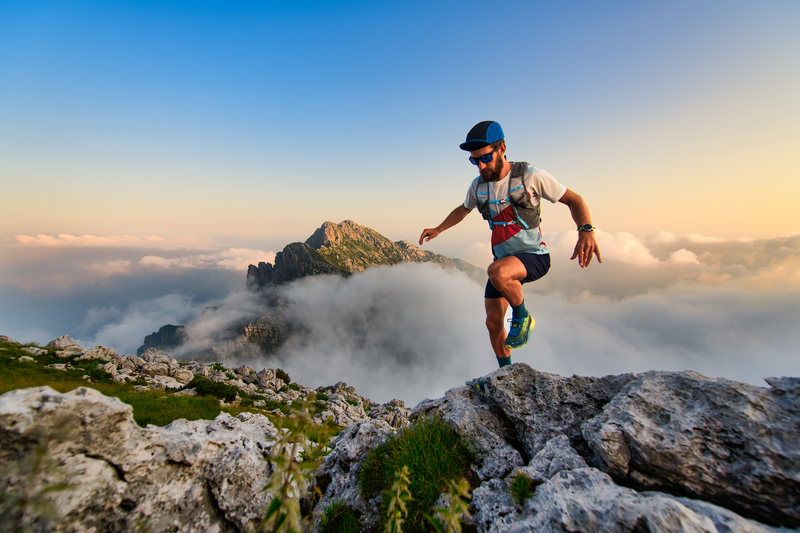
95% of researchers rate our articles as excellent or good
Learn more about the work of our research integrity team to safeguard the quality of each article we publish.
Find out more
ORIGINAL RESEARCH article
Front. Educ. , 15 June 2023
Sec. Language, Culture and Diversity
Volume 8 - 2023 | https://doi.org/10.3389/feduc.2023.1198709
This article is part of the Research Topic Insights in Assessment, Testing, and Applied Measurement: 2022 View all 21 articles
Based on multiple assessment approach, this study used factor analysis and neural network modeling methods to build a data-driven multidimensional assessment model for English listening and speaking courses in higher education. We found that: (1) Peer assessment, student self-assessment, previous academic records, and teacher assessment were the four effective assessors of the multi-dimensional assessment of English listening and speaking courses; (2) The multidimensional assessment model based on the four effective assessors can predict the final academic performance of students in English listening and speaking courses, with previous academic records contributing the most, followed by peer assessment, teacher assessment, and student self-assessment. Therefore, a multidimensional assessment model for English listening and speaking courses in higher education was proposed: the academic performance of students (on a percentage basis) should be composed of 29% previous academic records, 28% peer assessment, 26% teacher assessment, and 17% student self-assessment. This model can guide teachers to intervene with students who need help in a timely manner, based on various assessors, thereby effectively improving their academic performance.
Assessment is one of the most complex cognitive behavior in the cognitive domain of educational goals (Bloom, 1956), and requires a rational and in-depth assessment of the essence of things. Currently, educational assessment is mostly static assessment or standardized testing (normative/standardized assessment; Haywood and Lidz, 2006). The tools and processes used in such assessments are standardized, and individual abilities are represented by statistical numbers (Sternberg and Grigorenko, 2002). The advantage of static assessment lies in its design objectivity, precision, and structuralism. However, it only provides test scores, focuses only on students’ existing abilities, and the teacher is the only assessor. This assessment method is one-sided, easily leading students into a repetition of ineffective rote memorization tactics, seriously undermining students’ learning interest and confidence (Thanh Pham and Renshaw, 2015). The teaching assessment of listening and speaking courses in universities, which are often the first to incorporate new teaching methods such as digital technologies, must evolve from traditional static assessment methods to more comprehensive and accurate ones. Therefore, it is necessary to reform and improve the teaching assessment system of these courses.
The multiple assessment approach (Maki, 2002), based on the theory of multiple intelligences and constructivism, emphasizes the diversity of assessment methods, content, and subjects (Linn, 1994; Messick, 1994; Brennan and Johnson, 1995; Flake et al., 1997; Lane and Stone, 2006; Lane, 2013). Among them, the diversification of assessment subjects refers to the assessment of students by teachers (teacher assessment), student peers (peer assessment), and students themselves (student self-assessment). This is beneficial for expanding the sources of assessment information and potentially improving the reliability and validity of the assessment (Sadler, 1989; Shepard, 2000; Hattie and Timperley, 2007; Li et al., 2019; Ghafoori et al., 2021). The diversification of subjects aims to break the single-subject assessment model, allow more people to participate in the assessment activities, and transform assessment from one-way to multi-directional, to construct an assessment model that combines student self-assessment, peer assessment, and teacher assessment.
Student self-assessment is the learner’s value judgment of his or her own knowledge and ability level (Bailey, 1996); peer assessment is the value judgment of a student’s ability level, course participation, and effort by classmates (Topping et al., 2010); and teacher assessment is the value judgment of a student’s learning situation made by the teacher. Educationalist Rogers believes that true learning can only occur when learners have a clear understanding of learning goals and assessment criteria (Rogers, 1969). Students as the main assessors embody the idea that “assessment is a learning tool” (Sitthiworachart and Joy, 2003), which is conducive to enhancing students’ metacognitive and self-regulation abilities (Nicol, 2010), promoting teachers and students to discover each other’s strengths and weaknesses, and timely improving temporary shortcomings. However, when using these three sources of assessment, we must be cautious about potential biases, such as reliability, grading, social response bias, response style, and trust/respect (Dunning et al., 2004; van Gennip et al., 2009; Van Gennip et al., 2010; Brown et al., 2015; Panadero, 2016; Meissel et al., 2017). To ensure optimal conditions for accuracy and avoid known pitfalls, both teachers and students should strive to be as objective as possible when evaluating performance. This highlights the importance of having a comprehensive assessment system rather than relying on a one-sided system.
Research on the diversification of assessment subjects has mainly focused on exploring the relationship between the three types of assessment mentioned above (To and Panadero, 2019; Xie and Guo, 2022). Studies showed that the relationships between the assessment subjects are weak (Boud and Falchikov, 1989; Falchikov and Boud, 1989; Falchikov and Goldfinch, 2000; Chang et al., 2012; Brown and Harris, 2013; Double et al., 2020; Yan et al., 2022). For instance, the results of student self-assessment and peer assessment were not consistent with the assessments given by teachers (Goldfinch and Raeside, 1990; Kwan and Leung, 1996; Tsai et al., 2002). Some studies have found that 39% of students overestimate their performance (Sullivan and Hall, 1997), while other studies have found that students’ self-assessment scores were significantly lower than the scores given by teachers(Cassidy, 2007; Lew et al., 2009; Matsuno, 2009). The results of these studies were influenced by the type of task and the individual characteristics of the learners, and these factors need to be considered when interpreting the results. This allows for the possibility of conducting a factor analysis to differentiate assessments from the various assessors involved.
In addition to the factors related to the assessment subjects, students’ previous academic performance is also a key factor that influences their current academic performance due to the cumulative effect of learning (Plant et al., 2005; Brown et al., 2008). As a student progresses through their education, the knowledge and skills they acquire build upon each other. Thus, if a student struggles in a prerequisite course or fails to master certain concepts, it can hinder their ability to succeed in subsequent courses. Additionally, a student’s previous academic performance can affect their confidence and motivation, which can in turn impact their current academic performance (Ciarrochi et al., 2007). There are situations where previous performance has a stronger influence than other predictors, creating what is known as an autoregressive relationship (Biesanz, 2012). Overall, previous academic performance can serve as an assessor of future academic success, highlighting the importance of consistent effort and dedication in one’s education. Therefore, in this study, we also included previous academic performance in the construction of the multidimensional assessment model.
With the increase of assessors in the assessment system, it is a more meaningful research problem to mine the association of various assessors in the data to provide decision-making guidance for education. Currently, in the field of computer science, the representative methods for data dimensionality reduction and modeling are factor analysis (Kim et al., 1978) and machine learning-based predictive modeling (Alpaydin, 2016). This study used the two methods to reduce dimensionality and model different sources of assessment information, and discovered patterns in complex data.
Factor analysis is a multivariate statistical method that can screen out the most influential factors from numerous items and use these factors to explain the most observed facts, thus revealing the essential connections between things (Tweedie and Harald Baayen, 1998). Factor analysis has a long history of successful application in corresponding education research (Cudeck and MacCallum, 2007). For instance, in China scholars analyzed various items that affect students’ comprehensive quality using factor analysis, calculated each student’s comprehensive score, and compared it with traditional assessment methods. They found that this method can make up for the shortcomings of relying solely on Grade Point Average (GPA) (Chang and Lu, 2010).
The application of machine learning-based predictive modeling methods in assessment studies has also become increasingly widespread. Among them, the neural network model, inspired by the structure of the human brain neuron, can simultaneously include multiple predictive variables in the model and calculate the contribution of variables to the model (Lecun et al., 2015). It is a multilayer perceptron. During the training phase, the connections between layers are assigned different weights. The hidden layer(s) also performs a kind of dimensionality reduction (like PCA) which helps to learn the most relevant of the many (correlated) features. It can implicitly detect all possible (linear or nonlinear) interactions between predictors which is advantageous over general linear regression models when dealing with complex stimulus–response environments (e.g., Tu, 1996). Scholars found that compared to the regression methods, the deep learning-based models were more effective in predicting students’ performance (Okubo et al., 2017; Kim et al., 2018). Online English teaching assistance system, using decision tree algorithms and neural network models, was also implemented, which improved the efficiency of teaching (Fancsali et al., 2018; Zheng et al., 2019; Sun et al., 2021). However, the hidden layer(s) likes a black box, as the common factors inside cannot be directly observed. Therefore, it is necessary to combine with other methods to “unbox” the intermediate stage.
Factor analysis and machine learning methods have different approaches, but they can be combined to improve the interpretability of the model. For instance, factor analysis can be used as a pre-processing step to simplify the input items before feeding them into a machine learning model. This reduces model complexity and clearly extracts factors and their constituent components. Machine learning methods can improve the predictive accuracy, aiding in interpreting the factor analysis results. The combination of the two methods has been used in various fields (Nefeslioglu et al., 2008; Marzouk and Elkadi, 2016), including education (Suleiman et al., 2019). Hence, this study attempts to combine factor analysis and machine learning methods in assessing English listening and speaking courses in universities to investigate the feasibility of using a multidimensional assessment model in these courses.
As mentioned, this study aims to explore effective assessment methods for English listening and speaking courses in higher education and construct a multidimensional assessment model. Specifically, it has two main research questions: (1) What are the key factors of the multidimensional assessment model? (2) Can the multidimensional assessment model constructed based on these key factors predict students’ academic performance, and what is the significance of each factor in the model?
To address the above issues, we collected assessment data from various sources, including peer ratings of learners’ language abilities and classroom performance, self-ratings by learners, teacher ratings, and previous academic records. With the help of computational science methods, specific assessment factors were extracted from complex data, and the assessment factors were tested to see if they could successfully predict students’ current academic performance (See Figure 1 for an illustration). We hypothesized that: (1) factor analysis can distinguish different sources of assessment data, which can be summarized into four common factors: previous academic records, peer assessment, and teacher assessment, and student self-assessment; (2) the neural network model can use these four common factors to establish a prediction model for students’ academic performance.
Figure 1. Conceptual model of multidimensional assessment. Items from four assessors were collected. Factor analysis was used to identify the key factors, and neural network model was used to construct the multidimensional assessment model based on the key factors.
Sixty-two undergraduate students majoring in English from a university in China were recruited for this study. Among them, there were 55 female and 7 male students, with a mean age of 19.37 years (SDage = 0.71), ranging from 18 to 21 years old. All participants were native Chinese speakers with English as their second language, with a mean age of acquisition (AOA) of 8.65 years (SDAOA = 1.56). Prior to the experiment, all participants signed an informed consent form.
The research tools used in this study were mainly paper-and-pencil materials, including a background survey questionnaire, a student self-assessment scale, a peer assessment scale, a teacher assessment scale, and tests.
• The background survey questionnaire included three items: the English score in the college entrance examination (out of 150 points), the academic grade in the first semester of the listening and speaking course (out of 100 points), and the academic grade in the second semester of the listening and speaking course (out of 100 points).
• The student self-assessment scale required students to assess their own English proficiency, including listening ability, speaking ability, reading ability, and writing ability. It was a five-point Likert scale.
• The peer assessment scale required students to rate their classmates, except for themselves, based on their understanding of their classmates and their performance in the course. The scale included seven items: listening ability, speaking ability, reading ability, writing ability, class participation, cooperation and competitiveness awareness, and learning attitude and perseverance. It was also a five-point Likert scale.
• The teacher assessment scale required teachers to grade each student (out of 100 points) based on their comprehensive performance in the course.
• The tests included regular in-class tests and the final exam. There were seven regular in-class tests, with multiple-choice questions based on IELTS listening and a listening textbook. The average correct rate of the seven quizzes was used to represent the students’ in-class test score (in percentage). The final exam comprised of a combination of randomly selected textbook exercises and TOEFL listening questions. The students’ current academic performance was being evaluated based on the final exam score (out of 100 points).
This study was conducted in the English Listening and Speaking course for undergraduate English majors. The course lasted for 16 weeks, with two class hours per week, taught offline by one teacher. The textbook used in the course was the Viewing, Listening and Speaking, Student Book, authored by Zhang E., Deng Y., and Xu W., published by Shanghai Foreign Language Education Press in January 2020, with an International Standard Book Number of 978–7–5,446-6,080-8.
In the course small group presentation sessions were designed, with each group consisting of 3–4 students, who chose a topic to prepare and present together, thereby enhancing the teacher’s understanding of the students and the students’ understanding of each other. Starting from the seventh week, in-class tests were randomly arranged before class, totaling seven times. At the end of the course, students completed the background survey questionnaire, self-assessment scale, and peer assessment scale. Finally, a final exam was administered, and the teacher evaluated the students’ test scores and rate each student based on his/her classroom performance.
First, the data of 16 items were analyzed using Kaiser-Meyer-Olkin (KMO) test (Kaiser, 1974) and Bartlett’s sphericity test (Stone et al., 2008) in JMP 14 Pro software (SAS Institute Inc., Cary, NC) to determine if the data was the factorability of the data for factor analysis (a KMO value of less than 0.60 indicates unsuitability for factor analysis, and if the null hypothesis of Bartlett’s sphericity test is accepted, factor analysis cannot be performed). Second, items with loading of greater than or equal to 0.30 were determined to be statistically significant. Third, maximum likelihood method and oblimin rotation technique based on a correlation matrix were used to extract the factors and determine the number of factors. Fourth, common factors were extracted, and the factors were named to determine whether they reflected students’ self-assessment, peer assessment, teacher assessment, and previous academic records, respectively.
We also conducted a confirmatory factor analysis using seven items due to the small sample size, which is generally recommended to have at least 10 people per item for factor analysis (Costello and Osborne, 2005). By shrinking the items to seven (Marsh et al., 1998), our sample had approximately 9 people per item. The selected seven items were specifically focused on listening and speaking courses, including academic grades in the first and the second semester, self-assessed listening and speaking ability, peer-assessed listening and speaking ability, and teacher assessment. These items were chosen because they better represented the four hypothesized factors.
Using neural network modeling method in JMP 14 Pro software (SAS Institute Inc., Cary, NC), with the common factors extracted by factor analysis as the predictors (the standardized mean values of the items included in the common factors; e.g., Suhr, 2005, 2006), a predictive model for academic performance was constructed to explore the key assessment predictors affecting academic performance. The specific parameters of the model were as follows: the neural network model had three layers (input layer, hidden layer, and output layer), with three nodes in the hidden layer and a hyperbolic tangent (TanH) activation function. The model learning rate was set at 0.1, the number of boosting models was 10, and the number of tours was 10. In order to address the issue of overfitting, cross-validation was employed in the study. K-fold cross-validation was deemed more suitable when dealing with small sample sizes (Refaeilzadeh et al., 2009). This method divides the data into K subsets, and each of the subsets is used to test the model fit on the remaining data, resulting in K models. The best-performing model, based on test statistics, is selected as the final model. 10-fold cross-validation is typically recommended as it provides the least biased accuracy estimation (Kohavi, 1995).
The feature importance of each predictor in the model (feature importance) was calculated using the dependent resampled inputs method, with values ranging from 0 to 1. A value greater than 0.10 was considered a key factor affecting the outcome variable (Saltelli, 2002; Strobl et al., 2009). To avoid grouping errors in the cross-validation dataset, the 10-fold cross-validation process was repeated 100 times (iterations), and the model fit and the feature importance of predictors reported were the means of these 100 iterations (Were et al., 2015).
The results of the factorability test for factor analysis based on 16 items showed that the KMO value was 0.75, and the Bartlett’s sphericity test was significant (χ2 = 533.56, df = 120, p < 0.001), indicating the validity of conducting factor analysis on the data.
Four factors were extracted using the maximum likelihood method and oblimin rotation technique on a correlation matrix. The extraction was based on the eigenvalues (>1) and the “elbow” on the scree plot (refer to Figure 2) where the item’s load on the common factor reached 0.30. As shown in Table 1, the results of the factor analysis were ideal, with a cumulative explained variance of 74.22%.
The results of the factorability test for factor analysis based on 7 selected items showed that the KMO value was 0.63, and the Bartlett’s sphericity test was significant (χ2 = 156.78, df = 21, p < 0.001), indicating the validity of conducting factor analysis on the data. The four factors identified by the confirmatory factor analysis were shown in Table 2, and the cumulative explained variance of 84.99. Since this analysis was a supplementary analysis to validate the findings based on 16 items, we will continue to use the 16 items in the neural nets model.
As factor analysis cannot directly provide a predictive model for student academic performance, we need to use the neural network model method to build this predictive model on this basis. As shown in Figure 3, using the four standardized common factors obtained from factor analysis (the mean of the items contained in the standardized common factors) as predictor variables, the model fits of the predictive model for the academic performance in the current academic performance of the participants were acceptable (Mean r2training = 0.84, SD r2training = 0.02; Mean r2test = 0.78, SD r2test = 0.14).
Figure 3. Distribution of the model fits of the neural network model. This figure shows the mean r2 values obtained from 100 iterations of the training and test groups, and the error bars represent the standard deviations of 100 iterations.
We further calculated the feature importance of the four common factors in the model (see Figure 4). The results showed that all assessors played a critical role in predicting the academic performance in the current academic performance of the participants (Mean feature importance > 0.10). The feature importance of the four assessors was as follows: previous academic records (Mean = 0.38; SD = 0.09), peer assessment (Mean = 0.36; SD = 0.08), student self-assessment (Mean = 0.22; SD = 0.09), and teacher assessment (Mean = 0.33; SD = 0.09). Among the four assessors, previous academic records, peer assessment, and teacher assessment had a greater contribution than student self-assessment.
Figure 4. Distribution of the feature importance of the assessors in the neural network model. This figure shows the mean feature importance values obtained from 100 iterations, and the error bars represent the standard errors of 100 iterations.
Based on the above results, we preliminarily constructed a multidimensional assessment model for English listening and speaking courses in higher education institutions (as shown in Figure 5).
Figure 5. Distribution of the feature importance of the assessors in the neural network model. This figure shows the mean feature importance values obtained from 100 iterations, and the error bars represent the standard errors of 100 iterations.
The model was composed of a set of 16 assessment items. Based on the results of factor analysis, the four largest common factors that had the most impact were selected. The mean of the items included in the common factors was standardized as the predictive variable of the neural network model, and a predictive model of student academic performance was constructed. Since the contribution of each predictive variable in the original model included both the main effect of the predictive variable and the interaction effect with other variables, the sum of the feature importance was greater than 100%. In order to make the maximum predicted value of academic performance 100 points, we converted the model to a percentage system. While this process was deemed necessary for our study because most courses in China use the centesimal system, it may not be necessary for other studies. The results showed that students’ academic performance (in percentage) should be composed of 29% for previous grades, 28% for peer assessment, 26% for teacher assessment, and 17% for student self-assessment.
The four common factors reveal that the seven assessments from classmates have high loading on factor 1, which reflects the results of peer assessment. Therefore, factor 1 can be named “peer assessment.” The four assessments from student themselves have high loading on factor 2, which reflects the results of student self-assessment. Therefore, factor 2 can be named “self-assessment.” The four items from the first two semesters’ listening and writing grades, the in-class test score and the English college entrance exam score, have high loading on factor 3, reflecting the early academic performance of the participants. Therefore, factor 3 can be named “previous academic records.” The teacher’s assessment has high loading on factor 4, which reflects the rating results of the teacher. Therefore, factor 4 can be named “teacher assessment.” The result indicates that the assessment items from different sources are relatively independent and have a certain level of discriminant validity. The multiple assessments of students’ English listening and speaking courses can be composed of these four factors.
The results of the predictive model built using the neural network method showed that the four assessors (previous academic records, peer assessment, teacher assessment, and self-assessment) could predict student academic performance and all of them were key factors in predicting academic performance (Mean feature importance > 0.10). The order of their feature importance was previous academic records, peer assessment, teacher assessment, and student self-assessment.
The results of the present study indicate the previous academic grades and regular in-class test score had the strongest explanatory power (this may not necessarily hold true for other studies). Both of them were based on paper-and-pencil tests that were similar in form and content to the final exam of the current semester and were familiar to students. Research has shown that previous academic achievement can have a positive impact on learning strategies and motivation through the mediating effect of positive academic emotions (Elias and MacDonald, 2007; Vettori et al., 2020). When students have good previous academic performance, they experience positive emotions such as happiness, pride, and relaxation, which can motivate them to use cognitive strategies more flexibly, which in turn can have a positive impact on their subsequent academic performance.
Peer assessment was based on students’ mutual understanding and can more objectively and comprehensively reflect students’ abilities and daily performance (Shen et al., 2020). This study confirms previous findings that peer assessment scores have high reliability and are significantly correlated with students’ final grades (Li et al., 2016). For the evaluated students, the timely and rich feedback provided by peer assessment helps to avoid deepening confusion and accumulating mistakes. For teachers, peer assessment can to some extent replace teacher assessment, thereby reducing teachers’ workload.
Teacher assessment is also an important predictor of academic performance. Students who have positive and supportive relationships with their teachers are more likely to achieve higher levels of success than those with more conflicted relationships (Aultman et al., 2009). Teachers who report interacting with students more frequently may be better equipped to connect their subject matter to students’ interests. This, in turn, can help teachers to make the subject matter more relatable and engaging for the students, leading to better learning outcomes (Panadero et al., 2017). Based on one semester of communication, teachers may know students well, so they could successfully predict their performance.
Student self-assessment is also a good assessment index for predicting academic performance (Puustinen and Pulkkinen, 2010; Yan and Carless, 2022; Yan et al., 2023), but in this study, its predictive power was the lowest. This may be because individuals find it difficult to make accurate self-assessments of their abilities, for example, self-assessment of abilities such as humor, grammar, and logical reasoning can be easily influenced by other factors (Ferraro, 2010; Park and Santos-Pinto, 2010). Especially in a culture like China, where interdependence is emphasized, the habit of modesty may lead individuals to show self-depreciation when self-evaluating in order to obtain more social approval (Fay et al., 2012).
It is worth noting that the order of the four common factors in factor analysis and the order of the assessors’ feature importance in the neural network model were not consistent. The four common factors extracted by factor analysis were ranked: peer assessment, student self-assessment, previous academic records, and teacher assessment; while the order of the assessors’ feature importance in the neural network model was: previous academic records, peer assessment, teacher assessment, and student self-assessment. The reason for this discrepancy is that factor analysis adds up the loadings of the items contained in the common factor and ranks them according to the total amount. The more items, the higher the ranking. However, the neural network model takes the average and standardized score data of each item in the common factor and inputs it into the model for prediction, so the results obtained may be slightly different.
According to the data-driven multiple assessment model we constructed, the students’ final academic performance in the English Listening and Speaking III course in college can be roughly summarized as follows: Academic performance of the Listening and Speaking III = 29% × Previous academic records (standardized average scores of the college entrance examination English test, Listening and Speaking I, Listening and Speaking II, and in-class tests) + 28% × Peer assessment (standardized average scores of peers’ assessment of listening, speaking, reading, and writing abilities, class participation, cooperation and competitiveness, and learning attitude and perseverance) + 26% × Teacher assessment (standardized teacher ratings) + 17% × Self-Assessment (standardized average scores of students’ self-assessment of listening, speaking, reading, and writing abilities).
This model provides a new solution for course assessment. Practically, teachers can establish their own course assessment methods and assign course scores to students based on the model. Using only the final exam score to evaluate English listening and speaking courses in higher education is not adequate. Learners cannot receive accurate and timely feedback during the learning process, and teachers cannot provide personalized advice for each student. This is not conducive to language learners’ learning. Introducing the theory of multiple assessments into the educational assessment system can promote the theoretical construction and practical development of the assessment system in higher education. Based on the results of this study, we can try to incorporate different assessment subjects into educational assessments, such as allowing students to participate in assessments, and having students themselves and peers rate learners’ language abilities, and presentation skills and classroom performance. During the teaching process, teachers should actively collect data on students’ previous academic records, self-assessment, peer assessment, and teacher assessment to establish a more comprehensive assessment for each student. Before the final exam, predicting students’ learning performance can give more attention to students who may have lower grades, and ensure that each student can achieve satisfactory results in the final exam. Excellent performance in this semester will also have a positive impact on future semesters, forming a virtuous circle.
In this study, factor analysis and neural network models were used to explore the relationships between multiple assessments and academic performance in English listening and speaking courses in higher education. The results showed that factor analysis could sort out assessments from different sources, and the four factors were from the students themselves, their peers, teachers, and previous academic records, respectively. This demonstrated the independence of multiple assessments in practical applications. These four assessors were further incorporated into a predictive model for academic performance, and all of them were found to be important variables for predicting the current academic performance. Therefore, a data-driven multidimensional assessment model for English listening and speaking courses in higher education was constructed. This study actively responded to the demand for interdisciplinary research methods, integrated assessment, teaching, and computer science and technology based on multiple assessment theory, verified the effectiveness of multiple assessments, and provided a reference for the reform of English educational assessment in universities.
However, this study is a preliminary exploration of multiple assessment theory in educational practice, and there are still many shortcomings that need to be addressed through further research. This is mainly reflected in the fact that multiple assessments strive for holistic assessment, emphasizing the diversification of assessment methods, content, subjects, etc. The first limitation is that this study only focused on the diversification of assessment subjects, considering assessments from students, peers, and teachers, but the diversification of assessment methods and contents still requires further research. The second limitation is that due to the small-class setting in China and the avoidance of teacher variances, we only have a small sample size, which may cause the possible lack of statistical power. The third limitation is that we only used the eigenvalue and scree plot to determine the number of factors, which is a poor basis. The forth limitation is that we used a non-refined method to determine the factor scores. It is possible for future studies to refine our proposed method with a larger number of participants.
Moreover, our study focuses on courses that seek to establish a comprehensive assessment system for developing interpersonal abilities, such as speaking or listening skills. For courses that aim to provide fundamental knowledge or skills, such as programming, mathematics, or surgical skills, a comprehensive assessment system may not be urgent.
The raw data supporting the conclusions of this article will be made available by the authors, without undue reservation.
The studies involving human participants were reviewed and approved by The study was conducted in accordance with the Ocean University of China’s policies on Research Ethics for studies involving human participants. The patients/participants provided their written informed consent to participate in this study.
SX: conceptualization and funding acquisition. YJ and HZ: investigation. SX and XX: formal analysis. SX and YS: writing–original draft preparation. SX and SC: writing–review and editing. SC: resources. All authors have read and agreed to the published version of the manuscript.
This research was funded by the Postdoctoral Science Foundation of China [Grant no. 2021M703043], Shandong Provincial Education Science Planning Project [Grant no. 2021WYB005], Shandong Provincial Natural Science Foundation [Grant no. ZR2022QC261], Fundamental Research Funds for the Central Universities of China [Grant no. 202213007], and Chunhui Program of the Ministry of Education of China [Grant no. HZKY20220461].
The authors declare that the research was conducted in the absence of any commercial or financial relationships that could be construed as a potential conflict of interest.
All claims expressed in this article are solely those of the authors and do not necessarily represent those of their affiliated organizations, or those of the publisher, the editors and the reviewers. Any product that may be evaluated in this article, or claim that may be made by its manufacturer, is not guaranteed or endorsed by the publisher.
Aultman, L. P., Williams-Johnson, M. R., and Schutz, P. A. (2009). Boundary dilemmas in teacher–student relationships: struggling with “the line.”. Teach. Teach. Educ. 25, 636–646. doi: 10.1016/J.TATE.2008.10.002
Bailey, K. M. (1996). Working for washback: a review of the washback concept in language testing. Lang. Test. 13, 257–279. doi: 10.1177/026553229601300303
Biesanz, J. C. (2012). “Autoregressive longitudinal models” in Handbook of structural equation modeling. ed. R. H. Hoyle (New York: The Guilford Press), 459–471.
Boud, D., and Falchikov, N. (1989). Quantitative studies of student self-assessment in higher education: a critical analysis of findings. High. Educ. 18, 529–549. doi: 10.1007/BF00138746/METRICS
Brennan, R. L., and Johnson, E. G. (1995). Generalizability of performance assessments. Educ. Meas. Issues Pract. 14, 9–12. doi: 10.1111/J.1745-3992.1995.TB00882.X
Brown, G. T. L., Andrade, H. L., and Chen, F. (2015). Accuracy in student self-assessment: directions and cautions for research. Assess. Educ. 22, 444–457. doi: 10.1080/0969594X.2014.996523
Brown, G. T. L., and Harris, L. R. (2013). “Student self-assessment” in SAGE handbook of research on classroom assessment. ed. J. H. McMillan (Thousand Oaks, CA: Sage), 367–394.
Brown, S. D., Tramayne, S., Hoxha, D., Telander, K., Fan, X., and Lent, R. W. (2008). Social cognitive predictors of college students’ academic performance and persistence: a meta-analytic path analysis. J. Vocat. Behav. 72, 298–308. doi: 10.1016/J.JVB.2007.09.003
Cassidy, S. (2007). Assessing ‘inexperienced’ students’ ability to self-assess: exploring links with learning style and academic personal control. Assess. Eval. High. Educ. 32, 313–330. doi: 10.1080/02602930600896704
Chang, H., and Lu, J. (2010). Multivariate Statistical Analysis Application in the Evaluation of Student’s Synthesis Diathesis. J. Appl. Stat. Manag. 29, 754–760. doi: 10.13860/j.cnki.sltj.2010.04.008
Chang, C. C., Tseng, K. H., and Lou, S. J. (2012). A comparative analysis of the consistency and difference among teacher-assessment, student self-assessment and peer-assessment in a web-based portfolio assessment environment for high school students. Comput. Educ. 58, 303–320. doi: 10.1016/J.COMPEDU.2011.08.005
Ciarrochi, J., Heaven, P. C. L., and Davies, F. (2007). The impact of hope, self-esteem, and attributional style on adolescents’ school grades and emotional well-being: a longitudinal study. J. Res. Pers. 41, 1161–1178. doi: 10.1016/J.JRP.2007.02.001
Costello, A. B., and Osborne, J. (2005). Best practices in exploratory factor analysis: four recommendations for getting the most from your analysis. Pract. Assess. Res. Eval. 10:7. doi: 10.7275/jyj1-4868
Cudeck, R., and MacCallum, R. C. (2007). “Factor analysis at 100: historical developments and future directions” in Factor analysis at 100: Historical developments and future directions. eds. R. Cudeck and R. C. MacCallum (Mahwah, NJ: Lawrence Erlbaum Associates Publishers)
Double, K. S., McGrane, J. A., and Hopfenbeck, T. N. (2020). The impact of peer assessment on academic performance: a Meta-analysis of control group studies. Educ. Psychol. Rev. 32, 481–509. doi: 10.1007/S10648-019-09510-3/FIGURES/4
Dunning, D., Heath, C., and Suls, J. M. (2004). Flawed self-assessment: implications for health, education, and the workplace. Psychol. Sci. Public Interest 5, 69–106. doi: 10.1111/J.1529-1006.2004.00018.X
Elias, S. M., and MacDonald, S. (2007). Using past performance, proxy efficacy, and academic self-efficacy to predict college performance. J. Appl. Soc. Psychol. 37, 2518–2531. doi: 10.1111/J.1559-1816.2007.00268.X
Falchikov, N., and Boud, D. (1989). Student self-assessment in higher education: a meta-analysis. Rev. Educ. Res. 59, 395–430. doi: 10.3102/00346543059004395
Falchikov, N., and Goldfinch, J. (2000). Student peer assessment in higher education: a Meta-analysis comparing peer and teacher Marks. Rev. Educ. Res. 70:287. doi: 10.2307/1170785
Fancsali, S. E., Zheng, G., Tan, Y., Ritter, S., Berman, S. R., and Galyardt, A. (2018). Using embedded formative assessment to predict state summative test scores. ACM International Conference Proceeding Series, pp. 161–170.
Fay, A. J., Jordan, A. H., and Ehrlinger, J. (2012). How social norms promote misleading social feedback and inaccurate self-assessment. Soc. Personal. Psychol. Compass 6, 206–216. doi: 10.1111/J.1751-9004.2011.00420.X
Ferraro, P. J. (2010). Know thyself: competence and self-awareness. Atl. Econ. J. 38, 183–196. doi: 10.1007/S11293-010-9226-2/TABLES/2
Flake, B. S., Hambleton, R. K., and Jaeger, R. M. (1997). A new standard-setting method for performance assessments: the dominant profile judgment method and some field-test results. Educ. Psychol. Meas. 57, 400–411. doi: 10.1177/0013164497057003002
Ghafoori, M., Birjandi, M., and Izadpanah, P. (2021). Self-assessment, peer assessment, teacher assessment and their comparative effect on EFL learners’ second language writing strategy development. J. Engl. Lang. Teach. Learn. Univ. Tabriz 13, 201–216. doi: 10.22034/ELT.2021.48543.2456
Goldfinch, J., and Raeside, R. (1990). Development of a peer assessment technique for obtaining individual marks on a group project. Assess. Eval. High. Educ. 15, 210–231. doi: 10.1080/0260293900150304
Hattie, J., and Timperley, H. (2007). The power of feedback. Rev. Educ. Res. 77, 81–112. doi: 10.3102/003465430298487
Haywood, H. C., and Lidz, C. S. (2006). Dynamic assessment in practice: Clinical and educational applications. Cambridge: Cambridge University Press.
Kaiser, H. F. (1974). An index of factorial simplicity. Psychometrika 39, 31–36. doi: 10.1007/BF02291575/METRICS
Kim, J.-O., Ahtola, O., Spector, P. E., and Mueller, C. W. (1978). Introduction to factor analysis: What it is and how to do it. Thousand Oaks, CA: Sage.
Kim, B. H., Vizitei, E., and Ganapathi, V.. (2018). GritNet: student performance prediction with deep learning. Proceedings of the 11th international conference on educational data mining, EDM. Available at: https://arxiv.org/abs/1804.07405v1.
Kohavi, R. (1995). A study of cross-validation and bootstrap for accuracy estimation and model selection. Appears in the International Joint Conference on Arti Cial Intelligence (IJCAI), pp. 1137–1145. Available at: http://robotics.stanford.edu/~ronnyk
Kwan, K. P., and Leung, R. (1996). Tutor versus peer group assessment of student performance in a simulation training exercise. Assess. Eval. High. Educ. 21, 205–214. doi: 10.1080/0260293960210301
Lane, S. (2013). “Performance assessment in education” in APA Handbook of Testing and Assessment in Psychology. eds. K. F. Geisinger, B. A. Bracken, J. F. Carlson, N. R. Kuncel, S. P. Reise, and M. C. Rodriguez (Washington, DC: American Psychological Association), 329–339.
Lane, S., and Stone, C. A. (2006). “Setting performance standards” in Performance assessment. ed. R. L. Brennan. 4th ed (Westport, Connecticut: Praeger), 387–431.
Lecun, Y., Bengio, Y., and Hinton, G. (2015). Deep learning. Nature 521, 436–444. doi: 10.1038/nature14539
Lew, M. D. N., Alwis, W. A. M., and Schmidt, H. G. (2009). Accuracy of students’ self-assessment and their beliefs about its utility. Assess. Eval. High. Educ. 35, 135–156. doi: 10.1080/02602930802687737
Li, M., Liu, Y., and Zhou, Q. (2016). The analysis of the reliability and characteristics of peer assessment. E-Educ. Res. 9, 48–54. doi: 10.13811/j.cnki.eer.2016.09.008
Li, H., Xiong, Y., Hunter, C. V., Guo, X., and Tywoniw, R. (2019). Does peer assessment promote student learning? A meta-analysis. Assess. Eval. High. Educ. 45, 193–211. doi: 10.1080/02602938.2019.1620679
Linn, R. L. (1994). Performance assessment: policy promises and technical measurement standards. Educ. Res. 23:4. doi: 10.2307/1177043
Maki, P.. (2002). Using multiple assessment methods to explore student learning and development inside and outside of the classroom. NASPA’s Net Results.
Marsh, H. W., Hau, K. T., Balla, J. R., and Grayson, D. (1998). Is more ever too much? The number of indicators per factor in confirmatory factor analysis. Multivar. Behav. Res. 33, 181–220. doi: 10.1207/S15327906MBR3302_1
Marzouk, M., and Elkadi, M. (2016). Estimating water treatment plants costs using factor analysis and artificial neural networks. J. Clean. Prod. 112, 4540–4549. doi: 10.1016/J.JCLEPRO.2015.09.015
Matsuno, S. (2009). Self-, peer-, and teacher-assessments in Japanese university EFL writing classrooms. Lang. Test. 26, 075–100. doi: 10.1177/0265532208097337
Meissel, K., Meyer, F., Yao, E. S., and Rubie-Davies, C. M. (2017). Subjectivity of teacher judgments: exploring student characteristics that influence teacher judgments of student ability. Teach. Teach. Educ. 65, 48–60. doi: 10.1016/J.TATE.2017.02.021
Messick, S. (1994). The interplay of evidence and consequences in the validation of performance assessments. Educ. Res. 23:13. doi: 10.2307/1176219
Nefeslioglu, H. A., Gokceoglu, C., and Sonmez, H. (2008). An assessment on the use of logistic regression and artificial neural networks with different sampling strategies for the preparation of landslide susceptibility maps. Eng. Geol. 97, 171–191. doi: 10.1016/J.ENGGEO.2008.01.004
Nicol, D. (2010). From monologue to dialogue: improving written feedback processes in mass higher education. Assess. Eval. High. Educ. 35, 501–517. doi: 10.1080/02602931003786559
Okubo, F., Shimada, A., Yamashita, T., and Ogata, H. (2017). A neural network approach for students’ performance prediction. ACM International Conference Proceeding Series, 598–599.
Panadero, E. (2016). “Is it safe? Social, interpersonal, and human effects of peer assessment: a review and future directions” in Handbook of human and social conditions in assessment. eds. G. T. L. Brown and L. R. Harris (Abingdon: Routledge), 247–266.
Panadero, E., Jonsson, A., and Botella, J. (2017). Effects of self-assessment on self-regulated learning and self-efficacy: four meta-analyses. Educ. Res. Rev. 22, 74–98. doi: 10.1016/J.EDUREV.2017.08.004
Park, Y. J., and Santos-Pinto, L. (2010). Overconfidence in tournaments: evidence from the field. Theor. Decis. 69, 143–166. doi: 10.1007/S11238-010-9200-0/METRICS
Plant, E. A., Ericsson, K. A., Hill, L., and Asberg, K. (2005). Why study time does not predict grade point average across college students: implications of deliberate practice for academic performance. Contemp. Educ. Psychol. 30, 96–116. doi: 10.1016/J.CEDPSYCH.2004.06.001
Puustinen, M., and Pulkkinen, L. (2010). Models of self-regulated learning: a review. Scand. J. Educ. Res. 45, 269–286. doi: 10.1080/00313830120074206
Refaeilzadeh, P., Tang, L., and Liu, H. (2009). Cross-validation. Encyclopedia of database systems. Berlin: Springer.
Rogers, C. R. (1969). Freedom to learn: A view of what education might become. New York: CE Merrill.
Sadler, D. R. (1989). Formative assessment and the design of instructional systems. Instr. Sci. 18, 119–144. doi: 10.1007/BF00117714/METRICS
Saltelli, A. (2002). Sensitivity analysis for importance assessment. Risk Anal. 22, 579–590. doi: 10.1111/0272-4332.00040
Shen, B., Bai, B., and Xue, W. (2020). The effects of peer assessment on learner autonomy: an empirical study in a Chinese college English writing class. Stud. Educ. Eval. 64:100821. doi: 10.1016/J.STUEDUC.2019.100821
Shepard, L. A. (2000). The role of assessment in a learning culture. Educ. Res. 29, 4–14. doi: 10.3102/0013189X029007004/ASSET/0013189X029007004.FP.PNG_V03
Sitthiworachart, J., and Joy, M. (2003). Web-based peer assessment in learning computer programming. Proceedings 3rd IEEE International Conference on Advanced Learning Technologies, ICALT, 180–184.
Sternberg, R. J., and Grigorenko, E. L. (2002). Dynamic testing: The nature and measurement of learning potential. Cambridge, UK: Cambridge University Press.
Stone, H., Peet, M., Bhadeshia, H. K. D. H., Withers, P., Babu, S. S., and Specht, E. D. (2008). Properties of sufficiency and statistical tests. Proceedings of the Royal Society of London. Series a - mathematical and physical sciences, pp. 1009–1027.
Strobl, C., Malley, J., and Tutz, G. (2009). An introduction to recursive partitioning: rationale, application, and characteristics of classification and regression trees, bagging, and random forests. Psychol. Methods 14, 323–348. doi: 10.1037/a0016973
Suhr, D. D. (2005). Principal component analysis vs. exploratory factor analysis. SUGI 30 proceedings.
Suleiman, S., Lawal, A., Usman, U., Usman Gulumbe, S., and Bui Muhammad, A. (2019). Student’s academic performance prediction using factor analysis based neural network. Int. J. Data Sci. Anal. 5, 61–66. doi: 10.11648/j.ijdsa.20190504.12
Sullivan, K., and Hall, C. (1997). Introducing students to self-assessment. Assess. Eval. High. Educ. 22, 289–305. doi: 10.1080/0260293970220303
Sun, Z., Anbarasan, M., and Praveen Kumar, D. (2021). Design of online intelligent English teaching platform based on artificial intelligence techniques. Comput. Intell. 37, 1166–1180. doi: 10.1111/COIN.12351
Thanh Pham, T. H., and Renshaw, P. (2015). Formative assessment in Confucian heritage culture classrooms: activity theory analysis of tensions, contradictions and hybrid practices. Assess. Eval. High. Educ. 40, 45–59. doi: 10.1080/02602938.2014.886325
To, J., and Panadero, E. (2019). Peer assessment effects on the self-assessment process of first-year undergraduates. Assess. Eval. High. Educ. 44, 920–932. doi: 10.1080/02602938.2018.1548559
Topping, K. J., Smith, E. F., Swanson, I., and Elliot, A. (2010). Formative peer assessment of academic writing between postgraduate students. Assess. Eval. High. Educ. 25, 149–169. doi: 10.1080/713611428
Tsai, C. C., Lin, S. S. J., and Yuan, S. M. (2002). Developing science activities through a networked peer assessment system. Comput. Educ. 38, 241–252. doi: 10.1016/S0360-1315(01)00069-0
Tu, J. V. (1996). Advantages and disadvantages of using artificial neural networks versus logistic regression for predicting medical outcomes. J. Clin. Epidemiol. 49, 1225–1231. doi: 10.1016/S0895-4356(96)00002-9
Tweedie, F. J., and Harald Baayen, R. (1998). How variable may a constant be? Measures of lexical richness in perspective. Comput. Hum. 32, 323–352. doi: 10.1023/A:1001749303137/METRICS
van Gennip, N. A. E., Segers, M. S. R., and Tillema, H. H. (2009). Peer assessment for learning from a social perspective: the influence of interpersonal variables and structural features. Educ. Res. Rev. 4, 41–54. doi: 10.1016/J.EDUREV.2008.11.002
Van Gennip, N. A. E., Segers, M. S. R., and Tillema, H. H. (2010). Peer assessment as a collaborative learning activity: the role of interpersonal variables and conceptions. Learn. Instr. 20, 280–290. doi: 10.1016/J.LEARNINSTRUC.2009.08.010
Vettori, G., Vezzani, C., Pinto, G., and Bigozzi, L. (2020). The predictive role of prior achievements and conceptions of learning in university success: evidence from a retrospective longitudinal study in the Italian context. High. Educ. Res. Dev. 40, 1564–1577. doi: 10.1080/07294360.2020.1817875
Were, K., Bui, D. T., Dick, Ø. B., and Singh, B. R. (2015). A comparative assessment of support vector regression, artificial neural networks, and random forests for predicting and mapping soil organic carbon stocks across an Afromontane landscape. Ecol. Indic. 52, 394–403. doi: 10.1016/J.ECOLIND.2014.12.028
Xie, X., and Guo, J. (2022). Influence of teacher-and-peer support on positive academic emotions in EFL learning: the mediating role of mindfulness. Asia Pac. Educ. Res. 2:665. doi: 10.1007/s40299-022-00665-2
Yan, Z., and Carless, D. (2022). Self-assessment is about more than self: the enabling role of feedback literacy. Assess. Eval. High. Educ. 47, 1116–1128. doi: 10.1080/02602938.2021.2001431
Yan, Z., Lao, H., Panadero, E., Fernández-Castilla, B., Yang, L., and Yang, M. (2022). Effects of self-assessment and peer-assessment interventions on academic performance: a meta-analysis. Educ. Res. Rev. 37:100484. doi: 10.1016/J.EDUREV.2022.100484
Yan, Z., Wang, X., Boud, D., and Lao, H. (2023). The effect of self-assessment on academic performance and the role of explicitness: a meta-analysis. Assess. Eval. High. Educ. 48, 1–15. doi: 10.1080/02602938.2021.2012644
Keywords: assessment methods, English listening and speaking courses, factor analysis, neural network modeling, peer assensment, self assessement, teacher assessment
Citation: Xue S, Xue X, Son YJ, Jiang Y, Zhou H and Chen S (2023) A data-driven multidimensional assessment model for English listening and speaking courses in higher education. Front. Educ. 8:1198709. doi: 10.3389/feduc.2023.1198709
Received: 02 April 2023; Accepted: 30 May 2023;
Published: 15 June 2023.
Edited by:
Gavin T. L. Brown, The University of Auckland, New ZealandReviewed by:
Zhe Li, Osaka University, JapanCopyright © 2023 Xue, Xue, Son, Jiang, Zhou and Chen. This is an open-access article distributed under the terms of the Creative Commons Attribution License (CC BY). The use, distribution or reproduction in other forums is permitted, provided the original author(s) and the copyright owner(s) are credited and that the original publication in this journal is cited, in accordance with accepted academic practice. No use, distribution or reproduction is permitted which does not comply with these terms.
*Correspondence: Shuwei Xue, eHVlc2h1d2VpQG91Yy5lZHUuY24=; Shifa Chen, Y2hlbnNoaWZhOTlAb3VjLmVkdS5jbg==
†These authors have contributed equally to this work and share first authorship
Disclaimer: All claims expressed in this article are solely those of the authors and do not necessarily represent those of their affiliated organizations, or those of the publisher, the editors and the reviewers. Any product that may be evaluated in this article or claim that may be made by its manufacturer is not guaranteed or endorsed by the publisher.
Research integrity at Frontiers
Learn more about the work of our research integrity team to safeguard the quality of each article we publish.