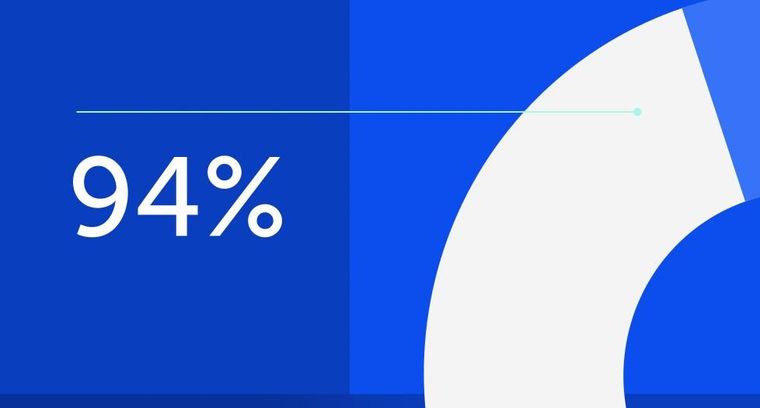
94% of researchers rate our articles as excellent or good
Learn more about the work of our research integrity team to safeguard the quality of each article we publish.
Find out more
SYSTEMATIC REVIEW article
Front. Educ., 13 September 2023
Sec. Digital Education
Volume 8 - 2023 | https://doi.org/10.3389/feduc.2023.1220688
Multimodal learning analytics (MMLA) has emerged as an encompassing approach to data collection, facilitating the analysis of student interactions across a variety of resources. MMLA capitalizes on data gleaned from diverse interactions, utilizing wearable devices to track physiological responses. This yields deeper insights into factors such as cognitive load, stress levels, interest, and other stimuli pivotal to the learning process. Nonetheless, it is crucial to acknowledge the theoretical and practical challenges underpinning the integration of wearable devices into learning experiences, both in academic settings and in everyday life activities. A systematic review of the literature (SLR) was conducted to identify the characteristics of studies that incorporate wearable devices into teaching-learning process analyses. The outcomes enabled us to discern key attributes such as participant descriptions, the activities implemented for data collection, and a broad spectrum of biometric indicators, with electrodermal activity (EDA) and heart rate (HR) among the most commonly employed methodologies in data analysis. Future endeavors should be centered on the formation of interdisciplinary teams. The objective is to devise novel methodologies for multimodal data collection and analysis that can discern performance variables, thereby enhancing learning in a manner conducive to more fluid, reflective educational experiences for all participants in the teaching-learning process.
The potential of learning analytics (LA) in education has been harnessed as a tool for the collection, measurement, and analysis of student data with the intent of understanding and constructing models that enhance learning experiences (Siemens and Long, 2011; Ferguson, 2012; Siemens and Gasevic, 2012). The data from LA have been utilized by various authors to explore and investigate factors that shape learning behavior in technology-mediated spaces (Lin and Hwang, 2018; Ullmann et al., 2019). Procedures carried out by institutions using learning analytics, which are based on clickstream data or the so-called time-stamped digital traces left by students during their interactions with the learning management system (LMS), have been recognized (Sedraz Silva et al., 2018). Given the ability to discern varied and intricate patterns regarding student behavior, the significance of other educational applications is also acknowledged (Cukurova et al., 2020). The potential of LMSs for early detection of students facing challenges in courses, predicting success, and promoting improved outcomes has been highlighted (Tempelaar et al., 2020).
Though LA has advanced the comprehension of students’ learning processes (Avella et al., 2016), its limitations stem from the one-dimensional nature of its data collection (Rodríguez-Triana et al., 2017). Crucial contextual student data, vital for understanding behaviors and influential factors in their learning process, are often omitted (Eradze and Laanpere, 2017). To address these limitations, multimodal learning analytics (MMLA) has been introduced as a comprehensive approach to data collection (Giannakos et al., 2022; Monsalves et al., 2023). MMLA is characterized by the study of data from diverse sources stemming from student interactions in a range of environments beyond just the digital; this includes physical, physiological, psychometric, and environmental spaces (Worsley et al., 2016; Mu et al., 2020; Giannakos et al., 2022). Such data are captured, amalgamated, and analyzed to provide a holistic understanding of factors associated with the learning process (Quadri and Shukor, 2021).
Specifically, technological advancements have paved the way for wearable devices in the realm of capturing multimodal data pertaining to the physiological environment. These advancements have also simplified the storage of vast amounts of data in the cloud, easing their collection and subsequent analysis (DiMitri et al., 2018). Wearable devices, especially multimodal computerized biofeedback wristbands, have become pivotal for real-time data collection (Garbarino et al., 2014; Regalia et al., 2019). Their widespread adoption is attributed to their increasing popularity in health tracking and monitoring (Vos et al., 2023). Notably, the Empatica E4 wristband, known for its noninvasive nature, cost-effectiveness, and cloud storage capabilities, is prominently utilized (Ollander et al., 2016; Koskimäki et al., 2017). This device has been employed in diverse studies encompassing music, stress, and emotion management (Borrego et al., 2019; Bulagang et al., 2021; Chandra et al., 2021; Rahman et al., 2021).
Recently, there has been considerable interest in creating multimodal systems that automatically analyze students’ states that are hard to observe directly, like cognitive load and stress levels, but can significantly affect their performance (Bustos-López et al., 2022). Some studies have focused on examining aspects such as self-regulation (Spann et al., 2017), motivation (Järvenoja et al., 2018), interest, and attention during activities (Tan et al., 2021). At this point, biometric data aids in managing learning-related information, such as assessment load (Hernandez-de-Menendez et al., 2021). Classroom biometric monitoring can usher in new learning approaches, as timely feedback to students with suitable teaching methods bolsters learning and retention (Ramírez-Moreno et al., 2021a,b).
Consequently, wearable devices provide a range of measurements to acquire biometric data, including physiological responses such as electrodermal activity (EDA), also referred to as galvanic skin response (GSR). This measurement is intimately linked to the sympathetic nervous system (SNS), as skin conductance primarily arises from sweat glands under the exclusive control of the SNS. EDA is widely recognized as a direct method to gauge stress associated with responses of the autonomic nervous system (ANS; Boucsein, 2012). In addition to this, these devices include other peripheral physiological measurements such as respiratory amplitude, skin temperature (ST), electrocardiogram (ECG), blood pressure volume (BVP), muscle electromyography (EMG), and electrooculogram (EOG; Koelstra et al., 2010).
Given the growing interest in wearable devices, it becomes imperative to undertake research in the field of education that addresses both the theoretical and practical issues and the current limitations of these tools. Consequently, this study attempts to pinpoint the characteristics of teaching-learning processes that integrate wearable devices for the collection of biometric data from students. The first section of the article provides an overview of the methodological strategy underpinning this research, based on a systematic literature review (SLR). The following section presents the results of the study, including analysis, interpretation, and findings. The final section addresses the central research question: What are the characteristics of studies that incorporate wearable devices into the analysis of teaching-learning processes?
The systematic review was conducted using the PRISMA (Preferred Reporting Items for Systematic Reviews and Meta-Analyses) guidelines (Page et al., 2021), facilitating the identification of potentially relevant articles. Extensive searches were performed in the Scopus and Web of Science (WoS) bibliographic databases to incorporate a wide range of scientific literature while excluding gray literature. Inclusion criteria were established to include peer-reviewed journal articles published in English between 2019 and 2022, spanning quantitative, qualitative, and mixed-methods studies. This approach ensured a comprehensive view of current research integrating wearable devices. Following the delineation of the study objectives, sub-research questions were subsequently defined to answer the main question (Table 1).
Consequently, the titles and abstracts of potential studies were scrutinized to ensure they met the predetermined eligibility criteria. Thorough reviews were then performed to confirm the relevance of the included information to the study, namely, whether it contributed sufficiently toward addressing the objectives of the present research. Full versions of the articles were subsequently assessed independently using the following criteria: (1) intervention studies examining the impact of wearable devices on learning processes; (2) studies incorporating at least one control group; and (3) peer-reviewed studies.
Data extraction from each database was independently executed by the authors. The principal metadata drawn from each study encompassed the following details: the first author, date and location of publication, authors’ affiliations, journal details, keywords, and the country where the study was conducted. Additionally, specific labels analyzed by the researchers for each study were included, such as the academic level of the study (elementary school, middle school, high school, college, or undergraduate) and the pedagogical strategy or activity utilized. This data was assembled in standard MS Excel files. Any discrepancies encountered were resolved through author discussion.
During the identification phase, a total of 85 records were initially recognized and subsequently imported for the construction of the database, sourced from Scopus (42) and Web of Science (43). With the use of Parsifal automation tools, these records were consolidated, resulting in the identification and removal of nine duplicates and six unsuitable records. This process yielded 70 articles for the subsequent screening phase. Nine articles written in Russian and Chinese were eliminated, leaving 61 reports for recovery. Of these, only 49 were evaluated for eligibility based on the application of selection criteria. Ultimately, the final review incorporated 30 articles that included the use of wearable devices. A depiction of this methodological process is provided in Figure 1.
The subsequent key findings were derived from the sub-research questions (RQ1). What is the research context that incorporates the use of wearable devices? (RQ2) Are wearable devices utilized to assess the development of any competency component, whether disciplinary or general? (RQ3) Which biometric indicators are associated with the use of wearable devices? (RQ4) What is the methodology for analyzing data gathered via wearable devices?
The context for the development of the selected studies was analyzed from two viewpoints. The first viewpoint encompasses the use of text mining to construct and visualize networks of co-occurring terms extracted from the chosen scientific literature. The second viewpoint pertains to descriptive variables, such as the academic level of the study and the employed pedagogical strategy or activity. Using VOSViewer software, terms drawn from the title and abstract fields of all articles were scrutinized employing a full-count method, where every occurrence of a term in a document is accounted for (Figure 2). Access to the interactive version is provided here: interactive bibliometric network.
The results of the terms linked to each database demonstrate the relationship between the variables that are part of the analyzed articles, where the key and relevant concepts of these studies are verified. The primary bibliometric network map generated a total strength of 1,347 links, which we classified into three clusters. The main findings identify the first cluster (colored in green) with 11 items and the highest number of occurrences (O), which indicates the number of documents in which a keyword occurs (55), links (L) (27), understood like a connection or a relation between two items, and total link strength (LS), which indicates the total strength of the co-authorship links of a given researcher with other researchers (176). It comprises terms that relate to the implementation and use of devices, such as activity, data, sensors, and research. The second cluster, highlighted in red, contains the concepts of anxiety, children, development, electrodermal activity, experience, learning, and user. The third cluster (blue) highlights the terms cognitive, case, test, person, and student.
The data amassed from the results of the biometric map enable the discernment of the interaction of the most pertinent constructs of the investigations. This is based on the data procured from the frequency of occurrence, links, and total strength of the data extracted from the titles and abstracts of each study. Notably, there is a conspicuous absence of terms that are connected with the constructs of pedagogical processes. However, the body of information provided by each cluster is underscored. In the green cluster, activities utilizing sensors to compute beats per minute (BPM) in various research activities are emphasized. Meanwhile, in the red and blue clusters, the student is recognized as the central figure and user in experiences that allude to physiological indicators such as the heart rate (HR).
Regarding the descriptive variables, Table 2 assembles information concerning the authors, the research objective, the methodology for data collection, the participant profiles, and the descriptions of activities involving wearable devices. It also includes biometric indicators, whose abbreviations are further detailed in Section 3.3.
From the preliminary investigation of the profiles of the participants, it was found that university students constituted the highest percentage (23.3%). In some of the studies, the characteristics of the participants were not mentioned (16.6%), while an equal percentage involved working with volunteers. Studies mentioned users involving children under 12 years of age (10%). The least represented participants were physicians (6%), office and industrial workers (6%), graduate students (3%), children with special educational needs (3%), and patients (3%).
Subsequent examination of portable device activities revealed that controlled studies and simulated activities were performed (33.3%). Virtual reality environments were involved (6.6%), as were activities in authentic contexts (13.3%), such as emergency rooms and courts. Other activities included activities of daily living (10%), games that integrate motion-based touchless games (MBTG), games with different ASRs (avatar representation), movement-based educational games (MBEG; 10%), pedagogical activities aimed at developing data analysis tools (6.6%), and standardized tests (3.3%).
In response to the second research sub-question (RQ2), competencies (C) are distinguished and classified into two categories: general (G) and specific (E). General competencies (G) are referenced as the extensive skill sets required across various professions, encompassing aspects like teamwork, working toward objectives, initiative, leadership, time management, and independence. The analyzed studies found that the development phase of these general competencies was in progress (60%). Specific or disciplinary competencies refer to skills unique to particular disciplines, such as using data analysis programs and programming abilities. In the collected studies, these competencies were exhibited through practices prevalent across different fields, such as the creation of technological tools and the analysis of physiological attributes and states like stress, mental processes, concentration, and memory (27%). Furthermore, it was noted that a segment of the studies could not be categorized (13.3%) owing to the lack of processes that were directly connected with the teaching-learning paradigm. In addition, information pertaining to the competency analysis was cross-verified with the country in which each study was conducted (Figure 3).
This analysis facilitated the understanding that the emphasis was predominantly on the development of general competencies. In terms of disciplinary competencies, they accounted for all studies conducted in Germany, followed by Australia (65%), Japan (50%), the United States (42%), and Spain (24%).
Specifically, in the studies that refer to formal educational contexts, the different educational levels are linked to specific pedagogical activities. Undergraduate-level studies (11) are primarily related to simulated activities such as controlled studies (45%), virtual reality environments (9%), and activities in a real context (9%), among others. The elementary level (three studies) all linked games with different ASR (avatar representation); motion-based educational games (MBEG) and high school technologies defined a study with controlled variables. On the other hand, studies linked to non-educational contexts involved daily life activities (25%), real-world contextual activities (18%), the development of data analysis tools (8%), and the use of controlled environments with virtual reality (7%).
The biometric indicators gleaned from the studies relevant to this analysis are depicted (Figure 4). These biomarkers are highlighted on the left side of the diagram, set against a gray backdrop. The four central circles represent various combinations of biometric indicators, indicating the number of different biomarkers examined in tandem within each study (1, 2, 4, 5). The smaller numbers represent the total number of studies according to their respective combinations, while the labels on the right depict the meta-skills, understood as the foundational knowledge, abilities, and experiences upon which all life-acquired skills (both soft and hard) are built for each study.
Biometric indicators recognized within these studies include heart rate (HR), its variation (HRV), and electrodermal activity (EDA), also known as galvanic skin response (GSR). Each of these indicators was used in five different studies, with their combination proving to be the most common (seven studies). Only a single study combined EDA and accelerometer (ACC) data. A significant discovery was that seven studies incorporated four distinct physiological indicators, with the most frequently used (three studies) being electrodermal activity (EDA), skin temperature (ST), blood pressure volume (BVP), electroencephalography (EEG), and accelerometer (ACC). The other seven studies combined four indicators, linking others such as gaze, movement, and interbeat interval (IBI). It is worth noting that a single study combined five indicators.
These findings imply that various studies integrate biometric indicators and propose constructs, viewed in this research as units of analysis, to imbue the collected data with meaningful interpretation. Three primary categories integrating meta-skills stand out: data estimation (three studies) and behavior (three studies), incorporating performance analysis (four studies), alongside social skills (two studies), attention/interest (two studies), and physical activity (one study). The third category is cognitive load as a unit of analysis (two studies), comprised of emotion classification (six studies), mental fatigue (two studies), pain management (one study), cyber sickness (one study), stress (five studies), and anxiety (two studies).
Regarding the methods of data analysis, the use of quantitative approaches that include automated and continuous monitoring of physiological response data through the use of biometric sensors has been indicated (66.6%). Among the most prominent analysis methods, classification models such as support vector machines (SVM), k nearest neighbors (kNN), random forests (RF), extra trees (ET), and gradient boosting (GB) are declared. Furthermore, machine learning includes models (Fucci et al., 2019; Wampfler et al., 2019), algorithms (Collins et al., 2019), and techniques (Lee-Cultura et al., 2020a,b; Aguilar-Herrera et al., 2021). In addition, the need to incorporate technological innovations to capture, measure, and analyze physiological data was recognized. Wearable devices mentioned in the research include Wristband Empatica E4 (Gouverneur et al., 2017; Ragot et al., 2018; Jenks et al., 2020; Poli et al., 2020; Cored Bandrés et al., 2021; Iadarola et al., 2021; Climent-Pérez et al., 2022; Raju et al., 2022), Biopac MP150 (Ragot et al., 2018), Fitbit Charge HR, Apple Watch Series 4, and TicWatch Pro (Choksatchawathi et al., 2020).
On the other hand, 33.3% of the studies accounted for were classified as mixed-type. In these cases, physiological parameters were measured, and these studies included data from self-report questionnaires and the results of standardized tests (Hardacre et al., 2021). Likewise, it is acknowledged that the studies analyzed the recording of biometric signals and conducted correlational analysis with self-report information (Ramírez-Moreno et al., 2021a,b). Additionally, the use of techniques such as questionnaires (Alfredo et al., 2023), for example, the Fatigue Assessment Scale questionnaire and Enophones (Wang et al., 2020), systematic observation (Cored Bandrés et al., 2021; Tan et al., 2021), and semi-structured interviews and surveys is recognized.
Wearable devices have been an alternative to identifying performance characteristics in activities contributing to teaching-learning. The study results allowed recognition of the main characteristics of research, such as describing the participants in the activities developed for data collection, the wide range of physiological indicators, and the methodologies to analyze multimodal data. These findings are consistent with those of the study by Giannakos et al. (2022) since they highlight the use of multiple sources of data obtained from the interactions of students using different resources such as videos, e-books, games, and attending face-to-face classes, among others. The wide range of activities associated with collecting multimodal data highlights the relevance of linking and generating multidisciplinary spaces for a better understanding of developing the teaching-learning process in both pedagogical and real-world contexts.
Similarly, a variety of biometric indicators are recognized, including the HR, its variation (HRV), and electrodermal activity (EDA), also known as galvanic skin response (GSR), skin temperature (ST), blood pressure volume (BVP), and accelerometer (ACC), with some being related to the development of disciplinary competencies, such as data estimation, while others focus on generic variables, such as attention, stress, and anxiety. This understanding is in line with the findings described by Hernandez-de-Menendez et al. (2021), who highlighted the potential of biometric data in managing information related to learning. There has been a demonstrated interest in creating multimodal systems that automatically analyze student states that are difficult to observe with the naked eye, such as cognitive load and stress levels (Bustos-López et al., 2022). At this point, the significance of comprehending how data collected through portable devices in pedagogical contexts can serve as a support tool for learning management is emphasized, considering that its collection, fusion, and analysis pose challenges for integration.
This review was initiated with the question, “What are the characteristics of studies that incorporate portable devices in the analysis of teaching-learning processes?” This question led to the development of a methodology that identified the most relevant characteristics responsive to the objectives framed in each investigation. At a general level, the types of participants have been recognized, with university students, graduate students, volunteers, physicians, office and industrial workers, children under 12 years old, children with special educational needs, and patients being among the most prominent ones. It is also acknowledged that these participants were involved in simulated activities, controlled studies, and pedagogical activities aimed at developing data analysis tools and standardized tests. The technological linkage between activities in virtual reality environments and authentic contexts, such as emergencies and courts, is highlighted.
Additionally, other activities encompassed activities of daily living, games that integrate motion-based touchless games (MBTG) with different avatar representations (ASRs), and educational games based on movement (MBEG). Moreover, the trend of utilizing biometric data such as the HR, its variation (HRV), and electrodermal activity (EDA) is highlighted. This is done to measure and detect stress, enable intervention, and improve attention and working memory. Additionally, it emphasizes the easy and portable use of sensors for measuring emotions and physical characteristics. Thus, the usefulness of technology (in this case, wearables) as tools for assessing the impact of an educational intervention is confirmed by our review.
Regarding the limitations of this research, an area of opportunity is identified in research sub-question number four. While the methods of multimodal data analysis are acknowledged, the characteristics considered for applying machine learning techniques to data gathered via wearable devices could be more comprehensively elucidated. Likewise, it is recognized that in this research, it is impossible to demonstrate the direct impact of interventionist improvements in the various integrated contexts. It is proposed that future work should focus on the generation of multidisciplinary teams that can understand performance variables, aiming to create new methods for multimodal data collection and analysis that promote personalized learning. Furthermore, research should be encouraged to identify factors that affect learning to promote more fluid and reflective educational experiences for all those involved in the teaching-learning process.
The raw data supporting the conclusions of this article will be made available by the authors, without undue reservation.
LG-M and MC-A contributed to the conception and design of the study. JR-R organized the database. CA-N wrote the first draft of the manuscript. All authors contributed to the article and approved the submitted version.
The authors acknowledge the financial and technical support of Writing Lab, the Institute for the Future of Education, Tecnologico de Monterrey, Mexico, in producing this study. The authors acknowledge the financial support of Tecnologico de Monterrey through the Challenge-Based Research Funding Program 2022. Project ID # I005-IFE001-C2-T3-T.
The authors declare that the research was conducted in the absence of any commercial or financial relationships that could be construed as a potential conflict of interest.
All claims expressed in this article are solely those of the authors and do not necessarily represent those of their affiliated organizations, or those of the publisher, the editors and the reviewers. Any product that may be evaluated in this article, or claim that may be made by its manufacturer, is not guaranteed or endorsed by the publisher.
Aguilar-Herrera, A. J., Delgado-Jiménez, E. A., Candela-Leal, M. O., Olivas-Martinez, G., Álvarez-Espinosa, G. J., Ramírez-Moreno, M. A., et al. (2021). Advanced learner Assistance System's (ALAS) Recent Results. 2021 Machine Learning-Driven Digital Technologies for Educational Innovation Workshop, Monterrey, Mexico 1–7.
Alfredo, R. D., Nie, L., Kennedy, P., Power, T., Hayes, C., Chen, H., et al. (2023). "That Student Should be a Lion Tamer!" StressViz: Designing a Stress Analytics Dashboard for Teachers. LAK23: 13th International Learning Analytics and Knowledge Conference, 57–67.
Avella, J. T., Kebritchi, M., Nunn, S. G., and Kanai, T. (2016). Learning analytics methods, benefits, and challenges in higher education: a systematic literature review. Online Learn 20, 13–29. doi: 10.24059/olj.v20i2.790
Borrego, A., Latorre, J., Alcañiz, M., and Llorens, R. (2019). “Reliability of the empatica E4 wristband to measure electrodermal activity to emotional stimuli” in 2019 International Conference on Virtual Rehabilitation (ICVR) (IEEE), Tel Aviv, Israel, 1–2.
Boucsein, W. (2012). Electrodermal Activity (2nd Ed.). Springer Science & Business Media. doi: 10.1007/978-1-4614-1126-0
Bulagang, A. F., Mountstephens, J., and Teo, J. (2021). Multiclass emotion prediction using heart rate and virtual reality stimuli. J Big Data 8, 1–12. doi: 10.1186/s40537-020-00401-x
Bustos-López, M., Cruz-Ramírez, N., Guerra-Hernández, A., Sánchez-Morales, L. N., Cruz-Ramos, N. A., and Alor-Hernández, G. (2022). Wearables for engagement detection in learning environments: a review. Biosensors 12:509. doi: 10.3390/bios12070509
Chandra, V., Priyarup, A., and Sethia, D. (2021). “Comparative study of physiological signals from empatica E4 wristband for stress classification” in Advances in Computing and Data Sciences: 5th International Conference, ICACDS 2021, Nashik, India, April 23–24, 2021, Revised Selected Papers, Part II 5, Eds. M. Singh, V. Tyagi, P. K. Gupta, J. Flusser, T. Ören, and V. R. Sonawane (Nashik, India: Springer International Publishing), 218–229.
Choksatchawathi, T., Ponglertnapakorn, P., Ditthapron, A., Leelaarporn, P., Wisutthisen, T., Piriyajitakonkij, M., et al. (2020). Improving heart rate estimation on consumer-grade wrist-worn device using a post-calibration approach. IEEE Sensors J. 20, 7433–7446. doi: 10.1109/JSEN.2020.2979191
Climent-Pérez, P., Muñoz-Antón, Á. M., Poli, A., Spinsante, S., and Florez-Revuelta, F. (2022). Dataset of acceleration signals recorded while performing activities of daily living. Data Brief 41:107896. doi: 10.1016/j.dib.2022.107896
Collins, J., Regenbrecht, H., Langlotz, T., Can, Y. S., Ersoy, C., and Butson, R. (2019). Measuring Cognitive Load and Insight: A Methodology Exemplified in a Virtual Reality Learning Context. 2019 IEEE International Symposium on Mixed and Augmented Reality (ISMAR), 351–362.
Cored Bandrés, S., Vázquez Toledo, S., Liesa Orús, M., and Baldassarri, S. (2021). La potencialidad de la tecnología en la medición del desarrollo de habilidades sociales en niños con TEA: un análisis desde parámetros fisiológicos. Rev Invest Educ 39, 445–462. doi: 10.6018/rie.430891
Cukurova, M., Giannakos, M., and Martinez-Maldonado, R. (2020). The promise and challenges of multimodal learning analytics. Br. J. Educ. Technol. 51, 1441–1449. doi: 10.1111/bjet.13015
DiMitri, D., Schneider, J., Specht, M., and Drachsler, H. (2018). From signals to knowledge: a conceptual model for multimodal learning analytics. J. Comput. Assist. Learn. 34, 338–349. doi: 10.1111/jcal.12288
Eradze, M., and Laanpere, M. (2017). “Lesson observation data in learning analytics datasets: observata” in Data Driven Approaches in Digital Education: 12th European Conference on Technology Enhanced Learning, EC-TEL 2017, Tallinn, Estonia, September 12–15, 2017, Proceedings 12. Eds. É. Lavoué, H. Drachsler, K. Verbert, J. Broisin, and M. Pérez-Sanagustín (Tallinn, Estonia: Springer International Publishing), 504–508.
Ferguson, R. (2012). Learning analytics: drivers, developments, and challenges. Int J Technol Enhanc Learn. 4, 304–317. doi: 10.1504/IJTEL.2012.051816
Fucci, D., Girardi, D., Novielli, N., Quaranta, L., and Lanubile, F. (2019). A Replication Study on Code Comprehension and Expertise Using Lightweight Biometric Sensors. 2019 IEEE/ACM 27th International Conference on Program Comprehension (ICPC), 311–322.
Garbarino, M., Lai, M., Bender, D., Picard, R. W., and Tognetti, S. (2014). “Empatica E3—a wearable wireless multi-sensor device for real-time computerized biofeedback and data acquisition” in 2014 4th International Conference on Wireless Mobile Communication and Healthcare-Transforming Healthcare through Innovations in Mobile and Wireless Technologies (MOBIHEALTH) (Athens, Greece: IEEE), 39–42.
Ghandi, M. (2019). Cyber-Physical Emotive Spaces: Human Cyborg, Data, and Biofeedback Emotive Interaction with Compassionate Spaces. Blucher Design Proceedings 2, 655–664.
Giannakos, M., Spikol, D., Di Mitri, D., Sharma, K., Ochoa, X., and Hammad, R. (2022). Introduction to multimodal learning analytics. In M. Giannakos, D. Spikol, D. MitriDi, K. Sharma, X. Ochoa, and R. Hammad, (Eds.), The Multimodal Learning Analytics Handbook. Springer Nature Switzerland AG.
Gouverneur, P., Jaworek-Korjakowska, J., Köping, L., Shirahama, K., Kleczek, P., and Grzegorzek, M. (2017). Classification of Physiological Data for Emotion Recognition. Artificial Intelligence and Soft Computing: 16th International conference, ICAISC 2017, Zakopane, Poland, June 11-15, 2017, Proceedings, Part I 10245, 619–627.
Hardacre, B., Hafner, A., and Nakama, P. (2021). The impact of test anxiety on teacher credential candidates. Teach. Educ. Q. 48, 7–28.
Hernandez-de-Menendez, M., Morales-Menendez, R., Escobar, C. A., and Arinez, J. (2021). Biometric applications in education. Int. J. Interact. Design Manuf. 15, 365–380. doi: 10.1007/s12008-021-00760-6
Iadarola, G., Poli, A., and Spinsante, S. (2021). Analysis of Galvanic Skin Response to Acoustic Stimuli by Wearable Devices. 2021 IEEE International Symposium on Medical Measurements and Applications (MeMeA), 1–6.
Järvenoja, H., Järvelä, S., Törmänen, T., Näykki, P., Malmberg, J., Kurki, K., et al. (2018). Capturing motivation and emotion regulation during a learning process. Frontline Learn. Res. 6, 85–104. doi: 10.14786/flr.v6i3.369
Jenks, S., Peacock, W. F., Cornelius, A. P., Shafer, S., Pillow, M. T., and Rayasam, S. S. (2020). Heart rate and heart rate variability in emergency medicine. Am. J. Emerg. Med. 38, 1335–1339. doi: 10.1016/j.ajem.2019.10.035
Koelstra, S., Yazdani, A., Soleymani, M., Mühl, C., Lee, J.-S., Nijholt, A., et al. (2010). Single-Trial Classification of EEG and Peripheral Physiological Signals for Recognition of Emotions Induced by Music Videos. Brain Informatics: International Conference, BI 2010, Toronto, ON, Canada, August 28–30, 2010. Proceedings, 89–100.
Koskimäki, H., Mönttinen, H., Siirtola, P., Huttunen, H. L., Halonen, R., and Röning, J. (2017). Early Detection of Migraine Attacks Based on Wearable Sensors: Experiences of Data Collection Using Empatica E4. In Proceedings of the 2017 ACM International Joint Conference on Pervasive and Ubiquitous Computing and Proceedings of the 2017 ACM International Symposium on Wearable Computers (pp. 506–511).
Lee-Cultura, S., Sharma, K., Cosentino, G., Papavlasopoulou, S., and Giannakos, M. (2021). Children’s play and problem-solving in motion-based educational games: Synergies between human annotations and multi-modal data. Interaction Design and Children, In IDC ‘21: Proceedings of the 20th Annual ACM Interaction Design and Children Conference, Association for Computing Machinery, New York, NY, United States. doi: 10.1145/3459990.3460702
Lee-Cultura, S., Sharma, K., Papavlasopoulou, S., and Giannakos, M. (2020a). Motion-Based Educational Games: Using Multimodal Data to Predict Player's Performance. 2020 IEEE Conference on Games (Cog), 17–24.
Lee-Cultura, S., Sharma, K., Papavlasopoulou, S., Retalis, S., and Giannakos, M. (2020b). Motion-based educational games: Using multi-modal data to predict player’s performance. In 2020 IEEE Conference on Games (CoG), Osaka, Japan: IEEE. doi: 10.1109/CoG47356.2020.9231892
Lin, C.-J., y Hwang, G.-J. (2018). A learning analytics approach to investigating factors affecting EFL students’ oral performance in a flipped classroom. J. Educ. Technol. Soc., 21, 205–219.
Magaki, T., and Vallance, M. (2019). Developing an Accessible Evaluation Method of VR Cybersickness. 2019 IEEE Conference on Virtual Reality and 3D User Interfaces (VR), 1072–1073.
Martinez, C. C. L. (2019). Implementation of a Multisensor Wearable Artificial Pancreas Platform: Ensuring Safety with Communication Robustness and Cyber Security. Illinois Institute of Technology ProQuest Dissertations Publishing.
Monsalves, D., Reyes, H. C., and Riquelme, F. (2023). Analítica multimodal del aprendizaje en Chile: una revisión sistemática. Apuntes Cienc. Soc. 11, 70–80. doi: 10.18259/acs.2023008
Mu, S., Cui, M., and Huang, X. (2020). Multimodal data fusion in learning analytics: a systematic review. Sensors 20:6856. doi: 10.3390/s20236856
Novak, J. (2019). Assessment of the impact of acute stress in cases of necessary defense by Czech courts. Ido movement for culture. J. Martial Arts Anthropol. 19, 89–91. doi: 10.14589/ido.19.1S.13
Ollander, S., Godin, C., Campagne, A., and Charbonnier, S. (2016). “A comparison of wearable and stationary sensors for stress detection” in 2016 IEEE International Conference on Systems, Man, and Cybernetics (SMC) (Budapest, Hungary: IEEE), 004362–004366.
Page, M. J., McKenzie, J. E., Bossuyt, P. M., Boutron, I., Hoffmann, T. C., Mulrow, C. D., et al. (2021). Updating guidance for reporting systematic reviews: development of the PRISMA 2020 statement. J. Clin. Epidemiol. 134, 103–112. doi: 10.1016/j.jclinepi.2021.02.003
Perales, F. J., Riera, L., Ramis, S., and Guerrero, A. (2019). Evaluation of a VR system for pain management using binaural acoustic stimulation. Multimed. Tools Appl. 78, 32869–32890. doi: 10.1007/s11042-019-07953-y
Peters, G. A., Wong, M. L., and Sanchez, L. D. (2020). Pedometer-measured physical activity among emergency physicians during shifts. Am. J. Emerg. Med. 38, 118–121. doi: 10.1016/j.ajem.2019.07.019
Poli, A., Cosoli, G., Scalise, L., and Spinsante, S. (2020). Impact of wearable measurement properties and data quality on ADLs classification accuracy. IEEE Sensors J. 21, 14221–14231. doi: 10.1109/JSEN.2020.3009368
Quadri, A., and Shukor, N. (2021). The benefits of learning analytics to higher education institutions: a scoping review. Int. J. Emerg. Technol. Learn. 16, 4–15. doi: 10.3991/ijet.v16i23.27471
Ragot, M., Martin, N., Em, S., Pallamin, N., and Diverrez, J.-M. (2018). “Emotion recognition using physiological signals: laboratory vs. wearable sensors” in Advances in Human Factors in Wearable Technologies and Game Design. eds. T. Ahram and C. Falcão (Budapest, Hungary: Springer International Publishing), 15–22.
Rahman, J. S., Gedeon, T., Caldwell, S., Jones, R., and Jin, Z. (2021). Towards effective music therapy for mental health care using machine learning tools: human affective reasoning and music genres. J. Artif. Intell. Soft Comput. Res. 11, 5–20. doi: 10.2478/jaiscr-2021-0001
Raju, G., Sorby, S. A., Panther, G., Reid, C., and Mogadam, J. H. (2022). Differences in Perceived Stress Levels and Measured Stress While Solving Spatial Tests. 2022 ASEE Annual Conference & Exposition.
Ramírez-Moreno, M. A., Carrillo-Tijerina, P., Candela-Leal, M. O., Alanis-Espinosa, M., Tudón-Martínez, J. C., Roman-Flores, A., et al. (2021b). Evaluation of a fast test based on biometric signals to assess mental fatigue at the workplace—a pilot study. Int. J. Environ. Res. Public Health 18:11891. doi: 10.3390/ijerph182211891
Ramírez-Moreno, M. A., Díaz-Padilla, M., Valenzuela-Gómez, K. D., Vargas-Martínez, A., Tudón-Martínez, J. C., Morales-Menendez, R., et al. (2021a). Eeg-based tool for prediction of university students' cognitive performance in the classroom. Brain Sci. 11:698. doi: 10.3390/brainsci11060698
Regalia, G., Onorati, F., Lai, M., Caborni, C., and Picard, R. W. (2019). Multimodal wrist-worn devices for seizure detection and advancing research: focus on the Empatica wristbands. Epilepsy Res. 153, 79–82. doi: 10.1016/j.eplepsyres.2019.02.007
Rivers, D. J. (2022). The situation specific arousal analyzer: innovation in the physiological assessment of foreign language education anxiety. Front. Educ. 7:10. doi: 10.3389/feduc.2022.802639
Rodríguez-Triana, M. J., Prieto, L. P., Vozniuk, A., Boroujeni, M. S., Schwendimann, B. A., Holzer, A., et al. (2017). Monitoring, awareness, and reflection in blended technology enhanced learning: a systematic review. Int. J. Technol. Enhanc. Learn. 9, 126–150. doi: 10.1504/IJTEL.2017.084489
Ronda-Carracao, M. A., Santos, O. C., Fernandez-Nieto, G., and Martinez-Maldonado, R. (2021). Towards Exploring Stress Reactions in Teamwork Using Multimodal Physiological Data. CEUR Workshop Proceedings.
Sedraz Silva, J. C., Souza, D., Daf, F., Cavalcanti Ramos, J. L., Rodrigues, R. L., Zambom, E., et al. (2018). Assessment of the usability of a learning analytics resource dedicated to promoting self-regulated learning in flipped classroom. Rev. Latinoam. Tecnol. Educ. Relatec 17, 9–23. doi: 10.17398/1695-288X.17.2.9
Siemens, G., and Long, P. (2011). Penetrating the fog: Analytics in learning and education. EDUCAUSE review, 46, 30.
Siemens, G., and Gasevic, D. (2012). Guest editorial-learning and knowledge analytics. Journal of Educational Technology & Society, 15, 1–2.
Spann, C. A., Schaeffer, J., and Siemens, G. (2017). Expanding the Scope of Learning Analytics Data: Preliminary Findings on Attention and Self-Regulation Using Wearable Technology. In Proceedings of the Seventh International Learning Analytics & Knowledge Conference (pp. 203–207).
Tan, A. L., Gillies, R., and Jamaludin, A. (2021). A case study: using a neuro-physiological measure to monitor students' interest and learning during a micro: bit activity. Educ. Sci. 11:379. doi: 10.3390/educsci11080379
Tempelaar, D., Rienties, B., and Nguyen, Q. (2020). Subjective data, objective data and the role of bias in predictive modelling: lessons from a dispositional learning analytics application. PLoS One 15:e0233977. doi: 10.1371/journal.pone.0233977
Ullmann, T. D., De Liddo, A., and Bachler, M. (2019). A visualisation dashboard for contested collective intelligence. Learning analytics to improve Sensemaking of group discussion. Ibero Am. J. Digital Educ. 22, 41–80. doi: 10.5944/ried.22.1.22294
Vos, G., Trinh, K., Sarnyai, Z., and Azghadi, M. R. (2023). Generalizable machine learning for stress monitoring from wearable devices: a systematic literature review. Int. J. Med. Inform. 173:105026. doi: 10.1016/j.ijmedinf.2023.105026
Wampfler, R., Klingler, S., Solenthaler, B., Schinazi, V., and Gross, M. (2019). Affective State Prediction in a Mobile Setting Using Wearable Biometric Sensors and Stylus. Proceedings of the 12th International Conference on Educational Data Mining, EDM 2019, Montréal, Canada, July 2–5, 2019. International Educational Data Mining Society (IEDMS) 2019, 198–207.
Wang, Q., Jing, S., Joyner, D., Wilcox, L., Li, H., Plötz, T., et al. (2020). Sensing Affect to Empower Students: Learner Perspectives on Affect-Sensitive Technology in Large Educational Contexts. Proceedings of the Seventh ACM Conference on Learning@ Scale, 63–76.
Keywords: wearable devices, multi-modal learning analytics, teaching-learning processes, biometric indicators, educational innovation
Citation: Glasserman-Morales LD, Carlos-Arroyo M, Ruiz-Ramirez JA and Alcantar-Nieblas C (2023) Use of wearable devices in the teaching-learning process: a systematic review of the literature. Front. Educ. 8:1220688. doi: 10.3389/feduc.2023.1220688
Received: 14 May 2023; Accepted: 16 August 2023;
Published: 13 September 2023.
Edited by:
Simon K. S. Cheung, Hong Kong Metropolitan University, Hong Kong SAR, ChinaReviewed by:
Ingrid Isenhardt, RWTH Aachen University, GermanyCopyright © 2023 Glasserman-Morales, Carlos-Arroyo, Ruiz-Ramirez and Alcantar-Nieblas. This is an open-access article distributed under the terms of the Creative Commons Attribution License (CC BY). The use, distribution or reproduction in other forums is permitted, provided the original author(s) and the copyright owner(s) are credited and that the original publication in this journal is cited, in accordance with accepted academic practice. No use, distribution or reproduction is permitted which does not comply with these terms.
*Correspondence: Martina Carlos-Arroyo, bWFydGluYS5jYXJsb3NAdGVjLm14
Disclaimer: All claims expressed in this article are solely those of the authors and do not necessarily represent those of their affiliated organizations, or those of the publisher, the editors and the reviewers. Any product that may be evaluated in this article or claim that may be made by its manufacturer is not guaranteed or endorsed by the publisher.
Research integrity at Frontiers
Learn more about the work of our research integrity team to safeguard the quality of each article we publish.