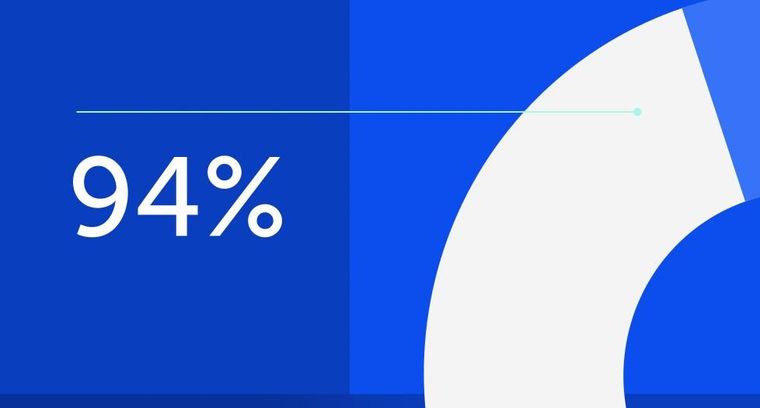
94% of researchers rate our articles as excellent or good
Learn more about the work of our research integrity team to safeguard the quality of each article we publish.
Find out more
REVIEW article
Front. Educ., 24 August 2023
Sec. STEM Education
Volume 8 - 2023 | https://doi.org/10.3389/feduc.2023.1226529
This article is part of the Research TopicExploring STEM Environments that Broaden ParticipationView all 15 articles
Citizen Science is an ever-growing field of public engagement with science, and recent years have seen an increasing number of studies examining its potential. This study reviews this research to determine the educational and scientific outcomes of Citizen Science. A literature search produced 1,240 articles that were subsequently coded according to their main focus. Articles fell into one of three main categories: (a) empirical scientific articles, (b) narrative project descriptions, and (c) theoretical and technical conceptualizations. Hundred and forty-eight studies investigated educational outcomes of participation in Citizen Science such as effects on interest in science or motivation. These studies were examined further to assess the achievement of educational outcomes of Citizen Science. In terms of changing epistemological beliefs, for example, Citizen Science seems to have little effect. Overall, there is currently a lack of empirical studies to assess the educational outcomes comprehensively. In contrast, many empirical scientific articles answered research questions in different scientific disciplines based on Citizen Science data and many studies confirmed a high level of Citizen Science data quality providing information on the scientific outcomes. Implications for future research on Citizen Science are discussed.
Recent years have seen an increasing number of Citizen Science (CS) projects designed to promote citizens’ participation in science. In CS, citizens contribute to clarifying current scientific issues through active participation in real scientific studies even though they are not institutionally involved in the field of research (Bonn et al., 2016; Haklay et al., 2020). In particular, the expansion of the internet and new technologies have made citizen involvement much easier and more extensive, because tools are designed to enable worldwide participation (Bonney et al., 2014). Although CS projects differ regarding the extent of citizen participation, their associated scientific disciplines, and the use of scientific methods (Bonney et al., 2009), a shared goal can be identified. This goal is to achieve two specific outcomes (Brossard et al., 2005): The first dimension represents educational outcomes and refers to citizens’ benefits through participating in CS. These outcomes include increased motivation and interest in science, trust in science, and expanded knowledge about the scientific inquiry or specific project contents. The second dimension represents scientific outcomes and refers to scientists and an added value for science. Participation in CS can help create large data sets contributing to the clarification of scientific research questions and advancing the scientific discourse. CS projects differ in the extent to which they address these two dimensions (Phillips et al., 2014; Bonney et al., 2016). Whereas some projects pursue more educational outcomes, others focus more on scientific ones. Meeting both dimensions in one CS project is preferable but particularly challenging, because they sometimes compete, e.g., due to the project design (Bonney et al., 2016; Roche et al., 2020). So far, despite an increasing number of research articles on CS, there is still no sufficient synthesis of the results that would allow a summary assessment of the degree to which these outcomes have been produced. The present review intends to contribute to clarifying this issue.
CS projects are special learning environments that correspond in many respects to the characteristics of informal learning settings (European Commission, 2016). However, there are many CS projects for school classes that qualify as non-formal education. Even though CS in schools occurs in a planned manner, it is much more flexible and adaptive than formal learning contexts and could contribute to authentic science education. Thus, whether and to what extent the educational outcomes are achieved is particularly interesting from an educational research perspective. Phillips et al. (2014) developed a framework for evaluating the effect of CS projects on the participants. In this framework, the outcomes likely to be influenced by participation in CS are divided into different categories. One of the outcomes of participation in CS is interest in science and the environment. According to Hidi and Renninger (2006), interest describes a specific relationship between a person and a learning object. Interest creates the basis for meaningful learning and enhances the autonomous acquisition of knowledge without external influences (Krapp, 1999). Especially in the institutional context, CS could counteract the declining interest in science to be found in many students over the path of their education (Potvin and Hasni, 2014). Further, Phillips et al. (2014) mention motivation to join scientific or environmental activities as a dependent variable resulting from participation in CS. With reference to Ryan and Deci (2000), motivation can be defined as an intentional action that directs behavior toward a future action outcome. Motivation is enhanced when the three basic needs of competence, autonomy, and social inclusion are satisfied in an authentic and interactive learning environment (Ryan and Deci, 2002). These three basic needs can be met extensively in CS projects, because participants are actively involved in the scientific process, contribute personally to the clarification of scientific research questions, and are part of a large community (Bonney et al., 2009). Other educational outcomes of CS might be changes in attitudes toward science that could be described as “cognitive and emotional opinions about various aspects of science” (Kind et al., 2007, p. 873). These are relatively stable mental constructs based on previous personal experiences (Sigel, 1985; Pajares, 1992). Because they are an essential aspect of scientific literacy, change in such attitudes toward science is an important aspect for evaluating the educational outcomes of CS. Roche et al. (2020) point out that “the development of scientific literacy in tandem with the contribution to genuine scientific outcomes has been a longstanding goal of the field” (p. 2). The second aim of CS is to produce scientific outcomes (Bonney et al., 2009). In the course of the change from the deficit model to the dialogue-between-science-and-society model (Stilgoe et al., 2014), it was recognized that not only science can have positive effects on society, but that there is also a reciprocal relationship between both sides. Individuals outside the scientific community can contribute to science and the clarification of scientific research questions by, for example, providing their own particular expert knowledge. Using data from individuals, socioscientific issues can be addressed more easily. Today, the extent of participation in the scientific process can vary (Bonn et al., 2016). Whereas some projects limit participation to classifying existing data, others involve citizens in collecting new data. Apart from collecting and analyzing data, citizens can also be involved in the publication process of the results. However, few projects actively involve citizens in all steps of an empirical study, i.e., from developing a research question, over planning and implementing a research design, all the way to analyzing and interpreting the data (Bonney et al., 2009; Shirk et al., 2012; Haklay, 2013).
As mentioned above, high expectations are placed on CS (Socientize, 2015). It is increasingly addressed from a variety of perspectives and “is establishing itself as a field of research” (Vohland et al., 2021, p. 2). The science of CS, considered as a collection of all scientific findings on CS (popularity/dissemination of CS, project forms, participants, data quality, ethical challenges, and more) is getting larger and more complex. At the same time, there is an increasing need for scientific evidence on the positive effects of CS practices; and the scientific community is required to respond to this development (Perelló et al., 2021). So far, there are a few reviews on the effects of CS on educational outcomes (e.g., Stepenuck and Green, 2015; Groulx et al., 2017; Schuttler et al., 2018; Peter et al., 2019; Aristeidou and Herodotou, 2020). However, the “existing literature reviews on the topic have had very specific thematic or methodological foci and, therefore, may have limited application for the wider field of citizen science” (When et al., 2021). To date, there is still no comprehensive review integrating all CS projects regardless of scientific discipline or project form. Further, most reviews focus on effects on behavior and knowledge (Stepenuck and Green, 2015; Groulx et al., 2017) and we know very little about motivational effects of CS. For example, in the studies reviewed by Peter et al. (2019), only one study on interest is considered and motivation is not examined at all as a potential outcome in the studies considered. Thus, one central aim is to provide a comprehensive review of the research on educational and scientific outcomes of CS across disciplines. Against the background of the intended outcomes of CS, we address the following research questions:
1. What conclusions can be drawn from previous studies regarding educational outcomes of CS in terms of interest, motivation and attitudes toward science?
2. What conclusions can be drawn from previous studies regarding scientific outcomes of CS?
To this end, we first need to identify studies on the outcomes of CS and synthesize those results that focus particularly on educational outcomes. To select relevant articles, the first step is to classify all studies on CS and to provide an appropriate structuring of the science of CS. This fine-grained portrayal of research in the field of CS serves to keep track of the increasing number of research articles. On the basis of this classification, we can select those studies that address which educational outcomes have been produced. We shall examine how many studies are available that report educational outcomes of participation in CS and whether these studies are sufficient to allow generalized statements (see 3.1). Because we quantify not only those studies that deal with educational outcomes of CS but also the remaining research articles, we shall also be able to make statements about scientific outcomes. This review will start by showing how many scientific publications answer their research question based on CS data. This number of studies provides first indications on scientific outcomes of CS (see 3.2).
Systematic literature reviews follow a guided and elaborated scientific procedure when searching for and analyzing literature, and they summarize the results concisely (Cooper, 2016). To gain a systematic overview of the literature on CS, we followed Cooper’s (2016) guidelines for research syntheses. We searched for the term “Citizen Science” within the titles of publications in English or German. At the beginning of May 2020, we searched the databases Web of Science and FIS Bildung for entries published up to and including April 2020. In addition, all articles of the journal Citizen Science: Theory and Practice (CSTP) were considered, as this journal is an important source for studies in the field of CS, but is not indexed in the databases. This search resulted in 1,495 hits (Web of Science: n = 1,315; FIS Bildung: n = 93; CSTP = 87). A first screening through exclusion criteria (e.g., removing duplicate articles and articles without an abstract available) left 1,240 research articles. We also excluded articles in which the participants themselves were the object under study. The understanding of CS underlying this article is not that participants are surveyed, which is a common social science research method, but that they conduct research themselves.
To provide a fine-grained portrayal of research in the field of CS and to retrieve studies related to educational and scientific outcomes, we developed a coding manual for our literature analysis. We developed the manual by following deductive and inductive processes: first, the intended outcomes of CS served as an initial orientation for sorting the studies (deductive process). Then, in an iterative process, a team of several social scientists grouped similar content areas of the articles (inductive process). The resulting coding manual differentiated between (a) empirical scientific articles, (b) narrative projects descriptions, and (c) theoretical and technical conceptualizations of CS (see Table 1). Within Category a, we first coded articles reporting educational outcomes of participating in CS projects (a1: educational outcomes). Further, we coded articles analyzing the quality of data gathered in CS projects (a2: data quality). Finally, we coded articles using CS data to answer scientific research questions in different disciplines (a3: data use). Within Category b, we coded descriptions of one to three CS projects (b1: ≤3 project descriptions) and descriptions of more than three CS projects (b2: >3 project descriptions). Within Category c, we coded theoretical representations of CS (c1: theory) and technical devices for use in CS projects (c2: technical devices). Research articles were sorted into these categories based on the information given in their abstracts containing, in most cases, between 150 and 300 words. Two hundred and twenty six articles were selected randomly by a random number generator to be subsequently coded for assessing interrater reliability (Cohen’s kappa = 0.78).
To gain insight into whether and to what extent CS produces educational outcomes, we examined the studies on educational outcomes separately and in detail. To identify the relevant research articles, we applied the following inclusion and exclusion criteria.
– Participation in a CS project: We included studies in which the respondents had already participated in a CS project. We excluded studies in which participants were asked, for example, about their interest in participating.
– Effect of project participation: We included studies in which participation in CS was considered to be the independent variable having a potential effect on educational outcomes. We excluded studies examining, for example, the effect of invitation emails on the participation rate.
– Effects on interest, motivation, or attitudes: We included studies examining motivational outcomes. We excluded studies on knowledge and behavior since the effect on knowledge and behavior has often been the subject of previous reviews (e.g., Stepenuck and Green, 2015). Further, many studies suffer from methodological limitations in terms of the operationalization of constructs as they measured knowledge/behavior with self-reporting questionnaires or interviews. Questionnaires are subjective ratings and record the subjective assessment of one’s knowledge/behavior that is often inaccurate (Zell and Krizan, 2014). For this reason, the results do not necessarily allow for conclusions about CS-influenced knowledge/behavior (Ajzen, 1991; Sheeran and Webb, 2016).
We could use the remaining studies to illustrate the implementation of research on CS and highlight associated problems and opportunities. The significance of their results will be reflected against the background of their methodological approach, referring in each case to their internal, external, and construct validity.
– Internal validity: This is the extent to which effects on educational outcomes can be causally attributed to participation in CS. It is influenced significantly by the implementation of control groups and multiple measurement points (Campbell and Stanley, 1963; Fraenkel et al., 2012).
– External validity: This is the extent to which a study’s results are transferable to other CS contexts and projects. It is influenced by, among other things, the sample. Similarly, results cannot be generalized if studies are always limited to a similar setting or a particular discipline. Generalizable statements on the effects of CS are possible if several studies in varying contexts and with different samples provide similar results regarding an effect on personal variables (Shadish et al., 2002).
– Construct validity: This is the extent of appropriateness of inferences drawn from the results of a measurement instrument and is given mostly in measurement instruments that are developed on the basis of theory (Cohen and Swerdlik, 2009). Besides developing an instrument, theoretical foundations are equally important for selecting existing instruments. By referring to adequate theory, a distinction is made from the everyday understanding of a construct. Then, the findings of individual studies can be used to test theoretical assumptions about CS and to further develop scientific theories.
The assessment of scientific outcomes requires a different approach and depends on various aspects. As a basis for scientific outcomes, citizens need to participate in collecting or analyzing data to create appropriate data sets. Narrative project descriptions (Category b) can provide information on how many people are participating in current CS projects. Furthermore, the data have to be of high scientific quality to allow them to be evaluated for scientific purposes. The quality of CS data, as well as possible strategies for quality assessment, can be addressed by articles on data quality (a2). For CS to achieve its goal of answering relevant research questions, researchers need to publish scientific research articles to make the findings available to the scientific community. In a first approach, this review shows how many scientific publications answer their research question based on CS data. This number of studies provides first indications of scientific outcomes of CS.
The following section presents findings on educational and scientific outcomes of CS. Before considering individual studies in detail, Figure 1 illustrates the process of categorization and the assignment of the studies to the three main categories: (a) empirical scientific articles, (b) narrative projects descriptions, and (c) theoretical and technical conceptualizations of CS.
About 50% of all studies were empirical scientific articles (Category a). The remaining 50% was split equally between narrative project descriptions (25%) and theoretical and technical conceptualizations of CS (25%). First, we focus on empirical articles on educational outcomes (a1) that may provide empirical evidence for the generally assumed effects of CS on individuals. We further look at studies on data quality and on scientific outcomes as well as the distribution of studies among the other categories to address whether scientific outcomes were achieved.
Overall, only about 10% of all studies (n = 148) investigated the effect of CS projects on individual participants and might provide evidence for educational outcomes. Applying the inclusion criteria (2.3) to these empirical articles left 51 studies with which to analyze in more detail the conception of the CS project as the independent variable, the sample composition, the study design, empirical methods, and educational outcomes in terms of dependent variables.
Seven studies examined the effect of CS on interest in science or project-specific content. Most of these studies had a low internal validity because they used simple research designs (e.g., Hiller and Kitsantas, 2014; Kelemen-Finan et al., 2018). For example, Kelemen-Finan et al. (2018) implemented a one-time posttest with N = 428 students after participation in a biodiversity CS project and had no control group. Because one major weakness of this research design is that it could not measure a change in individual outcomes (Campbell and Stanley, 1963), causal assumptions about the effects of CS on interest are not valid. Causally attributing a positive effect on interest to participation in CS, this study serves as an example of overly interpreted posttest data. As shown in Table 2, only two studies investigating the effect of CS on students’ interest met the criteria of two measurement points (Seifert et al., 2016; Wallace, 2018). Seifert et al. (2016) examined the effects of a CS project on ticks and Lyme disease. After a one-hour introduction in the classroom, more than 200 high school students collected field data and ticks along 100-m transects in the surroundings of their schools, which where then committed and analyzed in university research. They implemented a pre- and posttest with a subsample of n = 23 students and were able to measure changes in the interest in science. Their results showed that students had an increased interest in pursuing science in college and graduate school after participation in CS. However, in the absence of a control group, the increase in interest cannot be causally attributed to participation in the CS project. Further, the absence of theoretical work leads to low construct validity. That is, these findings are of limited use for testing theoretical assumptions about CS and further developing basic scientific theories. Wallace (2018) implemented experimental and control groups as well as pre- and posttests, and was thereby able to control for group differences. In this study, N = 137 ninth-grade students (53.3% female) participated in the CS project BudBurst and were asked to monitor phenological changes of local tree species. To further investigate the effects of mobile devices, students were divided into three groups (participation in CS, participation in CS with mobile devices, no participation in CS). The different groups were surveyed before and after participation, that consisted of an introduction in the classroom and –depending on condition– two data collection events over a 5 week period. Results indicated an increase in STEM interest through participation in CS. Groups had comparable pretest scores and differed from each other statistically on the posttest score. Both experimental groups (participation in CS) had a significantly higher score than the control group on the STEM interest scale, but they did not differ from each other. To sum up, both studies (Seifert et al., 2016; Wallace, 2018) had an institutional context, whereas CS addresses the general population. This casts results in a critical light regarding their transferability to the overall population (external validity). External validity was limited further because both studies investigated the effect of a multi-day participation in CS projects in the field of biodiversity. These limitations to a specific sample and certain project forms do not yet allow any general conclusion about the effect of CS on interest in science. Given the importance of interest in learning processes, the results at least supported the role of CS in the school context and have an individual informative value.
Table 2. Studies investigating the effect of CS on educational outcomes with two measurement points.
In contrast to the studies on CS and interest, the 43 studies found on motivation rarely investigated a change in educational outcomes. There was often a different research interest, such as why people participated in CS (descriptive) or how motivation related to the extent of participation (correlative). Consequently, only four studies implemented two measurement points providing evidence for changes in motivation. However, a key problem with two of these studies (Domroese and Johnson, 2017; Dem et al., 2018) was that they did not use the same pre- and posttest. Due to these methodological limitations, any potential change in motivation through CS cannot be correctly confirmed empirically. The remaining studies surveyed participants of the CS projects Gardenroots (Sandhaus et al., 2019) and STEMhero (Condon and Wichowsky, 2018). In the study by Sandhaus et al. (2019), N = 94 participants were trained in data collection and were asked to collect plant, water and soil samples in their private gardens. After the samples have been analyzed by researchers, the results were presented at multiple data sharing events. The study revealed few significant changes in motivation for science learning and environmental action on the level of individual items. Due to the small number of completed posttests (n = 16) the external validity is rather low. The study by Condon and Wichowsky (2018) was based on a larger sample of N = 551 students from Catholic middle schools. In course of the CS project STEMhero, that was carried out by the respective teacher of a class, students were asked to track their water meters at home, to analyze their consumption, and to implement strategies to increase water efficiency. Results revealed that the experimental group, that was participating in STEMhero over a period of 2.5 weeks showed significantly greater gains in motivation to pursue further study in science or math. However, since this study has particularities regarding the sample and the extent of participation, the available data on an eventual change in participants’ motivation do not allow for generalized statements.
We found 15 studies focusing on attitudes toward science. Similar to the studies on interest and motivation, only a few studies (n = 7) investigated the effect of CS on attitudes toward science using questionnaires at multiple measurement points (Table 2). Six studies (Brossard et al., 2005; Jordan et al., 2011; Basham, 2012; Crall et al., 2012; Gottschalk-Druschke and Seltzer, 2012; Vitone et al., 2016) did not find any effect of CS on attitudes toward science. In the study by Basham (2012), for example, the effect of the Mastodon Matrix Project was studied with N = 11 participants of an English Language course for adults. They were asked to collect data on organic and inorganic materials by sorting through soil samples over a period of 3 weeks after having received a short introduction. The project also included a collaborative analysis and discussion of data. However, the results did not show any significant change in attitudes toward science.
Beyond these results, what these studies did have in common was their advanced research design: all had two measurement points, and two studies implemented a control group (Brossard et al., 2005; Crall et al., 2012). The CS Project The Birdhouse Network (Brossard et al., 2005) asked participants to submit data on the use of nest boxes over a certain time period. In this study, the use of an established measurement scale (MATOSS) was theory-driven and provided high construct validity. However, the comparison between pre- and posttest in the treatment group did not show any statistically significant change in attitudes toward science. N = 214 participants (75% female in experimental condition, 61% female in control condition) in the NISS project (Crall et al., 2012) were required to map invasive plant species after receiving 8 h of training. Participants in the experimental and control groups similarly received the MATOSS (Brossard et al., 2005) for pre- and posttests to validly capture attitudes toward science and ensure comparability of findings. Nonetheless, as in the study of Brossard et al. (2005), the comparison between pre- and posttest did not show any differences in attitudes toward science. Only one study investigating the effect of a CS project in the field of astronomy found a positive effect of CS on attitudes toward science (Price and Lee, 2013). The effect of CS on attitudes toward science was studied using the online CS project Citizen Sky. Since this project placed particular emphasis on communication between scientists and citizens and on empowering citizens to independently conduct their research, this study further investigated whether the level of participation was responsible for these effects. Overall, N = 333 citizen scientists of which the majority were men participated in monitoring the star epsilon Aurigae and responded to pre- and posttest. Because this study included established measurement instruments, a certain degree of construct validity in the results can be assumed. Besides a positive effect of participation on attitudes toward science, the results of the study revealed that those who participated more actively in the communication (e.g., online discussion forums) showed a significantly greater change from pre- to posttest. In contrast to the other studies, the CS project Citizen Sky provided for a high degree of participation, and the sample consisted of people with long-term participation in the project (posttest during the first login to the website 6 months after pretest). Thus, long-term participation in the project might have been a crucial condition for this positive effect. However, results were of lower internal validity due to the absence of a control group. All things considered, the methodological approaches used in these studies allow more general statements to be derived. Due to the implementation of control groups in some studies, their results were of high internal validity because they were able to test for causal assumptions on CS (Kaya, 2015). The main trend is that attitudes toward science tend not to be changed by brief participation in a CS project. Initial study results suggest that long-term and intensive participation in a CS project is likely to be required to achieve change in this relatively stable personal variable (Price and Lee, 2013). Participation in the entire scientific process and its reflection can provide enough occasions to initiate change. To support this assumption, however, further research is necessary in this field. In terms of design, research should be oriented toward the studies conducted to date and, if possible, include further measurement instruments.
The next step is to look at the empirical studies using CS data (a3). Since the successful implementation of CS is a basic condition for successfully generating data and answering research questions, it is not just empirical scientific articles, but also narrative project descriptions and theoretical and technical conceptualizations of CS that provide information about scientific outcomes. That is why we briefly discuss the remaining categories below.
A total of 173 studies addressed the question of data quality. This high number is probably due to the controversy over the ability of CS data to answer scientific research questions (Hunter, 2013; Callaghan et al., 2019). Recent studies have reported an alignment of data derived by citizen scientists with those gathered by experts—e.g., a classification accuracy of trail camera images of more than 93% (Clare et al., 2019). Callaghan et al. (2020) also mentioned a similarity of data collection performance (regarding frogs in Australia) between citizen scientists and field experts. However, they also emphasized a bias toward certain sampling areas (i.e., citizen scientists favored areas with high human populations when collecting data). To overcome this issue and other potential flaws of citizen-generated data, Fucillo et al. (2015) found that training citizen scientists on data collection skills proved to raise data quality close to the experts’ level. Twenty-eight citizen scientists received formal training for plant observation and the accuracy of nearly 11,000 observations was compared to those collected by a professional. An overall accuracy of 91% was found for CS data, which did not differ for different extent of participation (few vs. many observations). A less resource-intensive strategy was introduced by Torre et al. (2019), who showed that giving the option “I do not know” in classification activities enhanced accuracy and thus data quality. Ninety-four participants were asked to classify images of polluted water bodies whether this pollution was a threat to the environment or not. If the participants received the option “I do not know,” there was an improvement in the true negative rate, i.e., this group showed higher accuracy in the identification of “no threat” than the group without this option. The increased data quality goes along with a reduction in data quantity, but may be accepted to ensure the accuracy of CS data.
About 20% of the studies (n = 279) used CS data to answer scientific questions. CS data is not limited to a few scientific fields but is very diverse. One example is clarifying how coral reefs change as an ecosystem and might react to future disturbances (Gouraguine et al., 2019). In this study, a decline in coral was revealed with data from about 275 citizen scientists who monitored permanent transects over a period of 10 years. Another example is using CS data to provide answers on how Yellowhammers’ song dialects are distributed in Czechia (Diblíková et al., 2018). For this purpose, nearly 4,000 recordings were used, which were collected from citizens over a period of 6 years. Apart from questions on various organisms, data gathered by citizen scientists have also been beneficial in other disciplines: for instance, American astronomers identified a new planet with the help of CS data from the project Planet Hunters (Citizen Science Finds Planet, 2012). Data from citizen scientists have also aided in the medical field, e.g., by providing information on the prevalence of ticks carrying pathogens causing borreliosis in the US (Nieto et al., 2018). Citizens collected over 16,000 ticks and submitted them with information on where they were found (host, location) to Northern Arizona University where the ticks were tested for pathogens.
There were 240 project descriptions. A suitable example for studies in this category is Chiovitti et al.’ (2019) presentation of an educational barcoding project in which students (N = 406) extracted and analyzed DNA from reptile livers. Inter alia, the authors describe the project’s process, the (scientific and educational) materials used, and compliance with the school curriculum. The participating students collected and analyzed about 200 samples creating also new sequences for 8 reptile species. Most studies in this category aimed to describe a particular CS project and present results covering the number of samples and species that the participants examined. However, they did not report empirical results on either a project’s effects on its participants or on a project’s empirical findings and the quality of generated data.
There were 74 studies dealing with the clustering of numerous studies in one area such as biodiversity. For example, the study by Pocock et al. (2017) conducted a systematic search for environmental and ecological CS projects. Each of the 509 projects found was scored systematically for 32 attributes—inter alia, methodological approaches, types of data recording, and target groups. It was found, for example, that the number of biodiversity projects increased exponentially and over 90% of the analyzed CS projects limit citizen involvement to data collection. Hence, this study clustered and described the projects without either going into detail on possible influences on the participants or discussing the data quality.
There were 105 publications theoretically describing the advantages and disadvantages of CS. Pocock et al. (2018), for example, derived opportunities for, benefits of, and barriers to CS in East Africa from a collaborative prioritization among 22 experts because CS is insufficiently distributed in developing countries. From this systematic theoretical assessment, the authors concluded that biodiversity and environmental monitoring are opportunities, that increasing environmental awareness as well as useful data and approaches are benefits, and that institutional capacity and (lack of) ascribed value to such activities are barriers that are applicable across developing countries. As another example, Fritz et al. (2019) described how CS as a non-traditional data source could support the Sustainable Development Goals (SDGs). As traditional data sources are presented as insufficient for measuring the SDGs, the potential uses of CS data are outlined as well as a roadmap for integrating CS in SDG reporting.
Almost 20% of the articles (n = 221) dealt with developing technical devices that could be used in the implementation of CS projects. Ožana et al. (2019) presented a mobile application to map species occurrences of dragonflies. The technical structure of the app as well as the various functions, such as support in the classification of dragonflies or the storage of GPS data, are presented. Another example is the study by Dennis et al. (2017) presenting new approaches for the analysis of biodiversity data with logistic regression, as CS data usually have a large spatial coverage and provide an important basis for biodiversity monitoring.
The large number of project descriptions (b1, b2) show that there are already many different CS projects in which numerous people participate and can thus contribute to an extensive database. Additionally, studies on technical devices (c2) show that numerous tools further facilitate participation in CS projects; and, in some cases, allow it to take place at any time around the globe. The large number of studies using CS data (a3) shows that scientific publications based on CS data have already been published very frequently, and that they have contributed to relevant research questions. Finally, many studies on data quality (a2) provide evidence of high quality in CS data allowing confidence to be placed in the scientific publications. All these results together provide initial evidence for scientific outcomes of CS.
CS is attracting increasing attention as an innovative form of science communication (Haklay et al., 2020). This is reflected by various CS projects and an increasing number of research articles in recent years. To give some structure to the growing literature in this emerging field, this review provides a fine-grained portrayal of research on CS. We developed a coding manual and sorted the systematically searched literature into predefined categories. Empirical scientific articles (Category a) account for a share of about 50% (n = 600). This points to a considerable need for empirical studies in the large research field of CS in general. Narrative project descriptions (Category b) usually do not have a specific research question that is investigated empirically. Instead, they present specific CS projects descriptively. With a share of around 25%, they make up a large proportion of all research articles and clearly show that there are already numerous and diverse CS projects. Theoretical and technical conceptualizations of CS (Category c) account for about 25% of all articles. Studies in this category present theoretical assumptions and frameworks about CS or the development of technical devices showing that many tools have already been created to support and facilitate participation in CS projects.
We initially used our coding to find out about both educational and scientific outcomes of CS. We asked how many studies are available that report educational outcomes of participation in CS and whether these are sufficient to allow us to make generalized statements in terms of motivational outcomes (interest, motivation, attitudes).
Although interest plays a central role in individual learning processes (Hidi and Renninger, 2006), only a few research articles are available on the development of interest through CS. The fact that only two studies used a scientifically sound research design emphasizes a substantial research gap in this area. Even though these two studies (Seifert et al., 2016; Wallace, 2018) provide initial evidence for the positive effect of CS on interest, it is not yet possible to generalize this assumption. Because both studies were conducted in institutional contexts and focused on one specific domain, the transferability (external validity) of their results is limited. We cannot conclusively assess whether participation in CS is likely to increase the interest of the participants and, thus, a great amount of research still needs to be conducted. In contrast to the small number of studies on interest, many studies are available on motivation. However, very few studies focus on a possible change of motivation through participation in CS, indicating a general deficit of research studies in this area. Due to this lack of empirical studies, it is not yet possible to provide any generalizable statements concerning the positive effect of CS on motivation. Research on the development of attitudes toward science is already quite extensive and provides consistent results. The present findings suggest that short-term participation in CS has no positive effect on attitudes toward science. Particularly those studies with an advanced design often fail to show any effect. One possible explanation for this lack of change could be the theoretical assumptions on the construct of attitudes. This points to the importance of sound theoretical foundations: due to their deep grounding in early subjective experiences, attitudes are stable personality traits (Pajares, 1992). Nevertheless, attitudes are assumed to be changeable to some extent. Although they may not be changed measurably by a short participation in CS, Price and Lee’s (2013) study does suggest that a high level of participation may effect such change. Potential changes in attitudes may well become visible when central theoretical assumptions and their implications are taken into account. To validate the results on the effect of CS on attitudes toward science, future research should focus mainly on project forms in which citizens are highly involved. Moreover, because our conclusion is limited on the effect of CS on attitudes toward science, future studies might consider investigating possible change in other attitudes (e.g., attitudes toward nature or wildlife).
To assess the scientific outcomes, we paid particular attention to studies using CS data (a3). Overall, they account for a large proportion (50%) of all empirical scientific articles. This demonstrates that a considerable number of scientific publications have already been generated with CS data. In addition, studies in other categories, such as studies on data quality, also provide important indications on scientific outcomes. About 25% of all empirical scientific articles relate to the quality of CS data in different scientific disciplines. In most cases, they indicate a high quality of CS data, that is an initial indication of scientific outcomes. To consider the scientific outcomes, however, it is not sufficient to publish scientific research articles. A precise distinction still has to be made between the mere number of studies based on CS data and the acceptance of these studies in the respective research discipline—which represents a further significant step toward solid scientific outcomes. However, due to the large number of studies from various disciplines using CS data, the impact of the individual research articles cannot be verified within the scope of this review, especially because such an in-depth analysis would also require expertise in all the respective disciplines. The study by Odenwald (2018) is a very successful example for the evaluation of scientific outcomes in a specific scientific discipline. Odenwald (2018) analyzes the citations of 143 CS publications in the specific field of astronomy. In total, the papers were cited over 4,500 times. Comparing these papers to a group of comparable papers in the same journals, results suggest that there is high interest in CS publication but “remain of interest for only half as long as other papers” (Odenwald, 2018, p. 1) in the field.
One potential limitation of the review is the reliance on quantitative studies. This focus was chosen with the aim of making generalizable statements on the outcomes of CS projects. Qualitative studies, in contrast, pursue the goal of exploring a research field and generating hypotheses rather than testing causal effects and do not allow for generalizable statements. However, since the effects of CS projects may be context dependent and qualitative studies provide insights into participants’ thoughts and perceptions, it may be useful to review these studies on educational outcomes of CS as well. The central issue in the present review is the limited sample size. We searched for relevant articles in the two different databases, Web of Science being only one of the international databases for scientific articles. In this database as well as the German database FIS Bildung, we searched for the term “citizen science” in the title, but not in the abstract or keywords, possibly further limiting the sample of relevant research articles. Since the terms used to describe CS are very diverse (for an overview see Haklay et al., 2021), the results should be consolidated with other search terms. However, since we have additionally taken into account all articles from the journal Citizen Science: Theory and Practice and our sample still includes more than 1,200 research articles, we can draw relatively firm conclusions from it. The results of the review show that, overall, there is still limited evidence on which to base generalizable statements on educational outcomes of CS. Also, with regard to the scientific outcomes, further efforts need to be made because of the need to evaluate the acceptance of the studies in the respective scientific community.
The great need for further research, especially with regard to educational outcomes, is due to the fact that the weak research designs in many studies do not allow causal inferences. Focusing on the assessment methods of environmental attitudes, behavior and knowledge, Somerwill and Wehn (2022) also point out that “in many citizen science projects, impact evaluation is still overly simplistic” (p. 1) despite the many frameworks and expertise from different specific disciplines. In order to obtain valid results, with which “a greater understanding of citizen science could be obtained, supporting relevant policy and research in the future” (Somerwill and When, 2022, p. 10) we derived central implications for future research in CS.
One key requirement for future studies is to be of high internal validity, because it “is the basic minimum without which any experiment is uninterpretable” (Campbell and Stanley, 1963, p. 5). Internal validity is significantly ensured by the implementation of control groups. To achieve a true experimental design, participants need to be randomly assigned to different groups (Campbell and Stanley, 1963). If random assignment cannot be realized (quasi-experiment), confounding variables must be controlled with at least one pretest allowing control of preexisting group differences. It is certainly challenging to conduct experimental designs in CS contexts, because numerous organizational and ethical aspects have to be taken into account. One organizational aspect that limits feasibility in the context of CS is the difficulty in recruiting comparable control groups. In addition, it is necessary to balance the contrast between the voluntary nature of participating in CS to actively conduct research and the fact that participants become the research object in the experiment itself. This is probably why only a few studies are available with quasi-experimental designs ensuring a moderate internal validity (e.g., Wallace, 2018). Because only studies implementing control groups provide robust results on the effect of CS on educational outcomes, the implementation of such designs should be a central goal for future research. Further, it is important to consider the evaluation of effects from the outset “largely because many impact measurement tools (particularly those offering high-quality data) require baseline measurements before participation” (Somerwill and Wehn, 2022, p. 9). Dickinson and Crain (2019) also emphasize that “controlled studies are needed to determine whether citizen science projects meet the specific learning objectives for which they are designed” (p. 1). This lack of experimental studies has already been noted in previous reviews focusing on changes in knowledge, behavior, and attitudes through participation in CS (Peter et al., 2019; Aristeidou and Herodotou, 2020) and we can generalize their demands to cover empirical studies.
Causal knowledge of individual experiments does not allow conclusions to be drawn on whether this causal relationship holds over variations in participants and settings (Shadish et al., 2002). To make generalized statements about the effect of CS on educational outcomes, numerous experiments would need to achieve similar results while differing in terms of sample, setting, treatment, and measure (Shadish et al., 2002). Many recent studies have been carried out in an institutional context granting continued access to the participants for recurring surveys. These limitations to a specific sample and setting restrict the transferability of previous results on the effect of CS. Future research should try to focus on CS projects outside of institutional boundaries, because the majority of projects have been developed for the general population and not for classroom implementation. Further, most of the studies on educational outcomes examine CS projects in the subject field of biodiversity (e.g., Aivelo and Huovelin, 2020). Other subjects that are also represented more frequently are astronomy and water quality (e.g., Price and Lee, 2013). To ensure the generalizability of results, future research needs to provide equal representation to the different scientific disciplines in which CS projects are offered. With regard to the treatment, the vast majority of studies investigate CS projects providing for a low level of citizen participation in the scientific process (e.g., Seifert et al., 2016; Tinati et al., 2017). To further clarify the effect of CS on educational outcomes and to confirm the findings with respect to attitudes toward science, future research should consider different project forms providing for different levels of participation (e.g., full participation in the scientific process). Finally, different (motivational) variables should become the object of investigation. This would allow comprehensive statements on educational outcomes. In this context, different and, ideally, already established measurement instruments should be used. This leads to the aspect of construct validity.
Construct validity is another fundamental concern in any empirical study on the effect of educational outcomes of CS. In the referenced studies, we observed a frequent absence of theory-based operationalizations of constructs and consequently the use of self-developed measurement instruments with no assessment of their quality. Our results also confirm previous reviews criticizing “the scarcity of established theoretical frameworks” (Peter et al., 2019, p. 14) and the “tendency to use self-reported methods” (Aristeidou and Herodotou, 2020, p. 9) in CS projects. Due to the lack of theoretical work, findings from individual studies can mostly be used only to a limited extent for testing theoretical assumptions about CS and further developing basic scientific theories. To validly draw inferences about the construct under consideration, it is important to define it theoretically before developing suitable items. Moreover, the use of established measurement instruments should be preferred. For instance, scales to measure attitudes have been developed and evaluated by, for example Kind et al. (2007), Lederman et al. (2014), and Hartman et al. (2017). Levontin et al. (2022) suggest a standardized, theory-based approach to measure participants’ motivation in a comparable manner. These measures ensure the validity of the intended interpretation of the results and enable further development of basic scientific theories. Because theoretical work and the use of instruments with high test quality are rare to date, this should be given greater consideration in future research. Interdisciplinary cooperation may be helpful in theoretically defining the constructs and in carrying out a well-founded selection of instruments here.
Concerning educational outcomes, the available studies on interest and motivation provide too little evidence to empirically confirm the assumed effects of CS on its participants. According to the data on attitudes available so far, CS seems to have a positive effect only if the level of participation is high and long lasting. Thus, the effect of CS on attitudes is minimal, and educational outcomes are produced only partially. With regard to scientific outcomes, review results indicate that CS has already taken an important step, because it has produced many scientific studies based on CS data. To come to reliable conclusions regarding scientific outcomes, future research must examine the acceptance and use of the CS studies in the respective disciplines (e.g., Odenwald, 2018). In general, there is a need for more research on CS, with the effect of CS on educational outcomes being just one of the many important questions for the future. The increasing number of exciting CS projects will make it possible to answer important research questions in various scientific disciplines and to investigate potential effects on participants in a variety of CS contexts. However, valid statements on the effect of CS on personal variables can be made only if future research takes even greater account of methodological standards than has been the case to date.
MS, VB, JW, and LF: conceptualization. LF and VB: literature search. LF: literature analysis. LF and LS: writing – original draft preparation. JF, KS, MS, VB, and JW: writing – review and editing. JW: supervision. All authors contributed to the article and approved the submitted version.
The present study was conducted within the doctoral program MeMo-akS of the Professional School of Education of the Ruhr University Bochum. We acknowledge support by the Open Access Publication Funds of the Ruhr University Bochum.
The authors declare that the research was conducted in the absence of any commercial or financial relationships that could be construed as a potential conflict of interest.
All claims expressed in this article are solely those of the authors and do not necessarily represent those of their affiliated organizations, or those of the publisher, the editors and the reviewers. Any product that may be evaluated in this article, or claim that may be made by its manufacturer, is not guaranteed or endorsed by the publisher.
Aivelo, T., and Huovelin, S. (2020). Combining formal education and citizen science: a case study on students’ perceptions of learning and interest in an urban rat project. Environ. Educ. Res. 26, 324–340. doi: 10.1101/2020.01.27.921395
Ajzen, I. (1991). The theory of planned behavior. Organ. Behav. Hum. Decis. Process. 50, 179–211. doi: 10.1016/0749-5978(91)90020-T
Aristeidou, M., and Herodotou, C. (2020). Online citizen science: a systematic review of effects on learning and scientific literacy. Citizen Sci Theory Practice 5:11. doi: 10.5334/cstp.224
Basham, M. (2012). Pathways of knowing: Integrating citizen science and critical thinking in the adult ELL classroom. Doctoral dissertation, AZ: Arizona State University.
Bonn, A., Richter, A., Vohland, K., Pettibone, E., Phillips, T., Shirk, J., et al. (2016). Green paper citizen science strategy 2020 for Germany. Berlin: GEWISS.
Bonney, R., Ballard, H., Jordan, R., McCallie, E., Phillips, T., Shirk, J., et al. (2009). Public participation in scientific research: defining the field and assessing its potential for informal science education. A CAISE inquiry group report. Washington, DC: Center for Advancement of Informal Science Education (CAISE).
Bonney, R., Phillips, T., Ballard, H. L., and Enck, J. W. (2016). Can citizen science enhance public understanding of science? Public Underst. Sci. 25, 2–16. doi: 10.1177/0963662515607406
Bonney, R., Shirk, J. L., Phillips, T., Wiggins, A., Ballard, H. L., Miller-Rushing, A. J., et al. (2014). Next steps for citizen science. Science 343, 1436–1437. doi: 10.1126/science.1251554
Brossard, D., Lewenstein, B., and Bonney, R. (2005). Scientific knowledge and attitude change: the impact of a citizen science project. Int. J. Sci. Educ. 27, 1099–1121. doi: 10.1080/09500690500069483
Callaghan, C. T., Roberts, J. D., Poore, A. G. B., Alford, R. A., Cogger, H., and Rowley, J. J. L. (2020). Citizen science data accurately predicts expert-derived species richness at a continental scale when sampling thresholds are met. Biodivers. Conserv. 29, 1323–1337. doi: 10.1007/s10531-020-01937-3
Callaghan, C. T., Rowley, J. J. L., Cornwell, W. K., Poore, A. G. B., and Major, R. E. (2019). Improving big citizen science data: moving beyond haphazard sampling. PLoS Biol. 17, e3000357–e3000311. doi: 10.1371/journal.pbio.3000357
Campbell, D. T., and Stanley, J. C. (1963). Experimental and quasi-experimental designs for research. Boston, MA: Houghton Mifflin Company.
Chiovitti, A., Thorpe, F., Gorman, C., Cuxson, J. L., Robevska, G., Szwed, C., et al. (2019). A citizen science model for implementing statewide educational DNA barcoding. PLoS One 14, e0208604–e0208618. doi: 10.1371/journal.pone.0208604
Citizen Science Finds Planet (2012). Citizen science finds planet. Astronomy Geophysics 53:6.4. doi: 10.1111/j.1468-4004.2012.53604_3.x
Clare, J. D. J., Townsend, P. A., Anhalt-Depies, C., Locke, C., Stenglein, J. L., Frett, S., et al. (2019). Making inference with messy (citizen science) data: when are data accurate enough and how can they be improved? Ecol. Appl. 29, e01849–e01815. doi: 10.1002/eap.1849
Cohen, R. J., and Swerdlik, M. (2009). Psychological testing and assessment: An introduction to tests and measurement. 7th edn. New York, NY: McGraw-Hill.
Condon, M., and Wichowsky, A. (2018). Developing citizen-scientists: effects of an inquiry-based science curriculum on STEM and civic engagement. Elem. Sch. J. 119, 196–222. doi: 10.1086/700316
Cooper, H. (2016). Research synthesis and meta-analysis. A step-by-step approach (5th edn.) Thousand Oaks, CA: Sage.
Crall, A. W., Jordan, R., Holfelder, K., Newman, G. J., Graham, J., and Waller, D. M. (2012). The impacts of an invasive species citizen science training program on participant attitudes, behavior, and science literacy. Public Underst. Sci. 22, 745–764. doi: 10.1177/0963662511434894
Dem, E. S., Rodríguez-Labajos, B., Wiemers, M., Ott, J., Hirneisen, N., Bustamante, J. V., et al. (2018). Understanding the relationship between volunteers’ motivations and learning outcomes of citizen science in rice ecosystems in the northern Philippines. Paddy Water Environ. 16, 725–735. doi: 10.1007/s10333-018-0664-9
Dennis, E. B., Morgan, B. J. T., Freeman, S. N., Ridout, M. S., Brereton, T. M., Fox, R., et al. (2017). Efficient occupancy model-fitting for extensive citizen-science data. PLoS One 12:e0174433. doi: 10.1371/journal.pone.0174433
Diblíková, L., Pipek, P., Petrusek, A., Svoboda, J., Bílková, J., Vermouzek, Z., et al. (2018). Detailed large-scale mapping of geographical variation of yellowhammer emberiza citrinella song dialects in a citizen science project. Int J Avian Sci 161, 401–414. doi: 10.1111/ibi.12621
Dickinson, J. L., and Crain, R. (2019). An experimental study of learning in an online citizen science project: insights into study design and waitlist controls. Citizen Sci Theory Pract 4, 1–13. doi: 10.5334/cstp.218
Domroese, M. C., and Johnson, E. A. (2017). Why watch bees? Motivations of citizen science volunteers in the great pollinator project. Biol. Conserv. 208, 40–47. doi: 10.1016/j.biocon.2016.08.020
European Commission (2016). Classification of learning activities (CLA) – Manual: 2016 edition. Luxembourg: Publications Office of the European Union.
Fraenkel, J. R., Wallen, N. E., and Hyun, H. H. (2012). How to design and evaluate research in education. 8th edn. New York, NY: McGraw-Hill.
Fritz, S., See, L., Carlson, T., Haklay, M., Oliver, J. L., Fraisl, D., et al. (2019). Citizen science and the United Nations sustainable development goals. Nat. Sustain. 2, 922–930. doi: 10.1038/s41893-019-0390-3
Fucillo, K. K., Crimmins, T. M., de Rivera, C. E., and Elder, T. S. (2015). Assessing accuracy in citizen science-based plant phenology monitoring. Int. J. Biometeorol. 59, 917–926. doi: 10.1007/s00484-014-0892-7
Gottschalk-Druschke, C., and Seltzer, C. E. (2012). Failures of engagement: lessons learned from a citizen science pilot study. Appl. Environ. Educ. Commun. 11, 178–188. doi: 10.1080/1533015X.2012.777224
Gouraguine, A., Moranta, J., Ruiz-Frau, A., Hinz, H., Reñones, O., Ferse, S. C. A., et al. (2019). Citizen science in data and resource-limited areas: a tool to detect long-term ecosystem changes. PLoS One 14, e0210007–e0210014. doi: 10.1371/journal.pone.0210007
Groulx, M., Brisbois, M. C., Lemieux, C. J., Winegardner, A., and Fishback, L. (2017). A role for nature-based citizen science in promoting individual and collective climate change action? A systematic review of learning outcomes. Sci. Commun. 39, 45–76. doi: 10.1177/1075547016688324
Haklay, M. (2013). “Citizen science and volunteered geographic information: overview and typology of participation” in Crowdsourcing geographic knowledge. eds. D. Sui, S. Elwood, and M. Goodchild (Dordrecht: Springer), 105–124.
Haklay, M., Dörler, D., Heigl, F., Manzoni, M., Hecker, S., and Vohland, K. (2021). “What is citizen science? The challenges of definition” in The science of citizen science. eds. K. Vohland, et al. (Cham, Switzerland: Springer), 13–34.
Haklay, M., Motion, A., Balázs, B., Kieslinger, B., Greshake Tzovaras, B., Nold, C., et al. (2020). ECSA’s characteristics of citizen science. Zenodo. doi: 10.5281/zenodo.3758668
Hartman, R. O., Dieckmann, N. F., Sprenger, A. M., Stastny, B. J., and DeMarree, K. G. (2017). Modeling attitudes toward science: development and validation of the credibility of science scale. Basic Appl. Soc. Psychol. 39, 358–371. doi: 10.1080/01973533.2017.1372284
Hidi, S., and Renninger, K. A. (2006). The four-phase model of interest development. Educ. Psychol. 41, 111–127. doi: 10.1207/s15326985ep4102_4
Hiller, S. E., and Kitsantas, A. (2014). The effect of a horseshoe crab citizen science program on middle school student science performance and STEM career motivation. Sch. Sci. Math. 114, 302–311. doi: 10.1111/ssm.12081
Hunter, J. (2013). Assessing the quality and trustworthiness of citizen science data. Concurr. Comput. 25, 454–466. doi: 10.1002/cpe.2923
Jordan, R. C., Gray, S. A., Howe, D. V., Brooks, W. R., and Ehrenfeld, J. G. (2011). Knowledge gain and behavioral change in citizen-science programs. Conserv. Biol. 25, 1148–1154. doi: 10.1111/j.1523-1739.2011.01745.x
Kaya, C. (2015). Internal validity: a must in research designs. Educ. Res. Rev. 10, 111–118. doi: 10.5897/ERR2014.1835
Kelemen-Finan, J., Scheuch, M., and Winter, S. (2018). Contributions from citizen science to science education: an examination of a biodiversity citizen science project with schools in Central Europe. Int. J. Sci. Educ. 40, 2078–2098. doi: 10.1080/09500693.2018.1520405
Kind, P. M., Jones, K., and Barmby, P. (2007). Developing attitudes towards science measures. Int. J. Sci. Educ. 29, 871–893. doi: 10.1080/09500690600909091
Krapp, A. (1999). Interest, motivation, and learning: an educational-psychological perspective. Eur. J. Psychol. Educ. 14, 23–40. doi: 10.1007/BF03173109
Lederman, J. S., Lederman, N. G., Bartos, S. A., Bartels, S. L., Meyer, A. A., and Schwartz, R. S. (2014). Meaningful assessment of learners’ understandings about scientific inquiry – the views about scientific inquiry (VASI) questionnaire. J. Res. Sci. Teach. 51, 65–83. doi: 10.1002/tea.21125
Levontin, L., Gilad, Z., Shuster, B., Chako, S., Land-Zandstra, A., Lavie-Alon, N., et al. (2022). Standardizing the assessment of citizen scientists’ motivations: a motivational goal-based approach. Citizen Sci Theory Pract 7, 1–15. doi: 10.5334/cstp.459
Nieto, N. C., Porter, W. T., Wachara, J. C., Lowrey, T. J., Martin, L., Motyka, P. J., et al. (2018). Using citizen science to describe the prevalence and distribution of tick bite and exposure to tick-borne diseases in the United States. PLoS One 13:e0199644. doi: 10.1371/journal.pone.0199644
Odenwald, S. (2018). A citation study of citizen science projects in space science and astronomy. Citizen Sci Theory Pract 3, 1–11. doi: 10.5334/cstp.152
Ožana, S., Burda, M., Hykel, M., Malina, M., Prášek, M., Bárta, D., et al. (2019). Dragonfly Hunter CZ: Mobile application for biological species recognition in citizen science. PLoS One 14, e0210370–e0210313. doi: 10.1371/journal.pone.0210370
Pajares, M. F. (1992). Teachers’ beliefs and educational research: cleaning up a messy construct. Rev. Educ. Res. 62, 307–332. doi: 10.3102/00346543062003307
Perelló, J., Klimczuk, A., Land-Zandstra, A., Vohland, K., Wagenknecht, K., Narraway, C., et al. (2021). “The recent past and possible futures of citizen science: final remarks” in The science of citizen science. eds. K. Vohland, A. Land-Zandstra, and L. Ceccaroni, et al. (Cham, Switzerland: Springer Nature), 517–529.
Peter, M., Diekötter, T., and Kremer, K. (2019). Participant outcomes of biodiversity citizen science projects: a systematic literature review. Sustainability 11:2780. doi: 10.3390/su11102780
Phillips, T., Ferguson, M., Minarchek, M., Porticella, N., and Bonney, R. (2014). User’s guide for evaluating learning outcomes in citizen science. Ithaca, NY: Cornell Lab of Ornithology.
Pocock, M. J. O., Roy, H., August, T., Kuria, A., Barasa, F., Bett, J., et al. (2018). Developing the global potential of citizen science: assessing opportunities that benefit people, society and the environment in East Africa. J. Appl. Ecol. 56, 274–281. doi: 10.1111/1365-2664.13279
Pocock, M. J. O., Tweddle, J. C., Savage, J., Robinson, L. D., and Roy, H. E. (2017). The diversity and evolution of ecological and environmental citizen science. PLoS One 12, e0172579–e0172517. doi: 10.1371/journal.pone.0172579
Potvin, P., and Hasni, A. (2014). Analysis of the decline in interest towards school science and technology from grades 5 through 11. J. Sci. Educ. Technol. 23, 784–802. doi: 10.1007/s10956-014-9512-x
Price, C. A., and Lee, H. S. (2013). Changes in participants’ scientific attitudes and epistemological beliefs during an astronomical citizen science project. J. Res. Sci. Teach. 50, 773–801. doi: 10.1002/tea.21090
Roche, J., Bell, L., Galvão, C., Golumbic, Y. N., Kloetzer, L., Knoben, N., et al. (2020). Citizen science, education, and learning: challenges and opportunities. Front. Sociol. 5:613814. doi: 10.3389/fsoc.2020.613814
Ryan, R. M., and Deci, E. L. (2000). Self-determination theory and the facilitation of intrinsic motivation, social development, and well-being. Am. Psychol. 55, 68–78. doi: 10.1037/0003-066X.55.1.68
Ryan, R. M., and Deci, E. L. (2002). “Overview of self-determination theory: an organismic-dialectical perspective” in Handbook of self-determination research. eds. E. L. Deci and R. M. Ryan (Rochester: University of Rochester Press), 3–33.
Sandhaus, S., Kaufmann, D., and Ramirez-Andreotta, M. (2019). Public participation, trust and data sharing: gardens as hubs for citizen science and environmental health literacy efforts. Int. J. Sci. Educ. Part B Commun. Public Engage. 9, 54–71. doi: 10.1080/21548455.2018.1542752
Schuttler, S., Sorensen, A., Jordan, R., Cooper, C., and Shwartz, A. (2018). Bridging the nature gap: can citizen science reverse the extinction of experience? Front. Ecol. Environ. 16, 405–411. doi: 10.1002/fee.1826
Seifert, V. A., Wilson, S., Toivonen, S., Clarke, B., and Prunuske, A. (2016). Community partnership designed to promote Lyme disease prevention and engagement in citizen science. J. Microbiol. Biol. Educ. 17, 63–69. doi: 10.1128/jmbe.v17i1.1014
Shadish, W. R., Cook, T. D., and Campbell, D. T. (2002). Experimental and quasi-experimental designs for generalized causal inference. Boston, MA: Houghton Mifflin Company.
Sheeran, P., and Webb, T. L. (2016). The intention-behavior gap. Soc. Personal. Psychol. Compass 10, 503–518. doi: 10.1111/spc3.12265
Shirk, J. L., Ballard, H. L., Wilderman, C. C., Phillips, T., Wiggins, A., Jordan, R., et al. (2012). Public participation in scientific research: a framework for deliberate design. Ecol. Soc. 17:29. doi: 10.5751/ES-04705-170229
Sigel, I. E. (1985). “A conceptual analysis of beliefs” in Parental belief systems: the psychological consequences for children. ed. I. E. Sigel (Hillsdale, NJ: Lawrence Erlbaum Taylor & Francis Group), 347–371.
Socientize (2015). White paper on citizen science for Europe. EU: European Commission. Available at: https://ec.europa.eu/futurium/en/content/white-paper-citizen-science.html
Somerwill, L., and Wehn, U. (2022). How to measure the impact of citizen science on environmental attitudes, behaviour and knowledge? A review of state-of-the-art approaches. Environ. Sci. Eur. 34, 1–29. doi: 10.1186/s12302-022-00596-1
Stepenuck, K. F., and Green, L. T. (2015). Individual- and community-level impacts of volunteer environmental monitoring: a synthesis of peer-reviewed literature. Ecol. Soc. 20:19. doi: 10.5751/ES-07329-200319
Stilgoe, J., Lock, S. J., and Wilsdon, J. (2014). Why should we promote public engagement with science? Public Underst. Sci. 23, 4–15. doi: 10.1177/0963662513518154
Tinati, R., Luczak-Roesch, M., Simperl, E., and Hall, W. (2017). An investigation of player motivations in Eyewire, a gamified citizen science project. Comput. Hum. Behav. 73, 527–540. doi: 10.1016/j.chb.2016.12.074
Torre, M., Nakayama, S., Tolbert, T. J., and Porfiri, M. (2019). Producing knowledge by admitting ignorance: enhancing data quality through an “I don’t know” option in citizen science. PLoS One 14, e0211907–e0211915. doi: 10.1371/journal.pone.0211907
Vitone, T., Stofer, K. A., Steininger, M. S., Hulcr, J., Dunn, R., and Lucky, A. (2016). School of Ants goes to college: integrating citizen science into the general education classroom increases engagement with science. J. Sci. Commun. 15, 1–24. doi: 10.22323/2.15010203
Vohland, K., Land-Zandstra, A., Ceccaroni, L., Lemmens, R., Perelló, J., Ponti, M., et al. (2021). “Editorial: the science of citizen science evolves” in The science of citizen science. eds. K. Vohland, A. Land-Zandstra, and L. Ceccaroni, et al. (Cham, Switzerland: Springer), 1–12.
Wallace, D. E. (2018). Creating citizen science identity: Growing conservation and environmentally-minded Stem interest through mobile learning and authentic practice. Doctoral dissertation, PA: Lehigh University.
When, U., Gharesifard, M., Ceccaroni, L., Joyce, H., Ajates, R., Woods, S., et al. (2021). Impact assessment of citizen science: state of the art and guiding principles for a consolidated approach. Sustain. Sci. 16, 1683–1699. doi: 10.1007/s11625-021-00959-2
Keywords: citizen science, science education, motivational effects, literature review, validity
Citation: Finger L, van den Bogaert V, Schmidt L, Fleischer J, Stadtler M, Sommer K and Wirth J (2023) The science of citizen science: a systematic literature review on educational and scientific outcomes. Front. Educ. 8:1226529. doi: 10.3389/feduc.2023.1226529
Received: 21 May 2023; Accepted: 14 August 2023;
Published: 24 August 2023.
Edited by:
Zakiya Wilson-Kennedy, Louisiana State University, United StatesReviewed by:
Styliani Kleanthous, Open University of Cyprus, CyprusCopyright © 2023 Finger, van den Bogaert, Schmidt, Fleischer, Stadtler, Sommer and Wirth. This is an open-access article distributed under the terms of the Creative Commons Attribution License (CC BY). The use, distribution or reproduction in other forums is permitted, provided the original author(s) and the copyright owner(s) are credited and that the original publication in this journal is cited, in accordance with accepted academic practice. No use, distribution or reproduction is permitted which does not comply with these terms.
*Correspondence: Lena Finger, bGVuYS5maW5nZXJAcnViLmRl
Disclaimer: All claims expressed in this article are solely those of the authors and do not necessarily represent those of their affiliated organizations, or those of the publisher, the editors and the reviewers. Any product that may be evaluated in this article or claim that may be made by its manufacturer is not guaranteed or endorsed by the publisher.
Research integrity at Frontiers
Learn more about the work of our research integrity team to safeguard the quality of each article we publish.