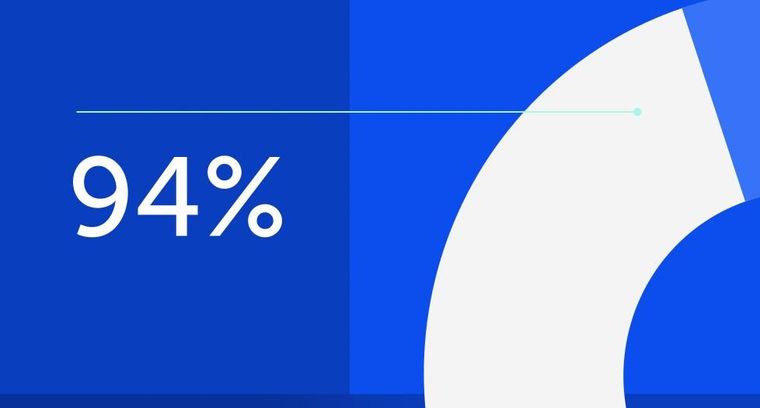
94% of researchers rate our articles as excellent or good
Learn more about the work of our research integrity team to safeguard the quality of each article we publish.
Find out more
ORIGINAL RESEARCH article
Front. Educ., 06 October 2023
Sec. STEM Education
Volume 8 - 2023 | https://doi.org/10.3389/feduc.2023.1170967
This article is part of the Research TopicInvestigating Complex Phenomena: Bridging between Systems Thinking and Modeling in Science EducationView all 13 articles
Introduction: Abductive reasoning is a type of reasoning that is applied to generate causal explanations. Modeling for inquiry is an important practice in science and science education that involves constructing models as causal explanations for scientific phenomena. Thus, abductive reasoning is applied in modeling for inquiry. Biological phenomena are often best explained as complex systems, which means that their explanations ideally include causes and mechanisms on different organizational levels. In this study, we investigate the role of abductive reasoning in modeling for inquiry and its potential for explaining biological phenomena as complex systems.
Methods: Eighteen pre-service science teachers were randomly assigned to model one of two biological phenomena: either a person's reddened face, for which participants knew of explanations from their everyday lives, or a clownfish changing its sex, for which participants did not know about explanations. Using the think-aloud method, we examined the presence of abductive reasoning in participants' modeling processes. We also analyzed modeling processes in terms of participants' ability to model the phenomena as complex systems.
Results: All participants reasoned abductively when solving the modeling task. However, modeling processes differed depending on the phenomenon. For the reddened face, participants generated simple models that they were confident with. In contrast, for the clownfish, participants generated more complex models that they were insecure about. Extensive engagement in abductive reasoning alone did not lead to the generation of models that explained the phenomena as complex systems.
Discussion: Based on the findings, we conclude that engagement in abductive reasoning will not suffice to explain phenomena as complex systems. We suggest examining in future studies how abductive reasoning is combined with systems thinking skills to explain phenomena as complex systems in biological model construction.
Modeling is a key practice in science (Koponen, 2007; Lehrer and Schauble, 2015; Frigg and Hartmann, 2020) and, thus, a central practice in standards for science education (OECD, 2008; NGSS Lead States, 2013; KMK, 2020). In science and science education, modeling has two functions. One is representational modeling, where a model is constructed as a focused representation of the phenomenon and is applied as a medium for communicating about the phenomenon (Oh and Oh, 2011; Gouvea and Passmore, 2017; Upmeier zu Belzen et al., 2021). The other function is modeling for inquiry, where a model is constructed as a possible explanation for the phenomenon, and it is applied as a research tool for deriving hypotheses and conducting investigations to test them (Oh and Oh, 2011; Gouvea and Passmore, 2017; Upmeier zu Belzen et al., 2021). Both functions of modeling deal with the explanation of phenomena, but they refer to different meanings of explaining (Rocksén, 2016; Ke and Schwarz, 2019; Upmeier zu Belzen et al., 2021): while representational modeling is about explaining to make something clear about a well-studied phenomenon, modeling for inquiry is about explaining to justify something about a so-far-explained phenomenon. In both explanatory senses, and thus in both functions of modeling, biological phenomena are often best explained as complex systems (Hmelo-Silver et al., 2017; Snapir et al., 2017). A phenomenon is explained as a complex system if its explanation includes causes and mechanisms on different organizational levels (Schneeweiß and Gropengießer, 2019, 2022; Ben Zvi Assaraf and Knippels, 2022; Penzlin et al., 2022).
Systems thinking is conceptualized as higher-order thinking skills that help learners to “make sense of complexity” (Ben Zvi Assaraf and Knippels, 2022, p. 250). Thus, systems thinking skills are needed to explain biological phenomena as complex systems. Scholars have argued that modeling scaffolds learners in applying systems thinking skills by providing a focused representation of complex phenomena (Ben Zvi Assaraf and Knippels, 2022; Dauer et al., 2022; Tamir et al., 2023). This bridges representational modeling and systems thinking skills.
Although representational modeling is highly important to teach content knowledge about concrete phenomena (Stieff et al., 2016; Upmeier zu Belzen et al., 2019) in science and biology education, it is “insufficient to capture the full scope of the function of models” (Cheng et al., 2021, p. 308). Therefore, it is also important to consider the function of modeling for inquiry and its relation to systems thinking in science and biology education (Passmore et al., 2014; Gouvea and Passmore, 2017). Adding to the bridge between representational modeling and systems thinking skills, we propose to link systems thinking skills and modeling for inquiry: modeling for inquiry involves generating explanations for so-far-unexplained phenomena (e.g., Gouvea and Passmore, 2017; Upmeier zu Belzen et al., 2021). Thus, systems thinking skills are needed in modeling for inquiry to explain so-far-unexplained phenomena as complex systems.
Abductive reasoning is defined as the type of reasoning that generates causal explanations (e.g., Peirce, 1978; Magnani, 2004). It has been stated that abductive reasoning is the primary mode in model construction for inquiry (e.g., Svoboda and Passmore, 2013; Oh, 2019). Modelers apply abductive reasoning in model construction when they generate novel explanations using creative analogies or when they select between concurring explanations (Clement, 2008; Schurz, 2008). This important role of abductive reasoning in modeling for inquiry in biology has been justified by historical analysis of modeling processes leading to important ideas in biology (Adúriz-Bravo and González Galli, 2022), theoretical argumentations (Upmeier zu Belzen et al., 2021), and case studies (Clement, 2008; Svoboda and Passmore, 2013). In this study, we aim to add to these findings by examining the role of abductive reasoning in modeling for inquiry and the relationships between abductive reasoning and the ability to explain biological phenomena as complex systems. Generated inferences will contribute to research by providing further empirical arguments discussing the role of abductive reasoning in modeling of complex biological phenomena. In addition, the findings of this study should help to develop instructional strategies for modeling of phenomena as complex systems in biology education.
We conceptualize modeling for inquiry as the iteration between model construction and model application (Krell et al., 2019; Upmeier zu Belzen et al., 2021). This concept of modeling is supported by empirical evidence from studies that have examined the modeling processes of middle-school students (Meister and Upmeier zu Belzen, 2020) as well as pre-service biology teachers (Göhner and Krell, 2020; Meister et al., 2021; Göhner et al., 2022) and matches concepts of modeling among other researchers who use similar terminology (constructing and evaluating models, see Cheng et al., 2021; construct and improve models, see Nicolaou and Constantinou, 2014, p. 53; creating and using models, see Oh, 2019).
In modeling for inquiry, model construction is about generating a plausible explanation for a so-far-unexplained phenomenon (Gouvea and Passmore, 2017; Upmeier zu Belzen et al., 2021). Based on this perspective, a generated explanation for a phenomenon is the product of model construction and conceptualized as the model (Rohwer and Rice, 2016; Rice et al., 2019). Scientific inquiry aims to find causal explanations, i.e., to explain why and how phenomena emerge (Perkins and Grotzer, 2005; Haskel-Ittah, 2022). Causal explanations should at least provide a cause for why phenomena occur. Ideally, causal explanations in science combine a cause with a concrete mechanism that explains not only why but also how phenomena have emerged (Salmon, 1990; Alameh et al., 2022; Penzlin et al., 2022). Different modelers have different views of what counts as a satisfying explanation (Cheng et al., 2021). However, if a modeler has generated a plausible explanation for themselves, then “model construction temporarily ends” (Upmeier zu Belzen et al., 2021, p. 4). In the following stage of model application, the generated explanatory model is used to derive predictions and strategies to test them with inquiry methods, such as experiments or observations (Giere, 2009; Gouvea and Passmore, 2017; Upmeier zu Belzen et al., 2021).
Different stages of scientific inquiry are connected to different types of reasoning (Lawson, 2003, 2010; Adúriz-Bravo and Sans Pinillos, 2019). The relationships between and definitions of reasoning types in inquiry are discussed in the philosophy of science literature (e.g., Kuipers, 2004; Adúriz-Bravo and González Galli, 2022). According to Peirce (1978), induction, deduction, and abduction are the types of reasoning that are involved in scientific inquiry. Within the Peircean framework, inductive reasoning is defined as generalizing from observations, deductive reasoning as predicting based on existing theories or rules, and abduction as generating and selecting causal explanations. The example of observing a wet sidewalk has previously been used to illustrate these reasoning processes (e.g., Adúriz-Bravo and González Galli, 2022). Using inductive reasoning, one would generalize that all sidewalks are wet. Using deductive reasoning, one would predict that the next sidewalk one walks on will also be wet. Using abductive reasoning, one could generate the explanation that the wet sidewalk is caused by cleaning activity in the city, but upon considering that people are walking with raincoats and seeing gray clouds in the sky, one would decide that the wet sidewalk having been caused by rain is a more plausible explanation.
The three reasoning types are involved in modeling for inquiry (Upmeier zu Belzen et al., 2021). Induction is involved if models are constructed based on the generalization of observations. Induction leads to testable models but does not bring new ideas into modeling for inquiry (Wirth, 2003; Magnani, 2004; Upmeier zu Belzen et al., 2021). New ideas in model construction are generated by abductive reasoning (Wirth, 2003; Magnani, 2004; Upmeier zu Belzen et al., 2021), since abduction is about generating causal explanations for a phenomenon and selecting between them. Deductive reasoning is involved in model application when using models to derive predictions that act as hypotheses for planning and conducting further inquiry into the phenomenon (Dunbar, 2000; Giere et al., 2006; Halloun, 2007). In this study, we focus on abductive reasoning. We operationalize this by applying the theoretical concepts of the steps of abduction that have been proposed in a cognitive psychological framework of abductive reasoning (Johnson and Krems, 2001; Baumann et al., 2007) and the patterns of abduction that are described in the philosophy of science literature (Habermas, 1968; Wirth, 2003; Schurz, 2008).
In their framework, Johnson and Krems (2001) proposed seven steps of abduction, which are not taken in a fixed sequence; hence, they interact with each other and depend on situational preconditions. In our study, we use six of the steps to operationalize abductive reasoning in model construction (Table 1).
Table 1. Steps of abduction, adapted from Johnson and Krems (2001).
Test is another step proposed in Johnson and Krems's (2001) framework of abductive reasoning. The test step is about developing strategies (e.g., experiments) to further investigate the generated model. Those testing strategies are ideally based on model-derived predictive hypotheses (Giere et al., 2006; Godfrey-Smith, 2006). Deductive reasoning is considered to be the type of logical reasoning that leads to predictive hypotheses about a phenomenon. Thus, we do not refer to the test step as a step in abductive reasoning in model construction, but rather as the step that indicates the transition from abductive reasoning in model construction to deductive reasoning in model application (Upmeier zu Belzen et al., 2019, 2021).
The pattern of abduction that is applied in modeling for inquiry depends on how much modelers already know about possible explanations for a phenomenon (Habermas, 1968; Wirth, 2003; Schurz, 2008). The pattern of creative abduction is applied if modelers do not know possible explanations for the phenomenon (Schurz, 2008). Thus, they need to create a novel one, e.g., by creating analogies, which means transferring knowledge from other contexts (Clement, 2008). When Darwin observed the diversity of finches with different beak shapes and diets, he explained it through the concept of a common ancestor and evolution by natural selection over time. This was a novel explanation that he generated creatively based on the analogy of change in domesticated animals under human selection (Adúriz-Bravo and González Galli, 2022). The pattern of selective abduction is applied if modelers know about explanations (or at least about concrete causes) for the phenomenon and need to apply their knowledge to select plausible ones (Schurz, 2008). For example, if a patient presents with a common symptom such as high blood pressure, a doctor needs to apply knowledge of the patient's medical history to select one among many possible explanations for the symptom.
Biology is the science of life (Hillis et al., 2020). Biological phenomena are observable processes or events that occur within or involving living organisms at various levels of organization, from molecular to populational or biosphere levels. Since interactions among these levels result in emergent properties (Schneeweiß and Gropengießer, 2019, 2022), biological phenomena are inherently complex (e.g., Ben Zvi Assaraf and Knippels, 2022; Haskel-Ittah, 2022) and best explained as complex systems (Duncan, 2007; Hmelo-Silver et al., 2017; Snapir et al., 2017). A biological phenomenon is explained as a complex system if its explanation involves causes and mechanisms at different levels of organization (Schneeweiß and Gropengießer, 2019, 2022; Penzlin et al., 2022). As systems thinking skills help learners to understand and interpret complex systems (Dor-Haim and Ben Zvi Assaraf, 2022), they are needed to explain biological phenomena (Verhoeff et al., 2018). Among others, cross-level reasoning and identification of system components and relationships are important systems thinking skills (Tamir et al., 2023). These skills are addressed in the component mechanism phenomena (CMP) approach by Hmelo-Silver et al. (2017). The CMP approach addresses the skill of identifying the components of systems (which we consider as causes;1 Penzlin et al., 2022) and their relationships by emphasizing whether they are linked by mechanisms. Furthermore, the CMP approach addresses cross-level reasoning by emphasizing whether causes and mechanisms refer to micro- or macro-levels of biological organization (Hmelo-Silver et al., 2017; Snapir et al., 2017).
The role of abductive reasoning in scientific inquiry has been justified by theoretical and historical argumentation. Philosophers of science argue that revolutionary scientific ideas, such as Kepler's model of elliptic planet orbits or Darwin's theory of biological evolution, emerged by abductive reasoning, which means the generation and selection of novel explanations that expand what is already known about a natural phenomenon (Wirth, 2003; Schurz, 2008; Lawson, 2010; Adúriz-Bravo and González Galli, 2022). Since in modeling for inquiry a model is constructed as a possible explanation for a phenomenon (Rohwer and Rice, 2016; Rice et al., 2019), it has been argued that “the primary mode of reasoning during model construction is abductive” (Svoboda and Passmore, 2013, p. 124). By analyzing historical episodes of mathematical model construction, Park and Lee (2018) assign an abductive nature to mathematical modeling that leads to new models that are applied subsequently in mathematical inquiry. In case studies with pre-service elementary school teachers, Oh (2019, 2022) provides empirical evidence about abductive reasoning in modeling of geoscientific phenomena. The author states that the participants struggle to generate a plausible explanation if they search for a linear and direct relationship between a single cause and the observed phenomenon. Oh (2022) concludes that abductive reasoning is well-suited to the construction of models that explain phenomena in earth science if abductive reasoning is combined with systems thinking skills. Based on case studies with middle- and high-school students who constructed models for physical and biological phenomena, Clement (2008) argues that abductive reasoning is present in model construction, i.e., when modelers rely on analogies when generating explanations. This analogical reasoning connects to the pattern of creative abduction suggested by Schurz (2008, see Chapter 2.2). Svoboda and Passmore (2013) explicitly describe the usage of the selective abduction pattern during biological model construction in their article about modeling strategies among undergraduate biology students. They also describe how students apply creative abduction when generating models to explain phenomena by using analogies. The case studies of Clement (2008) and Svoboda and Passmore (2013) provide evidence that indicates the important role of abductive reasoning in modeling of biological phenomena.
In these related studies, the authors define abductive reasoning broadly as the reasoning that leads to the generation and selection of causal explanations for so-far-unexplained phenomena. In this article, we add to these studies by applying concrete theoretical concepts to operationalize abductive reasoning. These concepts are the proposed steps from the cognitive psychological framework of abduction (Johnson and Krems, 2001) and the patterns of creative and selective abduction as proposed by philosophers of science (e.g., Schurz, 2008). Furthermore, we aim to examine the relationship of these abductive reasoning concepts to the ability to model biological phenomena as complex systems.
Our research questions (RQ) are:
RQ1: To what extent are the steps of abductive reasoning present in modeling processes to explain biological phenomena?
RQ2: What are the differences between patterns of selective abduction and creative abduction when modeling biological phenomena?
RQ3: How do steps and patterns of abductive reasoning relate to modeling of biological phenomena as complex systems?
This study investigated abductive reasoning in modeling and its relation to modeling of biological phenomena as complex systems. Participants were 20 pre-service biology teachers (mean age = 27, SD = 2.6) from master's programs at two German universities. Participants were recruited in university seminars and confirmed their intention to voluntarily participate in this study via email before the interviews.
Using modeling for inquiry is challenging for both students and teachers (e.g., Cheng et al., 2021; Göhner et al., 2022). Therefore, the inclusion criterion for the participants in this study was that they had completed a course on scientific inquiry methods. In this course, they learned about using modeling as a method for inquiry, such as constructing models based on evidence or using a model to predict a phenomenon. Although they most likely engaged intuitively in abductive reasoning during modeling activities as part of the seminar, they were not explicitly taught about the concept of abduction. This allowed the examination of abductive reasoning in modeling biological phenomena for inquiry among individuals who had learned how to use modeling for inquiry without having been explicitly taught about abductive reasoning.
To analyze abductive reasoning processes in modeling, think-aloud interviews (Ericsson and Simon, 1980) were conducted; these were implemented online due to the pandemic situation in the winter of 2021. During the interviews, participants worked on a modeling task implemented in SageModeler (Bielik et al., 2018), which is an online application that allows learners to be engaged in several modeling activities from the drawing of simple diagrams to the construction of semi-quantitative simulation models. In our study, SageModeler was used as a drawing tool for creating process diagrams, enabling participants to create and label boxes and arrows. The more advanced features of the program, e.g., performing semi-quantitative simulations, were not needed for our study. Therefore, these features were not introduced to the participants and were disabled in the settings section of the SageModeler online environment. We chose SageModeler as the drawing tool for this study because it allows the drawing of process diagrams on a computer. Thus, it was a solution to enable monitoring of the drawing processes, even in an online interview situation. Additionally, we had prior experience in using this tool for drawing diagrams in previous studies with pre-service science teachers. In those studies, we found that SageModeler had good usability for a task that requires the drawing of process diagrams (Engelschalt et al., 2023).
The instruction for the modeling task given to the participants was “Draw your solution process of how a specific phenomenon has emerged in a process diagram while referring to concrete causes.”
Abductive reasoning in model construction is about generating a causal explanation for a phenomenon. Causal explanations in science ideally include causes and mechanisms (Salmon, 1990; Alameh et al., 2022; Penzlin et al., 2022). By prompting the participants to find concrete causes and elaborate on how the phenomenon emerged, this instruction referred to both generating causes and mechanisms to explain a phenomenon and was applied to operationalize abductive reasoning processes in model construction. This instruction was also open for the participants to develop strategies to test their explanations; this corresponds to Johnson and Krems's (2001) test step, which according to our conception indicates the transition from abductive reasoning in model construction to deductive reasoning in model application.
Drawing their solution process in a process diagram was implemented as a way to scaffold participants' mental modeling activities. Furthermore, the models and modeling processes thereby externalized were analyzed regarding their complexity by applying the CMP approach (see Section Complexity in model construction processes). Examples of the process diagrams produced can be found in Figures 1A, B.
Figure 1. Examples process diagrams by (A) participant CFP08 and (B) participant RFP05. While participant CFP05 referred to causes and mechanisms that might explain the CFP, RFP05 referred only to causes that might explain the RFP but additionally added strategies to test the causes.
Two biological phenomena were chosen as contexts for the task. One phenomenon concerned a person with a reddened face (the reddened face phenomenon, RFP). The other concerned a male clownfish changing its sex after the only female fish in the population died (the clownfish phenomenon, CFP). We applied these phenomena to operationalize the patterns of abduction. Specifically, the RFP is relevant to participants' daily lives and most participants likely have personal experience with it. Therefore, we expected participants to know about explanations or at least causes for a person's reddened face. This argumentation is also supported by the findings of a previous study in which we implemented the RFP modeling task with pre-service science teachers and most participants generated multiple explanations for the phenomenon (see Upmeier zu Belzen et al., 2021). Thus, the RFP was used to operationalize the pattern of selective abduction (Schurz, 2008). On the other hand, as the CFP is a very specific biological phenomenon, we did not expect that most of the participants would know of an explanation for it. Given this, model construction for the CFP is about creating a plausible explanatory model by transferring knowledge from other contexts and the challenge is more to find a possible explanation meeting the given constraints. Thus, the CFP was applied to operationalize the pattern of creative abduction (Schurz, 2008). In this way, the patterns of abduction were operationalized by applying two phenomena as modeling contexts: in the RFP context, participants were expected to know about explanations or at least concrete causes, while in the CFP context, participants were not expected to have such knowledge. To ensure this difference in the modeling contexts, we excluded participants from the analysis if they reported in the think-aloud interview that they already knew about a specific explanation for the CFP or if they reported not knowing of explanations for the RFP (see Section Data processing). To generate more detailed evidence on participants' prior knowledge about explanations for the RFP and CFP, pre-tests could have been performed. Like other studies assessing knowledge and reasoning processes involved in modeling (Ruppert et al., 2017; Bennett et al., 2020), we decided against pre-testing our participants' prior knowledge about explanations for the CFP and RFP. We justify this with three arguments:
1. There are many causes to explain the RFP, and anticipation of all knowledge that is related to these causes is neither economic nor possible to fully achieve in a pre-test.
2. Prompts employed in prior knowledge pre-tests could have possibly influenced which knowledge participants would refer to, which would make their responses to the modeling task less spontaneous and less authentic.
3. Think-aloud interviews as conducted for this study are linked to high cognitive load and fatigue among the participants (Sandmann, 2014). Answering a knowledge pre-test before the interview could enhance cognitive load and fatigue.
Participants were interviewed using the think-aloud method (Ericsson and Simon, 1980; Sandmann, 2014). Under this method, participants were asked to speak out loud about any thoughts that came into their minds while working on the modeling task. The method of think-aloud has been shown to capture reasoning processes (Sandmann, 2014; Leighton, 2017). Matching this, think-aloud has been implemented in previous studies examining pre-service science teachers' (Meister et al., 2021; Göhner et al., 2022) and high-school students' (Meister and Upmeier zu Belzen, 2020) reasoning processes in modeling for inquiry. The structure of the interviews followed the suggestion by Sandmann (2014): after a short introduction about the aim of the interview and an explanation of the think-aloud method, participants started with a warm-up task to get used to speaking every thought out loud. In this study, the warm-up task was to formulate a heading for a short picture story. Before working on the modeling task, each participant watched a short video (1:42 min) that explained how to draw a process diagram in SageModeler. After watching the video, either the RFP or the CFP was randomly presented to the participant in the form of a short text to read. Randomization was automatically implemented in SoSci Survey. While the participant worked on the modeling task (either the CFP or the RFP modeling task), the interviewer did not comment on their thoughts. The interviewer only replied to questions from the participant that concerned their general understanding of the instruction. If a participant asked specific questions about the phenomena, the interviewer did not answer them concretely and just referred to the task. On average, the interviews lasted around 21 min each (M = 20.87 min, SD = 5.7 min).
The audio of the interviews and the screens of the interviewed participants were recorded. The audio was transcribed. Furthermore, the process diagrams produced were collected via a shared link. Two participants were excluded from the analysis. One was excluded since the participant (pseudonym CFP01) stated that they already knew of an explanation for the CFP. Therefore, the participant had explicit prior knowledge about the CFP, which does not match the definition of creative abduction (Schurz, 2008). The other participant (pseudonym RFP09) modeled the RFP and was excluded due to not being able to produce a process diagram in SageModeler, which inhibited this participant's progression in the task.
To analyze participants' engagement in abductive reasoning steps during model construction, a coding scheme was developed based on Johnson and Krems (2001, Table 2). In the development process, the steps collect data and comprehend were adapted from their original descriptions. This was necessary due to differences in the task format. In contrast to our task, the task used in the study by Johnson and Krems (2001) allowed the participants to always collect additional data, which they needed to comprehend. While in Johnson and Krems's framework collect data was about actively generating data and comprehend was about understanding the collected data, in our study collect data was about explicating ideas on how to generate data and comprehend was about understanding the data that were given in the modeling task instruction.
The test step, which is another step in Johnson and Krems's framework of abduction, was used to operationalize the transition from abductive reasoning in model construction to deductive reasoning in model application in this study.
The coding scheme shown in Table 2 was used to identify the abductive reasoning steps in the transcripts of the interviews. Coding was performed using the MAXQDA program (VERBI Software, 2022), which allowed coders to watch recorded videos while coding passages from the transcripts. Coders were instructed to assign codes to related passages that were as short as possible but as long as necessary. Therefore, passages of varying lengths (from small word groups to several sentences) were assigned to the steps. Passages that did not fit into any of the steps (such as when participants talked about how they arranged their diagram) were not coded. The reliability and objectivity of the analysis were supported by substantial intra-rater agreements for two transcripts (k = 0.73, calculated according to Brennan and Prediger, 1981; interpreted according to Landis and Koch, 1977) and substantial inter-rater agreements between two coders for six transcripts (k = 0.71, Landis and Koch, 1977). Agreement was counted if at least 95% of a passage received the same code from the two independent coders.
Referring to RQ1, the occurrence and frequency of each of the steps were analyzed. This was done by examining which of the steps occurred in each participant's transcript and how often they occurred. By counting occurrences of each step, we gathered information about how often a step occurred in modeling processes for each participant and overall for the 18 participants whose data were analyzed.
Referring to RQ2, frequencies of the abductive reasoning steps addressed were compared between CFP participants and RFP participants to examine possible differences between the modeling processes.
In modeling for inquiry, models are constructed as explanations for phenomena (Rice et al., 2019; Upmeier zu Belzen et al., 2021). If this explanation involves causes and mechanisms on different organizational levels, the phenomenon is explained as a complex system. However, modelers are not always able to formulate mechanisms. In such cases, phenomena are only explained by a cause. This is why, for our analysis, we defined a model as an attempt to explain the phenomenon that includes at least one concrete cause for its emergence.
Both implemented phenomena, the RFP and CFP, refer to physiological processes within an organism as well as the interplay of an organism with the environment. Thus, they can be explained as complex systems (Hmelo-Silver et al., 2017; Snapir et al., 2017). Our task instruction allowed the participants to suggest several concurring models for the same phenomenon. Therefore, we did not analyze the complexity of single models but all models that participants proposed in their model construction processes. Participants' model construction processes were analyzed discursively in terms of complexity by two coders who analyzed the diagrams in combination with the think-aloud protocols. Therefore, a coding scheme was adapted based on the CMP approach (Hmelo-Silver et al., 2017). The approach scores complexity based on connections between causes (C, originally labeled components by Hmelo-Silver et al., 2017, see Chapter 2.3), mechanisms (M), and the phenomenon (P) in the CMP score and the connection of micro- and macro-levels of organization in the micro–macro score.
The adaptation of the scheme for our study mainly involved changes in the CMP score. In the study of Hmelo-Silver et al. (2017), participants were instructed to model a lake ecosystem. The participants received points for describing concrete phenomena within their externalized models. The instruction of our study differed from the study of Hmelo-Silver et al. (2017) in that our participants were explicitly prompted to find causes for a given phenomenon (the RFP or CFP). Therefore, a concrete phenomenon was described in the instruction, and only representing this description in the instruction was not scored (“P,” Table 3). Participants who generated only one cause, which they directly connected to the emergence of the phenomenon (“C→P”), generated a simple linear explanation that is most likely not adequate for explaining biological phenomena (Haskel-Ittah, 2022). Participants who generated multiple causes (|:C→P:|) showed higher complexity in their model construction processes, because this indicates that they acknowledged the presence of more than one entity that might cause a phenomenon. However, only when they included at least one cause and a mechanism to explain the phenomenon had participants explained it as a complex system. Participants who connected several causes and mechanisms to explain the phenomenon (“|:C→M→P:|”) demonstrated the highest levels of complexity in their model construction processes. This indicates that they recognized that multiple entities in a system can cause a biological phenomenon and that there are hidden mechanisms that lead to the emergence of biological phenomena. Within the coding scheme, causes were defined as the initial entity for why the phenomenon emerged (Kampourakis and Niebert, 2018) and mechanisms were defined as the entity's activities and interactions describing how the phenomenon emerged (Craver and Darden, 2013; Haskel-Ittah, 2022). Direct arrows from cause to phenomenon without any descriptions or arrows containing verbal connection that include only vague filler terms such as influence, affect, and lead to (black boxes, Haskel-Ittah, 2022) were not counted as concrete mechanisms and thus not scored under our scheme. Technical terms summarizing concrete biological mechanisms such as natural selection or blood vessel dilation were coded as mechanisms.
The coding scheme for the micro–macro score was adopted from Hmelo-Silver et al. (2017). The lowest micro–macro scores were coded when participants only referred to either the micro- or macro-level of biological organization (Table 4). The highest scores were coded when participants connected elements on both the micro- and macro-levels during model construction. The latter indicates that participants took the complexity of biological organization into account.
The micro-level refers to “the part of reality that is only accessible through the use of science-based technologies such as microscopes” (see microcosm, Schneeweiß and Gropengießer, 2022, p. 145), which are parts on the cell level and below (Hmelo-Silver et al., 2017; Schneeweiß and Gropengießer, 2022). Parts on the tissue level and above (e.g., organisms and populations) were considered as macro-level entities (see mesocosm and macrocosm, Schneeweiß and Gropengießer, 2022). Emotions such as anger or shame were scored as macro-level causes for the RFP, since they are reactions of a person (organism) to a specific situation.
Referring to RQ3, the relationship between abductive reasoning patterns and complexity, as along with the relationship between the abductive reasoning steps and complexity, was examined. Specifically, the relationship between complexity in model construction and abductive reasoning patterns was analyzed by comparing how many participants achieved high scores in CMP (scores of 3 and 4) and micro–macro (score of 3) for the RFP (selective abduction) and the CFP (creative abduction). To investigate possible relationships between the complexity of generated models and abductive reasoning steps, we analyzed whether frequent engagement in abductive reasoning steps correlated with CMP and micro–macro scores. Therefore, the frequency of abductive reasoning steps that each participant engaged in was counted. Subsequently, Spearman's correlation coefficients (Field, 2013) between the frequency of engagement in abductive steps and complexity scores (CMP score and micro–macro score) were calculated.
In this study, we applied Johnson and Krems's (2001) framework to operationalize cognitive processes in abductive reasoning with six steps that were analyzed by a coding scheme. Analysis showed that all six steps were present in the modeling processes of our 18 participants (Table 5). According to our coding, abductive reasoning steps occurred approximately 9 times (M = 9.33, SD = 6.12) in the model construction processes of each participant. However, only the comprehend step was found in the transcripts of all participants. Although the refine and check steps were coded frequently, in most of the transcripts, the collect data and discriminate steps were coded rarely. The step resolve anomaly was coded once and independently by the two coders at the same position in the relevant transcript.
Table 5. Frequency of coded abductive reasoning steps and number of participants who referred to them when modeling the CFP or RFP.
We applied the CFP to operationalize the pattern of creative abduction and the RFP to operationalize the pattern of selective abduction. While the frequencies of refine, check, and discriminate were similar between the modeling processes for the CFP and the RFP (Table 5), we found five differences between the phenomena.
1. Presence of collect data. The collect data step was found in the modeling processes of the RFP, but not the CFP. The code appeared when participants explicated strategies for how to examine the phenomenon generally, without explicit assumptions, and mostly (in all but one case) before participants explicated a model for explaining the phenomenon.
“First of all, of course, I would examine the room, yes observe the room, I'll write ‘observe the room'. Then I would look if I found things or objects that explain the problem or the red face.” (Think-aloud transcript of RFP06, passage related to the code collect data, at the beginning of the transcript).
2. Initiation of the modeling process. While all CFP participants started their model construction with comprehend, this was only the case for four participants modeling the RFP. Three participants modeling the RFP explicated strategies referring to collect data first and two participants started immediately with the generation of models for the RFP. On average, CFP participants needed a longer period of time before they generated their first primary model to explain the phenomenon than RFP participants (Figure 2). As an extreme example, participant CFP09 only represented the information given by the instruction in the diagram and thus did not generate any model for the CFP. The participant finished the task by claiming not to be able to produce a better solution due to a lack of knowledge of clownfish.
3. Proposal of alternative models. For both phenomena, most participants generated at least two alternative models for their phenomenon (8 out of 9 for the RFP; 6 out of 9 for the CFP). However, while CFP participants worked longer on one generated model by checking its plausibility and refining it, participants modeling the RFP often continued in their modeling process by proposing alternative models to explain the RFP immediately. This was observed 13 times in the modeling processes of five RFP participants, as illustrated by the following quote, where three models to explain the RFP are generated immediately:
“Exercise is a possible explanation for the reddened face. I write down ‘Person may have done exercise'. The person could also have a fever […] I write down ‘Person may have fever'. Or the person could also deal with high blood pressure.” (Think-aloud transcript of RFP03, underlined passages are first primary models to explain the RFP).
4. Plausibility check of generated models. A plausibility check of generated models was found more often for the CFP (n = 37) than for the RFP (n = 18). Within the passages that were coded as check, participants modeling the CFP reported uncertainty about their models, as illustrated by this quote:
“I am uncertain if I have taken the right path, so I am going through it again. The phenomenon is: […] The female dies, the strongest male turns back into a female, and the same clownfish population is created. [...] I assume it could be death, which is related to the absence of certain hormones that are no longer released. Whether it has to do with fish perception, I am unsure, but it does somehow result in a change in gene expression.” (Think-aloud transcript of CFP06, passage related to the code check).
Furthermore, the uncertainty of CFP participants was frequently linked to vague explanations in combination with the explication of lacking specific prior knowledge about clownfish:
“The female changes something in the environment […]. So, it is not about other living beings. I do not know anything about clownfish. [The female clownfish] can send any information somehow into the water” (Think-aloud transcript of CFP02, passage related to the code check).
Plausibility checks in RFP modeling processes were less frequent (n = 18), and seldom linked to uncertainty and vague formulations. In contrast, participants referred to prior experiences from their everyday lives to justify the plausibility of their generated models:
“Nervosity makes sense. My best friend, for example, always blushed extremely when she had to present something in front of the class” (Think-aloud transcript of RFP01, passage related to the code check).
5. Although the focus of our study was on examining abductive reasoning in modeling, the fifth examined difference relates not only to abductive reasoning in model construction but moreover to the transition from abductive reasoning in model construction to deductive reasoning in model application. In our study, the transition to model application was operationalized by Johnson and Krems's (2001) test step, when strategies on how to investigate generated explanations were developed. Test was coded 18 times for eight of the 18 participants. It was considered twice as often for the RFP (n = 12, from six participants) as for the CFP (n = 6, from two participants).
“If I want to examine whether doing exercise is the cause, I could measure heart rate.” (Think-aloud transcript of RFP05, passage related to the code test. RFP05 also included testing strategies in the generated process diagram, see Figure 1B).
Figure 2. Amount of time that every participant needed to generate an initial explanatory model for the phenomenon*. *Participant CFP09 did not produce a model to explain the phenomenon. Participants RFP09 and CFP01 were excluded from the analysis (see Section Data processing).
We operationalized the extent to which participants modeled the phenomena as complex systems by examining CMP and micro–macro relations, as proposed by Hmelo-Silver et al. (2017). Participants achieved an average CMP score of 2.72 (SD = 1.23) and an average micro–macro score of 2.20 (SD = 0.97).
We found a significantly strong correlation between the frequency of abductive reasoning steps and CMP score (r = 0.52, p < 0.05; Cohen, 1988, Figure 3). On the level of concrete steps, significant correlations were found between the CMP score and the frequency of refine (r = 0.48, p < 0.05) and check (r = 0.51, p < 0.05). However, no correlation was found between the frequency of abductive reasoning steps and the micro–macro score (Figure 2).
Figure 3. Distribution of participants' CMP scores (left) and micro-macro scores (right) in relation to the frequency of abductive reasoning steps that occurred in their modeling processes.
Referring to both CMP and micro–macro scores, CFP participants addressed higher complexity in their model construction processes than RFP participants (Table 6). While most of the CFP participants achieved the highest scores for complexity regarding CMP relations (5 out of 9) and micro–macro relations (6 out of 9), this was only the case for two of the nine CFP participants.
It is also notable that six of the eight participants who transitioned from model construction to model application by developing strategies to test their explanations showed a low CMP score (all six received a CMP score of 1) and a low micro–macro score (five participants received a micro–macro score of 1 and one participant received a micro–macro score of 2). Thus, only two participants developed strategies to test generated explanations and received high complexity scores for model construction (a CMP score of 3 or 4 and a micro–macro score of 3). Both participants modeled the CFP.
Johnson and Krems (2001) stated that abductive reasoning processes do not always include all proposed steps. Congruently with this, we observed some steps more frequently than others. The steps collect data, discriminate, and resolve anomaly were only found rarely in the modeling processes of this study's participants. However, this does not necessarily indicate that these steps are not important in biological model construction, since their rare presence is probably explained by the limitations of this study's modeling task format. For instance, to be able to collect data, it is important to observe the phenomenon (Greve and Wentura, 1997; Constantinou, 1999). This was hardly possible in the modeling task of our study. Participants could only use information about the phenomenon that was given to them in the instruction to explicate ideas on how they might collect data.
Hence, comprehend, refine, and check were frequently found in the modeling processes in our study, and this indicates the important role of these steps in model construction for biological phenomena. We assume that the steps collect data, discriminate, and resolve anomaly, which we rarely found in our data, are also involved in model construction for biological phenomena. For instance, studies with more interactive modeling tasks have shown that collecting data is an important part of modeling for inquiry (e.g., Constantinou, 1999; Meister et al., 2021).
The pattern of abduction that is applied in model construction depends on the extent to which modelers already know about possible explanations for a phenomenon (Schurz, 2008). For operationalization of creative abduction, we applied the CFP as a modeling context in which participants did not know of explanations. For operationalization of selective abduction, we applied the RFP as a modeling context in which participants knew of explanations, e.g., from their everyday lives and individual experiences with the phenomenon. The findings that CFP participants explicated lacking knowledge and RFP participants referred to concrete examples from everyday life during their model construction support this methodological operationalization. Moreover, we identified five differences between the modeling processes of the CFP and the RFP, which is consistent with previous research suggesting that engagement in the modeling process is context-dependent (Svoboda and Passmore, 2013; Bennett et al., 2020; Schwarz et al., 2022).
The first difference examined relates to participants' wishes and ideas to collect data, only found in RFP modeling processes. This may be interpreted as a wish to obtain evidence to be able to select between possible alternatives in selective abduction. However, the format of the task did not allow the participants to collect new data about the phenomena, which might have inhibited them from discriminating between generated explanations based on data. Thus, this limitation of the modeling task might also explain the infrequent occurrence of discriminate, especially in modeling processes for the RFP, in which most participants generated concurring models.
The second difference examined was about the initiation of model construction: while all RFP participants generated their first explanatory model relatively quickly—two participants started generating models right away—all CFP participants began with an attempt to comprehend the phenomenon at first and needed more time to construct a (primary) explanatory model. The observation that learners spend a great deal of time comprehending what is going on when they construct models for phenomena that they do not know much about is also reported by other scholars (e.g., Bierema et al., 2017; Schwarz et al., 2022). Participant CFP09 only engaged in the comprehend step and did not generate a plausible model. This example illustrates how a lack of knowledge about a phenomenon and the inability to create analogies inhibit model construction in such a way that no plausible explanation for a phenomenon can be generated (Göhner and Krell, 2020; Göhner et al., 2022). We interpret the differences in initial model construction (i.e., longer time spent comprehending the CFP compared to the quick generation of explanatory models for the RFP) as indicators of higher difficulty in constructing explanatory models for the CFP than for the RFP. This is also supported by the third and fourth differences examined (RFP participants generated alternative models more quickly than CFP participants, and CFP participants checked their generated models for internal consistency more often than RFP participants did).
The fifth difference was that RFP participants engaged more frequently in the test step than CFP participants. Thus, RFP participants transitioned more often from generating explanations in model construction to testing explanatory model applications. This result might indicate that developing strategies to test generated models is easier when modelers can rely on explanations from their prior knowledge. This connects to studies in the field of experimental competencies stating that prior contextual knowledge influences students' ability to plan experiments for scientific inquiry (Schwichow and Nehring, 2018). To illustrate this argument with examples from this study's modeling contexts, it seems easier to develop testing strategies to determine whether a person's reddened face is caused by exposure to the sun or alcohol abuse than to develop strategies for testing whether the sex change of a male clownfish is caused by the absence of female pheromones. This supports argumentation from Schwarz et al. (2022), who argue that “the more a person or group ‘knows' about the phenomena […], the more they can do within that modeling context.” (p. 1,091). Another explanation for the fact that CFP participants engaged less frequently in the test step can be derived from the result that they needed more time to generate their models. Although there was no time limit for the interviews, constructing plausible models for the CFP was time-consuming (Figure 3) and thus might have been mentally exhausting. As a result, participants may have eventually become cognitively fatigued and lost further motivation to derive strategies to test their generated models.
Model construction is about generating a plausible explanation for a phenomenon (e.g., Upmeier zu Belzen et al., 2021; Adúriz-Bravo and González Galli, 2022). Phenomena are explained as complex systems if their explanations include causes and mechanisms on different organizational levels (Schneeweiß and Gropengießer, 2019, 2022; Penzlin et al., 2022). In this study, we analyzed the complexity of model construction processes determining the extent to which participants explain a phenomenon as a complex system during model construction. Therefore, we applied the CMP approach of Hmelo-Silver et al. (2017) to evaluate the extent to which participants linked causes and mechanisms to explain a phenomenon (CMP score) and the extent to which they linked micro and macro levels of biological organization (micro–macro score).
Adúriz-Bravo and González Galli (2022) assumed that the complexity of initial generated explanations will be low as a result of individuals staying close to intuitive formulations and will probably increase during the process of abductive reasoning in model construction. The significant correlation between frequencies of abductive reasoning steps with CMP scores supports this assumption, by indicating that extensive abductive reasoning in model construction is related to the connection of causes and mechanisms to explain the phenomenon in model construction. However, no correlations were found between the frequency of abductive reasoning steps and the micro–macro score. This implies that extensive abductive reasoning does not necessarily lead to the connection of macro and micro levels, which indicates that abductive reasoning alone is not enough to explain phenomena as complex systems in biological model construction. We assume that an interplay between abductive reasoning and systems thinking skills, such as cross-level reasoning (Tamir et al., 2023), is necessary for explaining biological phenomena as complex systems in model construction. This idea has also been proposed in the field of earth science education by Oh (2019, 2022). On the other hand, with respect to the large number of different organizational levels that can be addressed when generating biological explanations (Schneeweiß and Gropengießer, 2019, 2022), the distinction between micro- and macro-levels as suggested by the CMP approach (Hmelo-Silver et al., 2017) could fall short to examine a possible relationship with abductive reasoning steps. Consequently, it might be powerful to consider a more fine-grained analysis of the organizational levels addressed, and how they are connected in the interplay of cause, mechanism, and phenomenon, as was done in the study by Penzlin et al. (2022).
In addition to connecting causes and mechanisms on different organizational levels, the systems thinking literature suggests that further skills need to be applied to explain phenomena as a complex system. Among others, these skills also include developing complex mechanisms such as feedback loops or considering the system's change over time (Ben Zvi Assaraf and Knippels, 2022; Tamir et al., 2023). Future studies are needed to examine how cognitive processes of abductive reasoning, which we operationalized as the steps of abduction (Johnson and Krems, 2001), are related to further systems thinking skills.
CFP participants addressed higher complexity in their model construction processes than RFP participants according to both CMP and micro–macro scores. This indicates that participants modeling the CFP tended to explain their phenomenon as a complex system, combining causes and mechanisms across micro- and macro-levels of biological organization. In contrast, participants modeling the RFP mostly referred to simple cause-and-effect relationships in their model construction processes. We explain this by the strong everyday life relevance of the RFP. In everyday life situations, explanatory models usually do not refer to multiple causes and mechanisms on different organizational levels but to simple cause–effect relations. It is likely that the pre-service biology teachers engaged in their master's studies who participated in our study would be capable of explaining the RFP as a complex system. However, most of the RFP participants constructed simple models and transitioned to developing strategies to test them in model applications. Göhner et al. (2022) found that if modelers constructed complex models, this would not automatically lead them to engage in model application. Moreover, to transition from model construction to model application, modelers need to perceive their generated models as plausible. For our results, this might indicate that less complex models for the RFP were plausible and therefore suited to enabling the participants to move on by developing strategies to test their generated models. Since only two participants (both of whom modeled the CFP) engaged in model application and received high complexity scores, our results might suggest that addressing high complexity in model construction could stunt the transition to model application. Explaining phenomena as complex systems in model construction and developing strategies to test these complex explanations in model application are difficult tasks that require the highest level of systems thinking skills (Ben Zvi Assaraf and Knippels, 2022; Tamir et al., 2023) and modeling competencies (Upmeier zu Belzen et al., 2021). Thus, it is not surprising that only two of the 18 participants explained their phenomenon as a complex system in model construction and developed strategies to test generated explanations in model application.
An important role of abductive reasoning in modeling for inquiry in biology has been justified by historical analysis of modeling processes leading to important ideas, such as Darwin's theory of evolution (Adúriz-Bravo and González Galli, 2022), theoretical argumentations (Upmeier zu Belzen et al., 2021), and case studies (Clement, 2008; Svoboda and Passmore, 2013). With this study, we add to prior findings by applying concrete theoretical concepts to operationalize abductive reasoning in the form of the steps (Johnson and Krems, 2001) and the patterns of abduction (Schurz, 2008), and by examining their role in modeling of biological phenomena as complex systems. Our results provide evidence that the abductive reasoning steps comprehend (understanding the phenomenon), check (evaluating the plausibility of an explanation), and refine (specifying an explanation) are involved in model construction for biological phenomena. However, participants' frequent engagement with these steps alone did not indicate that they were explaining phenomena as complex systems. As also suggested in the field of earth science education (Oh, 2022), we assume that an interplay between abductive reasoning and systems thinking skills, such as cross-level reasoning (Tamir et al., 2023), is needed to explain biological phenomena as complex systems in model construction. Testing this assumption in future studies will require a fine-grained examination of abductively generated explanations, as in the study by Penzlin et al. (2022).
The creative pattern of abduction, as operationalized by the CFP modeling context, was associated with frequent consistency checks and high complexity in model construction. However, there were rare transitions from generating explanations in model construction to testing them in model application. This may suggest that modeling contexts in which learners need to creatively generate a novel explanation for a phenomenon do not encourage them to test the generated explanations. Nevertheless, these contexts may be suited to fostering learners' construction of complex explanatory models. On the other hand, the selective pattern of abduction, as operationalized by the RFP modeling context, was connected to rapid generation of multiple simple models and to frequent transitions from model construction to model application. This might indicate that modeling contexts in which learners already have explanations for a phenomenon may not foster learners' construction of complex models. However, such contexts could be suitable to foster learners' transition from generating explanations in model construction to testing them in model application.
The findings of this study are limited by the openness of the format of the modeling task and its small sample of 18 pre-service science teachers during their master's studies. The stated differences between creative and selective abduction operationalized by the CFP and RFP in this study need to be supported with further evidence by larger studies on pre-service teachers' modeling processes and studies that operationalize patterns of creative and selective abductive reasoning with other biological phenomena. To further investigate the other findings of this study (for instance, to examine the extent to which complexity in model construction stunts transition to model application), studies with focused modeling tasks that guide participants more during their modeling processes are needed.
The raw data supporting the conclusions of this article will be made available by the authors, without undue reservation.
In accordance with local legislation and institutional requirements, our study did not require the approval of an Ethics Committee because the research did not pose any threats or risks to the participants, and it was not associated with high physical or emotional stress. Nevertheless, it is understood, that we strictly followed ethical guidelines as well as the Declaration of Helsinki. Before taking part in our study, all participants were informed about its objectives, absolute voluntariness of participation, possibility of dropping out of participation at any time, guaranteed protection of data privacy (collection of only anonymized data), no-risk character of study participation, and contact information in case of any questions or problems. The studies were conducted in accordance with the local legislation and institutional requirements. The participants provided their written informed consent to participate in this study.
PE, AU, and DK: conceptualization. PE, MR, and JP: methodology, validation. PE and MR: analysis, investigation, and visualization. AU, PE, and MR: data curation. PE: writing—original draft preparation. PE, AU, JP, and DK: writing—review and editing. AU and DK: supervision. All authors contributed to the article and approved the submitted version.
This work was supported by a fellowship of the German Academic Exchange Service (DAAD; Grant number: 57647563). The article processing charge was funded by the Deutsche Forschungsgemeinschaft (DFG, German Research Foundation) - 491192747 and the Open Access Publication Fund of Humboldt-Universität zu Berlin.
The authors declare that the research was conducted in the absence of any commercial or financial relationships that could be construed as a potential conflict of interest.
All claims expressed in this article are solely those of the authors and do not necessarily represent those of their affiliated organizations, or those of the publisher, the editors and the reviewers. Any product that may be evaluated in this article, or claim that may be made by its manufacturer, is not guaranteed or endorsed by the publisher.
1. ^The CMP approach has been applied to assess how learners describe complex systems (Hmelo-Silver et al., 2017; Snapir et al., 2017). In the systems thinking literature, and under the CMP approach, the term “component” is commonly used to describe entities in a system's explanation (e.g., Goldstone and Wilensky, 2008; Ben Zvi Assaraf and Knippels, 2022). However, in this study, we apply the CMP approach to modeling for inquiry, which aims to explain the emergence of a phenomenon. In the context of scientific inquiry, the term “cause” is used to describe the initial entity that leads to the emergence of the phenomenon. Therefore, in this article, we use the term “cause” instead of “component” when applying the CMP approach to modeling for inquiry.
Adúriz-Bravo, A., and González Galli, L. (2022). “Darwin's ideas as epitomes of abductive reasoning in the teaching of school scientific explanation and argumentation,” in Handbook of Abductive Cognition, ed L. Magnani (Cham: Springer International Publishing), 1–37. doi: 10.1007/978-3-030-68436-5_50-1
Adúriz-Bravo, A., and Sans Pinillos, A. (2019). “Abduction as a mode of inference in science education,” in 15th International History, Philosophy and Science Teaching Conference (Thessaloniki).
Alameh, S., Abd-El-Khalick, F., and Brown, D. (2022). The nature of scientific explanation: examining the perceptions of the nature, quality, and “goodness” of explanation among college students, science teachers, and scientists. J. Res. Sci. Teach. 60, 100–135. doi: 10.1002/tea.21792
Baumann, M. R. K., Bocklisch, F., Mehlhorn, K., and Krems, J. F. (2007). “Changing explanations in the face of anomalous data in abductive reasoning,” in Proceedings of the Annual Meeting of the Cognitive Science Society, Vol. 29. Available online at: https://escholarship.org/uc/item/1zm7n9sq
Ben Zvi Assaraf, O., and Knippels, M.-C. P. J. (2022). “Lessons learned: synthesizing approaches that foster understanding of complex biological phenomena,” in Fostering Understanding of Complex Systems in Biology Education: Pedagogies, Guidelines and Insights From Classroom-Based Research, eds O. Ben Zvi Assaraf and M.-C. P. J. Knippels (Cham: Springer International Publishing), 249–278. doi: 10.1007/978-3-030-98144-0_12
Bennett, S., Gotwals, A. W., and Long, T. M. (2020). Assessing students' approaches to modelling in undergraduate biology. Int. J. Sci. Educ. 42, 1697–1714. doi: 10.1080/09500693.2020.1777343
Bielik, T., Opitz, S., and Novak, A. (2018). Supporting students in building and using models: development on the quality and complexity dimensions. Educ. Sci. 8:149. doi: 10.3390/educsci8030149
Bierema, A. M.-K., Schwarz, C. V., and Stoltzfus, J. R. (2017). Engaging undergraduate biology students in scientific modeling: analysis of group interactions, sense-making, and justification. CBE Life Sci. Educ. 16:ar68. doi: 10.1187/cbe.17-01-0023
Brennan, R. L., and Prediger, D. J. (1981). Coefficient kappa: some uses, misuses, and alternatives. Educ. Psychol. Measure. 41, 687–699. doi: 10.1177/001316448104100307
Cheng, M.-F., Wu, T.-Y., and Lin, S.-F. (2021). Investigating the relationship between views of scientific models and modeling practice. Res. Sci. Educ. 51, 307–323. doi: 10.1007/s11165-019-09880-2
Clement, J. (2008). Creative Model Construction in Scientists and Students: The Role of Imagery, Analogy, and Mental Simulation. Dordrecht: Springer. doi: 10.1007/978-1-4020-6712-9
Cohen, J. (1988). Statistical Power Analysis for the Behavioral Sciences, 2nd Edn. Hillsdale, NJ: L. Erlbaum.
Constantinou, C. P. (1999). The Cocoa Microworld as an environment for developing modelling skills in physical science. Int. J. Contin. Eng. Educ. Life Long Learn. 9, 201–209. doi: 10.1504/IJCEELL.1999.030149
Craver, C. F., and Darden, L. (2013). In Search of Mechanisms: Discoveries Across the Life Sciences. University of Chicago Press. Available online at: https://press.uchicago.edu/ucp/books/book/chicago/I/bo16123713.html
Dauer, J., Dauer, J., Lucas, L., Helikar, T., and Long, T. (2022). “Supporting university student learning of complex systems: an example of teaching the interactive processes that constitute photosynthesis,” in Fostering Understanding of Complex Systems in Biology Education: Pedagogies, Guidelines and Insights From Classroom-based Research, eds O. Ben Zvi Assaraf and M.-C. P. J. Knippels (Cham: Springer International Publishing), 63–82. doi: 10.1007/978-3-030-98144-0_4
Dor-Haim, S., and Ben Zvi Assaraf, O. (2022). “Long term ecological research as a learning environment: evaluating its impact in developing the understanding of ecological systems thinking – a case study,” in Fostering Understanding of Complex Systems in Biology Education: Pedagogies, Guidelines and Insights From Classroom-based Research, eds O. Ben Zvi Assaraf and M.-C. P. J. Knippels (Cham: Springer International Publishing), 17–40. doi: 10.1007/978-3-030-98144-0_2
Dunbar, K. (2000). How scientists think in the real world: implications for science education. J. Appl. Dev. Psychol. 21, 49–58. doi: 10.1016/S0193-3973(99)00050-7
Duncan, R. G. (2007). The role of domain-specific knowledge in generative reasoning about complicated multileveled phenomena. Cogn. Instruct. 25, 271–336. doi: 10.1080/07370000701632355
Engelschalt, P., Bielik, T., Krell, M., Krüger, D., and Upmeier zu Belzen, A. (2023). Investigating pre-service science teachers' metaknowledge about the modelling process and its relation to metaknowledge about models. Int. J. Sci. Educ. 59, 1–24.
Ericsson, K. A., and Simon, H. A. (1980). Verbal reports as data. Psychol. Rev. 87, 215–251. doi: 10.1037/0033-295X.87.3.215
Field, A. (2013). Discovering Statistics Using IBM SPSS Statistics, 4th Edn. Los Angeles, CA: SAGE Publications.
Frigg, R., and Hartmann, S. (2020). “Models in science,” in The Stanford Encyclopedia of Philosophy (Spring 2020), ed E. N. Zalta (Metaphysics Research Lab; Stanford University). Available online at: https://plato.stanford.edu/archives/spr2020/entries/models-science/
Giere, R. (2009). An agent-based conception of models and scientific representation. Synthese 172:269. doi: 10.1007/s11229-009-9506-z
Godfrey-Smith, P. (2006). The strategy of model-based science. Biol. Philos. 21, 725–740. doi: 10.1007/s10539-006-9054-6
Göhner, M. F., Bielik, T., and Krell, M. (2022). Investigating the dimensions of modeling competence among preservice science teachers: meta-modeling knowledge, modeling practice, and modeling product. J. Res. Sci. Teach. 59, 1354–1387. doi: 10.1002/tea.21759
Göhner, M. F., and Krell, M. (2020). Preservice science teachers' strategies in scientific reasoning: the case of modeling. Res. Sci. Educ. 52, 395–414. doi: 10.1007/s11165-020-09945-7
Goldstone, R. L., and Wilensky, U. (2008). Promoting transfer by grounding complex systems principles. J. Learn. Sci. 17, 465–516. doi: 10.1080/10508400802394898
Gouvea, J., and Passmore, C. (2017). ‘Models of' versus ‘models for': toward an agent-based conception of modeling in the science classroom. Sci. Educ. 26, 49–63. doi: 10.1007/s11191-017-9884-4
Halloun, I. A. (2007). Mediated modeling in science education. Sci. Educ. 16, 653–697. doi: 10.1007/s11191-006-9004-3
Haskel-Ittah, M. (2022). Explanatory black boxes and mechanistic reasoning. J. Res. Sci. Teach. 60, 915–933. doi: 10.1002/tea.21817
Hillis, D. M., Heller, H. C., Hacker, S. D., Hall, D. W., and Laskowski, M. J. (2020). Life: The Science of Biology, 12th Edn. Sunderland.
Hmelo-Silver, C. E., Jordan, R., Eberbach, C., and Sinha, S. (2017). Systems learning with a conceptual representation: a quasi-experimental study. Instruct. Sci. 45, 53–72. doi: 10.1007/s11251-016-9392-y
Johnson, T. R., and Krems, J. F. (2001). Use of current explanations in multicausal abductive reasoning. Cogn. Sci. 25, 903–939. doi: 10.1207/s15516709cog2506_2
Kampourakis, K., and Niebert, K. (2018). “Explanation in biology education,” in Teaching Biology in Schools: Global Research, Issues, and Trends, eds K. Kampourakis and M. J. Reiss (CRC Press), 237–248.
Ke, L., and Schwarz, C. V. (2019). “Using epistemic considerations in teaching: fostering students' meaningful engagement in scientific modeling,” in Towards a Competence-Based View on Models and Modeling in Science Education, eds A. Upmeier zu Belzen, D. Krüger, and J. van Driel (Cham: Springer International Publishing), 181–199. doi: 10.1007/978-3-030-30255-9_11
KMK (2020). Bildungsstandards im Fach Biologie für die Allgemeine Hochschulreife. Carl Link. Available online at: www.kmk.org
Koponen, I. T. (2007). Models and modelling in physics education: a critical re-analysis of philosophical underpinnings and suggestions for revisions. Sci. Educ. 16, 751–773. doi: 10.1007/s11191-006-9000-7
Krell, M., Walzer, C., Hergert, S., and Krüger, D. (2019). Development and application of a category system to describe pre-service science teachers' activities in the process of scientific modelling. Res. Sci. Educ. 49, 1319–1345. doi: 10.1007/s11165-017-9657-8
Kuipers, T. A. F. (2004). “Inference to the best theory, rather than inference to the best explanation—kinds of abduction and induction,” in Induction and Deduction in the Sciences, ed F. Stadler (Dordrecht: Springer Netherlands), 25–51. doi: 10.1007/978-1-4020-2196-1_3
Landis, J. R., and Koch, G. G. (1977). The measurement of observer agreement for categorical data. Biometrics 33, 159–174. doi: 10.2307/2529310
Lawson, A. E. (2003). The nature and development of hypothetico-predictive argumentation with implications for science teaching. Int. J. Sci. Educ. 25, 1387–1408. doi: 10.1080/0950069032000052117
Lawson, A. E. (2010). Basic inferences of scientific reasoning, argumentation, and discovery. Sci. Educ. 94, 336–364. doi: 10.1002/sce.20357
Lehrer, R., and Schauble, L. (2015). “The development of scientific thinking,” in Handbook of Child Psychology and Developmental Science: Cognitive Processes, Vol. 2, 7th Edn (Hoboken, NJ: John Wiley and Sons, Inc.), 671–714.
Leighton, J. P. (2017). “Collecting and analyzing verbal response process data in the service of interpretive and validity arguments,” in Validation of Score Meaning for the Next Generation of Assessments, eds K. Ercikan and J. W. Pellegrino (Oxfordshire: Routledge), 25–38. doi: 10.4324/9781315708591-4
Magnani, L. (2004). Model-based and manipulative abduction in science. Found. Sci. 9, 219–247. doi: 10.1023/B:FODA.0000042841.18507.22
Meister, J., and Upmeier zu Belzen, A. (2020). Investigating students' modelling styles in the process of scientific-mathematical modelling. Sci. Educ. Rev. Lett. 2019, 8–14. doi: 10.18452/21039
Meister, S., Krell, M., Göhner, M. F., and Upmeier zu Belzen, A. (2021). Pre-service biology teachers' responses to first-hand anomalous data during modelling processes. Res. Sci. Educ. 51, 1459–1479. doi: 10.1007/s11165-020-09929-7
NGSS Lead States (2013). Next Generation Science Standards: For States, By States. Washington, DC: The National Academy Press.
Nicolaou, C. T., and Constantinou, C. P. (2014). Assessment of the modeling competence: a systematic review and synthesis of empirical research. Educ. Res. Rev. 13, 52–73. doi: 10.1016/j.edurev.2014.10.001
OECD (2008). 21st Century Skills: How Can You Prepare Students for the New Global Economy? OECD. Available online at: https://www.oecd.org/site/educeri21st/40756908.pdf
Oh, P. S. (2019). Features of modeling-based abductive reasoning as a disciplinary practice of inquiry in earth science. Sci. Educ. 28, 731–757. doi: 10.1007/s11191-019-00058-w
Oh, P. S. (2022). “Abduction in earth science education,” in Handbook of Abductive Cognition, ed L. Magnani (Cham: Springer International Publishing), 1–31. doi: 10.1007/978-3-030-68436-5_48-1
Oh, P. S., and Oh, S. J. (2011). What teachers of science need to know about models: an overview. Int. J. Sci. Educ. 33, 1109–1130. doi: 10.1080/09500693.2010.502191
Park, J. H., and Lee, K. H. (2018). How can mathematical modeling facilitate mathematical inquiries? Focusing on the abductive nature of modeling. EURASIA J. Math. Sci. Technol. Educ. 14:em1587. doi: 10.29333/ejmste/92557
Passmore, C., Gouvea, J. S., and Giere, R. (2014). “Models in science and in learning science: focusing scientific practice on sense-making,” in International Handbook of Research in History, Philosophy and Science Teaching, ed M. R. Matthews (Dordrecht: Springer), 1171–1202.
Penzlin, J., Krüger, D., and Upmeier zu Belzen, A. (2022). “Sudents' explanations about co-evolutionary phenomena with regard to organisational levels,” in 13th ERIDOB 2022 Conference (Nicosia).
Perkins, D. N., and Grotzer, T. A. (2005). Dimensions of causal understanding: the role of complex causal models in students' understanding of science. Stud. Sci. Educ. 41, 117–165. doi: 10.1080/03057260508560216
Rice, C., Rohwer, Y., and Ariew, A. (2019). Explanatory schema and the process of model building. Synthese 196, 4735–4757. doi: 10.1007/s11229-018-1686-y
Rocksén, M. (2016). The many roles of “explanation” in science education: a case study. Cult. Stud. Sci. Educ. 11, 837–868. doi: 10.1007/s11422-014-9629-5
Rohwer, Y., and Rice, C. (2016). How are models and explanations related? Erkenntnis 81, 1127–1148. doi: 10.1007/s10670-015-9788-0
Ruppert, J., Duncan, R. G., and Chinn, C. A. (2017). Disentangling the role of domain-specific knowledge in student modeling. Res. Sci. Educ. 49, 921–948. doi: 10.1007/s11165-017-9656-9
Salmon, W. C. (1990). Four Decades of Scientific Explanation. Pittsburgh: University of Pittsburgh Press.
Sandmann, A. (2014). “Lautes denken – die analyse von Denk-, Lern- und problemlöseprozessen,” in Methoden in der Naturwissenschaftsdidaktischen Forschung, eds D. Krüger, I. Parchmann, and H. Schecker (Berlin: Springer), 179–188. doi: 10.1007/978-3-642-37827-0_15
Schneeweiß, N., and Gropengießer, H. (2019). Organising levels of organisation for biology education: a systematic review of literature. Educ. Sci. 9:3. doi: 10.3390/educsci9030207
Schneeweiß, N., and Gropengießer, H. (2022). “The zoom map: explaining complex biological phenomena by drawing connections between and in levels of organization,” in Fostering Understanding of Complex Systems in Biology Education: Pedagogies, Guidelines and Insights From Classroom-Based Research, eds O. Ben Zvi Assaraf and M.-C. P. J. Knippels (Cham: Springer International Publishing), 123–149. doi: 10.1007/978-3-030-98144-0_7
Schwarz, C. V., Ke, L., Salgado, M., and Manz, E. (2022). Beyond assessing knowledge about models and modeling: moving toward expansive, meaningful, and equitable modeling practice. J. Res. Sci. Teach. 59, 1086–1096. doi: 10.1002/tea.21770
Schwichow, M., and Nehring, A. (2018). Variablenkontrolle beim experimentieren in biologie, chemie und physik: höhere kompetenzausprägungen bei der anwendung der variablenkontrollstrategie durch höheres fachwissen? Empirische Belege aus zwei Studien. Zeitsch. Didaktik Nat. 24, 217–233. doi: 10.1007/s40573-018-0085-8
Snapir, Z., Eberbach, C., Ben-Zvi-Assaraf, O., Hmelo-Silver, C., and Tripto, J. (2017). Characterising the development of the understanding of human body systems in high-school biology students – a longitudinal study. Int. J. Sci. Educ. 39, 2092–2127. doi: 10.1080/09500693.2017.1364445
Stieff, M., Scopelitis, S., Lira, M. E., and Desutter, D. (2016). Improving representational competence with concrete models. Sci. Educ. 100, 344–363. doi: 10.1002/sce.21203
Svoboda, J., and Passmore, C. (2013). The strategies of modeling in biology education. Sci. Educ. 22, 119–142. doi: 10.1007/s11191-011-9425-5
Tamir, R., Ben-Zvi Assaraf, O., and Maman, S. (2023). System-thinking progress in engineering programs: a case for broadening the roles of students. Front. Educ. 8:1138503. doi: 10.3389/feduc.2023.1138503
Upmeier zu Belzen, A., Engelschalt, P., and Krüger, D. (2021). Modeling as scientific reasoning—the role of abductive reasoning for modeling competence. Educ. Sci. 11:495. doi: 10.3390/educsci11090495
Upmeier zu Belzen, A., van Driel, J., and Krüger, D. (2019). “Introducing a framework for modeling competence,” in Towards a Competence-Based View on Models and Modeling in Science Education, eds A. Upmeier zu Belzen, D. Krüger, and J. van Driel (Cham: Springer International Publishing), 3–19. doi: 10.1007/978-3-030-30255-9_1
Verhoeff, R. P., Knippels, M.-C. P. J., Gilissen, M. G. R., and Boersma, K. T. (2018). The Theoretical nature of systems thinking. perspectives on systems thinking in biology education. Front. Educ. 3:40. doi: 10.3389/feduc.2018.00040
Keywords: reasoning, modeling, abduction, explanation, inquiry, complexity, systems thinking (ST), mechanism
Citation: Engelschalt P, Röske M, Penzlin J, Krüger D and Upmeier zu Belzen A (2023) Abductive reasoning in modeling biological phenomena as complex systems. Front. Educ. 8:1170967. doi: 10.3389/feduc.2023.1170967
Received: 21 February 2023; Accepted: 14 September 2023;
Published: 06 October 2023.
Edited by:
Laura Zangori, University of Missouri, United StatesReviewed by:
Agustín Adúriz-Bravo, University of Buenos Aires, ArgentinaCopyright © 2023 Engelschalt, Röske, Penzlin, Krüger and Upmeier zu Belzen. This is an open-access article distributed under the terms of the Creative Commons Attribution License (CC BY). The use, distribution or reproduction in other forums is permitted, provided the original author(s) and the copyright owner(s) are credited and that the original publication in this journal is cited, in accordance with accepted academic practice. No use, distribution or reproduction is permitted which does not comply with these terms.
*Correspondence: Paul Engelschalt, cGF1bC5lbmdlbHNjaGFsdEBodS1iZXJsaW4uZGU=
Disclaimer: All claims expressed in this article are solely those of the authors and do not necessarily represent those of their affiliated organizations, or those of the publisher, the editors and the reviewers. Any product that may be evaluated in this article or claim that may be made by its manufacturer is not guaranteed or endorsed by the publisher.
Research integrity at Frontiers
Learn more about the work of our research integrity team to safeguard the quality of each article we publish.