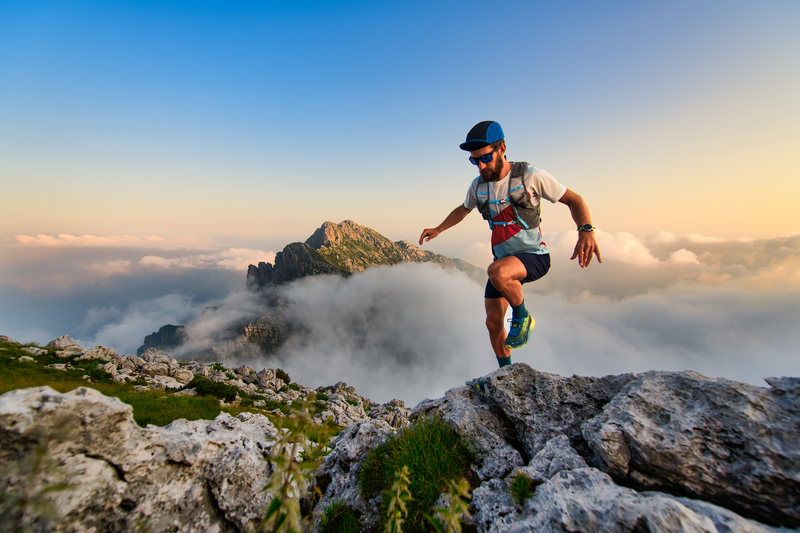
95% of researchers rate our articles as excellent or good
Learn more about the work of our research integrity team to safeguard the quality of each article we publish.
Find out more
EDITORIAL article
Front. Educ. , 25 April 2023
Sec. Educational Psychology
Volume 8 - 2023 | https://doi.org/10.3389/feduc.2023.1186326
This article is part of the Research Topic Progress Monitoring and Data-Based Decision-Making in Inclusive Schools View all 14 articles
Editorial on the Research Topic
Progress monitoring and data-based decision-making in inclusive schools
Despite extensive research and positive practices related to inclusive education, some students still struggle with academic skills. Progress monitoring (PM) is a valuable approach that can provide explicit feedback to teachers in schools about how students respond to instruction. The fundamental idea behind PM is to document the learning development of students and use the data to inform instructional decisions about interventions over time, using repeated, brief, and reliable standardized tests. PM is a formative diagnostic that enables the measurement and evaluation of learning development at multiple points in time, providing feedback to teachers and learners. Unlike summative assessment, which evaluates learning outcomes, PM aims to measure for the purpose of supporting learning.
Without PM, students with exceptional needs may be evaluated based on their performance relative to their classmates, rather than their own individual progress. Research indicates that when teachers use PM, positive effects on student outcomes can be seen. However, the use of PM is not widespread, which may be due to teachers having additional work or a lack of knowledge on how to use PM in the context of data-based decision making. Additionally, tests or online platforms for PM are not available in many countries and languages.
To effectively measure learning progress, PM measures must provide both the psychometric quality criteria for status tests and the quality to measure learning progress. Classical test theory is no longer sufficient for this purpose since learning trajectories differ among students. PM measures must be uniform over time, both for an individual student and for specific groups of students (measurement invariance), and PM must be sensitive to learning trajectories (i.e., sensitive to change, even for weak learners). Moreover, PM measures must be brief and easy to use so they can be used frequently in everyday teaching. Therefore, it is crucial that PM measures are practical, useful, and economical. This is because PM can only be effective when teachers reflect on their instructional decisions based on the new information provided by the PM data. Compared to status tests, the requirements of PM are much higher, both psychometrically and in terms of practical implementation. Therefore, PM should be supported by adapted materials and recommendations to aid teachers and students.
In the field of education, a range of studies have been conducted to improve the reliability and effectiveness of various methods used for assessment, monitoring and evaluation of student progress. The following studies are a few examples of such efforts.
Wilbert et al. conducted a study to analyze the statistical power of piecewise regression analyses in single-case experimental studies. Their research demonstrated that this method can be a useful tool for planning and assessing single case studies, which are crucial for reviewing evidence-based practice.
Forthmann et al. conducted a simulation study to assess the reliability of measures used for monitoring student progress. They found that reliability estimation works well across a variety of simulation conditions, but it can be biased under certain circumstances, such as when data quality is very poor or empirical reliability is estimated.
Ketterlin-Geller et al. described an approach to adapting Automated Item Generation (AIG) principles to develop parallel progress monitoring measures.
Schurig et al. presented a study on continuous norming in learning progress monitoring for a spelling test. Their data was obtained through a longitudinal study of students in grades 2 to 4.
Anderson et al. conducted a longitudinal study on mental computation over a period of 34 weeks with data collected for 12 measurement intervals. Their research was affected by the COVID-19 pandemic.
Israelsen-Augenstein et al. developed a new measure, the Monitoring Indicators of Scholarly Language (MISL), which was shown to be a valid measure of narrative production abilities.
Winkes and Schaller developed a written expression curriculum-based measurement (CBM-W) suitable as a universal screening tool but not for progress monitoring of individual students.
Leidig et al. conducted a study on the impact of the Good Behavior Game (GBG) on at-risk students' academic engagement and disruptive behavior. They used behavioral progress monitoring with a multiple baseline design in a German inclusive primary school sample.
Merlo et al. introduced a tool called BEHAVE to monitor inclusive interventions and presented two case studies involving kindergarten children with neurodevelopmental disorders.
Stecker and Foegen developed an online system to support algebra progress monitoring and determined that it improved teachers' scoring in algebra measures based on online instruction.
Jungjohann et al. developed a video intervention for linear trend identification using Tukey Tri-Split and demonstrated that the video instruction is more effective than text-based hints.
Hase et al. conducted an online survey study on the usage of learning data from Digital Learning Platforms (DLP).
Van den Bosch et al. examined teachers' visual inspection of Curriculum-Based Measurement (CBM) progress graphs using eye-tracking technology. Their study revealed variability in teachers' patterns of graph inspection, which was linked to their abilities to describe the graphs.
MG set up a draft and all authors improved it. All authors participated equally in writing the editorial. All authors contributed to the article and approved the submitted version.
The authors declare that the research was conducted in the absence of any commercial or financial relationships that could be construed as a potential conflict of interest.
All claims expressed in this article are solely those of the authors and do not necessarily represent those of their affiliated organizations, or those of the publisher, the editors and the reviewers. Any product that may be evaluated in this article, or claim that may be made by its manufacturer, is not guaranteed or endorsed by the publisher.
Keywords: CBM, progress monitoring (PM) measures, learning growth, teacher feedback, progress graph, single case analysis, competence and performance
Citation: Gebhardt M, Blumenthal S, Scheer D, Blumenthal Y, Powell S and Lembke E (2023) Editorial: Progress monitoring and data-based decision-making in inclusive schools. Front. Educ. 8:1186326. doi: 10.3389/feduc.2023.1186326
Received: 14 March 2023; Accepted: 13 April 2023;
Published: 25 April 2023.
Edited and reviewed by: Douglas F. Kauffman, Medical University of the Americas–Nevis, United States
Copyright © 2023 Gebhardt, Blumenthal, Scheer, Blumenthal, Powell and Lembke. This is an open-access article distributed under the terms of the Creative Commons Attribution License (CC BY). The use, distribution or reproduction in other forums is permitted, provided the original author(s) and the copyright owner(s) are credited and that the original publication in this journal is cited, in accordance with accepted academic practice. No use, distribution or reproduction is permitted which does not comply with these terms.
*Correspondence: Markus Gebhardt, bWFya3VzLmdlYmhhcmR0QHVyLmRl
Disclaimer: All claims expressed in this article are solely those of the authors and do not necessarily represent those of their affiliated organizations, or those of the publisher, the editors and the reviewers. Any product that may be evaluated in this article or claim that may be made by its manufacturer is not guaranteed or endorsed by the publisher.
Research integrity at Frontiers
Learn more about the work of our research integrity team to safeguard the quality of each article we publish.