (ICC = 0.46). Overall, the likelihood of taking a course where the grade received was DFNW did not significantly change over time (b = −0.11, OR = 0.90, p = 0.615). Unfavorable structural stratifiers predicted a 39% increased likelihood of receiving a DFNW grade (b = 0.33, OR = 1.39, p = 0.042). Similarly, greater unfavorable intermediary determinants was associated with a 49% increased likelihood of receiving a DFNW grade (b = 0.40, OR = 1.49, p = 0.018). There was no significant difference in the likelihood of receiving a DFNW grade between Cohort 1 and Cohort 2 (b = −0.34, OR = 0.71, p = 0.266). As with the models predicting GPA, the AIC increased slightly when cohort (M.2) was added to the model. The interaction between structural stratifiers and cohort was also not significant (b = 0.34, OR = 1.41, p = 0.259; see Figure 3B), suggesting that duration of enrollment in college during the pandemic was not independently associated with taking DFNW courses nor in the context of structural stratifiers. However, the interaction between intermediary determinants and cohort predicted the likelihood of receiving a DFNW grade (b = −0.89, OR = 0.41, p = 0.008). Simple slope analyses revealed that in the context of high (i.e., more unfavorable) intermediary determinants, Cohort 2 was 67% less likely than Cohort 1 to receive a DFNW grade (b = −1.10, OR = 0.35, p = 0.010). However, when intermediary determinants were more favorable, the cohorts did not differ in the likelihood of receiving a DFNW grade (b = 0.68, OR = 1.97, p = 0.170; see Figure 4B). The interaction results should be interpreted with caution, given that the AIC increased significantly upon adding the interactions between structural stratifiers/intermediary determinants and cohort to the model.
3.3. Graduation
Parameter estimates from a series of binary logistic regression models predicting the likelihood of graduating in 4 years from structural stratifiers and intermediary determinants and duration of enrollment during COVID-19, and their interactions, are presented in Table 4. Both structural stratifiers (b = −0.36, OR = 0.70, p = 0.124) and intermediary (b = −0.42, OR = 0.66, p = 0.106) determinants trended toward significance. Cohort was not a significant predictor of graduating in 4 years (b = 0.44, OR = 1.55, p = 0.314), nor was the interaction between structural stratifiers and cohort (b = −0.66, OR = 0.52, p = 0.156; see Figure 3C). The interaction between intermediary determinants and cohort was significant (b = 1.31, OR = 3.70, p = 0.025), such that Cohort 2 was 4.42 times more likely to graduate in 4 years than Cohort 1 in the context of high intermediary determinants (b = 1.49, OR = 4.42, p = 0.023). However, there was no significant difference between cohorts when intermediary determinants were low (b = −1.13, OR = 0.32, p = 0.213; see Figure 4C).
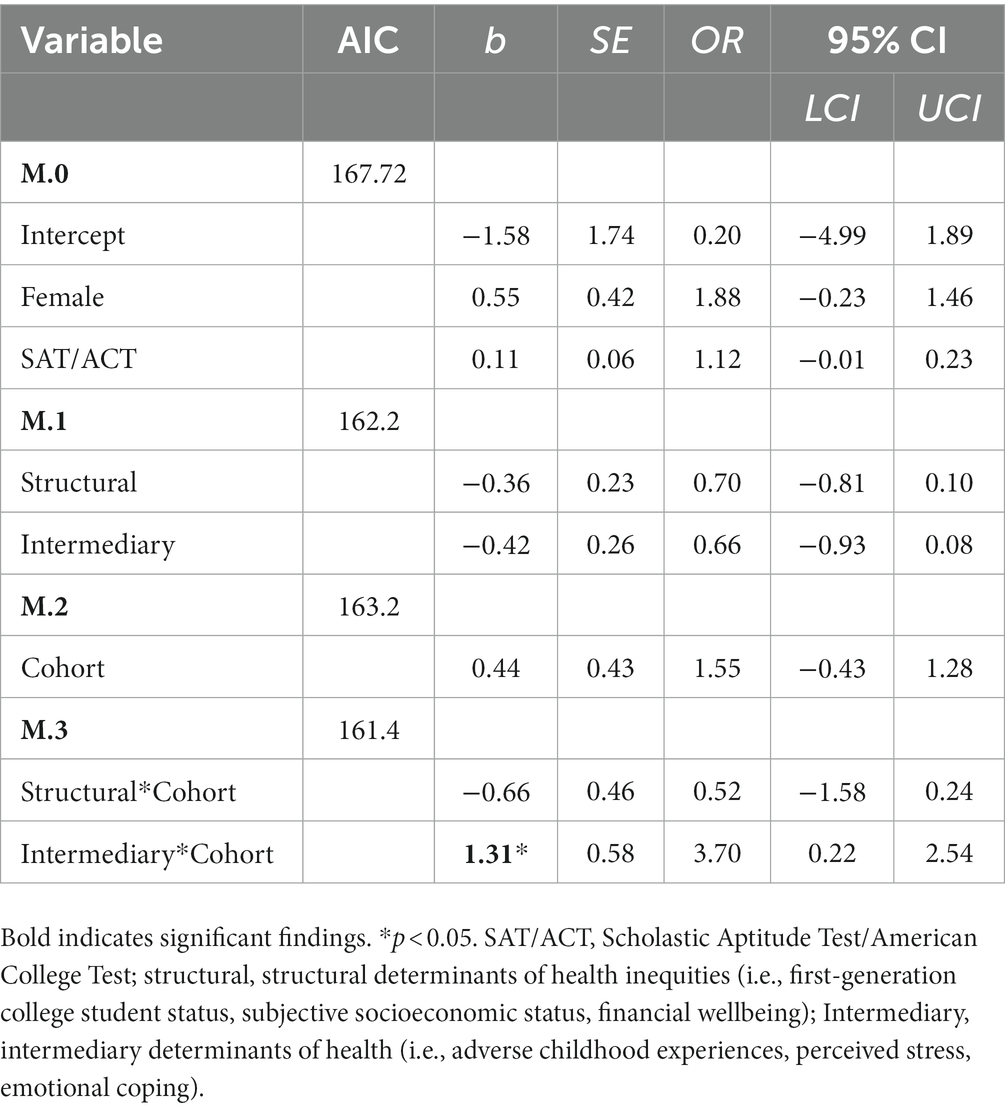
Table 4. Results from a series of binary logistic regression models predicting likelihood of graduating in 4 years.
4. Discussion
Overall, GPA was relatively high (3.58 out of 4.0), and most students graduated (~87%). Despite these positive outcomes, nearly half of students across cohorts received a grade of DFNW in at least one course. Aim 1 (i.e., the role of structural stratifiers and intermediary determinants of health and health inequities in predicting academic performance over time) was partially supported, such that unfavorable structural stratifiers and intermediary determinants were associated with a lower GPA and an increased likelihood of receiving a grade of DFNW, but not the likelihood of graduating in 4 years. There was no main effect of Cohort on any of the examined outcomes, indicating that the duration of enrollment during the first year of the pandemic did not have an overall impact on academic performance over time (Aim 2). However, in partial support for Aim 3, there were significant interactions between intermediary determinants and cohort (i.e., duration of enrollment in college during the pandemic) for DFNW grades and the likelihood of graduating in 4 years. Specifically, Cohort 1 (enrolled for only half a semester at the very beginning of the pandemic) was more likely to receive DFNW grades and less likely to graduate within 4 years than Cohort 2, but only in the context of high unfavorable intermediary determinants. This ran contrary to our expectations that Cohort 2 (enrolled during the first year of the pandemic) would experience poorer academic performance. The findings indicate that for those with unfavorable intermediary determinants during freshman year (i.e., more ACEs, greater perceived stress, greater use of emotional coping), there may have been an initial “shock” that stabilized over time. Surprisingly, the interactions between cohort and structural stratifiers for the three examined outcomes, as well as the interaction between cohort and intermediary determinants for GPA, were not significant.
4.1. Comparison with previous research
4.1.1. Association between structural stratifiers and intermediary determinants and academic performance
Although the social determinants of health literature, including the WHO framework (Solar and Irwin, 2010), typically emphasizes the effects of structural stratifiers and intermediary determinants on physical and mental health outcomes, the findings of the present study also support the pervasive role of these factors in educational achievement. Consistent with previous research, we found that structural stratifiers and intermediary determinants predict both GPA and courses taken that do not count toward degree progress (Struthers et al., 2000; Vizoso et al., 2018; Gresham and Karatekin, 2022). Contrary to our expectations, structural stratifiers and intermediary determinants were not associated with likelihood of timely graduation (i.e., within 4 years of enrollment). This lack of association contradicts previous research demonstrating that structural stratifiers, such as income, first-generation status (Cahalan et al., 2022), and intermediary determinants like ACEs (Pan et al., 2020) are associated with a lower likelihood of graduation. Nonetheless, the demonstrated importance of educational attainment on health through mechanisms such as social stratification (Solar and Irwin, 2010) highlights the need for future investigations into the influence of structural determinants and stratifiers and intermediary determinants on academic performance.
In terms of future research, these findings suggest that examining individual risk factors for poor academic performance, as opposed to simultaneously assessing these risks in a single, coherent, multi-level framework, hinders the ability to identify students most at risk, given that many of these risk factors co-occur (Bui, 2002). A simple cumulative-risk framework also misses the multi-level nature of these determinants and the interplay of how upstream factors influence more downstream, proximate determinants. Although the WHO’s framework emphasizes health outcomes, it and similar frameworks may be useful for conceptualizing how these risks may coincide and how they are inter-related. We used only a small subset of structural stratifiers and intermediary determinants based on secondary data analysis of a larger study. Thus, there is a need for future research extending to other structural determinants (e.g., laws, policies and their political determinants), structural stratifiers (e.g., racism, occupation, social class), and intermediary determinants (e.g., neighborhood cohesion, material circumstances, biomarkers of health risk) to test possible mechanisms linking these factors to educational outcomes. Moreover, attention should be given to the theorized sequential nature of structural statifiers and intermediary determinants in future research, by testing intermediary determinants as mediators of the association between structural stratifiers and academic performance, which was not possible in the present study.
4.1.2. Association between pandemic exposure (i.e., cohort) and academic performance
Currently, the literature is limited on associations between pandemic exposure and academic performance. However, the present study differs from a similar COVID-19 related study by Chang and Baer (2021). While we found that the trajectory of GPA did not differ according to the duration of time students were enrolled in college in relation to COVID-19, Chang and Baer (2021) found that course pass rate significantly differed pre- and mid-pandemic. These discrepant findings may be attributable to methodological differences. For example, Chang and Baer (2021) used data from a single year-long course, compared to the utilization of cumulative GPA over 4 years in the present study.
It is unclear why the pandemic, represented by cohort membership in the present study, was not associated with academic performance given the disruptions to learning that took place over the first year of the pandemic (Ali, 2020). It is possible that the accommodations made by universities at the onset of the pandemic, such as increased flexibility with remote learning, help explain the findings of the present study. However, additional research is needed to better understand whether, how, for whom, and in what contexts the COVID-19 pandemic may be associated with academic performance. Specifically, one avenue for future research is to examine how the association between the pandemic and academic performance continues to change over time, as the expectations and mandates related to the pandemic change, as people continue to contract COVID-19, as the number of people with long COVID2 and other downstream health effects of COVID-193 increases, and as students in various stages of their educational journey at the height of pandemic disruptions (e.g., middle and high school) transition to college.
4.1.3. Interactions between structural and intermediary determinants and cohort membership
While there are no directly comparable findings to discuss, extant research shows that the COVID-19 pandemic has exacerbated disparities among myriad outcomes, including health and mortality (Green et al., 2021 Miller et al., 2021 Xiao et al., 2022). The findings demonstrating an exacerbating association between structural stratifiers and intermediary determinants and health in the context of the COVID-19 pandemic is consistent with our findings for educational outcomes among college students (Green et al., 2021 Xiao et al., 2022). However, contrary to our expectations, belonging to Cohort 1 (i.e., having <1 semester of exposure to the pandemic) was associated with worse academic performance than belonging to Cohort 2 (i.e., having ~3 semesters of exposure to the pandemic) in the context of unfavorable intermediary determinants. One possible explanation for this finding is that the initial disruptions to education (e.g., abrupt shift to remote learning, school closures, etc.) had the greatest impact on students with other risk factors. Since Cohort 1 was expected to graduate the same semester that COVID-19 disruptions began, there was inadequate time to recover from or adjust to these changes prior to graduation for students already facing inequities.
Additional longitudinal research on educational outcomes for students with unfavorable structural stratifiers and intermediary determinants that spans pre- and post-March 2020 is needed to better understand the potential mechanisms underlying the findings of our study. Like the suggested research above, interactions between structural stratifiers and intermediary determinants and the pandemic using additional social determinants of health and health inequities prevalent among college students (e.g., low income, heightened stress, etc.) need to be examined to tease apart the role of the pandemic in academic performance in the context of additional risks and increasing inequities. Moreover, studies that accommodate the theorized sequential nature of structural and intermediary determinants in the context of the pandemic are needed.
4.2. Strengths and limitations
The findings from this study are strengthened by the use of multiple indicators of structural stratifiers and intermediary determinants, which were assessed before the pandemic began. Another strength is the use of two cohorts at different levels of academic progress at the onset of the pandemic, which allowed us to explore how the first year of the pandemic impacted students at different points in their education. Finally, a prospective, longitudinal design for assessment of academic problems and the use of administrative data rather than self-report for the tested academic outcomes further strengthens the method and findings.
Despite these strengths, the study sample was limited to students at one major state university and was predominantly White and female, and thus results may not be representative of all US college students. However, the findings of the present study generally align with nationally representative studies including students from multiple institutions in terms of the deleterious associations between structural stratifiers and intermediary determinants and academic outcomes (Pan et al., 2020). Further, we found significant associations between structural stratifiers and intermediary determinants and academic performance, despite the use of a more advantaged sample in the present study, highlighting the importance of these factors in predicting poor academic outcomes. This study was also limited by the use of retrospective reports to assess structural stratifiers and intermediary determinants, as responses may be influenced by memory limitations and mood states (Sato and Kawahara, 2011). Additionally, the study began in Fall 2016 with the self-report measures of structural stratifiers and intermediary determinants collected at baseline (i.e., Fall 2016 for Cohort 1 and Fall 2017 for Cohort 2) and included the longitudinal tracking of academic outcomes. Lastly, Cohort 1 was affected by the pandemic for only half of a semester, which may not have been enough time to attribute our findings to the pandemic.
4.3. Practical implications
The present study is valuable for understanding how to identify and assist college students most at-risk for poor educational outcomes (i.e., students with multiple risk factors). While policies and practices exist at the university level to reduce the disparities among students facing different structural and intermediary barriers to success, these programs often remain inaccessible and/or target only one risk factor, forcing students with co-occurring risks to seek resources from multiple programs. Even the federally funded TRIO, which serves low-income students, first-generation college students, and/or students with disabilities, has eight separate programs, potentially increasing the burden on multiply disadvantaged students.
It is also important to note that while this study indicates that both structural stratifiers and intermediary determinants are important for academic performance, and thus meaningful targets for intervention, it is structural determinants that are theorized to lead to stratification by factors such as race, income, and gender, which, in turn, lead to differential distribution of intermediary determinants. As such, it is not enough to simply treat the symptoms that arise from the downstream consequences of inequity (e.g., mental health support on college campuses). Although these programs are critical for individuals facing adversity, targeting the structural conditions and mechanisms that may give rise to poor outcomes is sorely needed. Thus, increasing financial accessibility to higher education for individuals from low-income backgrounds and pushing legislators to enact policies that promote livable and equitable wages are potential avenues for addressing structural determinants.
The present study also has important implications for how to assist students, particularly those with unfavorable structural stratifiers and intermediary determinants, during current and future pandemics to promote academic success. Importantly, there is a need for administrators to institute university-wide accommodations due to increased student needs and increase financial support for programs that benefit students from underrepresented backgrounds. Moreover, instructors should be receptive to increased student needs during prolonged exposure to stressful events.
4.4. Conclusion
This prospective longitudinal study makes a meaningful contribution to the literature by adding to the accumulating evidence on the role of structural stratifiers and intermediary determinants of health and health inequities in predicting academic performance and improving our understanding of the how the pandemic relates to multiple college achievement outcomes. The findings demonstrate that more unfavorable structural stratifiers and intermediary determinants are associated with poor academic performance. Additionally, although the pandemic may not have a direct contribution to academic performance, the very beginning of the pandemic may have exacerbated the impact of intermediary determinants (i.e., perceived stress, emotional coping, and ACEs) experienced by students prior to and during their first semester of college. This is particularly true for students expected to graduate in Spring 2020, when pandemic disruptions first took place.
Data availability statement
The raw data supporting the conclusions of this article will be made available by the authors, without undue reservation.
Ethics statement
The studies involving humans were approved by University of Minnesota Institutional Review Board. The studies were conducted in accordance with the local legislation and institutional requirements. The participants provided their written informed consent to participate in this study.
Author contributions
BG: conceptualization, formal analysis, methodology, writing – original draft, visualization. FC: conceptualization, methodology, writing – review and editing. CK: conceptualization, methodology, investigation, writing – review and editing, visualization, supervision. All authors contributed to the article and approved the submitted version.
Funding
The authors gratefully acknowledge support from the Minnesota Population Center (P2C HD041023) from the Eunice Kennedy Shriver National Institute of Child Health and Human Development Population Research Infrastructure Program.
Conflict of interest
The authors declare that the research was conducted in the absence of any commercial or financial relationships that could be construed as a potential conflict of interest.
Publisher’s note
All claims expressed in this article are solely those of the authors and do not necessarily represent those of their affiliated organizations, or those of the publisher, the editors and the reviewers. Any product that may be evaluated in this article, or claim that may be made by its manufacturer, is not guaranteed or endorsed by the publisher.
Footnotes
References
Adler, N. E., Epel, E. S., Castellazzo, G., and Ickovics, J. R. (2000). Relationship of subjective and objective social status with psychological and physiological functioning: preliminary data in healthy, white women. Health Psychol. 19, 586–592. doi: 10.1037/0278-6133.19.6.586
Ali, W. (2020). Online and remote learning in higher education institutes: a necessity in light of COVID-19 pandemic. High. Educ. Stud. 10, 16–25. doi: 10.5539/hes.v10n3p16
American College Health Association (2020). National college health assessment III: undergraduate student reference group executive summary. Silver Spring, MD: American College Health Association.
Bates, D., Mächler, M., Bolker, B., and Walker, S. (2014). Fitting linear mixed-effects models using lme4. ArXiv Preprint. doi: 10.48550/arXiv.1406.5823
Bui, K. V. T. (2002). First-generation college students at a four-year university: background characteristics, reasons for pursuing higher education, and first-year experiences. Coll. Stud. J. 36, 3–12.
Cahalan, M. W., Addison, M., Brunt, N., Patel, P. R., Vaughan, T. III, Genao, A., et al. (2022). Indicators of higher education equity in the United States: 2022 historical trend report. Pell institute for the study of opportunity in higher education. Available at: https://files.eric.ed.gov/fulltext/ED620557.pdf
Chang, A., and Baer, C. M. (2021). Academic outcomes of undergraduates learning at the age of COVID-19 pandemic. Docens Ser. Educ. 1, 13–31.
Charles, N. E., Strong, S. J., Burns, L. C., Bullerjahn, M. R., and Serafine, K. M. (2021). Increased mood disorder symptoms, perceived stress, and alcohol use among college students during the COVID-19 pandemic. Psychiatry Res. 296:113706. doi: 10.1016/j.psychres.2021.113706
Chen, X. (2005). First-generation students in postsecondary education: a look at their college transcripts. Washington, DC: US Department of Education, National Center for Education Statistics.
Cohan, S. L., Jang, K. L., and Stein, M. B. (2006). Confirmatory factor analysis of a short form of the coping inventory for stressful situations. J. Clin. Psychol. 62, 273–283. doi: 10.1002/jclp.20211
Cohen, S., Kamarck, T., and Mermelstein, R. (1983). A global measure of perceived stress. J. Health Soc. Behav. 24, 385–396. doi: 10.2307/2136404
Evans, G. W., Li, D., and Whipple, S. S. (2013). Cumulative risk and child development. Psychol. Bull. 139, 1342–1396. doi: 10.1037/a0031808
Frazier, P., Gabriel, A., Merians, A., and Lust, K. (2019). Understanding stress as an impediment to academic performance. J. Am. Coll. Heal. 67, 562–570. doi: 10.1080/07448481.2018.1499649
Gliem, J. A., and Gliem, R. R. (2003). Calculating, interpreting, and reporting Cronbach’s alpha reliability coefficient for Likert-type scales. 2003 Midwest Research to Practice Conference in Adult, Continuing, and Community Education, Columbus.
Green, H., Fernandez, R., and MacPhail, C. (2021). The social determinants of health and health outcomes among adults during the COVID-19 pandemic: A systematic review. Public Health Nurs. 38, 942–952. doi: 10.1111/phn.12959
Gresham, B., and Karatekin, C. (2022). The role of adverse childhood experiences (ACEs) in predicting academic problems among college students. Child Abuse Negl. 142:105595. doi: 10.1016/j.chiabu.2022.105595
Hatch, S. L., and Dohrenwend, B. P. (2007). Distribution of traumatic and other stressful life events by race/ethnicity, gender, SES and age: a review of the research. Am. J. Community Psychol. 40, 313–332. doi: 10.1007/s10464-007-9134-z
Hoyt, L. T., Cohen, A. K., Dull, B., Maker Castro, E., and Yazdani, N. (2021). “Constant stress has become the new normal”: stress and anxiety inequalities among U.S. college students in the time of COVID-19. J. Adolesc. Health 68, 270–276. doi: 10.1016/j.jadohealth.2020.10.030
Jenkins, S. R., Belanger, A., Connally, M. L., Boals, A., and Durón, K. M. (2013). First-generation undergraduate students’ social support, depression, and life satisfaction. J. Coll. Couns. 16, 129–142. doi: 10.1002/j.2161-1882.2013.00032.x
Junge, M., Li, S., Samaranayake, S., and Zalesak, M. (2022). Safe reopening of university campuses is possible with COVID-19 vaccination. PLoS One 17:e0270106. doi: 10.1371/journal.pone.0270106
Karatekin, C., and Hill, M. (2019). Expanding the original definition of adverse childhood experiences (ACEs). J. Child Adolesc. Trauma 12, 289–306. doi: 10.1007/s40653-018-0237-5
Miller, S., Wherry, L. R., and Mazumder, B. (2021). Estimated mortality increases during the COVID-19 pandemic by socioeconomic status, race, and ethnicity. Health Aff. 40, 1252–1260. doi: 10.1377/hlthaff.2021.00414
Musca, S., Kamiejski, R., Nugier, A., Méot, A., Er-rafiy, A., and Brauer, M. (2011). Data with hierarchical structure: impact of intraclass correlation and sample size on type-I error. Front. Psychol. 2:74. doi: 10.3389/fpsyg.2011.00074
Olneck-Brown, B. (2021). Public education’s response to the coronavirus (COVID-19) pandemic. National Conference of State Legislatures.
Pan, J., Zaff, J. F., and Porche, M. (2020). Social support, childhood adversities, and academic outcomes: a latent class analysis. J. Educ. Stud. Placed Risk 25, 251–271. doi: 10.1080/10824669.2019.1708744
Peralta, Y., Kohli, N., and Wang, C. (2018). A primer on distributional assumptions and model linearity in repeated measures data analysis. Quant. Methods Psychol. 14, 199–217. doi: 10.20982/tqmp.14.3.p199
Perry, B. L., Aronson, B., and Pescosolido, B. A. (2021). Pandemic precarity: COVID-19 is exposing and exacerbating inequalities in the American heartland. Proc. Natl. Acad. Sci. 118:e2020685118. doi: 10.1073/pnas.2020685118
Prawitz, A., Garman, E. T., Sorhaindo, B., O’Neill, B., Kim, J., and Drentea, P. (2006). InCharge financial distress/financial well-being scale: development, administration, and score interpretation. J. Financ. Couns. Plan. 17, 34–50. doi: 10.1037/t60365-000
R Core Team (2021). R: a language and environment for statistical computing. Available at: https://www.r-project.org
R Core Team (2023). R: A language and environment for statistical computing [Computer software]. Available at: https://www.r-project.org
Richardson, M., Abraham, C., and Bond, R. (2012). Psychological correlates of university students’ academic performance: a systematic review and meta-analysis. Psychol. Bull. 138, 353–387. doi: 10.1037/a0026838
Rodríguez-Planas, N. (2022). COVID-19, college academic performance, and the flexible grading policy: a longitudinal analysis. J. Public Econ. 207:104606. doi: 10.1016/j.jpubeco.2022.104606
Sato, H., and Kawahara, J. (2011). Selective bias in retrospective self-reports of negative mood states. Anxiety Stress Coping 24, 359–367. doi: 10.1080/10615806.2010.543132
Solar, O., and Irwin, A. (2010). A conceptual framework for action on the social determinants of health. Social determinants of health discussion paper 2 (Policy and Practice). Geneva: WHO.
Song, M.-K., Lin, F.-C., Ward, S. E., and Fine, J. P. (2013). Composite variables: When and how. Nurs. Res. 62:45.
Struthers, C. W., Perry, R. P., and Menec, V. H. (2000). An examination of the relationship among academic stress, coping, motivation, and performance in college. Res. High. Educ. 41, 581–592. doi: 10.1023/A:1007094931292
Vizoso, C., Rodríguez, C., and Arias-Gundín, O. (2018). Coping, academic engagement and performance in university students. High. Educ. Res. Dev. 37, 1515–1529. doi: 10.1080/07294360.2018.1504006
Keywords: social determinants of health, inequity, education, college, achievement
Citation: Gresham B, Corcoran F and Karatekin C (2023) Trajectories of academic performance over time: differences by social determinants of health and the COVID-19 pandemic. Front. Educ. 8:1252899. doi: 10.3389/feduc.2023.1252899
Edited by:
David Rodriguez-Gomez, Universitat Autònoma de Barcelona, SpainReviewed by:
Yan Liu, Carleton University, CanadaLaura Chamba Rueda, Universidad Técnica Particular de Loja, Ecuador
Copyright © 2023 Gresham, Corcoran and Karatekin. This is an open-access article distributed under the terms of the Creative Commons Attribution License (CC BY). The use, distribution or reproduction in other forums is permitted, provided the original author(s) and the copyright owner(s) are credited and that the original publication in this journal is cited, in accordance with accepted academic practice. No use, distribution or reproduction is permitted which does not comply with these terms.
*Correspondence: Bria Gresham, gresh027@umn.edu