- Biology Education Research and Learning Lab, University of Duisburg-Essen, Essen, Germany
The world is facing global ecological changes, making it essential to prepare the future generation with the necessary understanding to effectively navigate and address complex tasks. Previous research has shown that both systems thinking and scientific modeling are particularly relevant in investigating the comprehensive understanding of such complex phenomena. However, there has been little research on the interrelation between systems thinking and scientific modeling. To address this research gap, we conducted a thinking-aloud study with nine high school students by confronting them with a simulation of a dynamic ecological system. Our qualitative content analysis of the students’ statements indicates an interrelation between systems thinking and scientific modeling. The students infrequently show systems thinking during the exploration, whereas when developing a graphical model, the students are involved in identifying the system organization and analyzing the system behavior. When predicting future system states, students engage in modeling the system evolution. Furthermore, during verbalizing analogies and experiences, students refer to the system organization and behavior, whereas in mental modeling, students additionally model the system evolution. These results illustrate a central difference between the two perspectives. Thus, scientific modeling focuses on students’ activities during their understanding process, while systems thinking addresses students’ analysis of systems and their properties. While the phenomenon exploration may not require systems thinking, pattern recognition and model development are frequently associated with identifying the system organization and analyzing the system behavior. Systems thinking must also be applied when deriving possible future system states by modeling the system evolution, an activity that is closely related to the prediction phase of scientific modeling. Interestingly, in our study, the students also demonstrated the modeling of system evolution in their mental modeling. In conclusion, a complementary consideration of systems thinking and scientific modeling affords a deeper understanding of students’ cognitive processes in dealing with complex phenomena.
1. Introduction
Attention to global changes and its consequences have increased immensely over the last decades. The world is facing global social and environmental issues. One of them is biotic homogenization including several losing and a few winning species (McKinney and Lockwood, 1999). Of particular concern are invasive alien species, as they are a significant threat not only to biodiversity (Early et al., 2016). Invasions negatively affect ecosystem stability and have a direct impact on habitat design, ecosystem performance, agricultural success, the spread of diseases, and human well-being (IPBES, 2019). To deal with such global challenges, a comprehensive understanding of biodiversity and ecosystem functioning is critical for constructive policy and management (Early et al., 2016). Future generations must be prepared for these tasks by developing a holistic understanding of global changes facing the world community (NGSS Lead States, 2013; Ladrera et al., 2020). This issue persists, as the findings of the Programme for International Student Assessment (PISA) reveal that students with little knowledge and skills have naïve and unrealistic ideas about the self-regulation of the world, whereas scientifically educated students are able to make realistic assessments based on ethical reasoning (OECD, 2022). Hence, science education aims to provide students with a set of essential skills and subject-specific concepts that can be used as a basis for dealing with decision-making and problem solving in issues of global concern as well as everyday life challenges (National Research Council, 2012; NGSS Lead States, 2013). Systems thinking and scientific modeling are cognitive activities discussed in the context of a deeper understanding of such complex phenomena in dealing with personal, social, and global challenges (Jackson et al., 1991; Hogan, 2000; Gotwals and Songer, 2010; NGSS Lead States, 2013; Bielik et al., 2021). Both are directly related to understanding complex phenomena, but from different perspectives: Scientific modeling focuses on the process of accessing complex phenomena and primarily addresses how students proceed, whereas systems thinking primarily addresses how students analyze complex systems. Even though it seems plausible that there might be a close relationship between systems thinking and scientific modeling, the connection between the two perspectives has not yet been investigated systematically. This study investigates how students integrate systems thinking and scientific modeling when instructed to develop a model that explains the observed population dynamics of an ecological system invaded by an alien species.
1.1. Systems thinking
Systems thinking is a cognitive skill that provides the theoretical concepts for explaining and predicting natural phenomena (Verhoeff et al., 2018). In recent years, systems thinking has been examined for a variety of systems in manifold contexts, such as ecology (Riess and Mischo, 2010; Eliam and Reisfeld, 2017; Dor-Haim et al., 2022), human physiology (Liu and Hmelo-Silver, 2009; Tripto et al., 2018; Kiesewetter and Schmiemann, 2022), cell biology (Verhoeff et al., 2008), and biogeochemistry (Ben Zvi Assaraf and Orion, 2005; Lee et al., 2019; Torkar and Korfiatis, 2022). All (biological) systems share universal characteristics. For example, systems are composed of system elements that together form a complex whole. Ecosystems encompass the entirety of abiotic and biotic factors within a specific habitat. Despite their interconnectedness, (eco-)systems are open to their environment, allowing for the exchange of matter, energy, and information. Generally, the openness of systems is closely related to the dynamics of systems. Systems with constant composition of elements may exhibit temporary behavioral stability. However, over the long term, system dynamics can be significantly affected by internal factors or external disturbances, such as the presence of alien species as a destabilizing element (Andrade et al., 2015; Mehren et al., 2018). Understanding the complexity of system dynamics can be challenging (Wellmanns and Schmiemann, 2022). This especially applies to ecological systems undergoing degradation, which results in significant modifications to the ecosystem, leading to noticeable changes in species composition and overall dynamics (Zimmerman and Cuddington, 2007).
Several frameworks of systems thinking exist alongside each other (Ben Zvi Assaraf and Orion, 2005, 2010; Hmelo-Silver et al., 2007; Verhoeff et al., 2008; Riess and Mischo, 2010; Sommer and Lücken, 2010; Boersma et al., 2011; Hokayem and Gotwals, 2016; Hmelo-Silver et al., 2017; Snapir et al., 2017; Mehren et al., 2018; Gilissen et al., 2020; Mambrey et al., 2020; Momsen et al., 2022). However, many of these frameworks incorporate similar cognitive skills, even if individual frameworks assume different relationships between these skills or define additional skills (e.g., Ben Zvi Assaraf and Orion, 2005; Mehren et al., 2018). Most systems thinking frameworks consider in some way the following three skills: identifying the system organization, analyzing the system behavior, and modeling the system evolution. The first skill, identifying the system organization, covers recognizing the structure and boundaries of the system as well as identifying the elements and their relationships (Ben Zvi Assaraf and Orion, 2005; Evagorou et al., 2009; Riess and Mischo, 2010; Sommer and Lücken, 2010; Hmelo-Silver et al., 2015; Mehren et al., 2018; Mambrey et al., 2020). The second skill includes analyzing the system behavior by capturing system interactions and dynamics as well as emerging patterns (Ben Zvi Assaraf and Orion, 2005; Hmelo-Silver et al., 2007; Sommer and Lücken, 2010; Mehren et al., 2018; Mambrey et al., 2020). The third skill incorporates modeling the system evolution and thus the development of predictions (Ben Zvi Assaraf and Orion, 2005; Mambrey et al., 2020), possibly to derive regulatory measures (Riess and Mischo, 2010; Mehren et al., 2018). Overall, the naming of the individual skills is consistent with the terminology of Mambrey et al. (2020), who conducted research in the field of ecology, and thus based on the work of Mehren et al. (2018), who originally derived the three listed skills theoretically and empirically tested their model in the context of geography.
In addition to describing individual skills that constitute systems thinking, it is possible and reasonable to consider the complexity of systems thinking (Hokayem and Gotwals, 2016; Lee et al., 2019). Systems can be big or small, and relationships and behaviors may be simple or complex. Notably, there is a considerable difference between the degree of linkage in relationships (Jin et al., 2019; Mambrey et al., 2020, 2022b). Simple relations immediately connect two elements. Whereas relationships that involve a minimum of three elements form either a linear chain or a complex linkage through a central node. This can be well exemplified with a food web and the representation thereof. A statement mentioning rabbits eating grass would name a simple relationship (Mambrey et al., 2022b). This direct predator–prey relationship would be graphically represented by an arrow from prey to predator within a food web. In addition, a typical linear linked relationship is a food chain between a carnivore and a producer. The populations of grass, rabbit, and predator form a food chain as the elements are linked by two arrows in an unbranched manner. In contrast, we define all relationships as complex linked that do not represent mere chains but contain at least one branch (Mambrey et al., 2022a). The most simple example of a complex relationship is food competition between two herbivores such as rabbit and goose for grass, as the system is branched starting from the grass as central node. Previous research in the context of ecology shows that students often prefer to refer to low linked relationships including a small number of elements as an increased number of elements in a system strongly affects the difficulty of comprehension (Strogatz, 2001; Gotwals and Songer, 2010; Hokayem, 2016; Mehren et al., 2018; Mambrey et al., 2022b).
1.2. Modeling in systems thinking research
Various authors imply that systems thinking is connected in some way with models or modeling (Ben Zvi Assaraf and Knippels, 2022; Bielik et al., 2023). However, very different aspects of modeling have been emphasized in the systems thinking literature: Various frameworks explicitly include modeling as a systems thinking skill, which, however, can describe different skills. For example, modeling can involve inferring statements about future system states but also understanding and/or creating system models (e.g., Sommer and Lücken, 2010; Mehren et al., 2018; Gilissen et al., 2020; Mambrey et al., 2020; Streiling et al., 2021). From a different perspective, models are viewed as representations of complex systems (Streiling et al., 2021; Kiesewetter and Schmiemann, 2022; Mambrey et al., 2022b). In addition, they are the starting point for developing predictions (Gilissen et al., 2020). External representations of students’ mental models are evaluated as expressions of their systems thinking and thus serve as an indicator of skill (Ben Zvi Assaraf and Orion, 2005; Brandstädter et al., 2012; Tripto et al., 2013). From yet another perspective, modeling can be used as an activity and strategy to improve systems thinking (Verhoeff et al., 2008; Wilson et al., 2020). Modeling thus can have a variety of meanings in the context of systems thinking; in this study we focus on the cognitive activity of scientific modeling.
1.3. Scientific modeling
Scientific modeling is a practice aimed at examining and explaining complex phenomena or systems (NGSS Lead States, 2013; Krell et al., 2019; Schwarz et al., 2022). It describes the process of gaining access to phenomena that are not completely accessible without further examination (Krell et al., 2019). Accordingly, the emerging models are not just products but useful tools for investigating phenomena (Gouvea and Passmore, 2017). The process of scientific modeling entails activities of model development, evaluation, and revision (Schwarz and White, 2005), which can occur individually or continuously and in cycles (Oh and Oh, 2011; Campbell et al., 2013; Gilbert and Justi, 2016; Krell et al., 2019). The frameworks of Clement (1989), Krell and Krüger (2016) and Justi and Gilbert (2002) provide a process-oriented conceptualization of modeling activities. Usually, the process of scientific modeling starts with the perception of a real-world phenomenon (Krell et al., 2019), based on which and with the activation of analogies and experiences, a mental model arises (Upmeier zu Belzen et al., 2019), which may be labeled mental (Nersessian, 2008), initial (Clement, 1989), or proto-model (Gilbert and Justi, 2016). The mental model is expressed or externalized in any kind of representation (Justi and Gilbert, 2002; Gilbert and Justi, 2016; Upmeier zu Belzen et al., 2019), which is subsequently evaluated for consistency (Clement, 1989; Krell et al., 2019). Once this evaluation is completed, an epistemological change of perspective may take place: A shift toward the epistemic usage of the model can be accomplished by working beyond the developmental perspective of scientific modeling (Gouvea and Passmore, 2017). This perspective of model utilization is characterized by the performance of empirical tests of model-based predictions (Clement, 1989; Justi and Gilbert, 2002; Giere et al., 2006; Gilbert and Justi, 2016; Krell et al., 2019; Upmeier zu Belzen et al., 2019). Therefore, comparing obtained data and predictions provides information on model fit (Krell et al., 2019). If the predictions turn out to be true, the model has proven its validity, at least within the scope of the predictions, and can fulfill its epistemic aim (Justi and Gilbert, 2002; Gouvea and Passmore, 2017; Krell et al., 2019). If the data do not bear out the prediction, the model needs to be revised or rejected (Clement, 1989; Justi and Gilbert, 2002). This leads back to the process of model development, thereby revealing the cyclic nature of scientific modeling (Schwarz and White, 2005; Oh and Oh, 2011; Krell et al., 2019). However, recent research has demonstrated that this cyclic nature of scientific modeling does not necessarily describe students’ real procedures, as they tend to omit or jump between phases (Knuuttila and Boon, 2011; Meister and Upmeier zu Belzen, 2020; Göhner and Krell, 2022). To investigate the modeling process depicted, Krell et al. (2019) developed a coding manual that allows to analyze student activities during scientific modeling.
1.4. Simulation of complex systems
Exploring complex phenomena, like ecosystems in biology, is an essential part of science education. However, ecosystems are characterized by an effectively infinite number of variables that students cannot capture easily. A common tool to investigate the understanding processes of complex systems is simulations (Eilam, 2012; Grotzer et al., 2015; Hmelo-Silver et al., 2015; Yoon et al., 2018; Streiling et al., 2021). Simulations give access to complex systems, for example, by making the structure and dynamics observable. Due to this, simulations are employed in various studies, although there are major differences regarding the options for manipulation and the research aim. Often, these system simulations are used within interventional settings in order to improve systems thinking (Jordan et al., 2013; Hmelo-Silver et al., 2017; Yoon et al., 2017; Wiebe et al., 2019; Eilam and Omar, 2022; Rachmatullah and Wiebe, 2022; Torkar and Korfiatis, 2022). A slightly different approach is using simulations to foster systems thinking from a modeling perspective (Damelin et al., 2017; Bielik et al., 2022; Bowers et al., 2022). However, digital tools such as simulations can also be used as representations of complex systems within the context of non-interventional studies as integral parts of assessments (Sauvé et al., 2007).
2. Research questions and aim of the study
Given the current biodiversity crisis, understanding complex phenomena is of paramount importance. Systems thinking and scientific modeling are two different but likely interdependent perspectives on understanding complex phenomena. In the context of this study, we sought to examine the relationship between these concurrent perspectives more closely. Therefore, we address the following research questions:
1. Which systems thinking skills do students commonly use while analyzing a simulation of a dynamic ecological system?
2. Which activities of scientific modeling do students commonly use while analyzing a simulation of a dynamic ecological system?
3. How do students’ systems thinking and scientific modeling activities interrelate in terms of co-occurrence during their analysis of a simulation of a dynamic ecological system?
3. Method
3.1. Simulation of a dynamic ecological system
Following these considerations, to engage students with a dynamic ecological phenomenon we have developed an interactive data-driven web application in R (R Core Team, 2022) utilizing the packages Shiny (Chang et al., 2022) and ggplot2 (Wickham, 2016). The web application illustrates the dynamics of an ecological system comprising six different species, including pasture grass, red clover, European rabbit, graylag goose, Canada goose, and red kite through an adjustable line graph showing the relative population sizes and thus their changes over time (see Figure 1). Decisive for the dynamic of the simulation are multiple predator–prey relationships, whereas the individual population sizes are estimated based on coupled differential equations by the R package deSolve (Soetaert et al., 2010). Initially, the simulation includes populations of pasture grass, red clover, European rabbit, graylag goose, and red kite, with the Canada goose being introduced later as an alien species. In consequence of the invasion, the dynamics of the ecological system are clearly disturbed and demonstrate emergent behavior in the extinction of the red kite. The participants’ task was to infer the structure of the underlying food web based on the observed population dynamics. They were explicitly requested to develop a graphical model that explains the observed population trends by stating underlying relationships and how the species behave in relation to each other. Beyond that, the students did not receive any additional information about the species or instructions on how to proceed. The development of a graphical model was intended to ensure that the students keep their own modeling process in mind instead of reasoning on what they immediately (see Rellensmann et al., 2017). Within the web application, the participants could select the displayed time period and species at any time and as often as they wanted. Additionally, they could switch between one line graph for all species and individual graphs for each species (see Figure 1) to make it easier for the participants to examine individual relationships if desired. The correct solution to the students’ task is represented in the food web in Figure 2, which served as basis for programming the simulation.
3.2. Data collection
To gain insights into the thinking process of the participants, we asked them to think aloud while exploring the given phenomenon (Ericsson and Simon, 1993; Zhang and Zhang, 2019). Students were tested individually, which avoids social influences and takes into account that the given scientific modeling category system (Krell et al., 2019) has been proved for individual problem solving, unlike group proceedings (Métrailler et al., 2008). The think-aloud protocol was explained to the participants and practiced on a simple and non-specialized topic before the actual survey started (Oh and Wildemuth, 2016). The students were reminded to think aloud and graphically develop their model during the survey, if necessary. For later evaluation, students’ complete work was recorded (audio, screen recording). In addition, students were requested to develop their models on a tablet computer utilizing a digital whiteboard that enabled them to freely draw and illustrate their ideas (Microsoft Corporation, 2020). Since there was no time limitation, students could end the survey at any point they wanted, whether or not they judged the task to be completed. The mean processing time was M = 43.3 (SD = 17.7) minutes. Before further analysis, the collected data were processed through transcription of the audio files. Transcripts were supplemented by screenshots of the recorded graphical modeling process, where the audio transcript alone appeared to be ambiguous.
3.3. Sample
Nine German upper secondary level school students participated in our study (6 female and 3 male participants). Students’ age ranged from 15 to 18 (M = 16.7) years. One-third of the students attended an advanced biology course, while the others took a basic biology course. Unfortunately, we do not have detailed records of individual students’ current and completed biology teaching topics. However, all students were enrolled in the qualification phase for the Abitur, the highest secondary school diploma and higher education entry certificate in Germany. Ecology is one of three main subject areas in biology courses during this qualification phase. Courses at both levels include concepts regarding anthropogenic impacts on ecosystems and their consequences as well as concepts dealing with biodiversity and population dynamics (Kultusministerkonferenz, 2004). In addition, ecology is also part of the educational standards for the intermediate secondary level. Therefore, all students should in any case be familiar with basic concepts in the realm of ecology, for example regarding ecosystem organization, dynamic processes within ecosystems, and predator–prey relationships (Kultusministerkonferenz, 2005).
3.4. Coding scheme
To evaluate the students’ statements, we performed a qualitative content analysis (Schreier, 2012; Mayring, 2014) using MAXQDA 2022 (VERBI Software, 2022), which is a well-established method for analyzing qualitative data whose value has already been proven in the context of modeling processes and systems thinking approaches (Gogolin and Krüger, 2018; Krell et al., 2019; Wellmanns and Schmiemann, 2022; Mambrey et al., 2022a). For the qualitative content analysis, we developed coding schemes based on the model of systems thinking by Mambrey et al. (2020) and the activities of scientific modeling described by Krell et al. (2019). In order to evaluate students’ systems thinking in detail, we differentiated the levels of students’ systems thinking described by Mambrey et al. (2020). We added multiple subcategories using a deductive approach to account for nuances in students’ systems thinking (Schreier, 2012): We broke the level of direct relations down by distinguishing unidirectional relations and bidirectional relations. The level of linear relations was divided into linear relations bridging one element and linear relations bridging multiple elements. Similarly, we distinguished complex relations bridging one element from complex relations bridging multiple elements (see Mambrey et al., 2020). An overview of the coding scheme is given in Table 1. For the coding of students’ scientific modeling activities, we adjusted the descriptions of the coding framework of Krell et al. (2019) to match our ecological context (Schreier, 2012). In contrast to our procedure for systems thinking, we used an inductive approach to extend the coding framework for scientific modeling by new categories. This inductive extension was necessary for the analysis of scientific modeling activities as the existing framework of Krell et al. (2019), unlike the systems thinking framework of Mambrey et al. (2020), required adaption for the presented multilayered phenomenon in an ecological context. We disclose the final coding frame for students’ scientific modeling in the results section, as it is involved in answering the first research question. New categories are listed in Table 2 and marked with an asterisk.
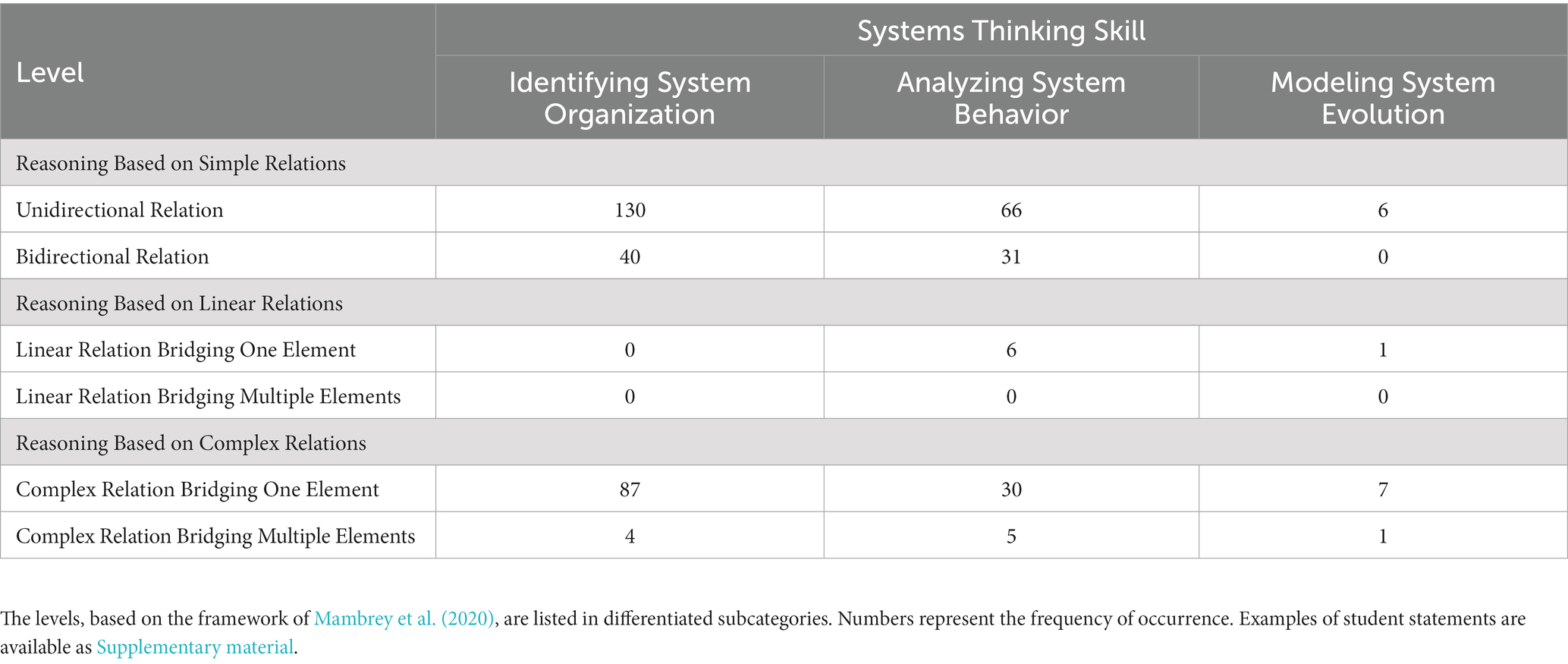
Table 1. Overview of students’ levels of systems thinking in the context of a dynamic ecological system.
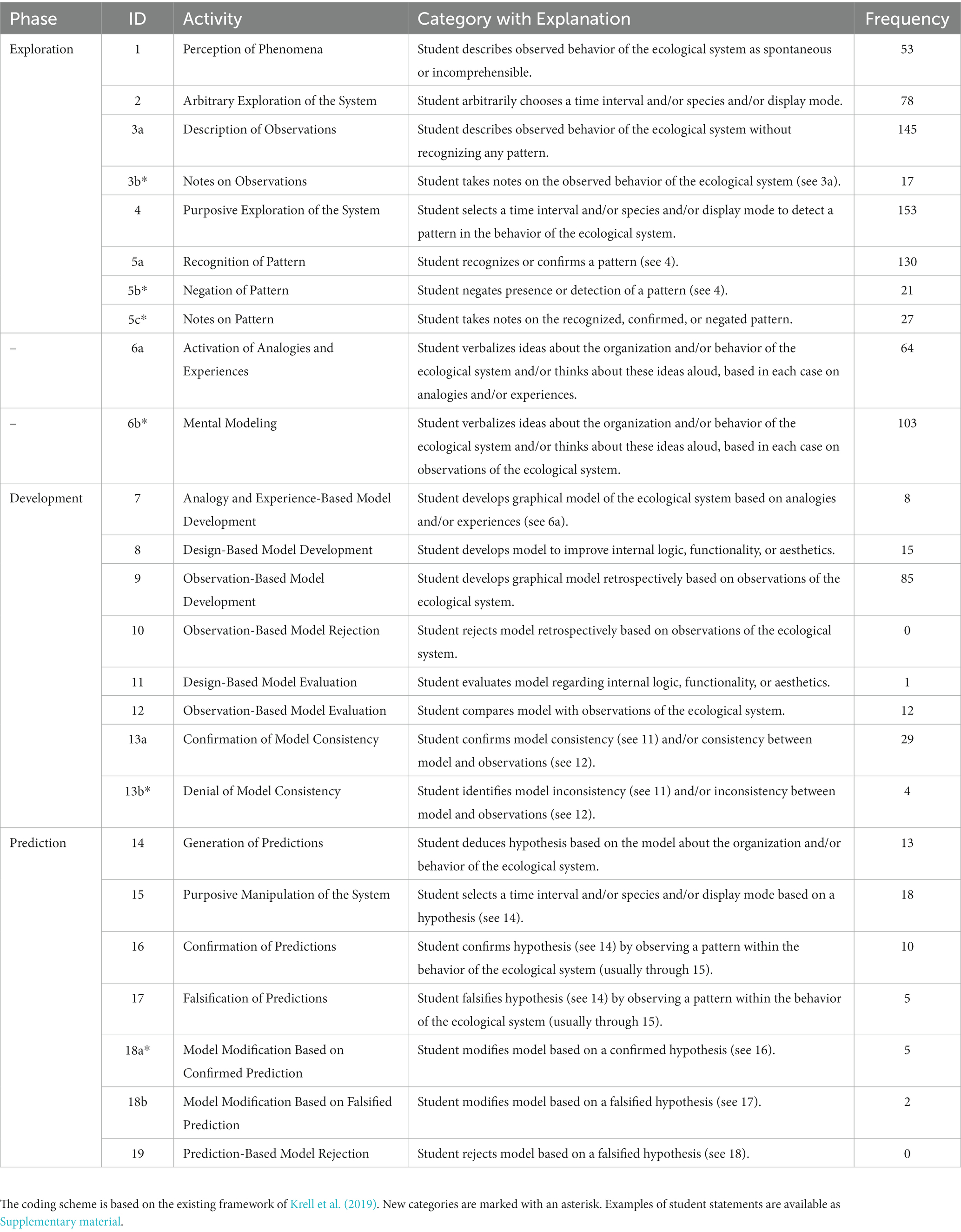
Table 2. Overview of students’ activities in the process of scientific modeling in the context of a dynamic ecological system.
3.5. Data analysis
Before coding the students’ statements, two raters extensively discussed the existing frameworks of Krell et al. (2019) and Mambrey et al. (2020) and adapted them to this study and the ecological context. They then independently coded the data of a test participant and compared their coding result afterwards. Non-matching codes were analyzed, and the coding instructions were refined, as was the inductive differentiation of the category system in scientific modeling. An analysis of inter-rater reliability after re-coding of the test participant’s statements revealed an almost perfect result for our coding schemes, for both systems thinking and scientific modeling (κ > 0.8) (Landis and Koch, 1977). As a result, the coding manual was classified as final and ready to use to code the participants’ statements (Kuckartz and Rädiker, 2019). In the next step, the two raters independently coded all statements of the participating students. To ensure that the complete thinking process is considered, we used event-based coding (Ciesielska et al., 2018), whereas content correctness was no criterion (e.g., Krell et al., 2019). Consistency of the coding was checked by measuring inter-rater reliabilities for each participant and for systems thinking and scientific modeling separately using Kappa (Brennan and Prediger, 1981). In this regard, a coding was scored as matching if the segments of both codings were assigned to the same category and substantially overlapped. Overall, the analysis of the inter-rater reliabilities showed good results: For systems thinking, we achieved values of Kappa between 0.84 and 0.96 (M = 0.88) for eight students, which indicates an almost perfect agreement (Landis and Koch, 1977). For one student, neither rater found any statement related to systems thinking. For scientific modeling, the estimated values of Kappa are in the range of 0.73 and 0.86 (M = 0.79) for the nine students, which may be interpreted as substantial (κ > 0.6) to almost perfect (κ > 0.8) agreement (Landis and Koch, 1977).
To address our first two research questions, we analyzed the observed activities of scientific modeling and systems thinking using absolute and relative frequencies. In order to examine our third research question, which refers to the interrelationship between both perspectives, we created a contingency table. Using MAXQDA’s Code Relations Browser (VERBI Software, 2022), we estimated the intersection of the applied codes of systems thinking with those of scientific modeling to reveal patterns of co-occurrence (Kuckartz and Rädiker, 2019).
4. Results
Overall, we coded 1,412 students’ statements, which included 414 statements regarding systems thinking and 998 statements regarding scientific modeling. The number of segments per student varied widely, ranging from 103 to 292 (M = 173). Statements regarding systems thinking were most frequently (63.0%; absolute frequency of 261) assigned to the skill of identifying the system organization, which means, they describe the type of relationship between two or more species. For instance, one student stated: “I would say now that European rabbits eat pasture grass.” (P. 4, Pos. 183). Also common were statements about analyzing the system behavior at 33.3% (absolute frequency of 138). Statements in this category describe a cause for a change in at least one population size usually by reference to another (changing) population size. An example of such a statement is: “The Canada goose gained quite strongly in population in the following year, which is probably due to the high food supply of pasture grass.” (P. 5, Pos. 93). The third skill of systems thinking, modeling the system evolution, in our context entails developing a forecast of one or more population size changes, such as: “My assumption is that if the [red] kite goes extinct, there must be more and more [European] rabbits because then there are no more predators.” (P. 10, Pos. 287). Statements regarding modeling the system evolution were rare (3.6%; absolute frequency of 15). The evaluation of the named linkage (see Table 1) reveals frequent mentions of simple relations, including unidirectional and bidirectional relations. For instance, one student pointed out the bidirectional predator–prey relation between European rabbits and red clover: “If the number of European rabbits is very low, the red clover can of course grow well and then it still rises here. There are more and more European rabbits because they find more to eat and at a certain point, the climax is reached by red clover [...] and then it [red clover] falls. And then at some point the European rabbit decreases as well.” (P. 10, Pos. 45). Furthermore, students frequently used complex relations, typically bridging one element: “Well, pasture grass and red clover is the food, especially for the European rabbit.” (P. 2, Pos. 12). Whereby complex relations bridging multiple elements were less frequent. Students rarely referred to linear relations bridging one element, like: “Then the European rabbit eats the red clover and if there is a lot of red kite, then there is little of European rabbits, which means that the population [of red clover] increases. And conversely, when there are few European rabbits, there is a lot of red clover.” (P. 5, Pos. 112), whereas linear relations bridging multiple elements were never mentioned. For additional examples of students’ statements on systems thinking see the Supplementary material.
The surveyed activities of scientific modeling were classified as follows: 62.5% exploration (absolute frequency 624), 6.4% activation of analogies and experiences (absolute frequency 64), 10.3% mental modeling (absolute frequency 103), 15.4% development (absolute frequency 154), and 5.3% prediction (absolute frequency 53). In addition to the deductively derived categories (Krell et al., 2019), six categories were added by induction. Five of these categories follow a logical addition (Schreier, 2012). For example, negating statements about pattern, like: “[...] however, bears no resemblance to either of the other two [graphs of red clover and pasture grass], i.e., the European rabbit” (P. 8, Pos. 147), were added in the newly included category 5b: Student negates presence or detection of a pattern. Statements describing a model’s inconsistency and/or inconsistency between model and observation, such as: “That means that [the drawn arrow] makes no sense to me here. The red kite decreases very strongly, very suddenly, and the graylag goose decreases only very slowly.” (P. 3, Pos 192), were covered by the new category 13b. Similarly, student statements about modifying the model based on confirmed hypothesis, like: “This allows us to conclude that the Canada goose feeds a bit on the red clover. [Student writes down] Canada goose eats red clover.” (P. 3, Pos 167–169), were added as affirmation in category 18a. During the survey, students repeatedly took written notes in their representation regarding descriptions and patterns of the ecological system. For example, one student pointed out while observing the system behavior: “I’m writing this down now. Pasture grass increases at 500, then decreases again.” (P. 3, Pos. 55–56). To differentiate these activities from the existing categories, which solely pertain to verbal expressions, we added the categories 3b: Student takes notes on the observed behavior of the ecological system, see previous student statement, and 5c: Student takes notes on the recognized, confirmed, or negated pattern. Another new activity that emerged within the process of scientific modeling is described in category 6b: Mental modeling, as the students in our study did not always document their ideas about the model mechanism in written form, opting to express them verbally instead. For instance, a student verbalizes the assumed correlation between the population dynamics of the Canada goose and its food sources: “I would have thought that if I added these two feed together, I could conclude that by having a lower feed supply, I would also have fewer populations [of Canada geese].” (P. 6, Pos 160). Such verbal expressions were even observed when the students were reminded to create a graphical representation. Table 2 shows the full coding scheme, including observed frequencies. Exemplary statements from the present study for each category of scientific modeling are provided in the Supplementary material.
To answer research question three on how students’ systems thinking and scientific modeling activities interrelate when they examine the dynamic ecological system, we determined co-occurrences of coded segments of both perspectives (see Table 3). This analysis revealed several noticeable interrelations. During system exploration, students demonstrated systems thinking almost exclusively in the area of system behavior and during the interaction with data patterns (modeling activities 5a-c). When students addressed notions referring to the system organization, it was in the context of deducing hypothesis-based graph interactions (modeling activity 4). In the phases of model development, we frequently observed systems thinking. Especially during the broadly observed phases of developing and refining the model, students dealt with the system organization and behavior. In both scientific modeling phases, system exploration and model development, no modeling of the system evolution took place. This changes for the development of predictions, where all three skills of systems thinking were observable. The system modeling, however, is almost entirely tied to the activity of using the model to deduce hypotheses. In addition, activities 6a, referring to the verbalization of analogies and experiences, and 6b, covering the development and usage of mental models, have a special status. During the activation of analogies and experiences (modeling activity 6a), students extensively refer to notions related to the system organization and occasionally deal with concepts regarding the system behavior as well. The mental modeling (modeling activity 6b) was accompanied by systems thinking skills of all kinds. While the system behavior and especially the system organization predominate, modeling system evolution was also observed at least occasionally.
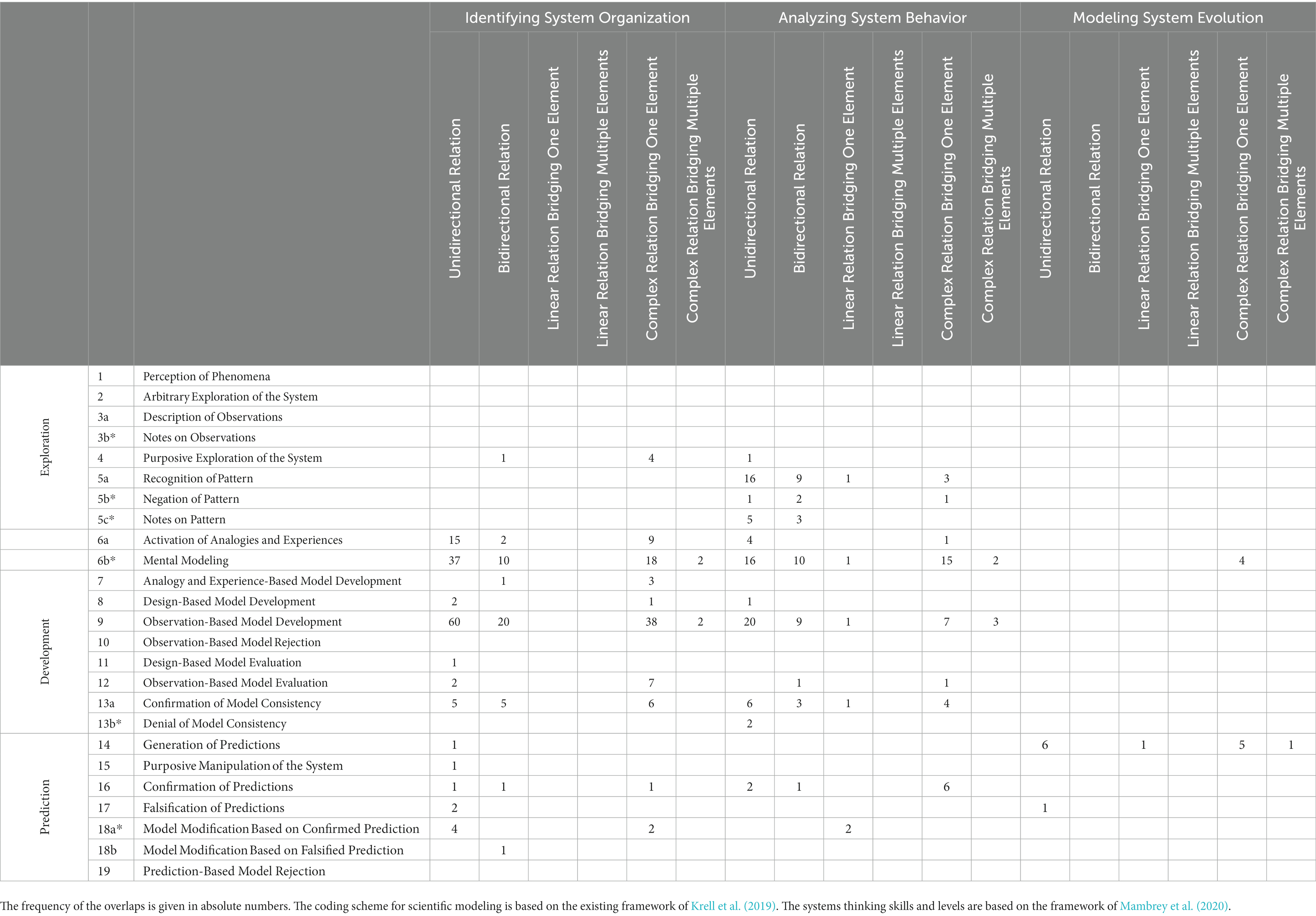
Table 3. Contingency table of the interrelationship between scientific modeling and systems thinking.
5. Discussion
In this study, we confronted students with a dynamic ecological phenomenon in order to examine their systems thinking and scientific modeling as well as the interrelations between these perspectives. The results enable us to provide a detailed account of the students’ comprehension process of a complex and dynamic system.
5.1. Systems thinking
We were able to assess students’ systems thinking using a coding scheme based on the framework of Mambrey et al. (2020). However, when examining the frequency of occurrence, it was observed that activities of identifying the system organization and analyzing the system behavior were much more frequent than modeling the system evolution. This pattern may be attributed to a difference in the cognitive perspective, as identifying the system organization and analyzing the system behavior requires the application of broad, globally applicable skills, whereas modeling the system evolution demands mental application specific to the task at hand. Students must use their gained knowledge about the system to derive predictions about system development and future states (Mehren et al., 2018), which also requires imagination and creative thinking (Mambrey et al., 2022a). Moreover, and due to the openness of the survey, the task may lack the imperative character necessary to evoke prediction generation. In our opinion, it could therefore be highly rewarding to fill this gap by adding tasks in future research that demand prediction generation, even when this means structuring the process through individual instructions or prompts. In order to analyze students’ systems thinking in detail, we also examined the linked structure of relations they took into account. First, and in line with previous results (Strogatz, 2001; Gotwals and Songer, 2010; Hokayem, 2016; Mehren et al., 2018; Mambrey et al., 2022b), we observed that students’ mentions of low linked relationships greatly outweighed the references to high linked relations connecting multiple elements. When examining the specific types of relations, there was a particularly high occurrence of unidirectional, bidirectional, and complex relations bridging one element. Whereas complex relations bridging multiple elements or linear relations were rarely mentioned. We suppose this pattern arises from a moderate linking performance in combination with a sound understanding of isolated predator–prey relationships among the participants (Grotzer and Basca, 2003; Sommer and Lücken, 2010; Mambrey et al., 2022a). Indeed, students often referred to feeding relationships commonly covered in class, such as a predator–prey and competitive relationship, with and without naming the competing food source (NGSS Lead States, 2013). This may even be a good strategy to identify the system organization. However, it is not possible to understand the emergent extinction of the red kite by analyzing individual predator–prey or competitive relationships. Even though the presented phenomenon demands an understanding of emergence, we have not explicitly demanded this and a food web, as typical representation of the trophic relationships within an ecosystem, does not directly reflect this emergent behavior. Therefore, future systems thinking research could focus on systems demonstrating emergent behaviors or systems with an ever-changing system organization in order to evoke more complex systems thinking.
5.2. Scientific modeling
We successfully utilized the scientific modeling framework developed by Krell et al. (2019) to analyze students’ scientific modeling activities in the context of a dynamic ecological system: Our analysis revealed that students were extensively engaged in the exploration of the system, which is consistent with previous research (Göhner and Krell, 2022). Frequently, we observed students taking notes during exploration, particularly when summarizing or describing an observed behavior (modeling activity 3) and recognizing or confirming data patterns (modeling activity 5). Therefore, we inductively added categories in each phase to account for this behavior (Schreier, 2012). We suppose that taking notes may be functional or even necessary in some situations due to the system’s complexity, which includes numerous not directly recognizable relations. Some students probably used this strategy as a cognitive aid to visually represent knowledge about specific relations (Peper and Mayer, 1986). Generally speaking, prior knowledge in the form of analogies and experiences (modeling activity 6a) is frequently observed during scientific modeling (Krell et al., 2019; Meister and Upmeier zu Belzen, 2020). Besides exploring the system or reflecting on prior knowledge (modeling activity 6a), students comprehensively dealt with graphical model development. They frequently incorporated findings from their observations of the system (modeling activity 9) and evaluated the model consistency (modeling activities 11, 12, 13). By extending the coding scheme to differentiate students who found consistency (modeling activity 13a) from those detecting inconsistency between their graphical model and the systems behavior, we noted an interesting pattern: At this point of model development, students almost never detect inconsistency. This could be the case, as they frequently perform mental modeling instead of recording their ideas about the model in a graphic format. Indeed, this process of mental modeling is a key phase of scientific modeling (Clement, 1989; Nersessian, 2008; Gilbert and Justi, 2016) and has been observed in previous modeling studies (Meister and Upmeier zu Belzen, 2020). We added a category to our coding scheme to account for this behavior (modeling activity 6b), though the frequency of occurrence of this activity certainly raises the question of the underlying substructure of mental modeling for future research. To investigate this question, analyzing students’ individual modeling processes will help determine the position and weight of mental modeling within the overall process of scientific modeling (e.g., Göhner and Krell, 2022). In particular, there is evidence that the mental model and the emerging graphical representation of the model may not be fully equivalent (Chandrasekharan and Nersessian, 2015).
Where we found limited evidence, however, was in the phase of predictive model usage. This could be the case because students often do not fully understand the use of models for prediction, as noted by Gogolin and Krüger (2018). In addition, previous research indicates that model application can be challenging (Campbell et al., 2013; Passmore et al., 2014; Krell and Krüger, 2016; Meister and Upmeier zu Belzen, 2020; Göhner and Krell, 2022). The lack of predictive model usage may also be an expression of students’ struggling to generate hypotheses from prior knowledge, their model in progress or previous observations of the system behavior. This would fit the results and the model of Klahr and Dunbar (1988), who assume that exploration without hypothesis is a strategy to search for some kind of pattern within the data and is applied if the generation of hypotheses fails. Surprisingly, when students derived hypotheses, they modified their model not only in response to falsified hypotheses but also in response to confirmed hypotheses (modeling activity 18b). This may be due to the complexity of the system, as new knowledge about individual relationships within the system may require adjustments to the model or allow for model enhancements without necessitating a complete overhaul of the previous understanding. In addition, confirming findings may be suitable not only to the recent hypothesis but also to previous ideas which appear more promising or worthy of investigation (Klahr and Dunbar, 1988).
5.3. Interrelation between scientific modeling and systems thinking
From the combined analysis of systems thinking and scientific modeling, we can identify the following relationships in our study: During the exploration of the ecological system, we rarely observed systems thinking. In the event of pattern recognition, we detected systems thinking almost exclusively with the objective of analyzing the system behavior. Otherwise, systems thinking occurred frequently during model development. We suppose that these findings perfectly illustrate a central difference in the perspectives of systems thinking and scientific modeling. While the former includes the description and explanation of complex phenomena as well as the prediction thereof, the latter additionally involves incidental perception as the early phase of exploration. Another difference in perspective is that systems thinking often describes several skills that relate to specific system properties (Mambrey et al., 2020; Momsen et al., 2022), whereas scientific modeling primarily focuses on the process and activities of modeling the initial natural phenomenon or system. However, identifying the system organization and analyzing the system behavior are mandatory preconditions for successfully developing a model that explains the phenomenon or system (Passmore et al., 2014; Gouvea and Passmore, 2017; Krell et al., 2019). Our results match this assumption, as the students frequently utilize both skills of identifying the system organization and analyzing the system behavior during model development. Another result of the independent coding of systems thinking and scientific modeling was the third skill of systems thinking, modeling the system evolution, being closely linked to the scientific modeling activity of hypothesis generation. This even makes sense, as the former entails statements developing a forecast of one or more population size changes, while the latter covers statements deducing predictions based on an existing model (Clement, 1989; Justi and Gilbert, 2002; Giere et al., 2006; Gilbert and Justi, 2016; Krell et al., 2019; Upmeier zu Belzen et al., 2019). The juxtaposition makes clear that the definition of modeling the system evolution and hypothesis generation in the realm of scientific modeling are closely related. It is therefore not surprising that beyond this, modeling the system evolution as one skill of systems thinking only appeared in conjunction with the phase of mental modeling. This seems even plausible, as the modeling process starts in mental space and can involve cycles of development, evaluation, and modification before any graphic development (Meister and Upmeier zu Belzen, 2020). And indeed, students generally utilize all systems thinking skills in the process of mental modeling. Considering the ratios throughout the mental modeling phase, the participants are more often involved in analyzing the system behavior than in identifying the system organization, while identifying the system organization appears slightly more frequently during the graphical production phase. Perhaps this may be explained by a finding of Mambrey et al. (2022a), who demonstrated that a food web as representation is strongly associated with identifying the system organization but rather no reference for students when analyzing the system behavior or modeling the system evolution. This may explain our observations, as most students drew food webs as models to represent the ecological system. As this type of representation does not provide quantitative information about population growth or decline (Begon and Townsend, 2021), it may, in fact, not evoke thinking about the system behavior. In contrast, the line graph we used in our task focuses on the population dynamics and should thus be a stronger trigger for analyzing the system behavior. Our data clearly support that assumption.
6. Limitations and implications
Our study is limited in some areas that we would like to discuss briefly in the following: to gain a comprehensive understanding of students’ systems thinking and scientific modeling, we provided each participant individual time for analyzing the ecological system simulation. Hence and due to the task complexity, it was not possible to test multiple systems. Therefore, the results may be limited regarding potential context effects (Grotzer and Basca, 2003; Verhoeff et al., 2013; Mambrey et al., 2020). Furthermore, our analysis focused on the general expression of systems thinking and scientific modeling. In consequence, we did not assess students’ content knowledge explicitly, even though modeling activity 7 does provide evidence of the incorporation of analogies and experiences that directly relate to the process of model development. In addition, our coding scheme as well as the underlying framework of Mambrey et al. (2020) do not address systems thinking from a perspective of system properties. As individual system properties, including dynamic changes and susceptibility to disruptive factors like invasive species, play an important role in our simulation, this perspective may be interesting for future research. Furthermore, characteristics of our simulation may also impact students’ thinking. For example, the simulated population dynamics may have drawn students’ attention on thinking about the system behavior in particular. We found that students rarely mentioned emergent effects resulting from complex interrelationships, as well as predictions about future system states or behaviors. One reason for this may be that the task and the simulation do not encourage this explicitly. Hence, future research should emphasize on systems with greater changes in their dynamics and complex emergent effects. Additionally, it would be worthwhile to include tasks that stimulate the prediction of these changes in system development.
Although we did not analyze the students’ individual procedures yet, we wish to discuss possible implications for engaging students in systems thinking and scientific modeling. Our results imply that a task involving students in the exploration of a dynamic system to deduce the system structure seems suitable to evoke systems thinking and modeling insofar as the students develop models. However, the generation of predictions and, consequently, the modeling of the system evolution as well fell short of our expectations. We suppose that the regular oscillatory nature in population dynamics is too foreseeable, even if the introduction of the invasive Canada goose was a functional disturbance. In this regard, it may be beneficial to utilize even less predictable system dynamics, for example, by modeling alternative stable states or multiple regime shifts in ecosystems (Scheffer and Carpenter, 2003), to focus on the cyclic nature of modeling through predicting outcomes and modifying the model. However, it remains open whether all students used the same approach or if they showed distinctive styles of scientific modeling and systems thinking (e.g., Meister and Upmeier zu Belzen, 2020; Göhner and Krell, 2022). In order to answer this research question, it might be beneficial to analyze the individual chronological sequences of students’ proceedings. Additional insights could be revealed by analyzing students’ drawings, as individual students also created representations other than food webs. In this context, we are especially interested in whether different types of representations correlate with distinct patterns of systems thinking and how students account for the system’s dynamic behavior in their representations.
Data availability statement
The raw data supporting the conclusions of this article will be made available by the authors, without undue reservation.
Ethics statement
Ethical review and approval was not required for the study on human participants in accordance with the local legislation and institutional requirements. Written informed consent to participate in this study was provided by the participants’ legal guardian/next of kin.
Author contributions
AL, JT, and PS: conception and design of the study and manuscript revision. AL and JT: development of the test instrument and manuscript writing. AL: data collection and data analysis. PS: supervision. All authors contributed to the article and approved the submitted version.
Funding
This project is part of the “Qualitätsoffensive Lehrerbildung,” a joint initiative of the Federal Government and the Länder which aims to improve the quality of teacher training. The program is funded by the German Federal Ministry of Education and Research (BMBF) under Grant Number 01JA1910. The Interdisciplinary Center for Educational Research (IZfB) of the University of Duisburg-Essen provided funding support for linguistic editing services. We perceived support from the Open Access Publication Fund of the University of Duisburg-Essen.
Acknowledgments
We would like to express our gratitude to all students, who participated in this study. Additionally, we extend our appreciation to our student research assistant, Annika Stratmann, for her invaluable contributions in transcription and co-coding of the think-aloud protocols.
Conflict of interest
The authors declare that the research was conducted in the absence of any commercial or financial relationships that could be construed as a potential conflict of interest.
Publisher’s note
All claims expressed in this article are solely those of the authors and do not necessarily represent those of their affiliated organizations, or those of the publisher, the editors and the reviewers. Any product that may be evaluated in this article, or claim that may be made by its manufacturer, is not guaranteed or endorsed by the publisher.
Author disclaimer
The opinions and statements presented in this article are the responsibility of the authors alone and may not reflect the views of their affiliated institutions, the funding agencies, the publisher, editors or reviewers.
Supplementary material
The Supplementary material for this article can be found online at: https://www.frontiersin.org/articles/10.3389/feduc.2023.1187237/full#supplementary-material
References
Andrade, B. O., Koch, C., Boldrini, I. I., Vélez-Martin, E., Hasenack, H., Hermann, J.-M., et al. (2015). Grassland degradation and restoration: a conceptual framework of stages and thresholds illustrated by southern Brazilian grasslands. Nat. Conserv. 13, 95–104. doi: 10.1016/j.ncon.2015.08.002
Ben Zvi Assaraf, O., and Knippels, M.-C. P. J., eds. (2022). Fostering understanding of complex systems in biology education: pedagogies, guidelines and insights from classroom-based research. Cham: Springer.
Ben Zvi Assaraf, O., and Orion, N. (2005). Development of system thinking skills in the context of earth system education. J. Res. Sci. Teach. 42, 518–560. doi: 10.1002/tea.20061
Ben Zvi Assaraf, O., and Orion, N. (2010). System thinking skills at the elementary school level. J. Res. Sci. Teach. 47, 540–563. doi: 10.1002/tea.20351
Bielik, T., Delen, I., Krell, M., and Assaraf, B. Z. (2023). Characterising the literature on the teaching and learning of system thinking and complexity in STEM education: A bibliometric analysis and research synthesis. J. STEM Educ. Res. doi: 10.1007/s41979-023-00087-9
Bielik, T., Fonio, E., Feinerman, O., Duncan, R. G., and Levy, S. T. (2021). Working together: integrating computational modeling approaches to investigate complex phenomena. J. Sci. Educ. Technol. 30, 40–57. doi: 10.1007/s10956-020-09869-x
Bielik, T., Stephens, L., McIntyre, C., Damelin, D., and Krajcik, J. S. (2022). Supporting student system modelling practice through curriculum and technology design. J. Sci. Educ. Technol. 31, 217–231. doi: 10.1007/s10956-021-09943-y
Boersma, K. T., Waarlo, A. J., and Klaassen, K. (2011). The feasibility of systems thinking in biology education. J. Biol. Educ. 45, 190–197. doi: 10.1080/00219266.2011.627139
Bowers, J., Damelin, D., Eidin, E., and McIntyre, C. (2022). Keeping cool with SageModeler: engaging students in systems thinking and computational thinking through modeling. Sci. Teach. 89, 18–25.
Brandstädter, K., Harms, U., and Großschedl, J. (2012). Assessing system thinking through different concept-mapping practices. Int. J. Sci. Educ. 34, 2147–2170. doi: 10.1080/09500693.2012.716549
Brennan, R. L., and Prediger, D. J. (1981). Coefficient kappa: some uses, misuses, and alternatives. Educ. Psychol. Meas. 41, 687–699. doi: 10.1177/001316448104100307
Campbell, T., Oh, P. S., and Neilson, D. (2013). “Reification of five types of modeling pedagogies with model-based inquiry (MBI) modules for high school science classrooms” in Approaches and strategies in next generation science learning. eds. M. S. Khine and I. M. Saleh (Hershey, PA: IGI Global), 106–126.
Chandrasekharan, S., and Nersessian, N. J. (2015). Building cognition: the construction of computational representations for scientific discovery. Cogn. Sci. 39, 1727–1763. doi: 10.1111/cogs.12203
Chang, W., Cheng, J., Allaire, J. J., Sievert, C., Schloerke, B., Xie, Y., et al. (2022). Shiny: Web application framework for R [computer program].
Ciesielska, M., Boström, K. W., and Öhlander, M. (2018). “Observation methods” in Qualitative methodologies in organization studies: Volume II: Methods and possibilities. eds. M. Ciesielska and D. Jemielniak (Cham: Palgrave Macmillan), 33–52. doi: 10.1007/978-3-319-65217-7
Clement, J. (1989). “Learning via model construction and criticism” in Handbook of creativity. eds. J. A. Glover, R. R. Ronning, and C. R. Reynolds (New York, NY: Springer), 341–381.
Damelin, D., Krajcik, J. S., McIntyre, C., and Bielik, T. (2017). Students making system models: an accessible approach. Sci. Scope 40, 78–82. doi: 10.2505/4/ss17_040_05_78
Dor-Haim, S., and Assaraf, B. Z. (2022). “Long term ecological research as a learning environment: evaluating its impact in developing the understanding of ecological systems thinking – A case study” in Fostering understanding of complex systems in biology education: pedagogies, guidelines and insights from classroom-based research. eds. O. B. Z. Assaraf and M.-C. P. J. Knippels (Cham, Switzerland: Springer), 17–40.
Early, R., Bradley, B. A., Dukes, J. S., Lawler, J. J., Olden, J. D., Blumenthal, D. M., et al. (2016). Global threats from invasive alien species in the twenty-first century and national response capacities. Nat. Commun. 7:12485. doi: 10.1038/ncomms12485
Eilam, B. (2012). System thinking and feeding relations: learning with a live ecosystem model. Instr. Sci. 40, 213–239. doi: 10.1007/s11251-011-9175-4
Eilam, B., and Omar, S. Y. (2022). “Science teachers’ construction of knowledge about simulations and population size via performing inquiry with simulations of growing vs. descending levels of complexity” in Fostering understanding of complex systems in biology education: pedagogies, guidelines and insights from classroom-based research. eds. O. B. Z. Assaraf and M.-C. P. J. Knippels (Cham: Springer), 205–226. doi: 10.1007/978-3-030-98144-0_10
Eliam, B., and Reisfeld, D. (2017). A curriculum unit for promoting complex system thinking: the case of combined system dynamics and agent based models for population growth. J. Adv. Educ. Res. 2, 39–60. doi: 10.22606/jaer.2017.22001
Ericsson, K. A., and Simon, H. A. (1993). Protocol analysis: Verbal reports as data. Cambridge, MA: MIT Press.
Evagorou, M., Korfiatis, K., Nicolaou, C., and Constantinou, C. (2009). An investigation of the potential of interactive simulations for developing system thinking skills in elementary school: a case study with fifth-graders and sixth-graders. Int. J. Sci. Educ. 31, 655–674. doi: 10.1080/09500690701749313
Giere, R. N., Bickle, J., and Mauldin, R. F. (2006). Understanding scientific reasoning. Belmont, CA: Wadsworth.
Gilbert, J. K., and Justi, R. (2016). Modelling-based teaching in science education. Cham: Springer.
Gilissen, M. G. R., Knippels, M.-C. P. J., Verhoeff, R. P., and van Joolingen, W. R. (2020). Teachers’ and educators’ perspectives on systems thinking and its implementation in Dutch biology education. J. Biol. Educ. 54, 485–496. doi: 10.1080/00219266.2019.1609564
Gogolin, S., and Krüger, D. (2018). Students’ understanding of the nature and purpose of models. J. Res. Sci. Teach. 55, 1313–1338. doi: 10.1002/tea.21453
Göhner, M., and Krell, M. (2022). Preservice science teachers’ strategies in scientific reasoning: the case of modeling. Res. Sci. Educ. 52, 395–414. doi: 10.1007/s11165-020-09945-7
Gotwals, A. W., and Songer, N. B. (2010). Reasoning up and down a food chain: using an assessment framework to investigate students’ middle knowledge. Sci. Educ. 94, 259–281. doi: 10.1002/sce.20368
Gouvea, J., and Passmore, C. (2017). ‘Models of’ versus ‘models for’: toward an agent-based conception of modeling in the science classroom. Sci. Educ. (Dordr.) 26, 49–63. doi: 10.1007/s11191-017-9884-4
Grotzer, T. A., and Basca, B. B. (2003). How does grasping the underlying causal structures of ecosystems impact students’ understanding? J. Biol. Educ. 38, 16–29. doi: 10.1080/00219266.2003.9655891
Grotzer, T. A., Powell, M. M., Derbiszewska, K. M., Courter, C. J., Kamarainen, A. M., Metcalf, S. J., et al. (2015). Turning transfer inside out: the affordances of virtual worlds and mobile devices in real world contexts for teaching about causality across time and distance in ecosystems. Technol. Knowl. Learn. 20, 43–69. doi: 10.1007/s10758-014-9241-5
Hmelo-Silver, C. E., Jordan, R. C., Eberbach, C., and Sinha, S. (2017). Systems learning with a conceptual representation: A quasi-experimental study. Instr. Sci. 45, 53–72. doi: 10.1007/s11251-016-9392-y
Hmelo-Silver, C. E., Liu, L., Gray, S., and Jordan, R. C. (2015). Using representational tools to learn about complex systems: A tale of two classrooms. J. Res. Sci. Teach. 52, 6–35. doi: 10.1002/tea.21187
Hmelo-Silver, C. E., Marathe, S., and Liu, L. (2007). Fish swim, rocks sit, and lungs breathe: expert-novice understanding of complex systems. J. Learn. Sci. 16, 307–331. doi: 10.1080/10508400701413401
Hogan, K. (2000). Assessing students’ systems reasoning in ecology. J. Biol. Educ. 35, 22–28. doi: 10.1080/00219266.2000.9655731
Hokayem, H. (2016). Patterns of reasoning about ecological systemic reasoning for early elementary students. Sci. Educ. Int. 27, 117–135.
Hokayem, H., and Gotwals, A. W. (2016). Early elementary students’ understanding of complex ecosystems: A learning progression approach. J. Res. Sci. Teach. 53, 1524–1545. doi: 10.1002/tea.21336
IPBES. (2019). Global assessment report on biodiversity and ecosystem services of the intergovernmental science-policy platform on biodiversity and ecosystem services. Bonn: IPBES.
Jackson, M. C., Mansell, G. J., Flood, R. L., Blackham, R. B., and Probert, S. V. E., Eds. (1991). Systems thinking in Europe. New York, NY: Springer.
Jin, H., Shin, H. J., Hokayem, H., Qureshi, F., and Jenkins, T. (2019). Secondary students’ understanding of ecosystems: A learning progression approach. Int. J. Sci. Math. Educ. 17, 217–235. doi: 10.1007/s10763-017-9864-9
Jordan, R. C., Hmelo-Silver, C. E., Liu, L., and Gray, S. A. (2013). Fostering reasoning about complex systems: using the aquarium to teach systems thinking. Appl. Environ. Educ. Commun. 12, 55–64. doi: 10.1080/1533015X.2013.797860
Justi, R., and Gilbert, J. K. (2002). Modelling, teachers’ views on the nature of modelling, and implications for the education of modellers. Int. J. Sci. Educ. 24, 369–387. doi: 10.1080/09500690110110142
Kiesewetter, A., and Schmiemann, P. (2022). Understanding homeostatic regulation: the role of relationships and conditions in feedback loop reasoning. CBE Life Sci. Educ. 21:ar56. doi: 10.1187/cbe.21-04-0092
Klahr, D., and Dunbar, K. (1988). Dual space search during scientific reasoning. Cogn. Sci. 12, 1–48. doi: 10.1207/s15516709cog1201_1
Knuuttila, T., and Boon, M. (2011). How do models give us knowledge? The case of Carnot’s ideal heat engine. Eur. J. Philos. Sci. 1, 309–334. doi: 10.1007/s13194-011-0029-3
Krell, M., and Krüger, D. (2016). Testing models: A key aspect to promote teaching activities related to models and modelling in biology lessons? J. Biol. Educ. 50, 160–173. doi: 10.1080/00219266.2015.1028570
Krell, M., Walzer, C., Hergert, S., and Krüger, D. (2019). Development and application of a category system to describe pre-service science teachers’ activities in the process of scientific modelling. Res. Sci. Educ. 49, 1319–1345. doi: 10.1007/s11165-017-9657-8
Kuckartz, U., and Rädiker, S. (2019). Analyzing qualitative data with MAXQDA: Text, audio, and video. Cham: Springer.
Kultusministerkonferenz. (2004). Einheitliche Prüfungsanforderungen in der Abiturprüfung Biologie [common examination requirements in the final exam in the abitur, the highest secondary school diploma, in biology]. Available at: (https://www.kmk.org/fileadmin/veroeffentlichungen_beschluesse/1989/1989_12_01-EPA-Biologie.pdf)
Kultusministerkonferenz. (2005). Bildungsstandards im Fach Biologie für den Mittleren Schulabschluss [educational standards in biology for the secondary school diploma]. München: Luchterhand.
Ladrera, R., Robredo, B., Ortega-Lasuen, U., Díez, J. R., and Ruiz-González, A. (2020). Unprepared to deal with invasion: pre-service teachers’ perception, knowledge and attitudes toward invasive species. Sustainability 12:10543. doi: 10.3390/su122410543
Landis, J. R., and Koch, G. G. (1977). The measurement of observer agreement for categorical data. Biometrics 33, 159–174. doi: 10.2307/2529310
Lee, T. D., Gail Jones, M., and Chesnutt, K. (2019). Teaching systems thinking in the context of the water cycle. Res. Sci. Educ. 49, 137–172. doi: 10.1007/s11165-017-9613-7
Liu, L., and Hmelo-Silver, C. E. (2009). Promoting complex systems learning through the use of conceptual representations in hypermedia. J. Res. Sci. Teach. 46, 1023–1040. doi: 10.1002/tea.20297
Mambrey, S., Schreiber, N., and Schmiemann, P. (2022a). Young students’ reasoning about ecosystems: the role of systems thinking, knowledge, conceptions, and representation. Res. Sci. Educ. 52, 79–98. doi: 10.1007/s11165-020-09917-x
Mambrey, S., Timm, J., Landskron, J. J., and Schmiemann, P. (2020). The impact of system specifics on systems thinking. J. Res. Sci. Teach. 57, 1632–1651. doi: 10.1002/tea.21649
Mambrey, S., Wellmanns, A., Timm, J., and Schmiemann, P. (2022b). “Systems thinking in ecological and physiological systems and the role of representations” in Fostering understanding of complex systems in biology education: Pedagogies, guidelines and insights from classroom-based research. eds. O. B. Z. Assaraf and M.-C. P. J. Knippels (Cham: Springer), 105–121.
Mayring, P. (2014). Qualitative content analysis: theoretical foundation, basic procedures and software solution. Klagenfurt.
McKinney, M. L., and Lockwood, J. L. (1999). Biotic homogenization: a few winners replacing many losers in the next mass extinction. Trends Ecol. Evol. 14, 450–453. doi: 10.1016/s0169-5347(99)01679-1
Mehren, R., Rempfler, A., Buchholz, J., Hartig, J., and Ulrich-Riedhammer, E. M. (2018). System competence modelling: theoretical foundation and empirical validation of a model involving natural, social and human-environment systems. J. Res. Sci. Teach. 55, 685–711. doi: 10.1002/tea.21436
Meister, J., and Upmeier zu Belzen, A. (2020). Investigating students’ modelling styles in the process of scientific-mathematical modelling. Sci. Educ. Rev. Lett. 2019, 8–14. doi: 10.18452/21039
Métrailler, Y. A., Reijnen, E., Kneser, C., and Opwis, K. (2008). Scientific problem solving in a virtual laboratory: A comparison between individuals and pairs. Swiss J. Psychol. 67, 71–83. doi: 10.1024/1421-0185.67.2.71
Microsoft Corporation (2020). Microsoft whiteboard [computer program]: Version 22.11028.0.9070. Redmond, WA: Microsoft Corporation.
Momsen, J., Speth, E. B., Wyse, S., and Long, T. (2022). Using systems and systems thinking to unify biology education. CBE: life. Sci. Educ. 21:es3. doi: 10.1187/cbe.21-05-0118
National Research Council. (2012). A framework for K-12 science education: Practices, crosscutting concepts, and core ideas. Washington, DC: National Academies Press.
NGSS Lead States. (2013). Next generation science standards: For states by states. Washington, DC: National Academies Press.
Oh, P. S., and Oh, S. J. (2011). What teachers of science need to know about models: an overview. Int. J. Sci. Educ. 33, 1109–1130. doi: 10.1080/09500693.2010.502191
Oh, S., and Wildemuth, B. M. (2016). “Think-aloud protocols” in Applications of social research methods to questions in information and library science. ed. B. M. Wildemuth (Santa Barbara, CA: Libraries Unlimited), 198–208.
Passmore, C., Gouvea, J. S., and Giere, R. (2014). “Models in science and in learning science: focusing scientific practice on sense-making” in International handbook of research in history, philosophy and science teaching. ed. M. R. Matthews (Dordrecht: Springer), 1171–1202.
Peper, R. J., and Mayer, R. E. (1986). Generative effects of note-taking during science lectures. J. Educ. Psychol. 78, 34–38. doi: 10.1037/0022-0663.78.1.34
R Core Team. (2022). R: A language and environment for statistical computing [computer program]. Vienna: R Foundation for Statistical Computing.
Rachmatullah, A., and Wiebe, E. N. (2022). Building a computational model of food webs: impacts on middle school students’ computational and systems thinking skills. J. Res. Sci. Teach. 59, 585–618. doi: 10.1002/tea.21738
Rellensmann, J., Schukajlow, S., and Leopold, C. (2017). Make a drawing: effects of strategic knowledge, drawing accuracy, and type of drawing on students’ mathematical modelling performance. Educ. Stud. Math. 95, 53–78. doi: 10.1007/s10649-016-9736-1
Riess, W., and Mischo, C. (2010). Promoting systems thinking through biology lessons. Int. J. Sci. Educ. 32, 705–725. doi: 10.1080/09500690902769946
Sauvé, L., Renaud, L., Kaufmann, D., and Marquis, J.-S. (2007). Distinguishing between games and simulations: a systematic review. Educ. Technol. Soc. 10, 247–256.
Scheffer, M., and Carpenter, S. R. (2003). Catastrophic regime shifts in ecosystems: linking theory to observation. Trends Ecol. Evol. 18, 648–656. doi: 10.1016/j.tree.2003.09.002
Schwarz, C. V., Ke, L., Salgado, M., and Manz, E. (2022). Beyond assessing knowledge about models and modeling: moving toward expansive, meaningful, and equitable modeling practice. J. Res. Sci. Teach. 59, 1086–1096. doi: 10.1002/tea.21770
Schwarz, C. V., and White, B. Y. (2005). Metamodeling knowledge: developing students’ understanding of scientific modeling. Cogn. Instr. 23, 165–205. doi: 10.1207/s1532690xci2302_1
Snapir, Z., Eberbach, C., Ben Zvi Assaraf, O., Hmelo-Silver, C. E., and Tripto, J. (2017). Characterising the development of the understanding of human body systems in high-school biology students: a longitudinal study. Int. J. Sci. Educ. 39, 2092–2127. doi: 10.1080/09500693.2017.1364445
Soetaert, K., Petzoldt, T., and Setzer, R. W. (2010). Solving differential equations in R: package deSolve. J. Stat. Soft. 33, 1–25. doi: 10.18637/jss.v033.i09
Sommer, C., and Lücken, M. (2010). System competence: are elementary students able to deal with a biological system? Nord. Stud. Sci. Educ. 6, 125–143. doi: 10.5617/nordina.255
Streiling, S., Hörsch, C., and Rieß, W. (2021). Effects of teacher training in systems thinking on biology students: an intervention study. Sustainability 13:7631. doi: 10.3390/su13147631
Torkar, G., and Korfiatis, K. (2022). “Pre-service teachers’ conceptual schemata and system reasoning about the carbon cycle and climate change: an exploratory study of a learning framework for understanding complex systems” in Fostering understanding of complex systems in biology education: Pedagogies, guidelines and insights from classroom-based research. eds. O. B. Z. Assaraf and M.-C. P. J. Knippels (Cham: Springer), 151–169.
Tripto, J., Ben Zvi Assaraf, O., and Amit, M. (2013). Mapping what they know: concept maps as an effective tool for assessing students’ systems thinking. Am. J. Oper. Res. 3, 245–258. doi: 10.4236/ajor.2013.31A022
Tripto, J., Ben Zvi Assaraf, O., and Amit, M. (2018). Recurring patterns in the development of high school biology students’ system thinking over time. Instr. Sci. 46, 639–680. doi: 10.1007/s11251-018-9447-3
Upmeier Zu Belzen, A., Van Driel, J., and Krüger, D. (2019). Introducing a framework for modeling competence, In Towards a competence-based view on models and modeling in science education, Ed. Belzen, A. Upmeier Zu, Krüger, D., and Van Driel, J. (Cham: Springer), 3–19.
Verhoeff, R. P., Boersma, K. T., and Waarlo, A. J. (2013). “Multiple representations in modeling strategies for the development of systems thinking in biology education” in Multiple representations in biological education. eds. D. F. Treagust and C.-Y. Tsui (Dordrecht: Springer), 331–348.
Verhoeff, R. P., Knippels, M.-C. P. J., Gilissen, M. G. R., and Boersma, K. T. (2018). The theoretical nature of systems thinking: perspectives on systems thinking in biology education. Front. Educ. 3:40. doi: 10.3389/feduc.2018.00040
Verhoeff, R. P., Waarlo, A. J., and Boersma, K. T. (2008). Systems modelling and the development of coherent understanding of cell biology. Int. J. Sci. Educ. 30, 543–568. doi: 10.1080/09500690701237780
Wellmanns, A., and Schmiemann, P. (2022). Feedback loop reasoning in physiological contexts. J. Biol. Educ. 56, 465–485. doi: 10.1080/00219266.2020.1858929
Wiebe, E., London, J., Aksit, O., Mott, B. W., Boyer, K. E., and Lester, J. C. (2019). Development of a lean computational thinking abilities assessment for middle grades students, In Proceedings of the 50th ACM technical symposium on computer science education, Eds. E. K. Hawthorne, M. A. Pérez-Quiñones, S. Heckman, and J. Zhang (New York, NY: ACM), 456–461.
Wilson, K. J., Long, T. M., Momsen, J. L., and Bray Speth, E. (2020). Modeling in the classroom: making relationships and systems visible. CBE: life. Sci. Educ. 19:fe1. doi: 10.1187/cbe.19-11-0255
Yoon, S. A., Anderson, E., Koehler-Yom, J., Evans, C., Park, M., Sheldon, J., et al. (2017). Teaching about complex systems is no simple matter: building effective professional development for computer-supported complex systems instruction. Instr. Sci. 45, 99–121. doi: 10.1007/s11251-016-9388-7
Yoon, S. A., Goh, S.-E., and Park, M. (2018). Teaching and learning about complex systems in K–12 science education: A review of empirical studies 1995-2015. Rev. Educ. Res. 88, 285–325. doi: 10.3102/0034654317746090
Zhang, L. J., and Zhang, D. (2019). “Think-aloud protocols” in The Routledge handbook of research methods in applied linguistics. eds. J. McKinley and H. Rose (London: Routledge), 302–311.
Keywords: complex phenomena, systems thinking, scientific modeling, dynamic ecological system, alien species, science education, secondary students, qualitative content analysis
Citation: Lankers A, Timm J and Schmiemann P (2023) Students’ systems thinking while modeling a dynamic ecological system. Front. Educ. 8:1187237. doi: 10.3389/feduc.2023.1187237
Edited by:
Moritz Krell, University of Kiel, GermanyReviewed by:
Elon Langbeheim, Ben-Gurion University of the Negev, IsraelAnna Beniermann, Humboldt University of Berlin, Germany
Copyright © 2023 Lankers, Timm and Schmiemann. This is an open-access article distributed under the terms of the Creative Commons Attribution License (CC BY). The use, distribution or reproduction in other forums is permitted, provided the original author(s) and the copyright owner(s) are credited and that the original publication in this journal is cited, in accordance with accepted academic practice. No use, distribution or reproduction is permitted which does not comply with these terms.
*Correspondence: Annika Lankers, YW5uaWthLmxhbmtlcnNAdW5pLWR1ZS5kZQ==