- 1Graduate School of Education, University of Pennsylvania, Philadelphia, PA, United States
- 2Graduate School of Education, Rutgers, The State University of New Jersey, New Brunswick, NJ, United States
- 3Princeton High School, Princeton Public Schools, Princeton, NJ, United States
- 4Governor's Academy, Bayfield, MA, United States
- 5Massachusetts Institute of Technology, Cambridge, MA, United States
Historic challenges in the biological sciences, such as the spread of disease and climate change, have created an unprecedented need for humans to engage with scientific information to address societal problems. However, understanding these socioscientific issues (SSI) can be hard due to the difficulty of comprehending their complex structures and behaviors, the intentional propagation of misinformation, and an insufficient understanding of the epistemic practices that scientists use to develop relevant knowledge. Education researchers have highlighted additional problems in the way science is taught with a focus mainly on concepts rather than practices, competing curricular mandates, and professional development activities that do not provide usable knowledge. The research reported here follows more than a decade of work using agent-based computational models to support the comprehension and analysis of complex biological systems. Our recent work has aimed to build tools and strategies to support students in decision making about complex SSIs. In this paper, we discuss 7 design challenges and principles that underpin this recent focus. Specifically, we combine agent-based modeling with strategies to develop students’ epistemic performance in high school biology curricula. We then provide a detailed case study of how the 7 design principles were used to create a disease epidemic model and unit anchored in the biology topic of the nature of science. Our goal is to offer a comprehensive set of research-derived design principles that can bridge classroom experiences in biology to applications of SSIs.
1. Introduction
The enormous impact of science and technology on every walk of life in contemporary society, through its applications for good and ill, puts extra requirements on scientists and science teachers. The former must accept responsibility for the social and moral consequences of their work; they should always be guided by ethical principles. The science teachers should draw attention to the universal nature of scientific research and to the global impact of its applications, making it necessary for everybody to think and act as a citizen of the world community.
–Joseph Rotblat, nuclear physicist and Nobel Peace Prize laureate (Rotblat 2002; p.190).
Nuclear physicist Joseph Rotblat was awarded the Nobel Peace prize in 1995 for his lifelong work on diminishing the impact and future development of nuclear weapons. In the opening quote above, he highlighted the roles of scientists and science teachers in working toward a global, scientifically literate citizenry capable of ethical decision making. Fast forward two decades, and we arguably face many more challenges that have been created by what Ord (2020) called our increased anthropogenic risks. These risks entail human-generated crises such as climate change, disease epidemics, and environmental degradation that encompass a set of complex socioscientific challenges that are situated in the discipline of biology. These challenges underscore the need to understand the scientific practices that underpin biological research; the limits of human cognition, especially in our increasingly (mis)information-saturated society; and how to improve educational systems that do not encourage teaching and learning about scientific content that can be applied in the real world (Gorman and Gorman, 2021).
In advocating for school science to attend more intentionally to these applications of science, Reiss (2020) suggested that the COVID-19 pandemic can be an opportunity to examine the sociological aspects of science that can lead to greater scientific literacy and, in turn, change human behavior to mitigate the anthropogenic risks we face. Like Rotblat, Reiss invoked biology teachers and biology curricula as vital to these efforts, noting that teachers need to collaboratively design curricula that are embedded in socioscientific issues (SSIs), “leveraging existing resources, mobilizing passions and exploring issue relevance” (p. 13). But if we consider SSIs as a class of complex systems with multiple interconnected and interdependent variables, designing curricula to represent their complex nature can be challenging. Complex systems can be defined as macro-level patterns and behaviors that emerge from micro-level interactions (Sherrington, 2010). Due to their weblike structures where components or units are connected to multiple other components or units, behaviors of complex systems can be non-linear, which makes it sometimes difficult to predict the exact pathway that information will travel along (Yoon, 2008). Thus, complex systems researchers in education have highlighted learning issues stemming from student’s difficulties in visualizing system structures and understanding the dynamic relationship between variables (e.g., Yoon, 2008). Fensham (2012) discussed this aspect of complexity as relating to the uncertain nature of science, especially as it relates to SSIs, in that often a scientific solution to ameliorating such issues is not fully understood. He asserts that school science pedagogies and tools ought to be able to illustrate and explore multiple hypotheses and perspectives with a stance that recognizes this uncertain aspect of SSI investigation.
Additionally, teachers experience a number of obstacles for determining whether and how to teach SSIs. For example, like everyone, teachers are part of our ever-expanding information system that includes anti-science rhetoric and deliberate malignment of facts (Chinn et al., 2020; Gorman and Gorman, 2021). Teachers need instructional strategies that can mitigate this misinformation foment and facilitate an appreciation for the scientific practices that scientists use to establish knowledge claims (Darner, 2019; Chinn et al., 2020; Duschl, 2020). We also know that teaching about SSIs requires a kind of inquiry-based instruction that takes more time than typical stand-and-deliver methods (Zeidler, 2014; Hodson, 2020). Because instruction on SSIs demands more time, it is in constant tension with other time-based pressures on high school biology courses, such as state-mandated testing. Relatedly, an emphasis on testing concepts has continued to thwart the Next Generation Science Standards’ (NGSS) efforts to focus on science and engineering practices as an important component of K-12 science learning (NGSS Lead States, 2013), leading to epistemic challenges in what Ford (2008) called “a grasp of practice.” Finally, while Wilson (2013) discussed the steep learning curve that teachers will face in shifting pedagogies to meet NGSS requirements, we also know that PD efforts have historically fallen short in providing teachers with useable knowledge and resources for classroom practice (Desimone and Garet, 2015; TNTP, 2015).
Building on over a decade of work developing agent-based modeling curricula and professional development for teaching and learning about scientific complex systems in biology (Yoon et al., 2015, 2016, 2017, 2020), in this paper we present design principles that consider the aforementioned challenges to teaching about contemporary SSIs. The paper is divided into two sections. In the first section, we describe in more detail the design challenges for teaching complex SSIs and the principles that we have constructed to address them. The second section presents a case study of how we have implemented these designs in an ongoing curriculum and PD project with an agent-based epidemic model and associated epistemic practices.
2. Design challenges and principles for teaching about complex socioscientific issues
SSIs provide an excellent instructional context for promoting understanding of science and effective engagement with science in peoples’ everyday lives. SSI curricula and instruction engage students in meaningful science that address societal problems, from climate change to misinformation. Zeidler (2014) suggested that effective SSI instruction should draw on controversial topics that are personally relevant to students, thereby making it easier for them to readily see the social ramifications. Furthermore, the instruction should involve implicit or explicit ethical components that require moral reasoning. Making school science personally relevant has been a long-standing issue of science education, and SSI scholars have noted that the decontextualized nature through which science content is often presented does little to engage students in real-world decisions (Hodson, 2009; Herman, 2018). Hodson (2020) argued that students’ curricular experiences must give them the opportunity to explore current complex sociopolitical contexts within which SSIs are located, attend to resolving conflicting interests, and develop a personal viewpoint. Empirical studies examining what students come to know about science through curriculum anchored in complex SSIs show improved understanding in multiple dimensions of learning. For example, on the issue of e-cigarette regulation, Ke et al. (2020) found that students were able to recognize mechanisms and system dimensions that were not immediately apparent. Herman (2018) found that when students were given field experiences to investigate an environmental issue, they were able to develop an ecological worldview that considered the interconnected and ethical aspects of human interactions with nature. These examples importantly illustrate how knowledge of complex scientific activities can be revealed through SSI exploration that can, in turn, respond to the challenge of science denialism by providing information that is not so easy to ignore.
In practice, challenges arise when implementing SSI instruction. This article focuses on seven of these challenges and principles for addressing them. Table 1 provides an advanced organizer of educational design challenges and corresponding principles for addressing these challenges. All 7 challenges and design principles center educational activities needed for successful classroom implementation. Specifically, the 7 design principles seek to address and mitigate the complexity inherent in SSIs (#1 and #2), the cognitive demands in decision making about SSIs (#3 and #4), and the challenges of teaching about SSIs including supporting teacher pedagogical shifts and improving PD experiences (#5 to #7).
2.1. Design challenge #1: seeing the system in all of its complexity
At a basic level, SSIs are a system of relationships. They can be defined as complex societal problems that require the consideration of scientific research and practices as they are applied to social, cultural, and environmental contexts (Zeidler, 2014; Sadler et al., 2016). Their complexity derives from challenges that include ill-defined system boundaries, the need to acknowledge and reconcile multiple perspectives, the need to integrate components of physical and social systems, and difficulty in identifying how system variables interact to produce global outcomes (Owens et al., 2021). It is precisely this complexity that has spawned numerous studies examining the mechanisms that fuel social scientific systems and how to support instruction and learning about them (Yoon et al., 2018). Because complex systems reside in nested web-like structures, variables interact in nonlinear ways that make it challenging to understand etiologies and pathways of information flow (Bar-Yam, 2016). Likewise, Grotzer and Tutwiler (2014) highlighted difficulties in determining cause-and-effect relationships among variables because emergent patterns at the system level may occur over large geographic (e.g., global warming) and temporal (e.g., natural selection) scales. Elsewhere we have written about the learning challenges that students experience in understanding how complex systems operate due to this hidden order (Yoon, 2018). Thus, to create learning experiences that can address the complexity within which SSIs exist, we must first support the ability to see the whole system and how it behaves.
2.2. Design principle #1: promote learning through complex systems modeling
Attending to the idea of seeing the system in all of its complexity, complex systems researchers in science education have investigated the learning affordances of agent-based computational models with K-12 students (e.g., Klopfer et al., 2009; Wilensky and Rand, 2015). These models are designed from the theoretical perspective that microscale (local) interactions lead to emergent macroscale (global) patterns. Two popular computational platforms are NetLogo and StarLogo, both of which specify the system bounds (e.g., predators, prey, trophic levels); the relationships between system agents (e.g., predators eat prey); and agent (e.g., birth and death rates) and population (e.g., size) characteristics. These features are actualized in the computational model through buttons and sliders that can be turned on and off and manipulated to change initial conditions. The models can be run at varying speeds and for varying amounts of time so that students can slow the model down enough to follow individual agents or observe change over multiple generations of system activity. These platforms are also built to offer multiple representations that include mathematical graphs that display dynamic population changes, the qualitative model showing variable interactions, and the computational code that defines agent characteristics and their relationships to each other (that can also be manipulated). Students can engage in experimentation, data collection, and data analysis; they can run multiple iterations and compare various hypotheses of different variable configurations. In a series of studies conducted to determine what and how students learn through agent-based computational models, we have found increases in several aspects of learning, including their understanding of complex systems mechanisms and biological content knowledge (Yoon et al., 2016) and their knowledge of modeling as a scientific tool to support sense making (Miller and Yoon, 2023). Importantly, this form of complex systems modeling offers students a way to investigate aspects of system dynamics that are normally hidden and that influence how and why we may see macro-scale patterns emerge. Seeing the system through multiple perceptual lenses can foster deeper levels of understanding of the complex interactions that lead to system outcomes.
2.3. Design challenge #2: science denialism
Recent years have seen an increase in science denialism (Sloman and Fernbach, 2017; Chinn et al., 2020; Gorman and Gorman, 2021; Sinatra and Hoffer, 2021), which can thwart our goals to address socioscientific problems. Gorman and Gorman (2021) highlighted a number of psychological biases that help explain why some of us will deny the scientific facts that could negatively impact our lives. For example, the human mind is naturally biased to underestimate large risks and to overestimate small risks, which has led, among other things, to nonscientific movements like antivaccination campaigns. Gorman and Gorman (2021) also pointed to the natural tendency for people to avoid complexity in learning something new, in part because of the necessary investment of time and effort. This tendency has resulted, for some, in ignoring scientific facts or believing that these issues do not relate directly to them (e.g., climate change is someone else’s problem). But as Sinatra and Hoffer (2021) stated, understanding the complex mechanisms that underpin topics such as climate change is essential in accepting the science that has led to consensus among the scientific community about its anthropogenic causes. In addition, it is important to understand the practices that render science a reliable way of knowing. Many people have come to view scientific practices as untrustworthy, because they view scientists as politically or financially biased, or because science is too uncertain to be counted on (Chinn et al., 2020; Kienhues et al., 2020). Therefore, our second design challenge is to construct educational experiences that can confront the increasing issue of science denialism.
2.4. Design principle #2: promote an understanding of why science is reliable
Because a failure to understand systems is, as we just noted, one source of science denialism, Principle #1 (Promoting learning through complex systems modeling) can play an important role in addressing science denialism. But it is also necessary to directly address the challenge that many people distrust science and believe scientific practices to be biased and unreliable. Therefore, it is critical to develop instruction that enables students to understand why it is that science is reliable, despite the fact that science is often highly uncertain, changing, and disputatious (Kienhues et al., 2020; Chinn et al., in press). Chinn et al. (in press) provided a detailed analysis of how instruction within SSIs can be organized to promote an understanding of why science is reliable. One component is helping students appreciate how scientists develop and apply socially shared criteria such as criteria for good scientific models (e.g., scientific models fit the evidence, they provide mechanistic explanations, etc.). Metz et al. (2018) found that adults’ commitment to fit with evidence was associated with greater endorsement of scientific findings such as evolution and climate change. Another component is helping students appreciate particular scientific practices that contribute to the reliability of scientific knowledge, such as: scientists’ use of evidence-centered social critique, work to constantly improve empirical methods, scientists’ methods for resolving disagreements, and their efforts to ensure that their models and explanations fit all the evidence, not just some of it. Instruction that includes a focus on the reliable practices of science can improve students’ endorsement of the scientific consensus on scientific topics (Leung, 2020). Instruction that promotes an appreciation that scientists base conclusions on a large body of evidence rather than one or two studies can similarly increase endorsement of claims supported by larger bodies of evidence rather than cherry picked evidence (Oura et al., 2023). Learning about why scientists change their minds also promotes greater endorsement of scientific claims (Barzilai et al., 2023).
2.5. Design challenge #3: misinformation foment
Another challenge facing people as they address SSIs is the rampant misinformation they encounter on these issues (Chinn et al., 2021). Rampant misinformation makes it difficult to make sense of scientific ideas and evidence. Sloman and Fernbach (2017) discussed the remarkable tenacity of false beliefs about scientific matters; in one study, for example, only 12 percent of respondents were even partially correct in identifying the causes of global warming. Nichols (2017) revealed issues in American education that do not enforce the critical thinking habits that are necessary for accurately evaluating misinformation. Other publications have pointed to the grave dangers in the growth of a public who are unable to distinguish between scientifically derived claims and politically sanctioned or economically motivated nonscientific claims (Manjoo, 2008; Bjornberg et al., 2017; Gorman and Gorman, 2021). Enlisting science teachers to stem the tide of this misinformation foment is essential. But in the face of powerful lobbyists, this may not be easy to do. For example, a Frontline PBS story in March 2017 described a free and professionally developed curriculum package aimed at rejecting human’s role in climate change; the package included a book titled, “Why Scientists Disagree About Global Warming.” Constructed by the Heartland Institute, a conservative libertarian thinktank well known for supporting conferences for climate change deniers, this package was sent to 25,000 teachers with an ultimate goal of reaching more than 200,000. For teachers, these findings mean that it is important for them, as well as their students, to develop the epistemic competence (Barzilai and Chinn, 2018) to distinguish between accurate information and misinformation. Although critical to address, current instructional practices have tended to fall short of developing students’ epistemic competence in this way (Elby et al., 2016; Chinn et al., 2020). Indeed, multiple contextual factors affect teachers’ abilities to focus on developing students’ epistemic competence in the classroom, as they are likely to hold a multitude of goals for their students (both epistemic and nonepistemic) simultaneously (Buehl and Fives, 2016; Fives et al., 2017). Furthermore, teachers need curricular models and strategies for developing students’ epistemic competence, particularly when engaging various knowledge domains (e.g., biology and scientific modeling) (Sandoval, 2014).
2.6. Design principle #3: emphasize apt epistemic performance
To address the misinformation foment, our work has followed Barzilai and Chinn (2018), who argued that the goal of epistemic education is apt epistemic performance, which refers to achieving epistemic goals (such as accurate beliefs) successfully through the use of one’s competence. Their Apt-AIR framework specifies the competencies necessary for good epistemic performance. This involves, first, the use of three main components of epistemic cognition from the AIR model of epistemic cognition (Chinn et al., 2014). Epistemic Aims and values are the goals that people have, such as finding things out, developing explanations, and so on, and the value that people place on these aims. Epistemic Ideals are the criteria that are used to evaluate whether the epistemic aims have been met (e.g., fit with evidence). Reliable epistemic processes are the procedures, strategies, and methods that have a good probability of achieving epistemic aims (e.g., careful procedures of selecting representative samples in a study). Apt epistemic performance involves engaging adeptly with epistemic aims, ideals, and reliable processes in five interlocking ways: cognitively, metacognitively, socially, adaptively, and motivationally and affectively. To learn to distinguish information from misinformation, students can develop aims, ideals, and processes for evaluating the expertise and bias of sources, for integrating information among sources, and for reasoning about evidence (Barzilai and Chinn, 2018). Research on promoting the development of valuable epistemic aims, ideals, and reliable processes has shown that students from elementary school to high school can develop and use these appropriately (Schwarz and White, 2005; Pluta et al., 2011; Murphy et al., 2022). Research directed at promoting these competences has produced improved students’ skill at evaluating scientific information. Barzilai and Ka’adan (2017) found that instruction directed at promoting apt epistemic performance relevant to documents-based inquiry improves students’ abilities to draw conclusions from conflicting information sources. In recent research with elementary students working with a complex agent-based model simulating a garden ecosystem (Cottone et al., 2023), the teacher modeled for students how data collected from the simulation should be used as evidence to make inferences about differential moisture and sunlight needs for individual plant varieties. Students were also repeatedly prompted to explain what data they used to justify their claims. Among other important trends, our findings revealed students’ abilities to use aggregate data to support their inferences–a key challenge in developing data literacy skills (Makar and Rubin, 2018). We believe that using the Apt-AIR framework to guide instruction can enable students to develop epistemic aims, ideals, and reliable processes that will help them to distinguish between accurate information and misinformation.
2.7. Design challenge #4: primacy of science concepts over scientific practices
In order to fully embrace the goal of promoting apt epistemic performance in science education, one critical challenge to overcome is the primacy that teachers place on the teaching of scientific concepts (e.g., well-established theories such as evolution) rather than on scientific practices (e.g., collecting and analyzing data to establish theories) (Chinn et al., 2020; Feinstein and Waddington, 2020). As we discuss in the section on Design Challenge #5, part of the challenge stems from teachers’ need to navigate institutional mandates. However, we also know teachers prefer to teach—or believe that they should teach—only scientific content, especially in the subject of biology (Ford, 2008). This is concerning for multiple reasons, including the fact that such emphases communicate a flawed understanding of science (e.g., knowledge claims are static and infallible) and misrepresent how scientific knowledge is actually generated (i.e., through the disciplinary practices of science) (Goldman et al., 2016; Duschl, 2020; Feinstein and Waddington, 2020), and progressive knowledge-building discourse (e.g., peer-review and critique) (Bereiter and Scardamalia, 2014). A focus only on known concepts runs the risk of imparting a false understanding of the uncertain nature of scientific investigations, as we discuss in the introduction, and does not enable students to learn the reasoning practices needed to engage with SSIs. Indeed, the primacy of science concepts in science education was a central concern that led to the construction of the NGSS and an articulation of the three-dimensional goals for science learning in K-12 education (i.e., disciplinary core ideas; science and engineering practices, and cross-cutting concepts) (Bybee, 2013).
2.8. Design principle #4: prioritize scientific practices over science concepts
To address the design challenge of the primacy of concepts, Ford (2008) called for restructuring science education to focus on a grasp of practice, which refers to engaging students in the construction and critique scientists use to evaluate evidence and knowledge claims. For topics in science that have multiple perspectives and contested beliefs, such as complex SSIs, Chinn et al. (2021) recommended creating epistemically complex learning environments that aim to (a) enable students to understand the social practices of science and what makes them reliable, (b) engage students with a range of good and bad evidence and sources to learn to address the range of information encountered in the digital sphere, and (c) facilitate metacognitive reflection on ways of knowing. Similar to our work, scholars like Duschl (2008, 2020) have identified scientific simulations as one method for building epistemically complex learning experiences, where students can construct knowledge claims by selecting data that will be used as evidence, use evidence to ascertain patterns, and propose explanations. Students can then participate with each other in critiquing knowledge claims vis-à-vis an examination of the practices or methods used to generate them (Duschl, 2008), such as whether the methods account for variability in the system or experimental error. For teachers, this also means developing their own grasp of practice, becoming comfortable and confident in shifting from instruction on concepts to instruction on practices, and making space in the curriculum for their students to engage in these scientific practices (Muis et al., 2016; Manz and Suárez, 2018; Feinstein and Waddington, 2020).
2.9. Design challenge #5: curricular coherence
To incorporate complex SSI instruction, as we advocate throughout this article, the ways of teaching school science need to change. Fensham (2012) raised a number of concerns with traditional school science, which includes imparting the belief that knowledge is firmly established and that there is only one single correct answer. Traditional school science is embedded in institutional mandates—such as the need to teach a required curriculum for the state standardized test, that reify these beliefs. Efforts to develop more progressive teaching expertise to navigate these mandates have run up against instructional barriers, including a lack of opportunities for teachers to implement what they learned in PD (TNTP, 2015). With myriad variables to orchestrate on a daily basis, any new addition to one’s teaching repertoire must be relatively easy to integrate into classroom practices (Desimone and Garet, 2015). We have seen this firsthand in our own research with agent-based modeling of complex systems. Teachers who were more flexible in applying their new pedagogical skills in their classroom and whose content knowledge understanding was more aligned with our curriculum showed higher levels of adaptive expertise that, in turn, influenced greater student learning gains (Yoon et al., 2019). There is also abundant research indicating that anchoring new learning experiences in prior knowledge can ease cognitive load to create space for information uptake in the short-term memory and coordination with long-term memory stores (e.g., Mayer, 2017).
2.10. Design principle #5: align PD with teachers’ existing curricula
One way to mitigate the challenges that teachers experience in navigating institutional mandates is to align new curricular and instructional approaches with their current practices. In a report on effective PD by Darling-Hammond et al. (2017), their review of the field revealed important characteristics that effective PD for teachers should encompass. One characteristic is engaging teachers in active learning and sense-making that involves using their past experiences as resources for new learning and using teachers’ own understanding of their situated classroom needs to develop relevant and authentic learning experiences for students. Similarly, in their review of best practices for teacher PD in the U.S., Desimone and Garet (2015) found that PD was more successful when linked to classroom lessons already taught. They discussed evidence from empirical studies that identifies this aspect of coherence—the notion that new learning coheres with teachers’ prior knowledge—as significantly producing higher student achievement than when PD experiences are not linked. They suggested that PD designs should consider the ease with which new instructional approaches can be integrated into existing classroom structures and curricula.
2.11. Design challenge #6: the steep curve of teacher knowledge and practice
Novice teachers—whether novice to the profession or novice to specific strategies or methods—typically face a steep learning curve in terms of the knowledge and skills required for effectively implementing new lessons and curricula (Lampert et al., 2013). Furthermore, like what we have argued, Osborne and Pimentel (2022) discussed the enormous problems that misinformation, the lack of focus on the complex nature of SSIs, and traditional curricula have created for school science. They advocate for, among other things, a major shift in teacher training. This issue is particularly germane to our work, in that SSI instruction of complex topics through agent-based modeling tools with an emphasis on epistemic performance is new to K-12 instruction. With the aforementioned lack of instructional models to help students develop capacities in epistemic performance and the primacy of teaching concepts over practices, we need to attend closely to how to support teacher knowledge and practice. Moreover, Owens et al. (2021) suggested that we know relatively little about both what teachers do to facilitate learning in the context of SSI instruction and what are successful strategies that PD experiences could employ to help flatten the steep curve of teacher learning. Grossman et al. (2009) provided a useful set of strategies to support novice instruction that includes: representation (i.e., making key practices visible), decomposition (i.e., examining the components of teacher moves that enable effective practice), and approximation (i.e., opportunities to experiment and rehearse with practices).
2.12. Design principle #6: develop high-leverage epistemic teacher moves and routines
Grossman et al.’s (2009) study represents broader research taking place in the field of teacher development around identifying high-leverage practices (HLPs) that promote effective teaching and learning (e.g., McDonald et al., 2013; Forzani, 2014; Cohen, 2015). HLPs can be defined as evidence-based teacher moves that support student achievement and the learning of disciplinary content (Cohen, 2015). Although research on HLPs for the effective teaching of SSIs is in nascent stages, a growing set of possible HLPs for teaching complex SSIs is emerging in the literature. For example, Owens et al. (2021) found that linking issues to personal experience, challenging students to examine SSIs from multiple perspectives, and requiring students to use skepticism when analyzing potentially biased sources of information constituted a set of HLPs for SSI instruction. Chan (2022) identified discourse-based HLPs for eliciting and supporting student thinking, such as creating opportunities for students to report their group’s thinking to other student groups and addressing students’ emergent thinking during group work. In our own pilot work (Hussain-Abidi et al., 2023), we have hypothesized a set of teacher moves that use epistemic callouts embedded in critical parts of the curriculum to support connections to real-world scientific reasoning and to elicit students’ metacognition about scientific practices. When teachers are equipped with HLPs in SSI instruction, they can then rehearse these teacher moves so they become routines that can ideally be applied to other curricular content (Kloser, 2014; Owens et al., 2021).
2.13. Design challenge #7: lack of useable PD
Any shift in teacher training must also contend with how best to provide PD to teachers. In-service teachers have historically identified the lack of useable PD as problematic, and this problem extends to PD for SSI instruction. The lack of usability stems from, among other things, the low utility of most PDs for real school contexts, its poor delivery quality, and its lack of customization to teacher needs (Hill, 2015; TNTP, 2015). It is widely known that the dearth of high-quality PD opportunities makes adopting reforms challenging (Blandford, 2012). This is particularly true for SSI instruction, as little is known about how teachers learn to adopt SSI curricula and how they should be supported (Feinstein and Waddington, 2020). Constructing effective PD opportunities is further complicated by what we know about how teachers learn. We know that optimal learning starts with teachers as knowers and agents of change, where social relationships are fostered for peer-to-peer support and where the examination of subject-matter pedagogy involves active sense making and problem solving with teachers who know the craft of teaching (Hatch et al., 2006; Lieberman and Mace, 2010; Moon et al., 2014; Yoon et al., 2020). A review of studies focused on PD qualities that support technology-enhanced inquiry instruction in science by Gerard et al. (2011) also highlighted the importance of access to expertise through facilitators and more senior teachers.
2.14. Design principle #7: co-design with teachers
To enact high-quality, useable PD, it is essential to capitalize on teacher practitioner knowledge and build on the experiences teachers bring to PD from the classroom. An area of research that ensures teachers will have these experiences is practice–research partnerships, where teachers and researchers work together to co-design curricular and instructional activities. Co-design work involves opportunities for stakeholders with varying kinds of expertise to work on shared educational products (Penuel et al., 2011; Matuk et al., 2016). Through ongoing collaboration and the sharing of multiple perspectives, knowledge, and skills, co-design models of PD can be beneficial to teachers who can shape instruction so that it is useable in their classroom contexts. Researchers also benefit by understanding what strategies will ultimately be used by teachers and, in turn, optimally support student learning. Ko et al. (2022) described a project called READI with goals similar to ours. They were interested in designing instructional strategies to engage in authentic disciplinary inquiry using explanatory models and data representations that are typically used by the science community. Their co-design model involved periodic convening in a professional learning community over 2 years, negotiation of viewpoints, reflection, and collective adaptation responding to implementation challenges. They documented that teachers and researchers came away with a deeper understanding of the impact of their instructional design and practices on learning.
In the next section, we present a case study of these principles in action as we designed and developed a disease epidemic model and curriculum unit.
3. Applications of the design principles to the construction of a disease epidemic model and unit
We describe activities undertaken in the conceptualization, design, and development of a disease epidemic model and unit for a high school biology class; the activities took place over approximately 3 months from April to July 2022. Readers can find the full set of unit lessons in the “Supplementary materials” section of the journal website. (In current and future work, we are creating a revised iteration of this unit.)
The entire unit is grounded in our commitment to using SSIs to connect students’ science instruction to real-world problems that are relevant to them. We chose the SSI topic of a respiratory disease epidemic because of its high personal relevance to our recent collective and global experiences with COVID. The COVID pandemic is a complex socioscientific topic both in how mitigation strategies emerged from the early days of cleaning surfaces and social distancing to vaccination protocols that were ultimately adopted and produced different health-related population outcomes based on individual choices. We hypothesized that students, as a sample of the broader population, may have developed opinions and practices concerning COVID that vary and may be in conflict with each other. We furthermore believed that learning about how to contain an airborne respiratory virus that students have lived through would invoke considerations of democratic values and personal real-world action.
The unit is underpinned by a challenge that students, assuming the role of research scientists, are given to propose a mitigation protocol for two fictional towns that have just reported a serious disease outbreak to the World Health Organization (WHO). Figures 1–4 display instructional slides that teachers use to introduce the challenge. Figure 1 provides some background information about the WHO to promote its legitimacy as a United Nations–sanctioned scientific organization. Figure 2 provides details of the disease outbreak reported to the WHO. Figure 3 gives initial details of the simulation that teams of scientists (students) will use to conduct their investigations. Figure 4 outlines the task that science teams are given to inform WHO decision making. The specific research question given to students is, “What are the best recommendations of mitigation strategies for the public to stop the spread of this disease and how did you arrive at your conclusions?” Throughout the unit, which spans 8 lessons lasting 1–2 h each, students are introduced to activities that engage their scientist teams in answering the research question. Lesson 1, “Why Model?” engages students in understanding why scientists model phenomena and asks them to develop a set of criteria for good models. In this lesson, they are given toy cars with a gear mechanism in them that, when pulled back, can be propelled to run forward on their own. Students develop explanations and models for how the cars operate using evidence that each team has collected from their explorations. The models and explanations are then shared with other teams for critique. The goal of this lesson is to come to consensus on a class set of criteria for good models to be used during the rest of the unit. Figure 5 shows an example of one team’s model. Figure 6 provides a screenshot of one class’s set of criteria for good models.
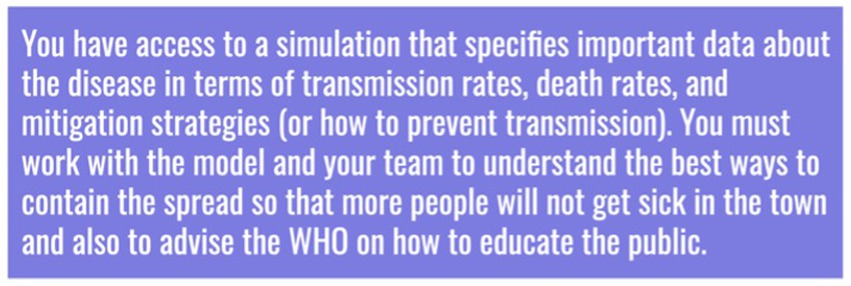
Figure 3. Details of the disease simulation that will be used by science teams (students) to investigate the outbreak.
Lesson 2 introduces students to the epidemic model and fictional problem to be solved. Lessons 3 through 6 engage student teams in a scaffolded set of experiences that aim to develop epistemic practices [discussed in more detail in sections “Using the AIR framework to establish the epistemic practices of coordinating explanations with evidence and generating good evidence (design principle #3: emphasize epistemic performance of science)” and “Curriculum focused on developing epistemic practices (design principle #4: prioritize scientific practices over science concepts)”]. These practices are using reliable strategies, testing the model, conducting productive disagreements, and making sense of the class data. Lesson 7 is organized around a class conference that requires student teams to construct and deliver a research poster with sections that detail their methods of data collection, results, and conclusions that state their response to the research question. After teams present their research, other teams are invited to critique the methods. After the conference, students are asked to modify their recommendations based on what they learned from the research of other teams. Lesson 8, the final lesson in the unit, examines the idea of epistemic practices and how they shape public understanding of science. In this examination, students are asked to reflect on what are good and not-so-good scientific practices. Figure 7 provides a screenshot of a summary slide teachers present to students.
3.1. The StarLogo disease epidemic model (design principle #1: promote learning through complex systems modeling)
Using the StarLogo agent-based modeling platform, upon which our previous complex systems biological models were constructed, we created a disease epidemic model that simulated the spread of an airborne respiratory virus among people in a town. In the simulation, the people are represented by spheres roaming around and interacting with each other. People are color coded to show uninfected people as yellow; infected people as red; recovered people as blue; and deceased people as gray. The simulation includes mitigation strategy buttons that users can manipulate by clicking on them, which then activates an adjacent label to change the status as “on.” Mitigation strategies are programmed to be followed by agents at variable rates simulating a percentage of people who will implement those mitigations at varying degrees of effectiveness (e.g., will not wash their hands thoroughly). Additionally, for some mitigation strategies, students can use programmed sliders to select the percentage in the population who will be compliant. Variability in the simulation is also exhibited through parameterized traits that can be changed in the code. For example, when virtual work/school is turned on, most of the population will be in lockdown. However, certain agents will represent essential workers and continue to go to work/school (the percentage of the population that this impacts can be changed). Users can also set the average size of peoples’ social circles, which impacts the number of people with whom they are likely to come into contact. There is also a status bar accompanying each person that aligns with the mitigation strategies activated. For example, when handwashing is turned on, red on the status bar indicates that the person is engaging in handwashing.
Other features of the simulation that allow users to see the whole system and to analyze data from experimental activities include a graph for users to observe population changes in response to how mitigation strategies are shaping the spread of the disease. They can also click on the table view to get specific population numbers at any point in time during a run of the simulation. At the end of a run, students are able to download the data table as a CSV file. This file can then be exported into Excel and used to ascertain data trends for eventual use as evidence to support claims about the best set of mitigation strategies to contain the spread of the virus. To understand how people in the system are programmed to have variable traits (even within characteristics of the population that the user can change, such as percentage of the population that gets the vaccine), and to understand how people have been programmed to interact with each other, users can look behind the qualitative simulation at the computational code. These three representations—the qualitative simulation, the mathematical tools, and the computational code—provide users with different cognitive tools to both understand system function and engage in experimental activities to make predictions. Figure 8 provides a screenshot of the simulation and the mathematical representations. Images spotlighted in the circle show the people and their status bars, with one person infected and colored red; one person deceased and colored gray; one person recovered and colored blue; and one person uninfected and colored yellow. The larger rectangle spotlights an image of mitigation strategies with other manipulatable factors to its left. The graph in the lower left contains a smaller rectangle spotlighting how students can recognize the trend lines as representing the overall population health. Users can also pull up a data table (lower right) that enables them to view and record their data over time. Figure 9 provides a screenshot of one portion of the computational code. This block coding is visible to students directly beneath the simulation. The code spotlighted in the rectangle enables or disables a mask mandate within the simulation. The code shows that the strategy can be toggled on or off with a slider, where users can select the percent of people who wear masks in the event of activation. The last row of block code shows that there is random variation regarding the effectiveness, in terms of preventing spread of the virus, of an individual person’s mask wearing.
3.2. Developing ideals for good models and reliable processes for conducting research (design principle #2: understanding why science is reliable)
In Lessons 1, students in each class engage in a community activity of developing ideals or criteria for good models (Chinn et al., 2014). The activity begins with students tasked with developing a model that explains what propels toy cars they are given; the mechanism cannot be observed so that students need to conduct a variety of experiments to try to work out possible mechanisms. After each group develops their models, students evaluate each other’s models in a gallery walk, and they write down their thoughts about characteristics of good models that are spurred by their reflections on all the models. At this point, the teacher leads a class discussion in which students take the lead in developing a list of criteria (equivalent to ideals in the AIR model) for good models. The lists may include criteria such as fitting all the evidence, giving an explanation, showing all the steps, being understandable, and the like. Students then apply these criteria to their subsequent modeling work both inside and outside the epidemic unit; they subsequently use the criteria to evaluate their own and their peers’ models, and they can revise them over time to make them better. Through class discussions, teachers help students see that the use of shared criteria like these is critical to advancing knowledge, both in their own class and in science as a whole.
Subsequent to this, students receive five empirical studies that investigate how an epidemic is spread. These studies vary widely in methodological quality, and students discuss what makes different studies better or worse. On the basis of this, students develop a class list of characteristics of good methods (equivalent to reliable methodological processes in the AIR model) that is parallel to their list of criteria for good models (e.g., avoiding confounds, including comparison groups, having a big enough sample). As we discuss below, students apply their list to evaluate their own and their peers’ planned experiments. Again, through class discussions, teachers help students appreciate how the use of methodological norms benefits both their own knowledge construction in class and, analogously through norms of peer review, the knowledge construction of science.
These are two examples of elements built into the unit that help students grasp some of the specific practices that help render scientific findings trustworthy.
3.3. Using the AIR framework to establish the epistemic practices of coordinating explanations with evidence and generating good evidence (design principle #3: emphasize epistemic performance of science)
Given the use of complex systems models, we chose to focus on developing two clusters of epistemic practices—namely, coordinating explanations with evidence and generating good evidence. Before designing the activities for students, our research team used the AIR framework to identify aims, ideals, and reliable processes within each cluster that are useful both in science class and in reasoning about scientific matters outside of school.
For the practice of coordinating explanations with evidence, the aim is to coordinate models, explanations, and/or theories with evidence. The ideal used to judge if a given model represents the world accurately is for the model to fit with all good evidence or at least as much good evidence as possible. This practice includes the following reliable processes that together can be used to both develop and evaluate models:
• Develop arguments with evidence-based reasons.
• Seek and use ample evidence.
• Evaluate evidence consistently.
• Revise beliefs to fit new evidence.
• Compare and contrast findings with others (to make sure that you are considering all the evidence that everyone has gathered).
For the practice of generating good evidence, the aim is straightforward: to develop good evidence. The practice includes two ideals to determine whether evidence is good—namely, that good evidence should be relevant and conclusive, meaning that it sufficiently rules out other explanations. The suggested reliable processes for generating good evidence are as follows:
• Run systematic experiments that control variables.
• Generate or consider multiple hypotheses (to help reduce the effect of confirmation bias).
• Make multiple predictions based on the consideration of multiple hypotheses.
• Generate enough data (in terms of sample size, multiple trials, etc.).
Within the unit, teachers are encouraged to help students make connections between the aims, ideals, and processes they use while working with the StarLogo simulations and those they can use when thinking about science in the real world (see also Section 3.6 below). For example, teachers help students notice that their own processes of changing their models to fit new evidence they have gathered is exactly parallel with what scientists do, which is why scientific knowledge regularly changes.
3.4. Curriculum focused on developing epistemic practices (design principle #4: prioritize scientific practices over science concepts)
As noted earlier, Lessons 3 through 6 were crafted to enable students’ development of the two selected epistemic practices related to modeling and evidence. In this section, we provide details of students’ curricular experiences in developing these practices.
3.4.1. Lesson 3: the historical case of Alexander Fleming
In this lesson, students are presented with Dr. Alexander Fleming’s historical case of the discovery of penicillin. Students focus on the processes Fleming undertook to ensure the reliability of his findings and the sound scientific strategies Fleming used to draw conclusions, which included conducting controlled experiments to eliminate confounding variables, collecting ample evidence to draw conclusions by using multiple test subjects (large sample size), using multiple experimental approaches, conducting a literature review, and receiving expert consultation. As students read, analyze, and justify Fleming’s case, they are exposed to an ideal model that fits with all the good evidence, and they gain familiarity with the reliable processes of seeking and using ample evidence and running systematic experiments that control variables.
3.4.2. Lesson 4: creating and carrying out experimental designs with peer review of methods
Using the disease epidemic model, students are asked to design experiments to answer a prompt asking which mitigation strategy would be the best recommendation for their town. Students are asked to record their methods and to justify each step. This lesson aims to provide students with opportunities to use the knowledge they gained about reliable processes from the Alexander Fleming case, such as seeking and using ample evidence, conducting systematic experiments that control variables, and focusing on generating enough data. In this lesson, student also evaluate their classmate’s work using the criteria for good models that they consensually developed in Lesson 1. This evaluation process allows students to engage in the practices of considering multiple hypotheses, critiquing others’ work with arguments and evidence-based reasons and, comparing and contrasting their methods with peers.
3.4.3. Lesson 5: revising methods
In this lesson, students reflect on their peers’ critiques and are asked to revise their initial methods with the aim of conducting reliable and scientifically sound experiments while collecting and recording more data from the simulation. This lesson specifically focuses on revising theories to fit new evidence, as students have to actively re-examine and alter their methods as they consider their peers’ feedback and their class criteria for good models. These activities can help them appreciate the value of getting feedback from peers on methods and then revising those methods to generate more reliable knowledge—a reliable practice that scientists also use (Principle #2: Understanding why science is reliable).
3.4.4. Lesson 6: scientific argumentation and productive disagreement
To edify students’ understanding of how to model epistemic practices, in this lesson they are asked to evaluate other examples of claims and reasoning relative to the evidence that they are presented with. The examples are meant to show potential challenges that arise from not running enough trials or not accounting for confounding variables, while also solidifying the value of clearly and fully communicating claims, evidence, and reasonings. This lesson highlights the importance of developing arguments with evidence-based reasons, evaluating evidence consistently, and generating enough data.
3.5. Aligning with the biology unit on the nature of science (design principle #5: align PD with teachers’ existing curricula)
To address the challenge of navigating institutional mandates, we wanted to ensure that our new curriculum would align with topics already being taught. Because many schools and districts around the country have adopted the NGSS or promote learning related to the goals espoused in the standards, we aimed to build the disease epidemic curriculum to align closely with an NGSS biology topic. We found that the topic with a near-perfect alignment to our unit was the Nature of Science (NOS). Targeting the NGSS science and engineering practices, the standards support the following NOS categories (NGSS Lead States, 2013; p. 431):
• Scientific Investigations Use a Variety of Methods
• Scientific Knowledge Is Based on Empirical Evidence
• Scientific Knowledge Is Open to Revision in Light of New Evidence
• Scientific Models, Laws, Mechanisms, and Theories Explain Natural Phenomena
Each of these categories are broken down into NOS characteristics that students should learn about in different grade bands. For example, in the category of “Scientific Knowledge is Based on Empirical Evidence,” the characteristics include the following for high school students:
• Scientific knowledge is based on empirical evidence.
• Science disciplines share common rules of evidence used to evaluate explanations about natural systems.
• Science includes the process of coordinating patterns of evidence with current theory.
• Scientific arguments are strengthened by multiple lines of evidence supporting a single explanation.
We saw very strong alignment between our curriculum and these NOS categories and characteristics (see pp. 431–432 for the full set https://nap.nationalacademies.org/read/18290/chapter/14#433). We also learned that NOS is the first unit taught in most high school biology classes, and we believed that a strong initial anchoring of learning in epistemic practices would carry over into future biology topics learned in class. In the summer PD workshop, we devoted time to explore with teachers their understanding of NOS, what they taught about NOS in the classroom, and how the goals of the epidemic unit overlapped with their understanding.
3.6. Curating a list of epistemic teacher moves and examining instructional practices (design principle #6: develop high-leverage epistemic teacher moves and routines)
Following the literature on high-leverage practices (HLPs), our team was interested in understanding how to support teachers in developing pedagogies that engage students in epistemic practices. We hypothesized a number of epistemic teacher moves that would work well with our goals of using models for SSI instruction. One category of moves includes discursive comments or callouts while working with models, such as the following:
• Comments about practices of science: Emphasize that disagreement is the vehicle that drives science forward, and that the ideas and arguments that end up eventually being set aside are vital to overall progress. Students are also introduced to some of the specific tactics that scientists use to resolve disagreements, such as carefully scrutinizing methodological differences between experiments to try to account for differing results. (Lesson 5 in the Epidemic Unit has several slides on these points.)
• Comments that link classroom activities to the practices of science: Note the similarity between the reasoning students use in their model activities and what scientists do. Emphasize the value of the reasoning practices that emerge through their modeling activities and in extensions to out-of-school science.
• Iterative and consistent callouts: Call out certain processes iteratively and consistently as you walk around the classroom during inquiry activities (e.g., What is your evidence? How do you know your methods are reliable? Why is one method better than another, and how do you know?) The goal is for students internalize these processes and to engage with them without teacher prompting.
• Be explicit about scientific norms (or criteria) and methodological processes: Discuss critical norms that scientists adhere to (e.g., fitting with all the good evidence or as much good evidence as possible, answering the question, providing an underlying process).
• Model uncertainty: Model not knowing the answers—in other words, being comfortable with being uncomfortable in front of your class and being okay with not knowing all the answers. This models for students the notion that science knowledge evolves and may not be complete at a particular point in time (e.g., what we knew about how COVID spread at different times).
• Model using criteria and processes: As you demonstrate how to use models, do a think aloud (e.g., “If I disable vaccines, how does that affect the population compared to if I disable handwashing? Let us see what I get when I run the model.”).
Another set of teacher moves describes extending reasoning to science outside of school, to real world problems, and to historical cases. Such moves include using news stories and other social media artifacts like tweets. Students can critique the methods in relation to their own epistemic practice and model criteria; incorporate multiple stakeholder opinions; and examine why their differing perspectives may exist. They can also use historical cases of SSIs where the outcome is already known to examine whether the decisions were good or bad and why.
We have modeled where these epistemic teacher moves can be inserted into the epidemic lessons. Figure 10 provides an example of a suggested epistemic move in the presentation notes of Lesson 6. Additionally, using the list, we have worked with teachers in PD to examine their own instructional practices. A common activity in our workshops has been to have teachers watch an excerpt of their classroom implementations and to evaluate whether and how epistemic teacher moves were used. Teachers also watch and comment on each other’s excerpts. The goal of sharing with other teachers is threefold: (a) to garner advice from peers who know the craft of teaching; (b) to learn other teacher moves to add to their teaching repertoires; and (c) to begin to identify a class of HLPs that can routinely be used to support the development of good student epistemic performance. We are continuing to curate this list of epistemic teacher moves in collaboration with our teacher participants.
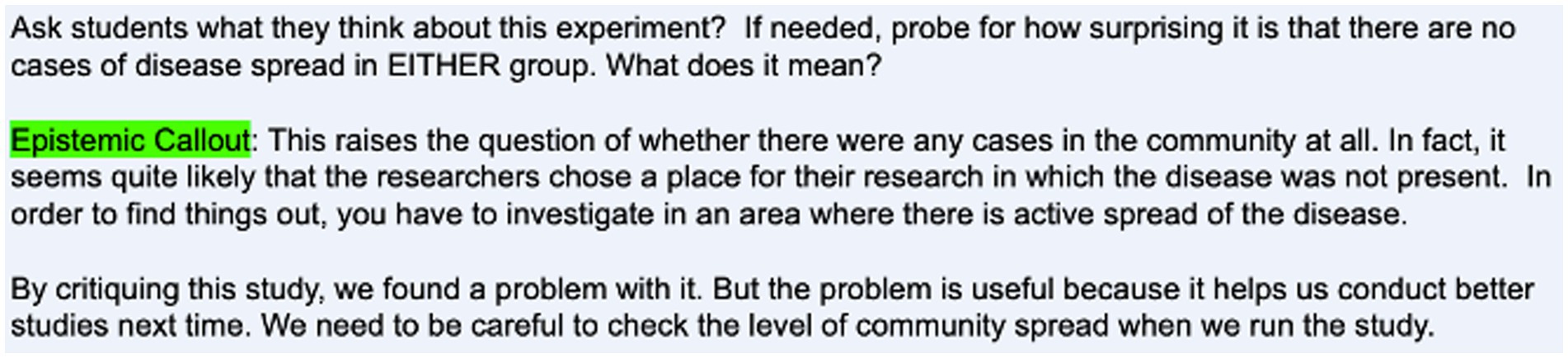
Figure 10. Teacher notes from a lesson 6 slide demonstrating where to insert an epistemic teacher move.
3.7. Co-designing with expert teachers (design principle #7: co-design with teachers)
One of the very fortunate benefits of building on a decade of research is the ability to work with expert teachers with whom we have formed relationships over many years. When we began constructing the epidemic model and unit, we were certain that we could not do this without the partnership of our teachers. We asked 2 teachers (who are also co-authors of this paper) to co-design the unit. They were both peer facilitators in our previous project (Marei et al., 2021) and thus were considered experts in using our complex systems modeling resources. As part of the first cohort of teachers with the present project, they had participated in PD during the summer of 2021 to learn about the next evolution of this work (i.e., incorporating a focus on epistemic performance). They were among the teachers who gave the best feedback on what did and did not work in the PD and how the modeling units could optimally be modified to achieve our teaching and learning goals. Both of them are also expert biology teachers who have taken leadership roles in their schools. Working collectively as a research–practice team, we held several virtual planning sessions in April and May 2022 to brainstorm what disease epidemic to model, how the StarLogo model would function, and how students were likely to use the model. We met with the StarLogo developer (also a co-author on this paper) to work out the design. In June, we convened for a 2-day curriculum-writing workshop in which various members of the research–practice team worked on parts of the curriculum. This co-design with teachers was critical in ensuring that the curriculum aligned with teachers’ prior knowledge and routines related to the Nature of Science biology topic. For example, the aforementioned lessons on using the toy cars and the Alexander Fleming case came directly from the teachers who had previously taught them or similar ones. After the workshop, the teachers took the lead on setting the scope and sequence, laying out the instructional resources for the unit that included a 116-slide teacher presentation and a 23-slide student activity packet. Both teachers instructed in parts of the summer 2022 PD where we rolled out the new model and unit to a larger group of teachers.
4. Conclusion
We are now in the midst of data collection and analysis of classroom implementation of the disease epidemic unit with 14 teachers. Early observations demonstrate high fidelity with the teaching and learning goals espoused in the 7 design principles detailed in this paper. The StarLogo model has provided students with a way to analyze both knowledge claims that they have heard about in the media and also established based on their own personal experiences with COVID. In other words, the model has offered a way to see details of the complex system that would normally be hidden to them (e.g., Grotzer and Tutwiler, 2014; Bar-Yam, 2016; Yoon, 2018). They have indeed shown some differing opinions and understanding of the issue that have been used to fuel debate about mitigation strategies. In this way, the use of complex SSI instruction has enabled students to engage in content that is personally relevant to them, to form opinions and make decisions, and to investigate a controversial real-world issue (Zeidler, 2014; Herman, 2018; Hodson, 2020). Each class has also developed their own set of criteria for good models with fairly common criteria across them, such as the fit with good evidence. The focus on using the AIR model in instruction has provided a way to examine the nature of scientific knowledge generation and given students opportunities to critique how it is generated (e.g., Barzilai and Chinn, 2018; Chinn et al., 2020). Observations further show that curricular experiences have shifted from a heavy reliance on biological concepts (Ford, 2008) to the use of scientific practices, such as collecting multiple sets of data and comparing data and claims generated from different teams. Teachers have noted in follow-up school year workshops that building on a unit already being taught in their curriculum has made it easier to integrate. We see this as a good sign that our efforts for curricular alignment have paid off in terms of building coherence for teachers in navigating competing institutional mandates (e.g., Desimone and Garet, 2015). We are also beginning to see some relatively common teacher epistemic moves being used in instruction that can orient students to the importance of employing reliable methods (e.g., “What is your evidence?” and “Show me how this evidence is reliable.”). We furthermore believe that curating a set of epistemic routines from this research will contribute to the literature on HLPs (e.g., Grossman et al., 2009; McDonald et al., 2013; Cohen, 2015) and specifically the nascent literature on how to work with teachers to improve students’ epistemic performance (e.g., Buehl and Fives, 2016; Chinn et al., in press) and delivery of SSI instruction in biology (Owens et al., 2021). Finally, through our ongoing partnership with teachers co-designing the curriculum (e.g., Penuel et al., 2011; Ko et al., 2022) and iterating on that design based on teacher feedback, the project resources are proving to be highly useable, the details of which will be reported in forthcoming publications.
Circling back to the historic challenges facing humanity that we discussed at the beginning of this article, as Rotblat (2002) and Reiss (2020) noted, school science must take a central role in mitigating our anthropogenic risks (Ord, 2020). Doing this kind of work in classrooms poses many design challenges related to the curriculum and inquiry approaches, instructional methods, contextual issues, and PD experiences, among others. The 7 design principles we present here are intended to provide education researchers with a heuristic for addressing those challenges.
Author contributions
SY did the major conceptualization and writing of the manuscript and is PI of the project. CC is co-PI of the project and supported the building and execution of the design principles. NN, TR, HH-A, and KH worked on writing portions of the manuscript. NN and HH-A in addition worked on constructing portions of the curricular unit. AC supported the conceptualization and execution of the design principles. JK and EM participated in co-designing and implementing the unit and constructed a large portion of the curriculum. DW constructed the epidemic model and provided feedback on that section of the manuscript. All authors contributed to the article and approved the submitted version.
Funding
This research was funded by two grants from the U.S. National Science Foundation (DRL #2009803 and DRL #1721003).
Conflict of interest
The authors declare that the research was conducted in the absence of any commercial or financial relationships that could be construed as a potential conflict of interest.
Publisher’s note
All claims expressed in this article are solely those of the authors and do not necessarily represent those of their affiliated organizations, or those of the publisher, the editors and the reviewers. Any product that may be evaluated in this article, or claim that may be made by its manufacturer, is not guaranteed or endorsed by the publisher.
Supplementary material
The Supplementary material for this article can be found online at: https://www.frontiersin.org/articles/10.3389/feduc.2023.1210153/full#supplementary-material
References
Bar-Yam, Y. (2016). From big data to important information. Complexity 21, 73–98. doi: 10.1002/cplx.21785
Barzilai, S., and Chinn, C. A. (2018). On the goals of epistemic education: promoting apt epistemic performance. J. Learn. Sci. 27, 353–389. doi: 10.1080/10508406.2017.1392968
Barzilai, S., and Ka’adan, I. (2017). Learning to integrate divergent information sources: the interplay of epistemic cognition and epistemic metacognition. Metacogn. Learn. 12, 193–232. doi: 10.1007/s11409-016-9165-7
Barzilai, S., Thomm, E., Weinstock, M., and Bauer, J. (2023). Flip-flopping or making progress? Explaining why scientists change their minds. Paper presented at the 2023 Meeting of the Society of Text and Discourse, Oslo, Norway.
Bereiter, C., and Scardamalia, M. (2014). “Knowledge building and knowledge creation: one concept, two hills to climb” in Knowledge creation in education. eds. S. C. Tan and S. J. Yeo (Singapore: Springer.)
Bjornberg, K. E., Karlsson, M., Gilek, M., and Hansson, S. O. (2017). Climate and environmental science denial: a review of the scientific literature published in 1990–2015. J. Clean. Prod. 167, 229–241. doi: 10.1016/j.jclepro.2017.08.066
Buehl, M., and Fives, H. (2016). “The role of epistemic cognition in teacher learning and praxis” in Handbook of epistemic cognition. eds. J. A. Green, W. Sandoval, and I. Bråten (New York: Routledge).
Chan, K. K. (2022). Eliciting and working with student thinking: preservice science teachers' enactment of core practices when orchestrating collaborative group work. J. Res. Sci. Teach. 60, 1014–1052. doi: 10.1002/tea.21823
Chinn, C. A., Barzilai, S., and Duncan, R. G. (2020). Disagreeing about how to know: the instructional value of explorations into knowing. Educ. Psychol. 55, 167–180. doi: 10.1080/00461520.2020.1786387
Chinn, C. A., Duncan, R. G., and Av-Shalom, N. Y. (2021). Applying the grasp-of-evidence framework to design and evaluate epistemically complex learning environments. Inf. Technol. Educ. Learn. 1:p004. doi: 10.12937/itel.1.1.Inv.p004
Chinn, C. A., Rinehart, R. W., and Buckland, L. A. (2014). “Epistemic cognition and evaluating information: applying the AIR model of epistemic cognition” in Processing inaccurate information: theoretical and applied perspectives from cognitive science and the educational sciences. eds. D. Rapp and J. Brassch (Cambridge, MA: MIT Press).
Chinn, C., Yoon, S. A., Hussain-Abidi, H., Hunkar, K., Noushad, N., and Richman, T. (in press). Designing learning environments to promote good thinking in a post-truth world: an example from science education. Eur. J. Educ.
Cohen, J. (2015). Challenges in identifying high-leverage practices. Teach. Coll. Rec. 117, 1–41. doi: 10.1177/016146811511700702
Cottone, A. M., Yoon, S. A., Shim, J., Coulter, B., and Carman, S. (2023). Evaluating apt epistemic processes in support of data literacy in elementary school students. Inst. Sci. 51, 1–37. doi: 10.1007/s11251-022-09610-8
Darling-Hammond, L., Hyler, M. E., and Gardner, M. (2017). Effective teacher professional development. Palo Alto, CA: Learning Policy Institute.
Darner, R. (2019). How can educators confront science denial? Educ. Res. 48, 229–238. doi: 10.3102/0013189X1984
Desimone, L. M., and Garet, M. S. (2015). Best practices in teachers’ professional development in the United States. Psychol. Soc. Educ. 7, 252–263. doi: 10.25115/psye.v7i3.515
Duschl, R. (2008). Science education in three-part harmony: balancing conceptual, epistemic, and social learning goals. Rev. Res. Educ. 32, 268–291. doi: 10.3102/0091732X07309371
Duschl, R. (2020). Practical reasoning and decision making in science: struggles for truth. Educ. Psychol. 55, 187–192. doi: 10.1080/00461520.2020.1784735
Elby, A., Macrander, C., and Hammer, D. (2016). “Epistemic cognition in science: uncovering old roots to turn over new leaves” in Handbook of epistemic cognition. eds. J. A. Green, W. Sandoval, and I. Bråten (New York: Routledge), 113–127.
Feinstein, N. W., and Waddington, D. I. (2020). Individual truth judgments or purposeful, collective sensemaking? Rethinking science education’s response to the post-truth era. Educ. Psychol. 55, 155–166. doi: 10.1080/00461520.2020.1780130
Fensham, P. J. (2012). “Preparing citizens for a complex world: the grand challenge of teaching socio-scientific issues in science education” in Science|environment|health. eds. A. Zeyer and R. Kyburz-Graber (Netherlands: Springer), 7–29.
Fives, H., Barnes, N., Buehl, M. M., Mascadri, J., and Ziegler, N. (2017). Teachers' epistemic cognition in classroom assessment. Educ. Psychol. 52, 270–283. doi: 10.1080/00461520.2017.1323218
Ford, M. (2008). Disciplinary authority and accountability in scientific practice and learning. Sci. Educ. 92, 404–423. doi: 10.1002/sce.20263
Forzani, F. M. (2014). Understanding “core practices” and “practice-based” teacher education: learning from the past. J. Teach. 65, 357–368. doi: 10.1177/002248711453380
Gerard, L. F., Varma, K., Corliss, S. B., and Linn, M. (2011). Professional development for technology-enhanced inquiry science. Rev. Educ. Res. 81, 408–448. doi: 10.3102/003465431141512
Goldman, S. R., Britt, M. A., Brown, W., Cribb, G., George, M., Greenleaf, C., et al. (2016). Disciplinary literacies and learning to read for understanding: a conceptual framework for disciplinary literacy. Educ. Psychol. 51, 219–246. doi: 10.1080/00461520.2016.1168741
Gorman, S. E., and Gorman, J. M. (2021). Denying to the grave. Why we ignore the facts that will save us. Oxford: Oxford University Press.
Grossman, P., Compton, C., Igra, D., Ronfeldt, M., Shahan, E., and Williamson, P. W. (2009). Teaching practice: a cross-professional perspective. Teach. Coll. Rec. 111, 2055–2100. doi: 10.1177/016146810911100905
Grotzer, T. A., and Tutwiler, M. S. (2014). Simplifying causal complexity: how interactions between modes of causal induction and information availability lead to heuristic-driven reasoning. Mind Brain 8, 97–114. doi: 10.1111/mbe.12054
Hatch, T., White, M. E., Raley, J., Austin, K., Capitelli, S., and Faigenbaum, D. (2006). Into the classroom: developing the scholarship of teaching and learning. Hoboken: Jossey-Bass.
Herman, B. C. (2018). Students' environmental NOS views, compassion, intent, and action: impact of place-based socioscientific issues instruction. J. Res. Sci. Teach. 55, 600–638. doi: 10.1002/tea.21433
Hill, H. (2015). Review of the mirage: confronting the hard truth about our quest for teacher development. Boulder, CO: National Education Policy Center.
Hodson, D. (2009). Teaching and learning about science: Language, theories, methods, history, traditions and values. Leiden: Brill.
Hodson, D. (2020). Going beyond STS education: building a curriculum for sociopolitical activism. Can. J. Sci. Math. Technol. Educ. 20, 592–622. doi: 10.1007/s42330-020-00114-6
Hussain-Abidi, H., Hunkar, K., Chinn, C. A., Yoon, S. A., Cottone, A. C., Noushad, N. F., et al. (2023). “Analyzing teacher epistemic moves in science classrooms” in Proceedings of the 17th International Conference of the Learning Sciences-ICLS 2023 (Montreal, Canada: International Society of Learning Sciences).
Ke, L., Sadler, T. D., Zangori, L., and Friedrichsen, P. (2020). Students’ perceptions of engagement in socio- scientific issue-based learning and their appropriation of epistemic tools for systems thinking. Int. J. Sci. Educ. 42, 1339–1361. doi: 10.1080/09500693.2020.17598432020.1759843
Kienhues, D., Jucks, R., and Bromme, R. (2020). Sealing the gateways for post-truthism: reestablishing the epistemic authority of science. Educ. Psych. 55, 144–154. doi: 10.1080/00461520.2020.1784012
Klopfer, E., Scheintaub, H., Huang, W., Wendel, D., and Roque, R. (2009). The simulation cycle: combining games, simulations, engineering and science using StarLogo TNG. E-Learn. Dig. Media 6, 71–96. doi: 10.2304/elea.2009.6.1.71
Kloser, M. (2014). Identifying a core set of science teaching practices: a delphi expert panel approach. J. Res. Sci. Teach. 51, 1185–1217. doi: 10.1002/tea.21171
Ko, M. L. M., Hall, A., and Goldman, S. R. (2022). Making teacher and researcher learning visible: collaborative design as a context for professional growth. Cogn. Instr. 40, 27–54. doi: 10.1080/07370008.2021.2010212
Lampert, M., Franke, M. L., Kazemi, E., Ghousseini, H., Turrou, A. C., Beasley, H., et al. (2013). Keeping it complex: using rehearsals to support novice teacher learning of ambitious teaching. J. Teach. 64, 226–243. doi: 10.1177/0022487112473837
Leung, J. S. C. (2020). A practice-based approach to learning nature of science through socioscientific issues. Res. Sci. Educ. 52, 259–285. doi: 10.1007/s11165-020-09942-w
Lieberman, A., and Mace, D. P. (2010). Making practice public: teacher learning in the 21st century. J. Teach. 61, 77–88. doi: 10.1177/0022487109347319
Makar, K., and Rubin, A. (2018). “Learning about statistical inference” in International handbook of research in statistics education. eds. D. Ben-Zvi and K. Garfield (Singapore: Springer).
Manz, E., and Suárez, E. (2018). Supporting teachers to negotiate uncertainty for science, students, and teaching. Sci. Educ. 102, 771–795. doi: 10.1002/sce.21343
Matuk, C., Gerard, L., Lim-Breitbart, J., and Linn, M. (2016). Gathering requirements for teacher tools: strategies for empowering teachers through co-design. J. Sci. Teach. 27, 79–110. doi: 10.1007/s10972-016-9459-2
Marei, A., Yoon, S. A., Yoo, J., Richman, T., Noushad, N., Miller, K., et al. (2021). Designing feedback systems: Examining a feedback approach to facilitation in an online asynchronous professional development course for high school science teachers. Syst. 9, 1–23. doi: 10.3390/systems9010010
Mayer, R. (2017). Using multi-media for e-learning. J. Comput. Assist. 33, 403–423. doi: 10.1111/jcal.12197
McDonald, M., Kazemi, E., and Kavanagh, S. S. (2013). Core practices and pedagogies of teacher education: a call for a common language and collective activity. J. Teach. 64, 378–386. doi: 10.1177/0022487113493
Metz, S. E., Weisberg, D. S., and Weisberg, M. (2018). Non-scientific criteria for belief sustain counter-scientific beliefs. Cog. Sci. 42, 1477–1503. doi: 10.1111/cogs.12584
Miller, K. M., and Yoon, S. A. (2023). Teaching complexity in biology through agent-based simulations: The relationship between students’ knowledge of complex systems and metamodeling knowledge. Front. Educ. 8. doi: 10.3389/feduc.2023.1198307
Moon, J., Passmore, C., Reiser, B., and Michaels, S. (2014). Beyond comparisons of online versus face-to-face PD. J. Teach. 65, 172–176. doi: 10.1177/0022487113511497
Muis, K. R., Trevors, G., and Chevrier, M. (2016). “Epistemic climate for epistemic change” in Handbook of epistemic cognition. eds. J. A. Green, W. Sandoval, and I. Bråten (New York: Routledge).
Murphy, D., Chinn, C. A., Duncan, R. G., Danish, J., Hmelo-Silver, C. E., Ryan, Z., et al. (2022). Students ideas about evidentiary fit and its role in modeling practice In C. A. Chinn, E. Tan, C. Chan, and Y. Kali (Eds.), Proceedings of the 16th International Conference of the Learning Sciences – ICLS 2022 Hiroshima, Japan: International Society of the Learning Sciences.
NGSS Lead States. (2013). Next generation science standards: for states, by states. Washington: National Academies Press.
Nichols, T. (2017). The death of expertise: The campaign against established knowledge and why it matters. Oxford: Oxford University Press.
Ord, T. (2020). The precipice: Existential risk and the future of humanity. New York: Hachette Books.
Osborne, J., and Pimentel, D. (2022). Science, misinformation, and the role of education. Science 378, 246–248. doi: 10.1126/science.abq8093
Oura, H., Mochizuki, T., Chinn, C. A., and Yamaguchi, E. (2023). Developing undergraduate students’ competence in reasoning about bodies of evidence. In P. Blikstein, et al. (Eds.), Proceedings of the 17th international conference of the learning sciences-ICLS 2023, International Society of Learning Sciences.
Owens, D. C., Sadler, T. D., and Friedrichsen, P. (2021). Teaching practices for enactment of socio-scientific issues instruction: an instrumental case study of an experienced biology teacher. Res. Sci. Educ. 51, 375–398. doi: 10.1007/s11165-018-9799-3
Penuel, W. R., Gallagher, L. P., and Moorthy, S. (2011). Preparing teachers to design sequences of instruction in earth systems science: a comparison of three professional development programs. Am. Educ. Res. J. 48, 996–1025. doi: 10.3102/0002831211410864
Pluta, W. J., Chinn, C. A., and Duncan, R. G. (2011). Learners’ epistemic criteria for good scientific models. J. Res. Sci. Teach. 48, 486–511. doi: 10.1002/TEA.20415
Reiss, M. (2020). Science education in the light of COVID-19. Sci. Educ. 29, 1079–1092. doi: 10.1007/s11191-020-00143-5
Rotblat, J. (2002). Citizenship and science. Phys. Educ. 37, 186–190. doi: 10.1088/0031-9120/37/3/301
Sadler, T. D., Romine, W. L., and Topçu, M. S. (2016). Learning science content through socio-scientific issues-based instruction: a multi-level assessment study. Int. J. Sci. Educ. 38, 1622–1635. doi: 10.1080/09500693.2016.1204481
Sandoval, W. (2014). Science education’s need for a theory of epistemological development. Sci. Educ. 98, 383–387. doi: 10.1002/sce.21107
Schwarz, C. V., and White, B. Y. (2005). Metamodeling knowledge: developing students' understanding of scientific modeling. Cogn. Instrc. 23, 165–205. doi: 10.1207/s1532690xci2302_1
Sherrington, D. (2010). Physics and complexity. Philos. Trans. Royal Soc. 368, 1175–1189. doi: 10.1098/rsta.2009.0208
Sinatra, G.M., and Hoffer, B.K. (2021). Science denial: why it happens and what to do about it. Oxford: Oxford University Press.
Sloman, S., and Fernbach, P. (2017). The knowledge illusion: Why we never think alone. New York, NY: Riverhead Books.
TNTP. (2015). The mirage: confronting the hard truth about our quest for teacher development. Available at: https://tntp.org/assets/documents/tntp-mirage_2015.pdf
Wilensky, U., and Rand, W. (2015). An introduction to agent-based modeling: modeling natural, social, and engineered complex systems with NetLogo. Cambridge, MA: MIT Press.
Wilson, S. M. (2013). Professional development for science teachers. Science 340, 310–313. doi: 10.1126/science.1230725
Yoon, S. (2008). An evolutionary approach to harnessing complex systems thinking in the science and technology classroom. Int. J. Sci. Educ. 30, 1–32. doi: 10.1080/09500690601101672
Yoon, S., Anderson, E., Koehler-Yom, J., Sheldon, J., Schoenfeld, I., Wendel, D., et al. (2015). Design features for computer-supported complex systems learning and teaching in high school science classrooms. J. Res. STEM Educ. 1, 17–30.
Yoon, S. A., Evans, D., Miller, K., Anderson, M., and Koehler, J. (2019). Validating a model for assessing science teacher’s adaptive expertise with computer-supported complex systems curricula and its relationship to student learning outcomes. J. Sci. Teach. Educ. 30, 890–905. doi: 10.1080/1046560X.2019.1646063
Yoon, S., Klopfer, E., Anderson, E., Koehler-Yom, J., Sheldon, J., Schoenfeld, I., et al. (2016). Designing computer-supported complex systems curricula for the Next Generation Science Standards in high school science classrooms. Syst. 4, 1–18. doi: 10.3390/systems4040038
Yoon, S., Anderson, E., Koehler-Yom, J., Evans, C., Park, M. J., Sheldon, J., et al. (2017). Teaching about complex systems is no simple matter: Building effective professional development for computer-supported complex systems instruction. Inst. Sci. 45, 99–121. doi: 10.1007/s11251-016-9388-7
Yoon, S. (2018). “Complex systems and the Learning Sciences: Implications for learning, theory, and methodologies” in The International Handbook of the Learning Sciences. eds. F. Fischer, C. Hmelo-Silver, S. Goldman, and P. Reimann (New York, NY: Routledge Press), 157–166.
Yoon, S., Goh, S., and Park, M. (2018). Teaching and learning about complex systems in K–12 science education: A review of empirical studies 1995–2015. Rev. Educ. Res. 88, 285–325. doi: 10.3102/00346543177460
Yoon, S. A., Miller, K., Richman, T., Wendel, D., Schoenfeld, I., Anderson, E., et al. (2020). Encouraging collaboration and building community in online asynchronous professional development: Designing for social capital. Int. J. Comput.-Support. Collab. Learn. 15, 351–371.
Keywords: complex systems, socioscientific issues, post-truth, science epistemic practices, agent-based modeling, biology, teacher professional development, K-12 curriculum
Citation: Yoon SA, Chinn C, Noushad N, Richman T, Hussain-Abidi H, Hunkar K, Cottone A, Katz J, Mitkus E and Wendel D (2023) Seven design principles for teaching complex socioscientific issues: the design of a complex systems agent-based disease epidemic model and the application of epistemic practices in high school biology. Front. Educ. 8:1210153. doi: 10.3389/feduc.2023.1210153
Edited by:
Tammy M. Long, Michigan State University, United StatesReviewed by:
Ian Thacker, University of Texas at San Antonio, United StatesBenedikt Heuckmann, University of Münster, Germany
Copyright © 2023 Yoon, Chinn, Noushad, Richman, Hussain-Abidi, Hunkar, Cottone, Katz, Mitkus and Wendel. This is an open-access article distributed under the terms of the Creative Commons Attribution License (CC BY). The use, distribution or reproduction in other forums is permitted, provided the original author(s) and the copyright owner(s) are credited and that the original publication in this journal is cited, in accordance with accepted academic practice. No use, distribution or reproduction is permitted which does not comply with these terms.
*Correspondence: Susan A. Yoon, eW9vbnNhQHVwZW5uLmVkdQ==