- 1Faculty of Educational Sciences, University of Helsinki, Helsinki, Finland
- 2School of Engineering, Aalto University, Espoo, Finland
- 3Optentia Research Focus Area, North-West University, Vanderbijlpark, South Africa
The purpose of this study was to analyze knowledge co-construction as a self-organization process and the role of technology as its catalyst. Novel AI-directed speech recognition technology and the artifacts it generates were deployed to scaffold the knowledge co-construction process in two groups of pre-service teachers in a science education context. Throughout the lesson, the focus of the learning tasks was on pedagogical content knowledge and students' preconceptions. Analysis was conducted through the key characteristics of the social system's self-organization theory. The process of self-organization refers to the system's capacity to diverge from familiar structures, perspectives, and operations. Through the lenses of system theories, the active role of artifacts in co-construction was grasped and the role of technology in the self-organization of knowledge was analyzed. The pedagogical design of knowledge co-construction followed the principles of student-engaging learning. The technology used in co-construction was novel speech recognition AI software, which produced visual and editable word cloud artifacts from oral discussions on the large-format screen to edit. The data included videos and audio recordings. In this qualitative study, a content analysis and interaction analysis were used with descriptive analysis. The results showed that when technology became visible, as an active component of the system, artifacts triggered key signs of the social system's self-organization in co-construction. Exchange of information, “entropy levels,” were rapidly increased, and different viewpoints were expressed. Also, “chaos zones,” far-from-equilibrium states, were reached in both groups. Editable artifacts on the screen represented bifurcation spaces where groups' discussions were crystallized for the first time. Information was further categorized and evaluated through artifacts and this demonstrated how the groups processed communication into learning insights. Based on the results, the role played by this kind of technology was significant in the self-organization of knowledge. Materialized artifacts pushed the groups from small group conversation phases, comfort zones, toward uncertainty and confusion, which are central in self-organization. Technology in the system is seen not only as an interactor but also as an active agent that can facilitate epistemic emotions and support the group in the self-organization of knowledge.
1. Introduction
In this study, the social system's self-organization theory approach (Ståhle, 1998) is adopted to analyze knowledge co-construction processes where technology is seen as one central component of the system. Through the lenses of system theories, it is possible to grasp the active role of artifacts in co-construction and analyze the role played by AI-directed speech recognition technology in the self-organization of knowledge. The system theory approach has not been widely used for analyzing co-construction processes. Group agency is widely studied (Dillenbourg et al., 2009; List and Pettit, 2011; Stenalt, 2021; Brod et al., 2023) but there are only a few empirical studies on group self-organizing processes of knowledge in the context of educational technologies (Scheel et al., 2022). Sawyer (2006, 2009) has connected a systemic approach to collaboration and formed the concept of collaborative emergence. Sawyer introduces the phenomena, studied by social scientists, that emerge from “complex systems of individuals in interaction.” Ritella and Hakkarainen (2012) see technology-mediated learning practices as a similar phenomenon, a distributed system involving inter-psychological (social) and intra-psychological (individual) levels, materially embodied artifacts, and different perceptions of time.
Productive collaborative processes share similarities with social systems' self-organization processes of knowledge, which can be also equated with innovative learning and the emergence of new knowledge (Ståhle et al., 2020). If the indications of self-organization exist when a group of learners constructs knowledge, the process includes affordances to support higher-level learning and deeper cognitive processes (Bloom et al., 1956), which are also the aims of novel pedagogical approaches and 21st-century competencies. The challenge in existing pedagogical practices is still to generate higher-order cognitive processes, even though novel curriculums' content in recent years has shifted learning objectives from the idea of information transfer to higher-level learning (Härkki et al., 2021) such as the skills to analyze and solve problems and apply complex ideas (Haataja et al., 2023). The demands and aims of 21st-century education also challenge the pedagogical practices of using digital tools (Schleicher, 2018; Sanina et al., 2020).
The importance of collaboratively developed artifacts is highlighted in idea development and productive collaboration (Hennessy and Murphy, 1999; Barron, 2003; Paavola and Hakkarainen, 2009; Kangas et al., 2013). Also, in more recent learning research, Sawyer (2022) highlights the role of material tools in learning and collaborative creativity. He points out that artifact agency is a “positive force that drives the creative process forward.” Jointly constructed artifacts have an agency that supports creativity.
In this study, a system refers to an entity composed of a student group, technology, artifacts produced by students and technology, and co-construction activities. In systems where knowledge is created by self-organization, the system must undergo iterative processes that involve feedback loops. Through iterative interactions between its components, a system is driven to a state of instability through which unexpected paths are opened for its development. These paths can format new structures, new knowledge, and higher-level learning outcomes. In the context of social systems, “entropy” is considered a key characteristic in generating iteration. The concept of entropy, used in this study (based on Ståhle, 1998) refers to the rich exchange of information, as well as increased disorder and randomness in a system. Self-organized systems must have the ability to produce and increase entropy: to discuss the topic from different points of view and elaborate on the information exchanged. An increase in entropy leads the system from its balance, “equilibrium,” toward a far-from-equilibrium state in which confusion and uncertainty are necessary elements for knowledge to be analyzed, evaluated, and finally crystallized. When a system can operate with increased entropy in a far-from-equilibrium state, classifying and reflecting on information and tolerating challenges, the system has the opportunity to evolve and innovate a new order.
The self-organizing process of knowledge differs, for example, from a linearly scripted learning process, where the learning path is defined as precisely as possible. In a system's behavior, there must be room for uncertainty which creates space and opportunities for innovation through self-organization of knowledge. A self-organizing system has the freedom to act on and influence its decisions. Small changes can lead to significant shifts in the system's patterns and structures through bifurcation moments. The new order emerges suddenly from the system itself. Bifurcation moments in a broader sense can lead to significant shifts in learners' understanding and knowledge structures.
This study aims to analyze the knowledge co-construction process and the role of technology in the self-organization of knowledge. The research interest lies especially in technology as one active component of the system in co-construction, besides the group of learners. By taking a social system theory approach to the analysis of knowledge co-construction processes, scaffolded by AI-directed speech recognition technology, patterns of the key components that enable a group to self-organize their learning together are delineated.
From this context, the following research question arises:
“What is the role of AI-directed speech recognition technology in the co-construction process in terms of self-organization of knowledge?”
In the following sections, the theoretical background is initially clarified. The main concepts of social self-organizing systems are revisited and our conceptual and analytical approach, reflecting learning theories, is framed. The research setting is then introduced, covering the technology-mediated co-construction of preservice teachers in science education and an empirical analysis of the case study. Finally, in the discussion, the focus is on evaluating the theoretical insights with empirical data aiming to identify the key components that enable a group to self-organize in knowledge co-construction.
2. Theoretical background
2.1. What is a system?
In systems thinking, the concept of a system refers to “a holistic entity composed of and dependent on a series of interconnected and interacting parts” (Ståhle et al., 2020, p. 191). Salazar (2002) defines a system in social sciences as a collection of mutually dependent elements that form a cohesive entity. A group can be construed as a system, given that it is comprised of mutually dependent elements, which may be conceptualized in various forms (such as group members, behaviors, and interactions) that contribute to a unified whole.
Systems have been studied from a variety of perspectives. Ståhle (1998, 2008) has distinguished three different research generations of systems paradigms, the first of which focused on systemic order and its predictability, the second on systems openness and steadiness, and the third on self-organizing systems. The systems thinking trend from the 1960s shifted research toward the unpredictable dynamics of systems, disorder, and the relationship between chaotic behavior and the emergence of order. These new viewpoints led to a new research approach, known as complexity theory (CT) or complex adaptive systems (CAS) theory (Ståhle, 2008). The field of complexity science refers to a growing body of research on dynamic non-linear feedback systems and self-organizing systems. Not only individuals but interconnected and interacting parts form a dynamic system whose ability to self-organize requires a chaotic state. In general, complexity sciences investigate the zone between order and chaos, where a system transitions to exhibiting a significant degree of dynamism, represented by an increased variety in the behavior of its constituent elements. The term “complexity” refers to the substantial interdependence among a system's elements, as well as a high degree of variety in their respective behaviors (Salazar, 2002).
2.2. Concept of self-organization
The process of self-organization refers to the ability of a system to move away from familiar structures, perspectives, and operations. The concept refers to the capacity of a system to create its own structure (mentally, socially, and physically) and to set rules that facilitate collaborative behavior without requiring external top-down control (Mitchell, 2009). Self-organization in a system may be said to occur when a system seemingly spontaneously develops new structural features and a new order after having progressed through a disruption. The disruption causes a kind of “crisis” in the system which moves it away from equilibrium, from its stable state. This movement is characterized by a display of a greater variety of behaviors than was the case when the system was functioning at, or close to, its equilibrium point (Salazar, 2002).
Prigogine's research can be said to be the most important contribution to the self-organization and dynamic systems paradigm. Prigogine, a famous chemist, pointed out in his theory of dissipative structures that physical or chemical systems appear to develop order out of chaos. Prigogine discovered new laws of nature that could connect the natural sciences to the human sciences, and he maintained that these laws are universal, and thus also applicable to social systems (Prigogine, 1976, p. 120–126; Ståhle et al., 2020).
According to Prigogine (1980), to fully grasp the concept of self-organization in social systems, one must understand the critical transitional changes between order and chaos, stability and confusion. In stable states, the system works like it used to work. However, this state of “equilibrium” also means that there is little room for new or unexpected developments. The system needs to be pushed to the “chaos zone” to achieve new developments and results. If the system always operates in a stable equilibrium, through its familiar practices, innovative developments do not emerge. Characteristics that promote stability inhibit creativity because they only allow group members to do things that are guided by the same frames of mental models as usual (Salazar, 2002). The space between stability and instability—the edge of chaos—is where higher-level learning and creativity take place.
Ståhle (1998) analysis of Prigogine's research is used in this study. Key characteristics (i.e., requirements) for all self-organizing systems: entropy, state of far-from-equilibrium, and momentums of bifurcation are extracted from Prigogine's work by Ståhle. The focus of this study is the self-organization of knowledge within a group.
Next, the process and requirements of self-organization in social systems are clarified.
Entropy, a fundamental concept in thermodynamics, also plays a crucial role in the self-organization process. In the context of social systems, it refers to the information that a system produces to generate iteration but also increased disorder and randomness in itself. The systemic basis of self-organization is interaction, a rich exchange of information among its components. The more that the system exchanges information, i.e., communicates, the more the level of entropy increases. However, there are certain requirements. First, the communication dynamics in the system must be non-linear, and second, it must include both positive and negative responses. Both are needed for fruitful discrepancies and confusion to emerge. Positive feedback alone creates unanimity and negative feedback alone prevents continuity (Ståhle, 1998). When communication increases and the dynamics of communication include positive and negative forms, the process is called iterative: a cyclic feedback process that is continuous and sensitive, which allows the information produced by a system to be quickly transmitted throughout the whole system. Increased entropy also means information that cannot be utilized. Contrary to earlier beliefs, Prigogine saw high entropy levels not as a waste, but instead as a necessary component of self-organization. According to Ståhle (1998), Prigogine argued that a self-organizing system always produces waste and abundant information. Paradoxically, this uselessness is also necessary for a system's evolution.
In Prigogine's theory, increasing entropy levels are also associated with information chaos and disorganized, unclassified, or unappreciated knowledge. Entropy also brings uncertainty, imbalance, and confusion into the system through increased communication and different perspectives. It is important to note that high entropy means greater disorder, wasted resources, lost information, and uncertainty in the system. For a social system, this means abundant communication and production of ideas, and different angles of information without any certainty as to whether they will prove useful. Entropy is key in the self-organization process. Without increased entropy levels, the system (group) stays in a stable state (equilibrium) where new developments can only be small steps without any radical transformations.
To sum up, the entropy level of the system is based on its iterative quality, i.e., the frequency of information exchanged and the number of different viewpoints, as well as the balance between positive and negative feedback and equal participation (power balance) of the system components.
2.3. From comfort equilibrium to chaotic far-from-equilibrium
As described above, high entropy levels are necessary for a social system to move from its stable state, equilibrium, toward self-organization. When the system moves from its comfort zone to “far-from-equilibrium”, it handles increasing entropy levels, uncertainty, and confusion. If the system can handle the disharmony and confusion of increased entropy levels, it has a chance to produce order out of chaos; thus, it is crucial to avoid making interpretations and crystallizing information too early in the system, as this can hinder the system's ability to reach the needed “chaos zone.” Far-from-equilibrium might lead to the creation of something genuinely new—a new order, new knowledge, out of chaos.
2.4. Bifurcation: a zone between determinism and free choice
The new order in a system's self-organization includes momentums of bifurcation. “In principle, a bifurcation is simply the appearance of a new solution” (Prigogine, 1980, p. 105). Bifurcation always produces a change that is not a logical continuation of the previous structure (Prigogine, 1980, p. 105), and thus bifurcation as an event is always also a source of innovation (Prigogine and Nicolis, 1989, p. 74). The change of the system to the new equilibrium state happens suddenly. At the point of bifurcation, the system rejects a large amount of information, causing the amount of entropy to decrease and a new order to emerge. Bifurcation requires chaos or a state of far-from-equilibrium, as stated by Prigogine (1980, p. 105), Prigogine and Stengers (1984, p. 169), Prigogine and Nicolis (1989, p. 74), and Ståhle (1998).
According to Ståhle (1998) analyses of Prigogine, bifurcation refers to a phenomenon characterized by irreversible changes. As noted by Prigogine and Nicolis (1989), bifurcation is a catalyst for innovation and diversification, leading to the emergence of new solutions in the system. This transition occurs abruptly, akin to a sudden leap, which the term “crystallization” accurately portrays. At the bifurcation moment, the system relinquishes a significant amount of information, resulting in a decrease in entropy and the creation of a new order.
Bifurcation moments are critical in comprehending the irreversible changes that occur in self-organization. These systems are pushed beyond their initial equilibrium states through fluctuations, leading them to the bifurcation moments where multiple new options can be established. At this point, the system must choose between the available alternatives, and upon passing through the bifurcation moment, the system assumes a new configuration with new properties and structures.
In this study, our conceptual and analytical approach to the social system's self-organization can be seen to bring forth interesting emphases to modern learning theories. Following this, the related learning research context is presented, and concepts from our analytical frame are interconnected.
2.5. Reflection on the learning research context
In this study, the knowledge co-construction process is seen to include affordances for the group to self-organize and support higher-level learning, such as critical thinking, problem-solving, creativity, and reflection, and a more comprehensive understanding of the subject matter. The social system's self-organization is an iterative process with the unpredictable resonance between its components. Similarly, in socio-constructivism, learning is an ongoing, iterative process built from unscripted dialogue, interaction, the agency of participants, and artifacts (Lonka, 2015; Lonka et al., 2018). Learning goes beyond individual cognitive processes and also includes distributed, group, and social aspects (Hontvedt et al., 2023). Learners engage in cycles of inquiry, reflection, and action during the construction process, accommodating existing knowledge structures and refining their understanding. Material facets and the role of artifacts in collaborative processes are emphasized (Papert and Harel, 1991; Paavola and Hakkarainen, 2005; Stahl, 2006; Stahl and Hakkarainen, 2020).
How is this kind of iterative co-construction process supported in practice? Lonka and Ahola (1995) summarized three general principles for student-activating teaching and learning methods in higher education: first, starting with activating, and diagnosing the previous knowledge and understanding of the participants (e.g., brainstorming and generating ideas); second, supporting the learning process and making the learning processes overt to the discussion in various ways (e.g., guided discussions and editing shared artifacts); and third, providing both formative and summative assessments throughout the learning process (e.g., learning diaries, evaluation discussions, and further editions of artifacts). These three phases of learning processes are iterative and cyclical, where a longer learning cycle (such as an entire course) consists of shorter cycles of tutorials, lessons, or group discussions. Project-, inquiry-, and problem-based learning are examples of methods that activate students (Barron et al., 1998; Bereiter, 2002; Hmelo-Silver, 2004; Pedaste et al., 2015). In more ordinary settings, such as student-activating lectures, student-activating principles are also effective (e.g., McKeachie, 1999; Lonka and Ketonen, 2012). Lonka (2012) and Lonka et al. (2018) has presented a synthetic Engaging Learning Model, adding engagement and interest to the cyclic learning process described above: starting by catching interest and curiosity, then maintaining interest, and finally, deepening the interest of the students during the cycle (based on Hidi and Renninger, 2006). Activating students' ideas and potential misconceptions may also trigger confusion when previously held ideas are challenged. In all these phases, tutoring, and scaffolding of learning are important (e.g., Muukkonen et al., 2005).
In this study, the system-theoretic approach of self-organization and analytical tools is used to bring an understanding of how technology, as an active component of the system, adds value to the co-construction process. Entropy refers to the iterative information exchange that a system produces, including increased randomness and uncertainty in a system. In a learning context, this relates to the concept of surprise, which is one epistemic emotion. Epistemic emotions, which relate to knowledge and the generation of knowledge, are critical drivers of cognitive performance and engagement in learning (Vogl et al., 2020). As fundamental epistemic emotions, surprise, interest, confusion, and curiosity are linked with antecedents (e.g., cognitive dissonance) and outcomes (e.g., knowledge creation) and are therefore critically important for learning. Created confusion (imbalance) can turn toward discoveries, and curiosity promotes new levels of thinking. The findings of several studies imply surprise focuses attention, enhances memory, triggers interest and curiosity, and indirectly influences motivation. Sudden changes can push the system beyond its boundaries, sparking epistemic emotions (Renninger and Hidi, 2015; Noordewier et al., 2016; Vogl et al., 2020).
Increased entropy nudges a system from equilibrium toward chaos, a vital state for self-organization. Similarly, learning also requires tolerance of negative emotions such as confusion, boredom, and frustration, as they serve to stretch our understanding (Lonka et al., 2018). Rather than signs of failure, these challenging emotions are a natural part of the learning process. Specifically, confusion can stimulate constructive learning and deep understanding (Craig et al., 2004; D'Mello and Graesser, 2014). The benefits of confusion for learning depend on how it arises within tasks and how students manage it (Lehman et al., 2012; Lodge et al., 2018; Arguel et al., 2019). Effective confusion resolution is crucial for successful learning outcomes, as unresolved confusion can dampen interest in learning (D'Mello and Graesser, 2012). Furthermore, the learning environment should facilitate confusion management through timely feedback and align cognitive disequilibrium with task context for problem-solving (D'Mello and Graesser, 2014). However, it is important to note that individual differences significantly affect how students experience confusion in the learning process.
These kinds of entropy-driven dynamics, triggering bursts of epistemic emotions, can catalyze bifurcation moments, innovations, and new solutions in the system. In collaborative learning, bifurcation momentums in self-organization can be harnessed toward the concept of collaborative emergence (Sawyer and DeZutter, 2009), which refers to the emergence of a new product or creative outcome, a collective chance for something novel and appropriate to occur. Cognitive processes, distributed across participants and artifacts, contribute to collaborative emergence (Sawyer, 2006, 2009). Characterizing this unrestricted process is a free-flowing collaboration marked by equal participation and flexible actions. Additionally, spontaneous responses to changing situations empower the occurrence of something novel (Sawyer, 2006, 2009).
3. Materials and methods
3.1. Case study
3.1.1. Context and participants
The research context of this case study was a science teacher education course. The focus was on pedagogical content knowledge, especially how to include science education research knowledge of students' conceptual understanding while planning science instruction. The course was part of a 1-year program of pedagogical studies, required in Finland to achieve formal teacher qualification in addition to master-level studies in the teaching subject, e.g., mathematics or physics. The program was conducted in English. In the course, there were two small groups, with a total of eight participating student teachers of mathematics or science. Due to the international study program, the students' backgrounds were notably diverse, including their experience with educational technology. Their ages ranged from 20 to 57 years. Participants' backgrounds varied, ranging from a career-changer transitioning from information technology to teaching, to a 4th-year student with little experience beyond their university studies. All participants gave their written consent to the planned research.
Focus is placed on one lesson (90 min) in this study. The collaborative activities of the lesson aimed at supporting reflection on why it is important for a teacher to track students' preconceptions and what pedagogical aspects to take into consideration when planning science and engineering practices for physics lessons. Group 1 (G1) comprised one female and three male participants, and group 2 (G2), three female and one male participants. There was one physics major in each group. The whole group tested the software during an earlier lesson on the previous day, so the functionality of the software was not totally new to them.
3.1.2. AI-directed speech recognition technology for self-organization of knowledge
The core concept of the AI-directed speech recognition technology used in this case study was based on theories of collaborative learning and knowledge co-construction. The main idea is that participants can focus on the flow of conversation and produce “notes”, digital artifacts, while speaking, without the additional effort required by typing or writing.
In this research, technology is seen as an inseparable part of the system. Technology provides a novel means for collaboration by enabling the co-creation of digital artifacts (word clouds and collective notes) through spoken contributions. The artifacts produced by technology during co-construction activities are seen as active components of the system, as a part of a group of learners. The notion of artifacts has been used interchangeably in learning sciences (Damşa, 2014). Artifacts are instruments to mediate learners' actions in dialogue and problem-solving, as well as engaging them in knowledge construction (Säljo, 1999). “Externalized and materialized artifact” refers to instruments that promote the evolution of understanding and guide personal or collective inquiry further (Ritella and Hakkarainen, 2012). The role of material artifacts during the construction process has more recently shifted from artifacts solely as learning outcomes to artifacts as an active part of learning. Artifacts have the agency to create situations where ideas emerge from a process of interaction with them (artifacts). “The pedagogical message throughout is that the student should welcome the artifact's agency as a positive force that drives the creative process forward” (Sawyer, 2022).
In this study, the AI-directed speech recognition technology used combines new disruptive technologies: (1) speech recognition as a novel collective way to produce artifacts orally without typing; and (2) artificial intelligence to transform recorded oral thoughts into visual, materially embodied digital artifacts. Automatic Speech Recognition (ASR) is a computerized procedure that decodes and transcribes spoken language, typically converting it into written text. Many empirical investigations have provided substantial evidence to support the positive impact of ASR on language learning in particular (Jiang et al., 2023). Speech recognition is one of the most complex areas of computer science—involving linguistics, mathematics, and statistics. The vagaries of human speech have made its development challenging. Numerous factors can impact word error rates, such as pronunciation, accent, pitch, volume, and background noise. Reaching human parity—meaning an error rate on par with that of two humans speaking—has long been the goal of speech recognition systems.
The core characteristics of the software used in this study are as follows. It is (1) built to work in the English language. After recording an oral group discussion, (2) the software materializes transcription, based on group discussion as a digital, frequency-based word cloud. It is (3) possible to move each word in the cloud separately, (4) resize the words, and (5) add new words and phrases to the word cloud by typing on a keyboard.
3.1.3. Pedagogical planning and co-construction activities
The pedagogical planning of this 90-min lesson was framed to apply the Engaging Learning Model (ELM) (Lonka, 2012; Lonka et al., 2018) with a simplified short learning cycle:
1. Phase 1—to organize activities and set goals, share current understanding, capture interest, and generate ideas.
2. Phase 2—to facilitate inquiries through a shared artifact and engage participants in knowledge co-construction process.
3. Phase 3—to assess learning gains and engage the participants in deepening their interest and motivating future learning.
1. Phase 1: The aim of this phase was to start the co-construction process: that is, to activate previous understanding and to start brainstorming based on oral discussions. The setting was a small group learning discussion around the table. The large format touch device was not in use during this phase. The participants were able to write their own notes. Microphones were set up on the table and voice-driven AI software recorded the learning discussion in the background.
2. Phase 2: Co-construction activity during the second phase aimed at deepening the learning process. The group activity consisted of editing the digital word cloud and organizing the single artifacts from the cloud on a large format touch device. Phase 2 began when the digital word cloud, externalized from oral group discussions and transcriptions, became visible, making digital artifacts available for knowledge co-construction.
3. Phase 3: In Phase 3, the co-construction activity of the groups was to present and share their learning outcomes for evaluation. Edited word clouds were available and displayed on the large format touch device during the groups' presentations. Table 1 summarizes the co-construction phases, activities, and technology setup.
3.2. Data collection and analysis
The video allowed us to examine the role of technology in group processes in depth (Derry et al., 2010). The present study employed the following data from the larger data corpus (240 min in total). Field notes were also collected. First, the video data were scrutinized. The main data set consisted of a 90-min lesson (co-construction Phases 1, 2, and 3). The data were collected using a video camera focused on a group with a microphone placed on the table in two separate classrooms. The selected video data (150 min in total) formed an entity where technology was used throughout the entire co-construction process. The data consisted of videos from both small groups' co-construction Phases 1 and 2, and from Phase 3 when the whole group and a teacher were together in one classroom.
The analysis proceeded as follows. The selected video recordings (150 min) were transcribed verbatim. Next, the video and transcripts were analyzed. The video data and transcriptions were imported into the Atlas.ti software. The data processing and analysis using Atlas.ti was carried out by the first author. The focus of the analysis was identified by the involvement of co-authors.
Initially, the participants' verbal acts were analyzed using the code-and-count technique. Each participant's discussion turns in both groups was divided into speech episodes, constituting the unit of analysis (Linell, 1998, 2009). In this study, a speech episode is defined as a thematically meaningful unit of interactional exchange. A new episode begins when the discussion turns to the next participant. By applying the code and count technique and calculating frequencies of speech episodes in co-construction processes, initial analyses of the changes in entropy levels (amount of information exchanged) during three different co-construction phases in both groups were performed.
Next, the speech episodes were analyzed according to the analytical framework (Ståhle, 1998). Our focus in the analysis was directed toward the identification of the concepts of (1) entropy, (2) far-from-equilibrium, and (3) bifurcation momentums across the three phases of co-construction.
Following the counting of speech episodes, the concept of entropy was further analyzed using qualitative content analysis (Braun and Clarke, 2006; Strijbos et al., 2006). Quantified speech episodes were also analyzed qualitatively, to understand the content dimensions and entropy subsections of the speech episodes. The unit of analysis was a speech episode that could be thematically categorized to represent characteristics of entropy. Three key entropy dimensions of variation in speech episodes were identified. In this study, it meant that the speech episodes either: (1) reinforced the previous speech act, (2) brought a new insight to discussion, or (3) represented an opposite viewpoint. Category (4) “Other” was created for some of the speech episodes that were not relevant to any of these three categories. Subsection criteria of entropy, examples of the thematic analysis, and data are presented in Table 2.
In the third phase of analysis, units that captured a meaningful unity from the pre-service teachers' verbal and non-verbal actions, characterizing signs of the system's behavior in far-from-equilibrium state, were identified. In this study, this state was indicated by signs of confusion, frustration, or challenges manifested in the group members' verbal acts or behavior. Video-based interaction analysis (Jordan and Henderson, 1995) consists of the in-depth microanalysis of how people interact with one another, their physical environment, and the documents, artifacts, and technologies in that environment. The transcripts were segmented into topical episodes based on the substantive content of the speech and non-verbal behavior. Simultaneously occurring episodes were considered to be separate episodes. The talk and actions in these episodes were analyzed in terms of signs of confusion, frustration, or a sense of challenge. These signs were also systematically compared with entropy levels (number of speech episodes) and the existence and utilization of digital artifacts.
Finally, the analysis of speech episodes was continued through the concept of bifurcation in social systems' self-organization using qualitative content analysis. Bifurcation moments in this research are referred to as crystallized learning insights resulting from co-construction. The speech episodes from the co-construction Phase 3 were analyzed and compared to the edited word cloud artifacts.
The general focus was on the complex arrangement of verbal, visual, and material conduct through which the participants interacted during co-construction, including the role of the digital artifacts in the process (cf. Wohlwend, 2009; Theobald, 2012).
4. Results
4.1. Description of co-construction phases
The aim of co-construction Phase 1 was to begin the co-construction process with small group discussion: To activate previous understanding and to start discussing “How to take into consideration physics abstract situations when planning teaching, so that students can understand what the key aspects of the motion and force as a physics phenomenon are.” Technology was an “invisible” component in Phase 1. Speech-recognition software recorded the learning discussion in the background. Participants were able to write their own notes. Both groups' discussions were content related. All participants in both groups were able to contribute something new to the discussion, which followed the principle of alternation.
Phase 2 aimed at deepening the co-construction process. This phase began when both groups, in their own classrooms, saw the production of the recording, the word cloud artifact, for the first time. The aim was to produce a summary of pedagogical ideas for presentations by using the artifacts available. From a technical point of view, AI-directed speech recognition technology merged and transacted individuals' speech, and externalized spoken discussion using data mining functions based on the recorded group discussions. Technology formulated the digital word cloud from Phase 1 group discussions. Each individual's intangible shared thoughts were brought and merged. In Phase 2, technology became visible to the groups, so it also became one component of the system. Both groups went in front of the touch device and started to edit the artifacts while continuing their discussions (Figure 1). Selected artifacts from the word clouds in both groups represented the learning task at hand. The classified and organized artifacts represent content knowledge, and examples of these from group 1 are “Physics,” “Experiment,” and “Force,” and from group 2 are “Motion,” “Laws,” and “Preconceptions.”
In Phase 3, the groups presented and shared their learning insights. In this third phase of co-construction, the two groups came together with a teacher and presented their findings, one group at a time. During the presentations, all members from both groups had the opportunity to participate in sharing ideas and thoughts from the previous phases.
4.2. The role of AI-directed speech recognition technology on co-construction in terms of self-organization of knowledge
To answer the research question “What is the role of the AI-directed speech recognition technology in the co-construction process in terms of self-organization of knowledge?,” the findings in terms of entropy are first illuminated. In the initial analysis, each participant's interactional speech episodes during the three co-construction phases were quantified. This was done with the intention of interpreting variation in entropy levels across both groups. The code and count approach, as explained in the data analysis, was used to get a full view of the interactional turns, which indicates the entropy levels in social systems' self-organization processes. The results showed that participants' discussion turns, or “entropy levels,” increased rapidly in Phase 2, when the digital word cloud became visible for the groups to edit on the large format touch device. Tables 3, 4 show the frequencies of the discussion turns of every participant in groups 1 and 2 in co-construction Phases 1 and 2. The groups worked on these two phases in their own classrooms. Table 5 shows the frequency of the discussion turns of every participant and the teacher in co-construction Phase 3 when both groups and the teacher came together in the same classroom. Entropy production was highest in Phase 2, concerning every participant in both groups (ID01–ID04 in G1 and ID05–ID08 in G2). The frequency of speech episodes was notably higher compared to Phases 1 and 3. The increased discussion turns mean more exchange of information and interaction between participants: Group 1: Phase 1 (variation between) 8–12 turns, Phase 2: 19–29, and Phase 3: 4–12. Group 2: Phase 1: 7–9 turns, Phase 2: 18–34, and Phase 3: 7–12.
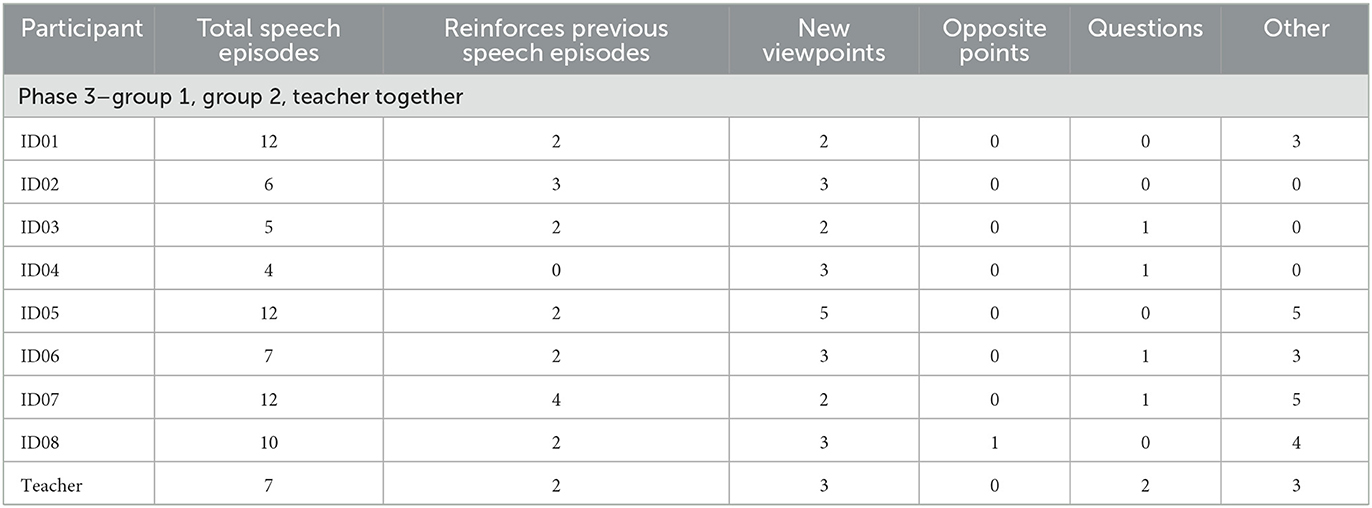
Table 5. Discussion turns and characteristics of speech episodes of group 1, group 2, and the teacher (everyone in the same classroom) in Phase 3.
A self-organizing system must have the ability to produce and accumulate entropy. Speech episodes of every participant in both groups were analyzed to determine how speech episodes were distributed among the group members and thus reveal the power balance of communication. The results demonstrate that every participant in each group contributed to the discussion evenly and no one dominated the discussions excessively. As presented earlier, the self-organization of the system requires the production and accumulation of entropy (the number of speech episodes), but it is also critical that all information is considered equal.
As noted above, producing and increasing entropy levels (the amount of communication) is one aspect of entropy, equally valued information is second, and the qualitative contents and meanings of speech episodes is third. Next, the content of the speech episodes was analyzed to understand the meanings and qualitative dimensions in terms of entropy in self-organization. It was shown by our results that speech episodes represented various dimensions of entropy: (1) reinforcement of previous speech episodes, (2) new viewpoints, and (3) opposite viewpoints. In the data, there were also many speech episodes that did not fit into any of these three categories. The (4) “Other” section includes speech episodes that do not represent dimensions of entropy. This section represents off-topic or random episodes. Tables 3, 4 show that in Phase 1, participants in both groups generated discussion by reinforcing previous speech episodes or presenting new viewpoints to the discussion. There was only one opposite viewpoint in each group in Phase 1. These results in Phase 1 reflect that co-construction activity is represented by a typical small group learning discussion where everyone shares their opinions on the topic at hand. Speech episodes in such activities might not contain that much dissonance: the focus is more on sharing one's own thoughts, one participant at a time. However, disharmony is needed for the system to be able to self-organize. In Phase 2, when the digital artifacts were available for groups to edit, there were more opposing viewpoints and also more new viewpoints from content knowledge that participants had brought to the discussion when compared to the results from Phase 1.
In summary, the results of the first analysis phase showed that both groups increased entropy levels by exchanging more information during Phase 2 when digital artifacts were visible and available to edit. The distribution of speech episodes among participants reflected equal participation. Speech episodes during this phase included key qualitative characteristics of entropy, not only reinforcing the previous opinions but also bringing opposed or new views of content knowledge into the process. Based on the results, the role of the artifacts—materialized digital word clouds—represented a new source of information, which increased entropy production and accumulation of entropy (amount of discussion turns), and also triggered new and opposing points into the discussion. Presence and working with the artifacts brought out the iterative nature of the co-construction process, which is explained next.
4.2.1. From equilibrium to far-from-equilibrium
As presented above, small group learning discussion represented a well-known and familiar educational practice where a group of learners share their thoughts. From the system's self-organizing perspective, during this activity, both groups were near equilibrium during Phase 1, based on earlier results regarding entropy levels and the qualitative content of speech episodes. Also, results from the interaction analysis showed that in Phase 1, there were no signs of confusion, uncertainty, or disorder which are indicators of a far-from-equilibrium state in our analysis.
Far-from-equilibrium is a central state in the system's self-organization. The far-from-equilibrium state opens the possibility to enter the “chaos zone”, which is imperative for the self-organization of knowledge. Far-from-equilibrium or chaos zone in social systems does not necessarily mean a real chaotic atmosphere, but there must be a redundancy of contradictory and opposed ideas, which are signs of uncertainty and confusion.
Selected examples of the data, which indicate a far-from-equilibrium state in the system's self-organization, are presented next. The results show that when Phase 2 began and digital word clouds became visible to the groups on the large format touch device for the first time, signs of surprise and confusion appeared. Excerpt 1 demonstrates the first signs of perplexity when group 1 saw the word cloud for the first time in their own classroom. Mistakes in speech recognition led to the appearance of unexpected and confounding words within the word cloud.
Based on the results of interaction analysis, the digital word cloud artifact played the key role and pushed the groups gently from the stable equilibrium state, which, based on the results, refers to the small group discussion in Phase1, to the far-from-equilibrium phase, which also evoked epistemic emotions among group members (Figure 2).
Signs of surprise, confusion, and frustration, which indicate a far-from-equilibrium state in this study, were the focus of our analysis. Laughing is one of the signs of raised epistemic emotions, representing surprise, and confusion. The occurrence of laughter implied that the situation held unexpected and therefore puzzling elements. The appearance of a word cloud revealed conversation points that the participants had not mentioned in the earlier discussion during Phase 1. Furthermore, laughter was also prompted by mistakes made by the technology. These errors relaxed the atmosphere and provided material to deepen the co-construction process.
Meanwhile, when group 2 saw their artifact for the first time in their classroom, they immediately went in front of the screen and started to work with the concepts of the word cloud. There were also signs of confusion among these participants (Excerpt 2) regarding the words in the word cloud and also challenges when they started to work with the cloud and move the single words on the screen.
The word cloud artifacts themselves included surprising and confusing elements. Wrong off-topic recognitions in the word clouds triggered expressions of epistemic emotions. Confusion and challenges are needed to move groups toward self-organization. The word clouds did not show the full sentences or the logical order of the discussion. The word cloud result was surprising, a mix of key concepts from participants' speech and also sound material from incorrect speech recognition by the technology. In normal open-ended conversation, vagaries of human speech and unexpected sounds occur, which were also captured by the recordings. The technology “took in” every sound from the other sound sources (such as sneezes, coughing, background noise, or talking over each other) and processed these other sounds as if they were speech. Speakers' accents can also cause occurrences that increased the error rate of speech recognition. Both types of wrong recognitions caused words that were not discussed to appear in the word clouds, which caused observable confusion when the editing of the word clouds continued. Excerpt 3 shows this while group 1 was editing the cloud.
Also in group 2, the group members were confused by the wrongly recognized words in the word cloud (Excerpt 4). They tried to find the concepts from the word cloud that they had been talking about during Phase 1.
Editing the word cloud created frustration among the group members. Group 2 was trying to change the size of the words to make them more visible. After changing the size of one word, the word cloud rearranged itself and mixed the already selected and arranged concepts into different places on the screen (Excerpt 5).
Despite these challenges, the groups did not give up on the task, and they continued to engage by discussing and shaping the word clouds to represent their thoughts regarding pedagogical aspects of physics teaching.
As stated above, in the process of self-organization, generating entropy (exchange of information), accumulating entropy (different viewpoints), confusion, and tolerance of challenges are crucial elements in reaching a far-from-equilibrium state. It is critical that the system should also be able to dissipate entropy to self-organize. In the context of social systems, this means that information needs to be analyzed and evaluated. The editing of the word cloud and discussions of the shared artifacts represents the analysis and evaluation of the information gained. In this study, technology, as one system component, scaffolded co-construction, and at the same time, the self-organization of knowledge by squeezing and visualizing group conversation into a word cloud format. The word cloud summarized parts of the exchanged information from Phase 1. With the visualized artifact, it was possible to grasp the thoughts of previous Phase 1 conversations, despite the wrong recognized words in the cloud, and dive more collectively into evaluating the topic at hand.
It is critical to avoid making interpretations and the final crystallization of information too early. If the system can handle the confused situation, a turmoil of chaos may produce order out of chaos. This makes it possible for new structures or innovations to arise. When information is analyzed, valued, or modeled, the entropy and chaos with uncertainty decrease. Working with digital artifacts led the groups to spend more time discussing the learning task at hand again. Crystallization of information was not done immediately after the Phase 1 conversations. In Phase 2, the co-construction process included a vivid process of classifying and organizing the information scaffolded by artifacts on the screens. Classifying of artifacts and discussions focused on building shared understanding and deepening perceptions, which started during Phase 1. From the systemic self-organizing view, when participants discussed, organized, and classified the artifacts into a summary of their pedagogical ideas, the atmosphere of confusion and uncertainty started to lift as the work with the clouds and discussions continued.
4.2.2. Momentums of bifurcation points
The phenomenon of self-organization in a social system includes characteristics of seemingly spontaneous emergence of new structural features and order following a period of disruption, “far-from-equilibrium”. As presented in the results above, in this study, digital word clouds were the key elements in leading and maintaining groups at a far-from-equilibrium state and also for keeping the systems and the process iterative. The word cloud artifacts also generated opposing viewpoints among the learners, which is essential for creating the conditions for bifurcations. As the iteration continues and the system operates long enough in this state by classifying information, the system can become increasingly receptive, and move closer to the bifurcation moments that can result in a new state, a new structure, or the crystallization of knowledge. Bifurcation is a catalyst for innovation and diversification, leading to the emergence of new solutions in the system.
A content analysis was conducted on the speech episodes from Phase 3 where both groups came together and presented their findings from the two earlier co-construction phases. First, in this study, the materialized word cloud itself can be seen to represent a significant turning point in the co-construction process, which can be referred to as a bifurcation point. The concepts in the clouds were based on groups' discussions so the clouds can be seen to be a crystallization of the exchanged information, which the technology made visible as one active component of the system. The technological artifact transformed the groups' monological flow of thought into collective views, visually merging consecutive speech turns from Phase 1 into a word cloud. The common visual output offered a turning point for the group's co-construction process. Misinterpretations in the clouds served as surprising elements and reflective mirrors that increased communication and confusion, thereby stimulating learners to collaboratively construct knowledge.
It may be too daring to talk about real bifurcation moments in this study, which in their wider meaning can be seen to lead to significant shifts in learners' understanding and knowledge structures in the context of the social systems. As a result, instead of using the concept of bifurcation moment, further editing the digital word cloud could be seen to represent a “bifurcation space” (Figure 3), where there are many different branches to work with knowledge and where groups are allowed to make free choices by picking up, removing, and adding the artifacts they like. The choices made in this state depend on the system and cannot be predicted in advance. These acts, every decision that the groups made regarding artifacts they selected from the word cloud, can be seen to crystallize information further and represent learning by insights, which are more likely to emerge in this kind of short co-construction cycle than bifurcation moments in their wider meaning. Interacting with and organizing digital artifacts, the system abandons a large amount of information and thus the amount of entropy decreases. It can be said that learning insights were reached by both groups during this moment of the process. Based on content analysis, bifurcation moments in this study represented collective and crystallized thoughts about the learning insights of the co-construction cycle. Excerpt 6 presents examples of learning insights from group 2 while they presented their thoughts in Phase 3.
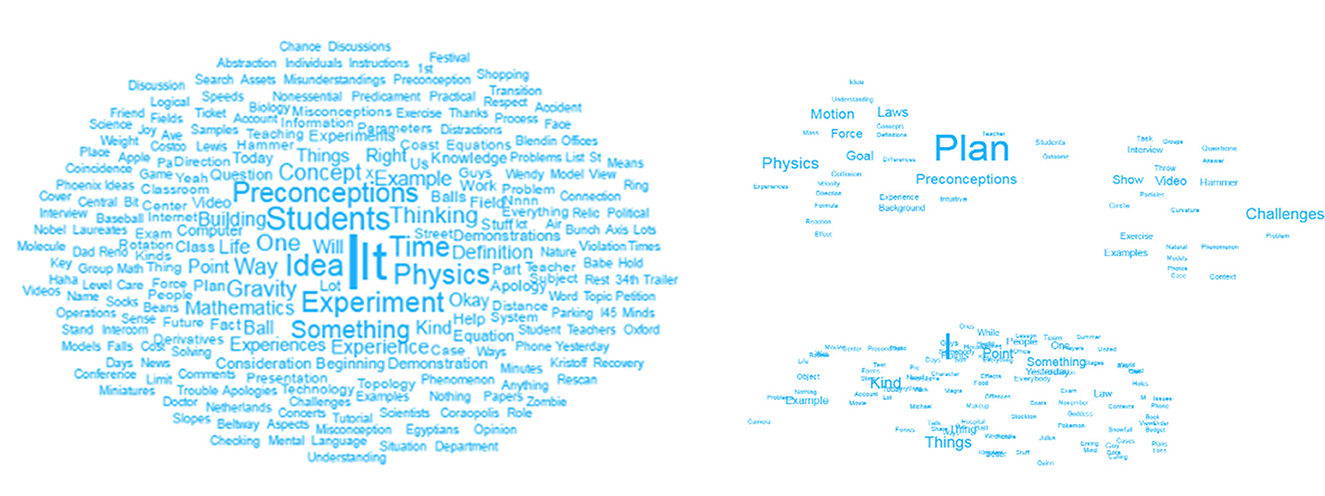
Figure 3. On the left, word cloud after Phase 1. On the right, edited word cloud after Phase 2. Both from group 2.
5. Discussion
The purpose of this study was to analyze knowledge co-construction as a self-organization process and the role of technology as its catalyst. Novel AI-directed speech recognition technology and the artifacts it generates were deployed to scaffold the knowledge co-construction process of pre-service teachers in two groups in a science education context. Analysis was conducted through key characteristics of the social system's self-organization based on Ståhle (1998). By taking a systems theory approach, a pattern was found that pointed out the key elements in enabling a group to use technology as a catalyst to self-organize in knowledge co-construction.
The self-organization of knowledge involves a sequence of iterative steps. First, the system must produce entropy, promoting a rich exchange of information and increased randomness. Next, an increase in entropy leads the system from its stableness, “equilibrium”, toward a far-from-equilibrium state, in which confusion and uncertainty are necessary elements for knowledge to be analyzed, evaluated, and finally crystallized. When a system can operate with increased entropy, classifying and reflecting on information and tolerating challenges, it has the opportunity to evolve and innovate a new order through bifurcation moments.
In this study, a system referred to an entity composed of a student group, technology, artifacts produced by students and technology, and co-construction activities. Based on the results of this study, the role of technological artifacts to enable signs of self-organization during knowledge co-construction was significant in both groups. First, the results showed that in every participant's discussion turns, the “entropy levels of the systems” increased rapidly in Phase 2, when technology became an active component of the system. The digital word cloud and artifacts became visible for the groups to edit on large-format screens. The number of speech episodes—entropy—increased in both groups. Also, the speech episodes included various qualitative forms of entropy, such as opposing views and new ideas. Visible and perplexing artifacts and engaging collaboration with them pushed the groups from stable small-group discussion in an equilibrium state (Phase 1) toward far-from-equilibrium (Phase 2). Without the digital word cloud artifacts, mandatory signs of self-organization (increasing entropy levels, different forms of entropy, and far-from-equilibrium states) were not found in either group through data analysis. The presence of technology and artifacts spawned more communication, and participation was distributed equally among the group members. Technology, as an inseparable part of the system, brought surprising and confusing elements to co-construction. It can be said that the technological artifacts destabilized a familiar educational structure, small-group discussion, and gave a new boost to the process, enabling observable signs of self-organization processes. The word clouds and artifacts can be seen to present a sort of bifurcation moment. The participants' earlier discussions were crystallized and materialized by technology. AI-directed technology offered bifurcation, a concrete and visible summary draft that scaffolded groups to construct knowledge and gain learning insights into the topic at hand. Working with the digital artifacts represented a bifurcation space where groups were able to follow different branches and co-constructed knowledge further.
The results of this study raise questions: how can new technologies be built and used to support novel kinds of co-construction processes and the emergence of beneficial epistemic emotions? What new practices then emerge? The prevailing assumption has often been that advanced educational technology should adjust the learning process in real-time to suit the learners' current knowledge and skills. This adjustment aims to prevent uncertainty or confusion. Simply put, common wisdom holds that confusion should be avoided during learning and rapidly resolved if and when it arises (D'Mello et al., 2014). But when it comes to the construction process, epistemic emotions such as surprise or confusion relate to learning itself and have an object focus on knowledge construction (Pekrun et al., 2017). Positive learning experiences should include enough challenges and even confusion (Vilhunen et al., 2023). If technology-mediated processes are scripted and built to keep learners “always on track,” predictability is maximized, and emergence is minimized. This might diminish the appearance of epistemic emotions, which in the co-construction process have the potential, based on our results, to lead the group toward deeper collaborative efforts and self-organization. As D'Mello et al. (2014) claim, confusion plays an important role during complex learning activities and, if appropriately regulated, it can cause learners to process the material more deeply to resolve their confusion. Learning environments need to challenge learners substantially to elicit critical thought and deep inquiry. To continue, Scardamalia and Bereiter (2014) state that scripted instruction stands in contrast to the emergent character of human action, creative thinking, and constructive work with ideas. To foster higher-level “emergent,” technologies can play a role as enablers, not only supporting productive interaction between people but also promoting engagement between people and ideas, which can lead to the emergence of innovations (Scardamalia and Bereiter, 2014).
Teachers play an important role in fostering learning and emotional scaffolding (Halonen et al., 2016; Vilhunen et al., 2023). The role of a modern teacher is also to plan and facilitate learners to move beyond their comfort zones, transitioning from stable equilibrium states toward dynamic far-from-equilibrium situations. In such situations, learners have opportunities and freedom to interact and develop innovations together. According to the findings of this study, if co-construction activities and the use of technology are creative and framed pedagogically meaningfully, learners acquire opportunities for deeper knowledge construction processes. Thus, as pedagogical experts, teachers can employ elements of surprise and confusion in relation to technology-mediated learning activities. Embracing mistakes, incorporating randomness, and confronting challenges are essential aspects of innovative knowledge co-construction. The three-phase, student-activating pedagogical framework (Lonka et al., 2018), also used in this study, could aid in the planning of technology-enhanced learning activities that may induce confusion, unexpected turns, or other challenges designed to evoke epistemic emotions.
In the current era of expanding artificial intelligence (AI), it is necessary for existing pedagogical structures to harness technologies more extensively to support self-organizational elements in the learning context. Instead of merely speeding up the tasks and giving ready-made answers, EdTech could be a catalyst for co-construction and offer surprising collective twists to evoke epistemic emotions that feed creativity, engagement, and higher-level learning elements as a natural part of non-linear pedagogy and collaborative learning. When technology scaffolds self-organizational processes and fosters the emergence of knowledge co-construction, teachers can focus more on crucial aspects of human learning that also contribute to a communal and resilient future such as facilitating students' social-emotional wellbeing, school engagement, and metacognitive skills.
The significance of this study emerges from a systemic approach where reflections regarding the role of technology in co-construction were illuminated by our results. It was demonstrated that technology, as an active component of the system, can catalyze co-construction. The analysis and results of this study underscore the need for systemic thinking in a Computer-Supported Collaborative Learning (CSCL) context. Emerging technologies and digital artifacts are more than just interactors, they are increasingly active agents within the system. Together with the group of learners, they can facilitate the whole system toward the self-organization of knowledge, thus creating a valuable space for innovative learning as knowledge creation.
The limitations of this research are acknowledged. It is recognized that the results cannot be generalized due to the case study nature of this research. Follow-up studies are necessitated in other learning contexts and on other learning elements, as well as the use of different educational technologies. Despite these limitations, this study creates interesting openings for applying the analytical tools of self-organization in technology-mediated co-construction.
Data availability statement
The datasets presented in this article are not readily available as the research rights are only applicable to this project. Requests to access the datasets should be directed to NH, niina.halonen@helsinki.fi.
Author contributions
NH: writer and owner of the manuscript, planning from the start of the research project, involvement in every phase, and has done most of the writing and analysis. PS: planning, writing, and commenting the manuscript. KJ: planning and involvement of the co-construction activities of the study and involvement in analysis. SP: writing and commenting the manuscript. KL: head of the whole research project, planning and commenting of the manuscript, and involvement in analysis. All authors contributed to the article and approved the submitted version.
Funding
NH received a personal research grant from the Jenny and Antti Wihuri Foundation. This work was also supported by the Digiconsumers Strategic Research Council (SRC) at the Academy of Finland (1352545).
Conflict of interest
The authors declare that the research was conducted in the absence of any commercial or financial relationships that could be construed as a potential conflict of interest.
Publisher's note
All claims expressed in this article are solely those of the authors and do not necessarily represent those of their affiliated organizations, or those of the publisher, the editors and the reviewers. Any product that may be evaluated in this article, or claim that may be made by its manufacturer, is not guaranteed or endorsed by the publisher.
References
Arguel, A., Lockyer, L., Kennedy, G., Lodge, J. M., and Pachman, M. (2019). Seeking optimal confusion: a review on epistemic emotion management in interactive digital learning environments. Inter. Lear. Environ. 27, 200–210. doi: 10.1080/10494820.2018.1457544
Barron, C. (2003). Problem-solving and EAP: themes and issues in a collaborative teaching venture. English Specific Purp. 22, 297–314. doi: 10.1016/S0889-4906(02)00016-9
Barron, O. E., Kim, O., Lim, S. C., and Stevens, D. E. (1998). Using analysts' forecasts to measure properties of analysts' information environment. Account. Rev. 73, 421–433.
Bereiter, C. (2002). Design research for sustained innovation. Cogn. Stud. 9, 321–327. doi: 10.11225/jcss.9.321
Bloom, B. S., Engelhart, M. D., Furst, E. J., Hill, W. H., and Krathwohl, D. R. (1956). Handbook I: Cognitive Domain. New York: David McKay.
Braun, V., and Clarke, V. (2006). Using thematic analysis in psychology. Qualit. Res. Psychol. 3, 77–101. doi: 10.1191/1478088706qp063oa
Brod, G., Kucirkova, N., Shepherd, J., Jolles, D., and Molenaar, I. (2023). Agency in educational technology: Interdisciplinary perspectives and implications for learning design. Educ. Psychol. Rev. 35, 25. doi: 10.1007/s10648-023-09749-x
Craig, S., Graesser, A., Sullins, J., and Gholson, B. (2004). Affect and learning: an exploratory look into the role of affect in learning with AutoTutor. J. Educ. Media 29, 241–250. doi: 10.1080/1358165042000283101
Damşa, C. I. (2014). The multi-layered nature of small-group learning: Productive interactions in object-oriented collaboration. Int. J. Comput. Supp. Collabor. Lear. 9, 247–281. doi: 10.1007/s11412-014-9193-8
Derry, S. J., Pea, R. D., Barron, B., Engle, R. A., Erickson, F., Goldman, R., et al. (2010). Conducting video research in the learning sciences: Guidance on selection, analysis, technology, and ethics. J. Learn. Sci. 19, 3–53. doi: 10.1080/10508400903452884
Dillenbourg, P., Järvelä, S., and Fischer, F. (2009). “The evolution of research on computer-supported collaborative learning,” in Technology-Enhanced Learning (Springer, Dordrecht) 3–19. doi: 10.1007/978-1-4020-9827-7_1
D'Mello, S., and Graesser, A. (2012). Dynamics of affective states during complex learning. Lear. Instr. 22, 145–157. doi: 10.1016/j.learninstruc.2011.10.001
D'Mello, S., and Graesser, A. (2014). Confusion and its dynamics during device comprehension with breakdown scenarios. Acta Psychol. 151, 106–116. doi: 10.1016/j.actpsy.2014.06.005
D'Mello, S., Lehman, B., Pekrun, R., and Graesser, A. (2014). Confusion can be beneficial for learning. Lear. Instr. 29, 153–170. doi: 10.1016/j.learninstruc.2012.05.003
Haataja, E. S., Tolvanen, A., Vilppu, H., Kallio, M., Peltonen, J., and Metsäpelto, R. L. (2023). Measuring higher-order cognitive skills with multiple choice questions–potentials and pitfalls of Finnish teacher education entrance. Teach. Teacher Educ. 122, 103943. doi: 10.1016/j.tate.2022.103943
Halonen, N., Hietajärvi, L., Lonka, K., and Salmela-Aro, K. (2016). Sixth graders' use of technologies in learning, technology attitudes and school well-being. Eur. J. Soc. Behav. Sci. 18, 2307–2324. doi: 10.15405/ejsbs.205
Härkki, T., Vartiainen, H., Seitamaa-Hakkarainen, P., and Hakkarainen, K. (2021). Co-teaching in non-linear projects: A contextualised model of co-teaching to support educational change. Teach. Teacher Educ. 97, 103188. doi: 10.1016/j.tate.2020.103188
Hennessy, S., and Murphy, P. (1999). The potential for collaborative problem solving in design and technology. Int. J. Technol. Design Educ. 9, 1–36. doi: 10.1023/A:1008855526312
Hidi, S., and Renninger, K. A. (2006). The four-phase model of interest development. Educ. Psychol. 41, 111–127. doi: 10.1207/s15326985ep4102_4
Hmelo-Silver, C. E. (2004). Problem-based learning: What and how do students learn? Educ. Psychol. Rev. 16, 235–266. doi: 10.1023/B:EDPR.0000034022.16470.f3
Hontvedt, M., Prøitz, T. S., and Silseth, K. (2023). Collaborative display of competence: A case study of process-oriented video-based assessment in schools. Teach. Teacher Educ. 121, 103948. doi: 10.1016/j.tate.2022.103948
Jiang, M. Y. C., Jong, M. S. Y., Lau, W. W. F., Chai, C. S., and Wu, N. (2023). Exploring the effects of automatic speech recognition technology on oral accuracy and fluency in a flipped classroom. J. Comput. Assist. Learn. 39, 125–140. doi: 10.1111/jcal.12732
Jordan, B., and Henderson, A. (1995). Interaction analysis: Foundations and practice. J. Lear. Sci. 4, 39–103. doi: 10.1207/s15327809jls0401_2
Kangas, K., Seitamaa-Hakkarainen, P., and Hakkarainen, K. (2013). Design thinking in elementary students' collaborative lamp designing process. J. Design Technol. Educ. 18, 30–43.
Lehman, B., D'Mello, S., and Graesser, A. (2012). Confusion and complex learning during interactions with computer learning environments. Internet. Higher Educ. 15, 184–194. doi: 10.1016/j.iheduc.2012.01.002
Linell, P. (1998). Approaching Dialogue: Talk, Interaction and Contexts in Dialogical Perspectives (Vol. 3). Amsterdam: John Benjamins Publishing. doi: 10.1075/impact.3
Linell, P. (2009). Rethinking Language, Mind, and World Dialogically. New York, NY: Information Age Publishing.
List, C., and Pettit, P. (2011). Group Agency: The Possibility, Design, and Status of Corporate Agents. Oxford: Oxford University Press. doi: 10.1093/acprof:oso/9780199591565.001.0001
Lodge, J. M., Kennedy, G., Lockyer, L., Arguel, A., and Pachman, M. (2018). “Understanding difficulties and resulting confusion in learning: An integrative review,” in Frontiers in Education (Frontiers Media SA) 49. doi: 10.3389/feduc.2018.00049
Lonka, K. (2012). “Engaging Learning Environments for the Future: The 2012 Elizabeth W. Stone Lecture,” in The road to information literacy: Librarians as facilitators of learning: IFLA Publications Series 157, eds. R., Gwyer, R., Stubbings, G., Walton (Berlin/Munich: De Gruyter Saur) 15–30. doi: 10.1515/9783110281002.15
Lonka, K., and Ahola, K. (1995). Activating instruction: How to foster study and thinking skills in higher education. Eur. J. Psychol. Educ. 10, 351–368. doi: 10.1007/BF03172926
Lonka, K., and Ketonen, E. (2012). How to make a lecture course an engaging learning experience? Stud. Learn. Soc. 2, 63–74. doi: 10.2478/v10240-012-0006-1
Lonka, K., Makkonen, J., Berg, M., Talvio, M., Maksniemi, E., Kruskopf, M., et al. (2018). Phenomenal learning from Finland. Helsinki: Edita.
McKeachie, W. J. (1999). “Teaching, learning, and thinking about teaching and learning,” in Higher Education: Handbook of Theory and Research (Dordrecht: Springer Netherlands) 1–38. doi: 10.1007/978-94-011-3955-7_1
Muukkonen, H., Lakkala, M., and Hakkarainen, K. (2005). Technology-mediation and tutoring: How do they shape progressive inquiry discourse? J. Learn. Sci. 14, 527–565. doi: 10.1207/s15327809jls1404_3
Noordewier, M. K., Topolinski, S., and Van Dijk, E. (2016). The temporal dynamics of surprise. Soc. Person. Psychol. Compass 10, 136–149. doi: 10.1111/spc3.12242
Paavola, S., and Hakkarainen, K. (2005). The knowledge creation metaphor–An emergent epistemological approach to learning. Sci. Educ. 14, 535–557. doi: 10.1007/s11191-004-5157-0
Paavola, S., and Hakkarainen, K. (2009). “From meaning making to joint construction of knowledge practices and artefacts–A trialogical approach to CSCL,” in Computer Support Collaborative Learning Practices: CSCL2009 Conference Proceedings.
Pedaste, M., Mäeots, M., Siiman, L. A., De Jong, T., Van Riesen, S. A., Kamp, E. T., et al. (2015). Phases of inquiry-based learning: Definitions and the inquiry cycle. Educ. Res. Rev. 14, 47–61. doi: 10.1016/j.edurev.2015.02.003
Pekrun, R., Vogl, E., Muis, K. R., and Sinatra, G. M. (2017). Measuring emotions during epistemic activities: The epistemically-related emotion scales. Cogn. Emot. 31, 1268–1276. doi: 10.1080/02699931.2016.1204989
Prigogine, I. (1976). “Order through fluctuations: Self-organization and social systems,” in Evolution and consciousness: Human systems in transition, eds. E. Jantsch and C. H. Waddington (Boston: Addison-Wesley) 93–133.
Prigogine, I. (1980). From Being to Becoming: Time and Complexity in the Physical Sciences. San Francisco: W.H. Freeman.
Prigogine, I., and Stengers, I. (1984). Order Out of Chaos: Man' s Dialogue With Nature. New York: Bantam.
Renninger, K. A., and Hidi, S. (2015). The Power of Interest for Motivation and Engagement. London: Routledge. doi: 10.4324/9781315771045
Ritella, G., and Hakkarainen, K. (2012). Instrumental genesis in technology-mediated learning: From double stimulation to expansive knowledge practices. Int. J. Comput. Support. Collabor. Learn. 7, 239–258. doi: 10.1007/s11412-012-9144-1
Salazar, A. J. (2002). “Self-organizing and complexity perspectives of group creativity: Implications for group communication,” in New Directions in Group Communication, ed. L. W. Frey (London: Sage) 179–200. doi: 10.4135/9781412990042.n10
Sanina, A., Kutergina, E., and Balashov, A. (2020). The Co-Creative approach to digital simulation games in social science education. Comput. Educ. 149, 103813. doi: 10.1016/j.compedu.2020.103813
Sawyer, K. (2009). “Writing as a collaborative act,” in The psychology of creative writing 166–179. doi: 10.1017/CBO9780511627101.012
Sawyer, R. K. (2006). Educating for innovation. Think. Skills Creat. 1, 41–48. doi: 10.1016/j.tsc.2005.08.001
Sawyer, R. K. (2022). The dialogue of creativity: Teaching the creative process by animating student work as a collaborating creative agent. Cogn. Instr. 40, 459–487. doi: 10.1080/07370008.2021.1958219
Sawyer, R. K., and DeZutter, S. (2009). Distributed creativity: How collective creations emerge from collaboration. Psychol. Aesthet. Creat. Arts 3, 81. doi: 10.1037/a0013282
Scardamalia, M., and Bereiter, C. (2014). Smart technology for self-organizing processes. Smart Lear. Environ. 1, 1–13. doi: 10.1186/s40561-014-0001-8
Scheel, L., Vladova, G., and Ullrich, A. (2022). The influence of digital competences, self-organization, and independent learning abilities on students' acceptance of digital learning. Int. J. Educ. Technol. High. Educ. 19, 1–33. doi: 10.1186/s41239-022-00350-w
Ståhle, P. (1998). Supporting a system's capacity for self-renewal (Research Report 190). Department of Teacher Education, University of Helsinki.
Ståhle, P. (2008). “The dynamics of self-renewal: A systems-thinking to understanding organizational challenges in dynamic environments,” in Organisational Capital (London: Routledge) 133–160. doi: 10.4324/9780203885215.ch7
Ståhle, P., Mononen, L., Tynjälä, P., and Kallio, E. K. (2020). “Systems thinking and adult cognitive development,” in Development of Adult Thinking (London: Routledge) 191–207. doi: 10.4324/9781315187464-12
Stahl, G. (2006). Group Cognition: Computer Support for Building Collaborative Knowledge (Acting with Technology). New York, NY: The MIT Press. doi: 10.7551/mitpress/3372.001.0001
Stahl, G., and Hakkarainen, K. (2020). Theories of CSCL. International Handbook of Computer-Supported Collaborative Learning. New York, NY: Springer. doi: 10.1007/978-3-030-65291-3_2
Stenalt, M. H. (2021). Researching student agency in digital education as if the social aspects matter: students' experience of participatory dimensions of online peer assessment. Assess. Eval. Higher Educ. 46, 644–658. doi: 10.1080/02602938.2020.1798355
Strijbos, J. W., Martens, R. L., Prins, F. J., and Jochems, W. M. (2006). Content analysis: What are they talking about? Comput. Educ. 46, 29–48. doi: 10.1016/j.compedu.2005.04.002
Theobald, M. (2012). Video-stimulated accounts: Young children accounting for interactional matters in front of peers. J. Early Childhood Res. 10, 32–50. doi: 10.1177/1476718X11402445
Vilhunen, E., Chiu, M. H., Salmela-Aro, K., Lavonen, J., and Juuti, K. (2023). Epistemic emotions and observations are intertwined in scientific sensemaking: A study among upper secondary physics students. Int. J. Sci. Math. Educ. 21, 1545–1566. doi: 10.1007/s10763-022-10310-5
Vogl, E., Pekrun, R., Murayama, K., and Loderer, K. (2020). Surprised–curious–confused: Epistemic emotions and knowledge exploration. Emotion 20, 625. doi: 10.1037/emo0000578
Keywords: knowledge co-construction, self-organization of knowledge, systems theoretic approach, technology-enhanced learning, artificial intelligence, speech recognition, technology-mediated learning
Citation: Halonen N, Ståhle P, Juuti K, Paavola S and Lonka K (2023) Catalyst for co-construction: the role of AI-directed speech recognition technology in the self-organization of knowledge. Front. Educ. 8:1232423. doi: 10.3389/feduc.2023.1232423
Received: 31 May 2023; Accepted: 08 August 2023;
Published: 07 September 2023.
Edited by:
Wang-Kin Chiu, The Hong Kong Polytechnic University, ChinaReviewed by:
Maria Beatrice Ligorio, University of Bari Aldo Moro, ItalyMilan Kubiatko, J. E. Purkyne University, Czechia
Copyright © 2023 Halonen, Ståhle, Juuti, Paavola and Lonka. This is an open-access article distributed under the terms of the Creative Commons Attribution License (CC BY). The use, distribution or reproduction in other forums is permitted, provided the original author(s) and the copyright owner(s) are credited and that the original publication in this journal is cited, in accordance with accepted academic practice. No use, distribution or reproduction is permitted which does not comply with these terms.
*Correspondence: Niina Halonen, niina.halonen@helsinki.fi
†ORCID: Niina Halonen orcid.org/0000-0002-8353-7457