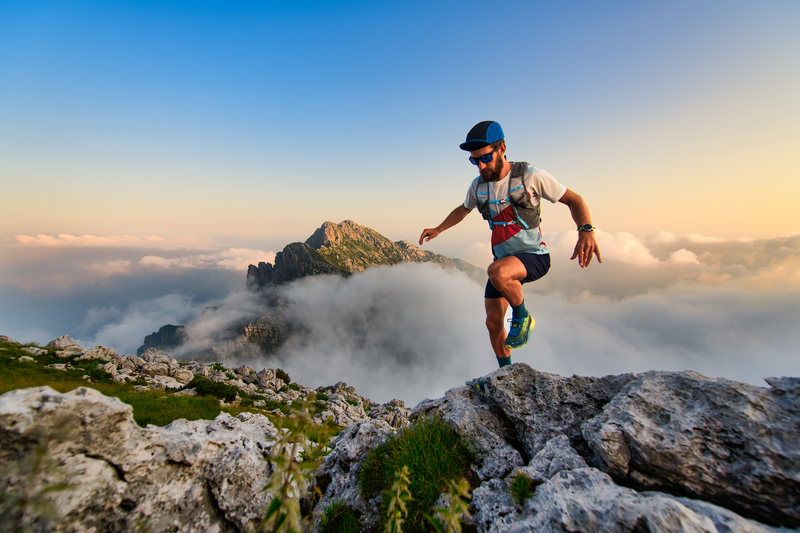
94% of researchers rate our articles as excellent or good
Learn more about the work of our research integrity team to safeguard the quality of each article we publish.
Find out more
ORIGINAL RESEARCH article
Front. Educ. , 28 July 2023
Sec. Digital Education
Volume 8 - 2023 | https://doi.org/10.3389/feduc.2023.1223656
This article is part of the Research Topic Recent Innovations on Applied Technology in Education View all 5 articles
Despite the profusion of studies on the use of augmented reality (AR) for teaching, the scientific results on the students’ learning do not fully converge. On the one hand, some studies have shown AR’s benefits, including autonomy and improvement of learning by reducing mental effort. On the other hand, other studies have highlighted the strong limitations of AR technology for learning, such as cognitive over loading in some specific cases. This study investigates the impact of AR on the mental effort and learning outcomes of students who were involved in an inquiry-based science training session. The sample was composed of French Master’s degree students. A control group who used physical modeling activities and an experimental group who used an AR model for the first time studied the phenomenon of the seasons. The students were tested on their learning outcomes and mental effort during the training session. Results revealed no significant differences, except for the short-term test which showed better learning results for the control group. Moreover, a link between mental effort and learning outcomes was observed independently of the group conditions. Despite the first use of an AR model to study a complex scientific phenomenon, the experimental group (AR) performs in similar way to the control group (without AR) in long-term learning outcomes and mental effort.
The use of augmented reality (AR) in the learning of scientific concepts is highly emphasized (Bujak et al., 2013; Fleck and Audran, 2016; Huang et al., 2016; Arici and Yilmaz, 2023; Yilmaz, 2023) especially when it allows access to the invisible, which is important in science learning (Cuendet et al., 2013; Di Serio et al., 2013; Ruiz-Ariza et al., 2018). Chen et al. (2017) analyzed the database of social sciences citation index, and found that 55 studies about AR were published between 2011 and 2016, which shows the increasing interest in this field. Another metanalysis based on 68 studies pointed out advantages and challenges of using AR in education (Akçayır and Akçayır, 2017). Indeed, despite the profusion of AR studies, the scientific results on the students’ learning do not fully converge. On the one hand, some studies have shown AR’s advantages, including collaborative learning (Bujak et al., 2013; Peramunugamage et al., 2023), autonomy (Amadieu and Tricot, 2014; Elmunsyah et al., 2019), improvement of learning by reducing cognitive load and mental effort (Chen and Huang, 2020; Yilmaz, 2023; Zhao et al., 2023), or modeling abstract concepts, such as astronomy (Fleck and Audran, 2016). On the other hand, AR’s has some disadvantages, such as attention problems (Radu, 2014) or the difficulty of using digital equipment in school conditions (Radu, 2014; Ali et al., 2022). Moreover, in the case of learning with new material, learners can experience a high cognitive load (Sawicka, 2008).
According of our knowledge, the use of AR 3D-interactive objects as inquiry-based modeling activity has been very rarely approached. Nevertheless, one of the principle affordance of AR environment during the investigation phase would be to give the opportunity to learners to manipulate and interact with the AR model to collect data and information (Wu et al., 2013; Pedaste et al., 2020). For instance, a recent research made a systematic review of articles about the use of AR in K12 inquiry-based learning (Pedaste et al., 2020) and only for few of them AR models were used to collect data in the investigation phase. Moreover, none of the studies among the Pedaste et al. review addressed the question of the mental effort during inquiry-based learning. Actually, we found studies addressing the impact of AR on cognitive load or mental effort during the inquiry process (e.g., Chiang et al., 2014), however none of them used AR as 3D interactive object for learners’ investigation but rather as scaffolding tools for supporting inquiry process.
Hence, the objective of this study was to investigate the possible effect of a first time-use 3D interactive AR model on university students’ mental effort and scientific learning outcomes during an inquiry-based modeling activity. During university training, we provide an immersive environment and a holistic approach to the earth-sun system. We expect an improvement in the students’ learning outcomes. However, the expectations could be balanced by an increasing mental effort of novices because of the introduction of new learning material (Van Bruggen et al., 2002; Hron and Friedrich, 2003; Sawicka, 2008; Wu et al., 2013).
Milgram and Kishino (1994) defined AR as a system that makes it possible to superimpose a virtual object on a real image. AR takes place in a Reality–Virtuality continuum (Figure 1), which is very close to the real environment side and can be considered as a composite view. On the opposite continuum side, the virtual environment is a computer-generated environment (which is also called virtual reality).
One of the main objectives of AR is to allow clear connections to be made between the real-world and abstract scientific concepts (Laine et al., 2016). AR allows natural interactions between students and learning objects, encouraging the creation of embodied representations of learning concepts. The cognitive dimension helps students to better symbolically understand the scaffolding of learning progression. This dimension is made possible thanks to the spatio-temporal alignment of information. Thus, the cognitive dimension improves the understanding of abstract concepts (Bujak et al., 2013). Moreover, AR creates opportunities for collaborative learning around virtual content and in a non-traditional environment (Bujak et al., 2013; Martín-Gutiérrez et al., 2015; Peramunugamage et al., 2023). More recently, Chang et al. (2018) argued that AR technology can be used to teach scientific reasoning and promotes students’ positive attitudes toward scientific issues. AR improves students’ reflective skills in science lessons (Arici and Yilmaz, 2023). AR can also promote student autonomy by allowing an adaptation of the content for each student. Hence, teachers can adapt the support according to the learners’ demands and constraints, and their different learning processes (Amadieu and Tricot, 2014).
Several studies have highlighted the strong limitations of AR in teaching. For instance, Huang et al. (2016) and Wang (2017) showed that the students’ learning performances are better when AR is coupled to more traditional learning support. Furthermore, a clear negative impact of AR has been also highlighted. Some studies that have used a new learning environment have found that the AR-based learning approach fostered the cognitive overload of students (Van Bruggen et al., 2002; Hron and Friedrich, 2003; Ali et al., 2022). Indeed, cognitive overload corresponds to a mental state in which a student is engaged in a task that is very demanding. In this case, the student does not have sufficient cognitive resources to perform the task easily. This demanding cognitive task requires the student to devote a great deal of attention, to take longer to complete a task with risk of making a lot of mistakes (Tricot, 2023). AR could be considered as a deleterious tool because of the cognitive overload generated using this new technology related to the complex tasks they perform (Wu et al., 2013). According to Dugas (2018), some elements still need to be improved before the generalization of this type of technology. The hardware may have some technical limitations, or the interface may lack ergonomics or adaptation that can sometimes lead to visual and cognitive overload. The way the pictures are displayed (arrangement in the operator’s visual field, ergonomics of the symbols, etc.) is crucial to achieve efficient performance for learners. If the technology mediates too much or too less information, the performance of the participants could be impacted (Alexander et al., 2005). Students can be cognitively overload by the related learning-environment information and by the complexity of the tasks that they have to complete (Wu et al., 2013; Ali et al., 2022; Buchner et al., 2022). Nevertheless; the use of guidance in AR animations is important for increasing students learning and reducing their cognitive load (Yilmaz, 2023). Other findings have outlined that AR learning can generate attention issues, showing that the learners’ attention is more focused on the AR system than on the content knowledge (Tang et al., 2003; Morrison et al., 2009; Radu, 2014).
Many researchers consider that mental effort is a good indicator for tracking cognitive load (Paas and Van Merriënboer, 1994; Antonenko and Niederhauser, 2010; Haji et al., 2015; Yilmaz, 2023). Mental effort and cognitive load are two close concepts that are rooted in Sweller’s cognitive load theory Sweller (1988). The objective of this theory is to understand the mechanisms that are involved in school performance differences in learning tasks. According to the classical architectures of the cognitive system, cognitive load theory describes how the limited resources of working memory are used when the cognitive system is in a learning situation (Sweller, 1988; Kirschner, 2002; Chen et al., 2018). Sweller (1988) distinguishes three cognitive load plans: first, the unnecessary extrinsic cognitive load corresponds to the organization of learning materials; second, the intrinsic cognitive load corresponds to the interaction between the cognitive task to be performed and the hardware to be used; and finally, the relevant cognitive load corresponds to the efforts invested in the construction of cognitive schemas. Although the assessment of mental effort fails to distinguish Sweller’s three dimensions, this kind of subjective rating scale is efficient for reflecting general cognitive processes (Ouwehand et al., 2021).
The inquiry-based learning is defined as a process who highlights new causals relations that can be likened to problem solving. The students formulating hypothesis and testing them before concluding (Pedaste et al., 2015). It involves students in a usually complex process of scientific discovery, in a process divided into logically connected parts, allowing the student to be guided through the main features of the method. These parts are called inquiry phases and together they form an inquiry cycle (Pedaste et al., 2015).
Based on a metanalysis of 32 articles, Pedaste et al. (2015) analyzed the inquiry processes addressed in the scientific literature. Despite the variety of the inquiry-based learning approach, they built an inquiry-based learning framework including five general inquiry phases common to the majority of the analyzed articles. This framework is seen as a circle including many relationships and feedback loops between the different phases. However, it can be summarized as follow:
i. Orientation defined as the phase fostering the learners’ curiosity and the statement of the problem to be solved.
ii. Conceptualization is the phase of the generation of research questions and hypotheses.
iii. Investigation phase allowing to explore or experiment, to collect and analyze data based on the designed protocol
iv. Conclusion phase allows to note findings and compare them with the tested hypotheses. At the end it is possible to identify the main findings extract from the data analysis.
v. Discussion is a transversal phase including communication and reflexion. Communication is defined as the process of debate, discuss or share with others any information seen during the other phases of the inquiry. Reflection is connected to the idea that the learners have to make sense about what they are learning during the different phases of the inquiry circle. To support this self-reflection, guiding questions can be seen as an effective scaffolding tool (Runnel et al., 2013).
One of the reasons why inquiry-based learning is increasingly present in education is that its success can be greatly enhanced by recent technical developments that allow the enquiry process to be supported by electronic learning environments (Pedaste et al., 2015). In our context, the French school curriculum promotes the inquiry approach. The teaching scenario proposed in this study is based on the inquiry model drawn up by Pedaste et al. (2015) and fits with the recommendation of the French school institution.
Despite the large number of studies on the effect of AR on mental effort and learner performance, to our knowledge very few of the study has investigated the impact of AR modeling activities in inquiry-based approach. Hence, this study aims to contribute to the exploration of the AR technology’s effectiveness on mental effort and learning outcomes in this specific educational approach. In particular, this study attempts to answer the following questions:
1.To what extent is mental effort impacted by AR during inquiry-based science training (about seasonal phenomena)?
2.How does AR impact the students short-term and long-term learning outcomes?
3.How does mental effort during learning tasks influence scientific learning outcomes?
According to the literature review, AR technology was mainly reported as beneficial for the learner’s mental effort and learning outcomes, even if there is a lack of knowledge about the specific case of AR modeling activities during inquiry-based training. Moreover, an alternative hypothesis emerged from the previous studies, as follows: when technology is used by the learners for the first time, then the learning effect could be limited or may even be negatively impacted.
An experimental protocol has been set up to address the three research questions. The sample consisted of 33 first year Master’s degree students (27 years old, ± 7.5 on average) from the Aix-Marseille University. The students were all engaged in this Master’s degree to be primary school teachers. They are all involved in a training program devoted to the development of their teaching skills and scientific knowledge through inquiry approaches. And all the students agreed to take part in the research process. Since the research objectives fit with training program objectives, the experiment took place during a usual face-to-face science lesson for 90 min and the training was animated by the usual science trainer.
The students were randomly assigned to one of two groups. The control group (n = 17) learned with different physical modeling technologies (e.g., terrestrial globes, torch light, diagrams, pictures), while the experimental group (n = 16) learned with the AR-learning system (i.e., an interactive 3D representation of the Earth-Sun system). The experimental group used only the mobile application ARTEfac (AR for Teachers Education Faculties) to learn the scientific concepts. ARTEfac (Aix-Marseille-Université, 2020) is a free mobile learning app developed by Aix-Marseille University where it is possible for trainers to create simple multimedia mobile learning scenarios or to customize already built-in 3D interactive models developed by researchers, university science trainers and programmers (for more information see: Mascret et al., 2023). The Earth-Sun AR model is one of the already built-in models that we have designed and integrated to the experimental learning scenario. Following the suggested affordances by the literature (Wu et al., 2013; Pedaste et al., 2020), the Earth-Sun AR model was used at the investigation phase and was thought for exploring the model with the possibility to change parameters and observe the consequences on the Earth-Sun system (for instance change the Earth’s tilt).
The experimental group had the opportunity to use an interactive AR 3D-model of the Earth-Sun system (Figure 2A). The AR-based model allowed the students to interact with the Earth-Sun system by changing different parameters through a button-menu (Figure 2B). This marker-based AR modeling activity has been developed in collaboration with university science trainers, researchers in science education and specialists in AR object design from a private company. This model has been thought specifically to be used with university students. However, for any digital learning object, it is possible to reuse it in multiple contexts depending of the learning scenario and objectives targeted. For instance, some users of ARTEfac who are mainly teachers, created learning scenarios integrating the AR Earth-sun system and used it with primary school students.
Figure 2. Screenshot of the AR scenario of the Earth-Sun system: (A) interactive model of the Earth-Sun system and (B) the menu to manipulate the model.
The experimental group used 10-inch tablets to run the application. The AR model was triggered by contrast recognition (a black and white piece of paper was used). The different buttons in the menu gave the students the opportunity to change various parameters, such as: show the light beams, show the Sun’s rays, show the trajectory, starting rotation and revolution of the Earth, increase the speed of rotation and revolution and modify the Earth’s tilt (Figure 2B). The AR model has been used during the investigation phase of the inquiry circle. To drive the students during the learning activity, a worksheet has been given to all the students helping them to take some notes about the inquiry activity and foster the reflective process engaged in the discussion phase of the inquiry circle. The learners were invited to answer questions focusing on the main scientific concepts linked to the phenomenon of the seasons, which were included in the worksheet.
During the activity, learners approached the following concepts that are necessary to understand the phenomenon of seasons: first, tilt (Earth’s axis of rotation, light beams) through the variation of the tilt of the Earth’s axis of rotation; second, exposure time (presence of the Sun on the Earth’s surface); third, solstices (fall, winter); fourth, hemispheres (north, south); and finally, energy of the surface.
For the control group, the students manipulated the traditional material used in science courses. The material included globes, flashlights and documents. The documents were mainly pictures and drawings, which have been selected to illustrate the concepts that the students had to learn (e.g., two drawings of the June solstice showing the polar night on the south pole and the midnight sun on the north pole). The documents have been chosen to specifically help the students to complete the worksheet, which was focused on the main scientific concepts linked to the phenomenon of the seasons. The control group’s worksheet was identical to the experimental worksheet (except on some few indications related to the use of the AR model). In short, both groups (control and experimental) followed a very similar inquiry-based science education training about the phenomenon of the seasons.
The learning outcomes have been measured using three identical tests, as follows: pre-test, short-term test and long-term test. They were developed based on the objectives of the training, which was to understand the phenomenon of the seasons, the Earth-Sun system and their interactions.
The learning outcomes test was divided into 11 closed-ended multiple-choice items (four possible answers). For each item, the students had to choose among: one correct answer, two incorrect answers and one “I do not know” answer (Figure 3). We expected that the neutral answer would limit the random answers. The learning outcome total score is calculated out of 11 points and coded as follows: correct = +1, incorrect = 0 and neutral = 0. The rational for the learning outcome test designed was based on the analysis of the knowledge about the phenomenon of seasons that students should acquire by the end of the training session.
Figure 3. Learning outcomes assessment technology, items 1 and 2 as examples (English translation from French).
To measure the students’ mental effort during the learning activities described in the worksheet, 10 Likert scale items have been used to evaluate their perceived mental effort. The students were led to choose the load level among seven possibilities: level 1 corresponds to the lowest mental effort, where the mental effort to achieve the worksheet tasks is very low; while level 7 corresponds to the highest mental effort, the mental effort is considered by learners as very high (Figure 4). The mental effort total score is calculated out of seven points and coded as 1 to 7.
Figure 4. On-task self-assessment mental effort assessment test (perceived mental effort), example of the first item (English translation from French).
This test was adapted from El Hader (2016), Mattis (2015), and Schmeck et al. (2015), assuming that the students’ on-task self-reported mental effort is directly linked to their cognitive load (Paas and Van Merriënboer, 1994; Tuovinen and Sweller, 1999; Kalyuga et al., 2001; Paas et al., 2003).
The researchers and trainer paid particular attention to provide at maximum the same information to the experimental and control groups during the training session. In this way, the trainer (who was the same in both groups, both experimental and control) began by orally announcing the instructions and objectives of the training session before starting. The students were led to make the link between phenomena observed on the Earth and the different parameters, such as the Earth’s rotation and revolution through an inquiry scenario.
More precisely, the training session has been designed on the inquiry leaning principles, respecting the five inquiry phases (Pedaste et al., 2015). During the Orientation phase, the trainer introduced the topic by using images and graphs about the variation of temperature in Europe. The conceptualization was led by the trainer fostering the setup of learners’ inquiry questions and hypothesis. It was the time of the emergence of very common misconceptions such as: the seasons are controlled by the distance between the earth and the sun with the earth farther from the sun in winter than in summer. The Investigation phase was mainly based on the physical or AR modeling in order to explore, observe and interpret the evidences and scaffolded by the worksheet. The only difference between the control and experimental group approaches is rooted in the investigation phase using either the physical modeling or the AR modeling activities. The Conclusion phase was focused on the learners’ main knowledge outcomes about the seasonal phenomenon that they have to retain. The discussions within and between the groups of students were held all along the previous phases. Students communicated and justified their ideas/findings/decisions with the help of the trainer all along the phases of the training session.
The training session lasted 90 and 10 min were necessary to fill-in the retention test 30 days after the training session. At the very beginning of the training session, the students started by filling-in a pre-test to assess their knowledge of the subject. They then participated in the activities about the Earth-Sun system. Finally, they had to complete a short-term test just after the training session and a long-term test 30 days after the session (all were similar to the pre-test). The experimental protocol is illustrated in Figure 5.
Figure 5. Summary of the protocol: difference and similarity between control and experimental groups.
Each task, guided by the worksheet, was identical for the control and the experimental group and was evaluated through the mental effort test that was filled-in individually. During the training session, the students were grouped by four or five individuals using one or two tablets in each group. The trainer moved between the groups to ensure a good understanding of the given tasks. The control group used hands-on materials, whereas the experimental group, were introduced to manipulate the AR model. To guide students across the understanding of the Earth-Sun system, they had to complete 10 different tasks (identical for control and experimental groups) that were described in the worksheet. This study was approved by the ethics committee of Aix-Marseille Université (no: 2022-03-10-003).
The students used the proposed AR model for the very first time during this study.
Quantitative dependent variables such as the mental effort and learning outcomes that were obtained in the pre-test, short-term test and long-term tests do not meet the assumption of normality (Shapiro–Wilk test; p < 0.05). Thus, non-parametric tests have been used for this study.
To answer the first research question (To what extent is the mental effort is impacted by AR?), an unpaired two-samples Wilcoxon test comparing the two groups’ mental effort scores was performed. To address the second research question (How does AR impact the students short-term and long-term learning outcome), an unpaired Wilcoxon test was performed to compare the learning outcome scores (post and retention) of both groups. To investigate the results and compare the evolution of scores in each group (experimental or control), paired samples Wilcoxon test have been performed. Furthermore, Spearman correlation tests were performed to analyze how mental effort can be related to learning outcome performances (research question 3: How does mental effort during learning tasks may influence scientific learning).
The significance level is considered at p = 0.05 or lower. More specifically, significant results have been interpreted as follows: p < 0.05, significant; p < 0.01, strongly significant; p < 0.001, very strongly significant; and p > 0.05, not significant. All of the statistical analyses were computed through the use of the R software V. 4.1.3 (R Core Team, 2020) using the Rcmdr package V. 2.8 (Fox and Bouchet-Valat, 2022).
Figure 6 illustrates that there were no significant differences in the mental effort scores between the experimental group and the control group. The average score for the experimental group is 4 (out of 1 to 7), SD = 1. The scores obtained by the control group is 4 (out of 1 to 7), SD = 1.2. There are no significant differences between the two groups [Wilcoxon test: T (16) = 62; p = 0.76].
Figure 6. Comparison of mental effort scores of the experimental and control groups. The horizontal bars represent inter-individual medians, the amplitude of the rectangles represent the 25–75% deviation of the individual data and the vertical bars represent the min-max deviations of the individual data.
As shown in Figure 7, the learning outcome scores increased for both groups from pre to short-term tests and from pre to long-term tests. The scores obtained for pre-test, short-term test and long-term test were, respectively: 2 (SD = 3), 8.5 (SD = 2), 4.4 (SD = 3) for the experimental group and 1 (SD = 4), 10 (SD = 3), 6 (SD = 5) for the control group. The paired Wilcoxon test underlines that the variations of both groups across the pre, short- and long-term tests are significant: first, experimental group (pre-test/short-term test: T (16) = 0, p = 0.0006; pre-test/long-term test: T (14) = 11.5, p = 0.01; short-term test/long-term test: T (16) = 2.5, p = 0.0007); second control group (pre-test/short-term test: T (17) = 0, p = 0.0003; pre-test/long-term test: T (17) = 4, p = 0.00059; short-term test/long-term test: T (16) = 6, p = 0.001). Not surprisingly, both groups’ modalities have a positive impact on short- and long-term learning outcome scores. Thus, test scores are significantly higher in short-term tests (experimental group = 8.5; control group = 10) than in the pre-tests (experimental group = 2; control group = 1) and long-term tests (experimental group = 4.4; control group = 6).
Figure 7. Comparison of the learning outcomes’ scores of pre-test, short-term test and long-term test for the experimental and control groups. The horizontal bars represent the inter-individual medians, the amplitude of the rectangles represent the 25–75% deviation of the individual data and the vertical bars represent the min-max deviations of the individual data. (*p < 0.05; **p < 0.01; ***p < 0.001).
A comparison of the learning outcomes’ scores of both groups showed no significant difference for pre-and long-term scores (pre-test: H (1.33) = 1.4; p = 0.24; long-term test: H (1.33) = 2.18; p = 0.14, unpaired two-samples Wilcoxon). However, the control group significantly outperformed the experimental group in the short-term test score, showing a short-term negative effect of the AR on learning outcomes [H (1.33) = 5.64; p = 0.017]. This result suggests that RA can negatively impact knowledge in the short-term.
Figure 8 highlights that there was a negative correlation between the scores obtained on short-term test and long-term test, and the mental effort scores.
Figure 8. Scatter plot of the learning outcome scores and mental effort scores based on the 33 participants (control and experimental groups). Correlations have been calculated for learning mental effort scores and pre-, short- test and long- tests’ scores (*p < 0.05).
Although a moderate negative correlation between the pre-test scores and mental effort (r = −0.31; p = 0.077) has been detected, the correlation is not significant but close to the significance level of 0.05. The lack of correlation between short-term test and mental effort scores is clearer (r = −0.14; p = 0.44). A significant moderate negative correlation between mental effort scores and long-term learning outcomes’ scores is highlighted (r = −0.38; p = 0.031). Indeed, a link between mental effort and pre-test and shorts-term test is not underlined within the total sample. However, the correlation between mental effort and long-term test scores is significant. In other words, the long-term learning outcomes score is lower when the mental effort is higher, and vice versa.
The objective of this study was to investigate the impact of the AR on mental effort and learning outcomes scores in a learning context. Based on Sweller’s cognitive load theory Sweller (1988), we expected that AR would not increase the students’ mental effort and consequently impact negatively their learning outcomes in the short and long term. Indeed, because all of the students used this AR model for the first time, the risk of increasing mental effort was high. By analyzing the metal effort scores of both groups (i.e., experimental and control) we investigated the first research question (To what extent is the mental effort is impacted by AR during a science training learning?). Interestingly, our results based on the self-assessment metal effort test do not differ between the experimental group (using AR) and the control group. Even though the AR model was used for the first time, it was possible to drastically limit the mental effort (at least at the same level when not using AR models). In contrast to earlier findings (Van Bruggen et al., 2002; Hron and Friedrich, 2003; Sawicka, 2008; Wu et al., 2013), we find that using new learning material such as an AR model does not increase the on-task perceived mental effort during the investigation phase of the inquiry learning process.
The second research question (How does AR impact the students short-term and long-term learning outcome?) was investigated by analyzing the learning outcome scores of the experimental and control group. No significant difference was found by comparing the experimental group (with AR) and the control group (without AR) regarding long-term learning outcomes. Nevertheless, significant results were highlighted with better short-term learning outcome scores for the control group. Accordingly, it can be hypothesized that this lack of positive effect on learning can be attributed to a knowledge related loss of attention (Tang et al., 2003; Morrison et al., 2009; Radu, 2014). Our results demonstrate that the use of AR helps the students to focus on the pre-tests AR modeling task at the expense of the related content knowledge without impacting the perceived mental effort. Thus, we believe that a more specific guidance for the use of the AR model would be very helpful for the AR users by making the related knowledge more explicit, especially when they use the AR model for the first time. The construction of knowledge from learning activities without any guidance is possible, but the amount of guidance received has a direct influence on the student’s understanding of the concept that is addressed (Kirschner et al., 2006; Siméone et al., 2007; Moli et al., 2017).
We investigated the last research question (How does mental effort during learning tasks may influence scientific learning outcomes?) by investigating the correlation between learning outcome scores and mental effort score. Our findings revealed that the long-term learning outcome scores are low when the students’ mental effort is high (independently of group conditions, control or experimental), and vice versa. In other words, there is a link between learning mental effort and the long-term learning outcome score for the experimental and control groups. When the mental effort is higher, the long-term learning outcome score is lower. Conversely, when mental effort is lower, the long-term learning outcome score is higher. The correlation between pre-test learning outcomes and mental effort is close to significant. During the learning session activities, students who have better knowledge of the scientific subject are logically less cognitively loaded than the other students during the learning activities, and vice versa. No correlation was found between short-term test and mental effort, which shows that mental effort level preferentially impacted long-term outcomes. Our experiments are in line with previous results that suggest a correlation with long terms outcomes (Örün and Akbulut, 2019).
We are aware that our research may have three limitations. The first limitation is the restricted number of participants in this study, which would explain why some findings are unclear (type II error may occur). Non-parametric statistical tests limited our ability to detect significant differences. To support the results, it would be interesting to extend the sample size and perform parametric tests. The second limitation is the use of an indirect measure of mental effort by using a mental effort test during the training session. However, this way of assessing mental effort has already shown satisfying results (Paas and Van Merriënboer, 1994; Kalyuga et al., 2001). The duration of empirical study may be seen as short. However, this duration is the one usually devoted to study the phenomenon of the seasons with student teachers. Spending more time on seasonal phenomenon at the expense of other scientific topics is inappropriate considering the respect of the provided science teaching quality for the master students engaged in this study.
The third limitation is due to the instrument for assessing participants’ mental effort. It is clear that self-reported measures may be very different form direct measure in the sense of possible lack of correlation between perception of mental effort and mental effort (Yates et al., 2022).
Both AR model and physical model represent valuable educational materials in inquiry approach of learning, especially during the investigation phase. Both have established clear improvements in short- and long-term learning outcomes about seasonal phenomenon in a very equivalent way (specifically considering learning outcomes and mental effort), except at the short-term learning score which was slightly better for the control group.
The datasets presented in this article are not readily available because Due to the nature of this research, participants of this study did not agree for their data to be shared publicly, so supporting data is not available. Requests to access the datasets should be directed to ZWxlbmEubWFydGluQHVuaXYtYW11LmZy.
The studies involving human participants were reviewed and approved by Aix-Marseille Université (n°: 2022-03-10-003). The patients/participants provided their written informed consent to participate in this study.
EM, JC, HC-A, and PB-P contributed to conception and design of the study. EM organized the database and wrote the first draft of the manuscript. EM and JC performed the statistical analysis. JC wrote sections of the manuscript. All authors contributed to manuscript revision, read, and approved the submitted version.
This work was supported by the Excellence Initiative of Aix-Marseille University - A*MIDEX, under Grant IDEX N°AAP-2017-AE-57 and the French Ministry of Higher Education, Research and Innovation under the Doctoral Grant. This work was carried out within the pilot Centre Ampiric, which is funded by the French State’s Future Investment Program (PIA3/France Relance) as part of the “Territories of Educational Innovation” action.
The authors declare that the research was conducted in the absence of any commercial or financial relationships that could be construed as a potential conflict of interest.
All claims expressed in this article are solely those of the authors and do not necessarily represent those of their affiliated organizations, or those of the publisher, the editors and the reviewers. Any product that may be evaluated in this article, or claim that may be made by its manufacturer, is not guaranteed or endorsed by the publisher.
Aix-Marseille-Université. (2020). ARTEfac (version 1.2.6) [Mobile app]. Google play store and app store. Available at: https://artefac.univ-amu.fr/ (Accessed July 20, 2023).
Akçayır, M., and Akçayır, G. (2017). Advantages and challenges associated with augmented reality for education: a systematic review of the literature. Educ. Res. Rev. 20, 1–11. doi: 10.1016/j.edurev.2016.11.002
Alexander, A. L., Wickens, C. D., and Hardy, T. J. (2005). Synthetic vision systems: the effects of guidance symbology, display size, and field of view. Hum. Factors 47, 693–707. doi: 10.1518/001872005775571005
Ali, N. A., Sadiq, M. H., Albabawat, A. A., and Salah, R. M. (2022). Methods and applications of augmented reality in education: a review. Int. Conf. Comp. Sci. Softw. Eng. 2022, 175–181. doi: 10.1109/CSASE51777.2022.9759807
Amadieu, F., and Tricot, A. (2014). Apprendre avec le numérique: Mythes et réalités [learning with digital: Myths and realities]. France: Retz.
Antonenko, P. D., and Niederhauser, D. S. (2010). The influence of leads on cognitive load and learning in a hypertext environment. Comput. Hum. Behav. 26, 140–150. doi: 10.1016/j.chb.2009.10.014
Arici, F., and Yilmaz, M. (2023). An examination of the effectiveness of problem-based learning method supported by augmented reality in science education. J. Comput. Assist. Learn. 39, 446–476. doi: 10.1111/jcal.12752
Buchner, J., Buntins, K., and Kerres, M. (2022). The impact of augmented reality on cognitive load and performance: a systematic review. J. Comput. Assist. Learn. 38, 285–303. doi: 10.1111/jcal.12617
Bujak, K. R., Radu, I., Catrambone, R., MacIntyre, B., Zheng, R., and Golubski, G. (2013). A psychological perspective on augmented reality in the mathematics classroom. Comput. Educ. 68, 536–544. doi: 10.1016/j.compedu.2013.02.017
Chang, H.-Y., Hsu, Y.-S., Wu, H.-K., and Tsai, C.-C. (2018). Students’ development of socio-scientific reasoning in a Mobile augmented reality learning environment. Int. J. Sci. Educ. 40, 1410–1431. doi: 10.1080/09500693.2018.1480075
Chen, O., Castro-Alonso, J. C., Paas, J., and Sweller, J. (2018). Extending cognitive load theory to incorporate working memory resource depletion: evidence from the spacing effect. Educ. Psychol. Rev. 30, 483–501. doi: 10.1007/s10648-017-9426-2
Chen, C.-C., and Huang, P.-H. (2020). The effects of STEAM-based mobile learning on learning achievement and cognitive load. Interact. Learn. Environ. 31, 100–116. doi: 10.1080/10494820.2020.1761838
Chen, P., Liu, X., Cheng, W., and Huang, R. (2017). “A review of using augmented reality in education from 2011 to 2016” in Innovations in smart learning. eds. E. Popescu, M. K. K. Kinshuk, R. Huang, M. Jemni, N.-S. Chen, and D. G. Sampson (Singapore: Lecture Notes in Educational Technology; Springer), 13–18.
Chiang, T. H., Yang, S. J., and Hwang, G.-J. (2014). An augmented reality-based mobile learningsystem to improve students’ learning achievements and motivations in natural science inquiry activities. J. Educ. Technol. Soc. 17, 352–365.
Cuendet, S., Bonnard, Q., Do-Lenh, S., and Dillenbourg, P. (2013). Designing augmented reality for the classroom. Comput. Educ. 68, 557–569. doi: 10.1016/j.compedu.2013.02.015
Di Serio, Á., Ibáñez, M. B., and Delgado Kloos, C. (2013). Impact of an augmented reality system on students’ motivation for a visual art course. Comput. Educ. 68, 586–596. doi: 10.1016/j.compedu.2012.03.002
Dugas, J. (2018). La réalité augmentée dans un contexte d’apprentissage: bibliographie thématique commentée. [Augmented reality in a learning context: an annotated thematic bibliography.] Adjectif.net. Mis en ligne mercredi 7 novembre 2018 [En ligne] Available at: http://www.adjectif.net/spip/spip.php?article480 (Accessed July 20, 2023).
El Hader, C. (2016). “L'effet du guidage dans l'environnement GeoGebra et au niveau du raisonnement déductif: une propédeutique à la résolution des problèmes de démonstration de géométrie plane en 6e dans les écoles libanaises francophones homologuées.” [The effect of guidance in the GeoGebra environment and at the level of deductive reasoning: a propaedeutic to the solution of plane geometry demonstration problems in 6th grade in French-speaking Lebanese accredited schools] Thèse de doctorat., Université d’Aix-Marseille.
Elmunsyah, H., Hidayat, W. N., and Asfani, K. (2019). Interactive learning media innovation: utilization of augmented reality and pop-up book to improve user’s learning autonomy. J. Phys. Conf. Ser. 1193:012031. doi: 10.1088/1742-6596/1193/1/012031
Fleck, S., and Audran, J. (2016). Réalité augmentée et interfaces tangibles, quels liens avec la formation par simulation? [augmented reality and tangible interfaces, what links whith simulation training?]. Recherche et formation 82:Art. 82.
Haji, F. A., Rojas, D., Childs, R., de Ribaupierre, S., and Dubrowski, A. (2015). Measuring cognitive load: performance, mental effort and simulation task complexity. Med. Educ. 49, 815–827. doi: 10.1111/medu.12773
Hron, A., and Friedrich, H. F. (2003). A review of web-based collaborative learning: factors beyond technology. J. Comput. Assist. Learn. 19, 70–79. doi: 10.1046/j.0266-4909.2002.00007.x
Huang, T.-C., Chen, C.-C., and Chou, Y.-W. (2016). Animating eco-education: to see, feel, and discover in an augmented reality-based experiential learning environment. Comput. Educ. 96, 72–82. doi: 10.1016/j.compedu.2016.02.008
Kalyuga, S., Chandler, P., Tuovinen, J., and Sweller, J. (2001). When problem solving is superior to studying worked examples. J. Educ. Psychol. 93, 579–588. doi: 10.1037/0022-0663.93.3.579
Kirschner, P. A. (2002). Cognitive load theory: implications of cognitive load theory on the Design of Learning. Learn. Instr. 12, 1–10. doi: 10.1016/S0959-4752(01)00014-7
Kirschner, P. A., Sweller, J., and Clark, R. E. (2006). Why Minimal Guidance During Instruction Does Not Work: An Analysis of the Failure of Constructivist, Discovery, Problem-Based, Experiential, and Inquiry-Based Teaching. Educational Psychologist 41, 75–86. doi: 10.1207/s15326985ep4102_1
Laine, T. H., Nygren, E., Dirin, A., and Suk, H.-J. (2016). Science spots AR: a platform for science learning games with augmented reality. Educ. Technol. Res. Dev. 64, 507–531. doi: 10.1007/s11423-015-9419-0
Martín-Gutiérrez, J., Fabiani, P., Benesova, W., Meneses, M. D., and Mora, C. E. (2015). Augmented reality to promote collaborative and autonomous learning in higher education. Comp. Hum. Behav. 51, 752–761. doi: 10.1016/j.chb.2014.11.093
Mascret, N., Marlin, K., Laisney, P., Castéra, J., and Brandt-Pomares, P. (2023). Teachers’ acceptance of an open-source, collaborative, free m-learning app: the predictive role of teachers’ self-approach goals. Educ. Inf. Technol. doi: 10.1007/s10639-023-11832-3
Mattis, K. V. (2015). Flipped classroom versus traditional textbook instruction: assessing accuracy and mental effort at different levels of mathematical complexity. Technol. Knowl. Learn. 20, 231–248. doi: 10.1007/s10758-014-9238-0
Milgram, P., and Kishino, F. (1994). A taxonomy of mixed reality visual displays. Inform. Syst. E77-D, 1321–1329.
Moli, L., Pedregosa Delserieys, A., Impedovo, M. A., and Castéra, J. (2017). Learning density in Vanuatu high school with computer simulation: influence of different levels of guidance. Educ. Inf. Technol. 22, 1947–1964. doi: 10.1007/s10639-016-9527-4
Morrison, A., Oulasvirta, A., Peltonen, P., Lemmela, S., Jacucci, G., Reitmayr, G., et al. (2009). Like bees around the hive: a comparative study of a Mobile augmented reality map. In Proceedings of the SIGCHI conference on human factors in computing systems, 1889–1898. CHI ‘09. New York, NY, USA: Association for Computing Machinery.
Örün, Ö., and Akbulut, Y. (2019). Effect of multitasking, physical environment and electroencephalography use on cognitive load and retention. Comput. Hum. Behav. 92, 216–229. doi: 10.1016/j.chb.2018.11.027
Ouwehand, K., Van der Kroef, A., Wong, J., and Paas, F. (2021). Measuring cognitive load: are there more valid alternatives to Likert rating scales? Front. Educ. 6, 146–158. doi: 10.3389/feduc.2021.702616
Paas, F., Tuovinen, J. E., Tabbers, H., and Van Gerven, P. W. M. (2003). Cognitive load measurement as a means to advance cognitive load theory. Educ. Psychol. 38, 63–71. doi: 10.1207/S15326985EP3801_8
Paas, F. G. W. C., and Van Merriënboer, J. J. G. (1994). Variability of worked examples and transfer of geometrical problem-solving skills: a cognitive-load approach. J. Educ. Psychol. 86, 122–133. doi: 10.1037/0022-0663.86.1.122
Pedaste, M., Mäeots, M., Siiman, L. A., De Jong, T., Van Riesen, S. A., Kamp, E. T., et al. (2015). Phases of inquiry-based learning: definitions and the inquiry cycle. Educ. Res. Rev. 14, 47–61. doi: 10.1016/j.edurev.2015.02.003
Pedaste, M., Mitt, G., and Jürivete, T. (2020). What is the effect of using mobile augmented reality in K12 inquiry-based learning? Educ. Sci. 10:94. doi: 10.3390/educsci10040094
Peramunugamage, A., Ratnayake, U. W., and Karunanayaka, S. P. (2023). Systematic review on mobile collaborative learning for engineering education. J. Comp. Educ. 10, 83–106. doi: 10.1007/s40692-022-00223-1
R Core Team (2020). “R: A language and environment for statistical computing ” Vienna, Austria: R Foundation for Statistical Computing.
Radu, I. (2014). Augmented reality in education: a Meta-review and cross-media analysis. Pers. Ubiquit. Comput. 18, 1533–1543. doi: 10.1007/s00779-013-0747-y
Ruiz-Ariza, A., Casuso, R. A., Suarez-Manzano, S., and Martínez-López, E. J. (2018). Effect of augmented reality game Pokémon GO on cognitive performance and emotional intelligence in adolescent young. Comput. Educ. 116, 49–63. doi: 10.1016/j.compedu.2017.09.002
Runnel, M. I., Pedaste, M., and Leijen, Ä. (2013). Model for guiding reflection in the context of inquiry-based science education. J. Balt. Sci. Educ. 12, 107–118. doi: 10.33225/jbse/13.12.107
Sawicka, A. (2008). Dynamics of cognitive load theory: a model-based approach. Comp. Hum. Behav. Instruct. Supp. 24, 1041–1066. doi: 10.1016/j.chb.2007.03.007
Schmeck, A., Opfermann, M., van Gog, T., Paas, F., and Leutner, D. (2015). Measuring cognitive load with subjective rating scales during problem solving: differences between immediate and delayed ratings. Instr. Sci. 43, 93–114. doi: 10.1007/s11251-014-9328-3
Siméone, A., Eneau, J., and Rinck, F. (2007). Scénario d’apprentissage collaboratif à distance et en ligne: des compétences relationnelles sollicitées et /ou développées? Informations, Savoirs, Décisions et Médiations [Informations, Sciences for Decisions Making]. Available at: https://halshs.archives-ouvertes.fr/halshs-01565163. (Accessed July 20, 2023).
Sweller, J. (1988). Cognitive load during problem solving: effects on learning. Cogn. Sci. 12, 257–285. doi: 10.1016/0364-0213(88)90023-7
Tang, A., Owen, C., Biocca, F., and Mou, W. (2003). Comparative effectiveness of augmented reality in object assembly. In Proceedings of the SIGCHI Conference on Human factors in Computing Systems, 73–80. CHI ‘03. New York, NY, USA: Association for Computing Machinery.
Tricot, A. (2023). « Surcharge cognitive » [Cognitive overload], Encyclopædia Universalis [en ligne], consulté le 31 mai 2023. Available at: https://www.universalis.fr/encyclopedie/surcharge-cognitive/
Tuovinen, J. E., and Sweller, J. (1999). A comparison of cognitive load associated with discovery learning and worked examples. J. Educ. Psychol. 91, 334–341. doi: 10.1037/0022-0663.91.2.334
Van Bruggen, J. M., Kirschner, P. A., and Jochems, W. (2002). External representation of argumentation in CSCL and the Management of Cognitive Load. Learn. Instr. 12, 121–138. doi: 10.1016/S0959-4752(01)00019-6
Wang, Y.-H. (2017). Exploring the effectiveness of integrating augmented reality-based materials to support writing activities. Comput. Educ. 113, 162–176. doi: 10.1016/j.compedu.2017.04.013
Wu, H.-K., Wen-Yu Lee, S., Chang, H. Y., and Liang, J.-C. (2013). Current status, opportunities and challenges of augmented reality in education. Comput. Educ. 62, 41–49. doi: 10.1016/j.compedu.2012.10.024
Yates, N., Gough, S., and Brazil, V. (2022). Self-assessment: with all its limitations, why are we still measuring and teaching it? Lessons from a scoping review. Med. Teach. 44, 1296–1302. doi: 10.1080/0142159X.2022.2093704
Yilmaz, R. M. (2023). Effects of using cueing in instructional animations on learning and cognitive load level of elementary students in science education. Interact. Learn. Environ. 31, 1727–1741. doi: 10.1080/10494820.2020.1857784
Zhao, G., Zhang, L., Chu, J., Zhu, W., Hu, B., He, H., et al. (2023). An augmented reality based Mobile photography application to improve learning gain, decrease cognitive load, and achieve better emotional state. International journal of human– computer. Interaction 39, 643–658. doi: 10.1080/10447318.2022.2041911
Keywords: augmented reality, mental effort, learning outcomes, higher education, inquiry-based learning
Citation: Martin E, Castéra J, Cheneval-Armand H and Brandt-Pomares P (2023) The use of augmented reality for inquiry-based activity about the phenomenon of seasons: effect on mental effort and learning outcomes. Front. Educ. 8:1223656. doi: 10.3389/feduc.2023.1223656
Received: 16 May 2023; Accepted: 17 July 2023;
Published: 28 July 2023.
Edited by:
Hadipurnawan Satria, Sriwijaya University, IndonesiaReviewed by:
Janika Leoste, Tallinn University, EstoniaCopyright © 2023 Martin, Castéra, Cheneval-Armand and Brandt-Pomares. This is an open-access article distributed under the terms of the Creative Commons Attribution License (CC BY). The use, distribution or reproduction in other forums is permitted, provided the original author(s) and the copyright owner(s) are credited and that the original publication in this journal is cited, in accordance with accepted academic practice. No use, distribution or reproduction is permitted which does not comply with these terms.
*Correspondence: Elena Martin, ZWxlbmEubWFydGluQHVuaXYtYW11LmZy
†ORCID: Jérémy Castéra https://orcid.org/0000-0003-1520-3620
Hélène Cheneval-Armand https://orcid.org/0000-0001-7554-537X
Pascale Brandt-Pomares https://orcid.org/0000-0003-0632-9803
Disclaimer: All claims expressed in this article are solely those of the authors and do not necessarily represent those of their affiliated organizations, or those of the publisher, the editors and the reviewers. Any product that may be evaluated in this article or claim that may be made by its manufacturer is not guaranteed or endorsed by the publisher.
Research integrity at Frontiers
Learn more about the work of our research integrity team to safeguard the quality of each article we publish.