- Department of Sociology, Faculty of Social and Political Sciences, Universitätsstrasse 15, University of Innsbruck, Innsbruck, Austria
The Gender-Equality Paradox (GEP) describes the phenomenon that the gender gap in the preference for and choice of science, technology, engineering, and mathematics (STEM) majors is larger in more affluent and gender-egalitarian societies. GEP has theoretically been explained by greater economic opportunities in affluent societies for gendered self-realization, yet the literature lacks a test of this explanation on the individual level. This study tests (a) whether household wealth is associated with a greater male-favorable gender gap in student's math intentions, (b) whether this association, if any, is different in size and shape in more affluent and less affluent countries, and (c) whether household wealth can account for GEP regarding math intentions. Multilevel regression analyses of 15-year-old students' intentions to study math rather than language from 60 countries of the Programme for International Student Assessment (PISA) 2012 display that household wealth is only weakly and positively related to girls' and boys' math intentions and does not increase or decrease the gender gap in math intentions. This pattern of household wealth effects does not differ between more affluent and less affluent countries, and household wealth cannot account for GEP regarding math intentions. These findings underline that the economic need/opportunity interpretation of GEP does not hold on the micro level and requires further research into the drivers of GEP.
1. Introduction
A surprising finding from the social science literature concerns the cross-national variation in the gender gap in preference for and choice of science, technology, engineering, and mathematics (STEM) majors. The gender gap—women aspiring and choosing math and STEM fields of study less than men—is larger the more affluent and gender—equal societies are (Barinaga, 1994; Charles and Bradley, 2009; Charles, 2011, 2017; Sikora and Pokropek, 2012; Charles et al., 2014; but see McDaniel, 2016 and Richardson et al., 2020 regarding gender equality; Stoet and Geary, 2018; Breda et al., 2020). This so-called Gender-Equality Paradox (GEP; cf. Stoet and Geary, 2018) is remarkable because of greater gender equality in economic and political opportunities and rights in more developed countries and more egalitarian gender-role attitudes. GEP is next to remarkable societally concerning. GEP may raise existing gender inequalities due to the better wage and career prospects of STEM than non-STEM education (Christie and Shannon, 2001; Black et al., 2008; Organization for Economic Co-operation and Development OECD, 2017). GEP may also hamper economic growth due to shortages of STEM supplies in labor markets (OECD, 2017). In addition, GEP undermines the global trend of decreased gender segregation in educational attainment and labor market participation (Dorius and Firebaugh, 2010).
The societal affluence effect on the gender gap in STEM preferences and choices, i.e., GEP, has theoretically been explained by greater economic opportunities for gender-essentialist self-expression in more advanced societies (Charles and Bradley, 2002, 2009). So far, this economic opportunity explanation holds against rival explanations of GEP, such as the educational system and labor market features of affluent and non-affluent societies and gendered math and STEM stereotypes. These rival explanations hardly account for GEP (cf. Charles, 2017). However, Breda et al.'s (2020) study seems an exception. They have fully accounted for GEP regarding students' math intentions by stronger math gender stereotypes in more affluent societies. Yet, Breda et al.'s interpretation can be doubted since their measure of gender stereotypes is not an independent measure (it is obtained from the students themselves and concerns math attitudes, just as the dependent variable analyzed), potentially biased (students assessing the extent their parents believe that math is important for their career), and not assessed with proper multilevel analyses techniques (mostly their analyses are on the country level).1
However, whether the economic opportunity explanation is a valid explanation of GEP is itself not been well tested. The literature lacks a test of this explanation on the individual level, specifically of the effect of (parental) household affluence on the gender gap in students' STEM preferences and choices. A test on the individual level is important as the proposed mechanism in the economic opportunity theory of GEP concerns individual-level economic opportunities. The argument is that in richer contexts, people can afford more to prefer and choose studies freely, without financial pressure, and can “indulge their gendered selves” to a greater extent than in less affluent contexts (Charles and Bradley, 2009; Charles, 2011, 2017; Charles et al., 2014). Other reasons to do a test on the individual level are that the statistical power is greater than on the contextual, cross-national level and that unobserved heterogeneity is less problematic.
Therefore, this study tests the effect of household wealth on male and female math intentions. Math intentions are students' plans to pursue studies in math rather than language and have proven to be a strong predictor of future STEM vs. non-STEM study choices (Legewie and DiPrete, 2014; Breda et al., 2020). Next to testing the effect of household wealth on individual math intentions, I compare its effect across countries, using a multilevel design on more than 250,000 students in 60 countries from the Programme for International Student Assessment (PISA) 2012. I apply this cross-comparative, multilevel design first to validate household wealth effects across different settings (the scarce literature indicates instability in social origin effects); second, to assess whether household wealth can account for the macro empirical regularity of GEP, a regularity which has also been found regarding math intentions (Breda and Napp, 2019; Breda et al., 2020); and, third, to test whether the (gendered) household wealth effect varies with societal affluence (as one might hypothesize; see below). Thus, I pose the following questions: (a) is household wealth associated with a greater gender gap in math intentions in favor of boys (e.g., a more negative household wealth effect for girls than boys)?; (b) is this association different in size and shape in affluent and non-affluent countries?; (c) and can household wealth account for GEP regarding math intentions?
The article is structured as follows: first, I discuss existing studies on household wealth and math and STEM aspirations and choices; second, I derive hypotheses from the economic need/opportunity explanation on household wealth and girls' and boys' math intentions; third, I outline the data, measures, and method employed; fourth, I present the findings regarding the tests of hypotheses; fifth, and finally, I draw conclusions and discuss findings.
2. Existing studies
To my knowledge, existing studies did not directly assess the effects of household economic resources on boys' and girls' math and STEM study preferences and choices. A study by Wright et al. (2021) seems an exception. They tested the effect of family income on the choice of female-dominated majors and observed no effect of family income net of ability measures. However, this outcome measure—the share of women in a major—does not have a one-to-one relation with the STEM/non-STEM distinction. For example, there are relatively many women in the STEM field of biology.
Studies indirectly testing the effect of household economic resources on students' math and STEM preferences and choices assessed the effects of parental socio-economic status (SES) measures, such as parental education and occupational status (Ma, 2009; Charles et al., 2014; Charles, 2017; Van de Werfhorst, 2017), and composite SES measures (Codiroli McMaster, 2017). The findings are inconsistent. Parental SES sometimes decreased men's and women's STEM preferences and STEM choice probabilities (Leppel et al., 2001; Ma, 2009; Charles et al., 2014), sometimes did not affect it (Mau, 2003; Xie and Killewald, 2012; Chen and Soldner, 2014), and sometimes increased these preferences and probabilities (Charles, 2017; Van de Werfhorst, 2017). Also, parental SES sometimes decreased the gender gap in STEM study preferences and choices (Codiroli McMaster, 2017; Van de Werfhorst, 2017), sometimes did not affect it (Charles, 2017), and sometimes increased the gender gap (Leppel et al., 2001; Ma, 2009). A study by Marsh et al. (2021), finally, investigated whether a parental SES index could account for GEP, which it could not. Yet, the study focused on GEP regarding math self-concepts rather than math or STEM study preferences.
The inconsistency in the findings of parental socio-economic effects on math and STEM preferences and choices may be explained by the fact that SES is an indirect measure of household economic resources and also captures relevant non-economic household factors such as gender-role attitudes. Higher-educated families, for example, are wealthier but also hold more egalitarian gender-role attitudes (Boehnke, 2011). These two processes may have offsetting effects on offspring's math and STEM orientation: wealth may decrease girls' math/STEM preferences, and egalitarian gender-role attitudes may increase these preferences. This is why I focus on directly measuring household economic resources in this study.
3. Theoretical perspective and hypotheses
The most prominent explanation of GEP is the economic opportunity explanation, also known as the resource hypothesis (Falk and Hermle, 2018). The explanation stems from Charles et al. seminal work on cross-national patterns of horizontal gender stratification (Charles and Bradley, 2002, 2009). Charles and colleagues observed a larger gender gap in math aspirations and STEM graduation in economically more developed countries (Charles and Bradley, 2009; Charles, 2011, 2017; Charles et al., 2014). Their initial explanation of this GEP is based on varying economic opportunities across societies and omnipresent gendered-essentialist attitudes. Gender essentialism refers to the societal beliefs about the fundamentally different natures of men and women, such as that women are better at nurturing and interpersonal relationships and men physically stronger and more analytical (cf. McDaniel, 2016, p. 124). These gender-essential attitudes, which are not so much innate but a response to existing gender stereotypes and gender segregation, would be about similarity everywhere (cf. Charles et al., 2014). A later explanation also allowed these gender-essentialist attitudes to vary across contexts (Charles, 2017).
The economic opportunity explanation argues that with greater economic development, men and women have more individual-level economic opportunities to make choices in line with their gender-essentialist attitudes. In these more prosperous conditions, people will—congruent with Inglehart's theory of post-materialistic value orientations (Inglehart and Welzel, 2005)—aspire stronger to (gendered) self-realization. As a result, women have “greater latitude to indulge their gendered aspirations for less lucrative non-STEM pursuits in more affluent contexts” (Charles, 2017: 1) and will be “more willing [author: and capable] to accept the economic costs associated with the pursuit of personally attractive career paths” (Charles, 2017, p. 2). That is, women will more often opt for non-STEM educational and occupational pathways in more affluent settings. In poorer contexts, on the other hand, women have to forego gender-essentialist attitudes out of economic need and more often opt for the financially more lucrative STEM pathways. They must pursue math and STEM when getting a stable and well-paying job is a priority. In addition, in these contexts, there may be family pressure on girls to pursue STEM as a safe option in terms of career choice (Marsh et al., 2021, p. 180–181).
The implied micro-level hypothesis of this theoretical perspective is, first, that household wealth lowers girls' math intentions (H1a). Affluence ‘liberates' girls from the economic need to opt for math and STEM and enables girls to follow gender-essentialist ideals, which include non-STEM more often than STEM. Second, for boys, no effect of household wealth on math intentions might be expected (H1b). Boys from poorer families may be economically necessitated to aspire to math and STEM. Boys from richer families may aspire to STEM to a similar extent, yet for the sake of gendered self-realization. Third, given H1a and H1b, one may expect that household wealth is associated with an increased (male-favorable) gender gap in math intentions (H1c). Children from wealthier households can more “indulge their gendered selves” than children from less wealthy households.
Notwithstanding, it is questionable whether the presumed positive association of household wealth with the gender gap in math intentions is functionally the same in each context. Due to high welfare levels, most women in developed, affluent countries can afford to carry the costs associated with less lucrative non-STEM pursuits, coming from a richer or poorer background. In addition, societal affluence likely raises wages in non-STEM occupations to acceptable living standards so that career perspectives may play less of a role in choosing a study major. Only among the children from the poorest families may an economic need exist to prefer STEM pathways. In developing, less affluent countries, on the other hand, the low economic prospects of families may necessitate most men and women, except for the very rich, to opt for more lucrative math and STEM pathways. This is because more non-STEM jobs often pay below acceptable living conditions (unfortunately, there is no data on STEM vs. non-STEM wages in developing countries). For example, opting for humanities in these countries is a relatively great economic risk. Thus, I expect that in both affluent and less affluent countries, the association of household wealth with the gender gap in math intentions is positive but with a functionally different form. In developed, affluent countries, only children from the poorest households differ from the rest by a smaller gender gap in math intentions (H2a). In developing, less affluent countries, only children from the richest households differ from the rest by displaying a greater gender gap in math intentions (H2b).
A final expectation pertains to the explanation of GEP regarding math intentions. Given greater household wealth in economically more developed countries and the expected gendered effect of household wealth on math intentions (as in H1c), one may expect that household wealth can account for GEP (H3). Greater economic development will increase the gender gap in math intentions by increasing household wealth. Household wealth, therefore, is the presumed mediator of the economic development effect on the gender gap in math intentions (i.e., GEP).
4. Data, measures, method
4.1. Data
To assess the effect of household wealth on (the gender gap in) math aspirations, I use data from PISA 2012 (https://www.oecd.org/pisa/data/pisa2012database-downloadabledata.htm). PISA is an educational survey that assesses science literacy, reading comprehension, and mathematics knowledge among about half a million 15-year-old students through standardized tests every 3 years in over 60 countries. Country samples are representative of each country's population of 15-year-olds who attend educational institutions and are in grade seven and higher, and countries include developed and developing countries covering 80 percent of the world economy (OECD, 2014a). In 2012, the study focused on math competencies and interests and addressed questions about students' intentions to pursue math vs. other subjects in secondary school and beyond. These math intention measures are missing in other PISA waves. Other cross-comparative data, such as Trends in International Mathematics and Science Study (TIMSS), include appropriate measures on math and STEM preferences but comprise fewer countries and weaker measures of household wealth.
In PISA 2012, 64 countries/regions and 478,413 students participated. As to the countries and regions, I excluded Liechtenstein because of missing data on math intentions and Gross Domestic Product (GDP) and Shanghai, Macao, and Chinese Taipei because of missing data on GDP (17k students dropped). As to the individual respondents, I dropped one-third of the student sample because of the PISA questionnaire's rotational design. Questions on math intentions were addressed to about two-thirds of all PISA students, selected at random. This random selection guarantees that the sample of analysis does not differ in background characteristics from the original sample. Due to missing values on relevant independent variables, I dropped 3% of the students. Since the loss of cases is low, I do not see any danger of bias by applying listwise deletion. My final sample of analysis consists of 60 countries and 273,833 students. To account for the larger sample sizes in some countries, I used the available senate weights in PISA.
4.2. Measures
The dependent variable is the student's math intention. Math intentions are students' plans to pursue studies in math rather than language. They strongly predict future study choices regarding STEM and non-STEM (Maltese and Tai, 2011; Legewie and DiPrete, 2014; Breda et al., 2020). Math intentions are measured by responses to the question whether students are “willing to study harder in their mathematics classes than is required [which we coded as one] or study harder in their language classes than is required [coded as zero]” (introductory text to these items is: “For each pair of statements, please choose the item that best describes you”). Breda and Napp's (2019) and Breda et al.'s (2020) studies on students' math intentions, using the same PISA data employed here to analyze GEP, included four additional items. The first of these items (“intention to take additional mathematics courses after school finishes or additional language courses”) has the disadvantage that additional math courses can be taken to compensate for weaknesses in math. However, robustness analyses showed the same basic outcomes as observed for our main dependent variable (cf. Supplementary Table A1). The other three items (“intention to major in a subject that requires mathematics vs. science skills”; “intention to take as many math classes vs. science classes as possible”; “intention to pursue a career that involves a lot of math vs. a lot of science”) have the disadvantage that they do not focus on the key form of horizontal gender segregation observed in the literature, the gender gap in STEM vs. non-STEM aspirations and choices.
The main independent micro-level variable in my analysis is household wealth. This is measured by the PISA 2012 index of family wealth possessions, which is viewed as a valid proxy of household wealth (Traynor and Raykov, 2013; note that family income is not included in PISA).2 Family wealth is a summary index, standardized over all countries, constructed by the PISA team with Item Response Theory (IRT) scaling of the presence of 11 items in the household (cf. OECD, 2014b). The first three of the items are generic, i.e., not country-specific, and concern presence within the household (a room of your own, a link to the Internet, and a DVD player); the following three items are country-specific, assumed to be appropriate measures of family wealth within the country's context, and concern presence within the household (e.g., the first country-item is a microwave for Albania, air conditioning for Argentina, and a tablet device for Australia); the last five items are generic and concern frequency of an item within the household (cellular phones, televisions, computers, cars, and rooms with a bath or shower). Cronbach's alpha reliability for the index for all OECD countries within PISA 2012 is 0.62, and for all partner countries and economies, 0.74 (OECD, 2014b).3 The combination of generic and country-specific items is an advantage over using either generic or country-specific items since it allows household wealth to differ between countries (as for generic items) and within countries (as for country-specific items). Variance decomposition shows that 37% of household wealth variance is due to between-country variance and 63% due to within-country variance.
To isolate the effect of household economic resources, I control for two additional parental household variables: parental education and cultural possessions. This is important since these measures are (potentially) correlated with household wealth and students' math intentions, yet may capture non-economic parental influences such as educational aspirations, support, and gender-role views.4 Parental education refers to parents' highest achieved educational level measured by the International Standard Classification of Education (ISCED) in six categories. Cultural possession is a standardized PISA index, also constructed with IRT scaling, of cultural items in the household (classic literature, books of poetry, and works of art). I also control for household size to account for the greater number of household possessions in larger households. Household size is a by the author constructed variable from questions on whether the following types of persons usually live with the responding student: mother (including stepmother or foster mother), father (including stepfather or foster father), brother(s) (including stepbrothers), sister(s) (including stepsisters), grandparents, and others (e.g., cousin). We measure household size as a count over these six types of persons per respondent so that it is scored from 0 to 6. Alternative specifications, for example, modeling categories separately, are less useful. For example, households including grandparents and grandchildren are rare in some countries. Other potentially relevant household composition variables, such as birth order or the number of siblings, are missing in PISA 2012.
Other micro-level variables that I include in the analyses are the student's gender (women coded as one and men as zero), student's age, student's grade level (relative to a country's modal grade), and student's math test score (standardized summary measure; cf. OECD, 2014b). Gender is a focal variable. Age, grade level, and math test scores are control variables in the analyses. Math test scores are particularly important to control because they associate positively with the intention to study harder in math and also associate with parental wealth (Xie et al., 2015).
The macro-level variable that I include in the analyses is economic development. Economic development is measured with Gross Domestic Product (GDP) per capita for 2012 divided by midyear population, expressed in constant 2010 U.S. dollars. I obtained this measure from Breda et al. (2020)—who retrieved it from the World Bank (2020)—to replicate their analyses of GEP and have comparable estimates. To test GEP (as in H3), I linearly model GDP using the natural logarithm. To test whether the parental wealth effect differs between affluent and non-affluent countries (as in H2a and 2b), I dichotomized GDP. I define less affluent countries as countries belonging to the first two quintiles of GDP and affluent countries as belonging to the last three quintiles of GDP at the country level (cf. Supplementary Table A4 for a country list). This definition largely overlaps with a measure of OECD membership.5 I do not use the Human Development Index (HDI) as a societal affluence measure, as some scholars do (e.g., Charles, 2017), since this factor is less clearly related to the economic opportunity argument. HDI includes per capita income, life expectancy, and national educational levels.
Table 1 reports descriptive statistics of all variables used in the analyses. These are unstandardized. In regression analyses, I used standardized measures to compare effect sizes.
4.3. Method
I apply multilevel regression models to assess the effect of household wealth on (the gender gap in) students' math intentions. Multilevel regression models allow one to take account of the nested data structure (here: students within countries), correctly estimating parameter estimates and standard errors (Snijders and Bosker, 1999).6 The models also allow the effects of individual-level factors to vary across contexts (here, random slopes of gender and, in some additional models, also of household wealth). I use multilevel linear probability models instead of multilevel logistic models to compare parameter estimates across models. With logistic models, comparison of parameter estimates across models can be problematic (as in H3; cf. Mood, 2010). In addition, multilevel linear regressions provide less biased estimates of cross-level interactions than logistic variants (ibid.). Given that the distribution of predicted probabilities of math intentions does not exceed the 20–80% thresholds, it is safe to apply linear probability models (Cox and Snell, 1970). Robustness analyses with multilevel logistic models display the same findings regarding the effects of household wealth and GDP on men's and women's math intentions as multilevel linear regressions (Supplementary Table A6).
5. Findings
Multilevel regressions of boys' and girls' intentions to study math rather than language display evidence against the micro-level hypotheses derived from the economic opportunity/need explanation. Table 2 shows that household wealth does not lower girls' math intentions (H1a) but generally, overall 60 PISA countries increase it. Similarly, household wealth is not unrelated to boys' math intentions (H1b) but is positively associated with it.7 Still, the effects of household wealth are small: a one standard deviation change in household wealth raises math intentions for boys and girls by less than one percentage point. To compare, a one standard deviation change in math competencies increases boys' and girls' math intentions by almost 10 percentage points. Furthermore, household wealth has a smaller effect than national wealth (GDP).
The regressions in Table 2 also display that the gender gap in math intentions is unaffected by household wealth. The gender gap is, on average, eight percentage points in favor of boys (compare the constants for boys and girls in Table 2, which are the average math intentions). This gap is neither in nor decreased by household wealth, as evidenced by the absence of a statistically significant difference in the coefficient of household wealth between boys and girls (see the last column of Table 2 and the interaction model in the pooled data, Model 2 of Table 3). This finding also goes against our hypotheses (H1c).
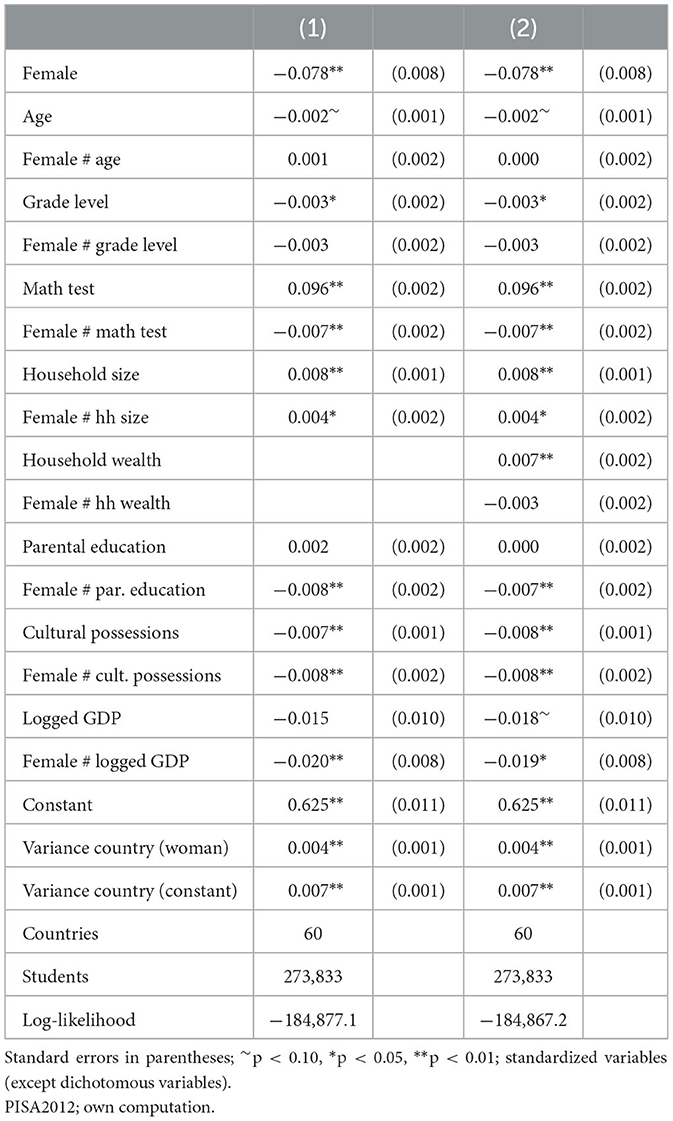
Table 3. Multilevel (linear probability) regressions of students' math intentions: interactive effects by gender.
Interestingly, parental education and possession of cultural resources in the student's household raise the gender gap in math intentions. Parental education decreases girls' math intentions and does not affect boys' math intentions (gender difference in coefficient is significant). Cultural possessions decrease girls' math intentions more than they decrease boys' math intentions. The negative effect of parental education on girls' math intention surprises given the generally more egalitarian gender-role attitudes in higher-educated families.
The household wealth effects for boys and girls can also be seen in Figures 1A, B. Figure 1A plots estimated girls' and boys' math intentions by household wealth deciles, where higher deciles are coded to be wealthier (average marginal effects, where we controlled for all other variables). Figure 1B plots the estimated gender gap by household wealth decile (average marginal effect of gender, controlling all other variables). Girls' and boys' math intentions only weakly increase over household wealth deciles, and they do so to the same extent (Figure 1A), so the gender gap in math intentions hardly changes over wealth deciles (Figure 1B).
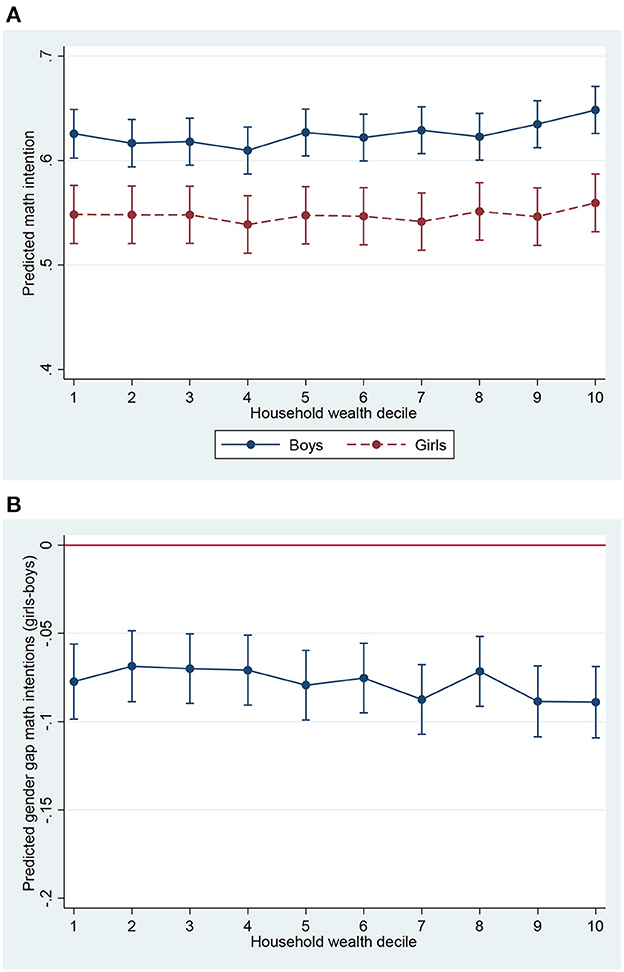
Figure 1. (A) Predictive margins of students' math intentions by household wealth decile and gender (with 95% confidence intervals). (B) Predicted gender gap (girls minus boys) in students' math intentions by household wealth decile (with 95% confidence intervals).
Figures 2A–D display that this finding, the insignificant effect of household wealth on the gender gap in math intentions, also shows separately for less affluent and affluent countries. In both country types, the gender gap in math intention hardly changes with household wealth (this is also shown in multilevel analyses; cf. Supplementary Table A7). The pattern in affluent countries is least in line with our hypotheses (Figures 2A, B). We expected children from the poorest households to stand out with a smaller gender gap in math intentions (H2a). Yet, we find these children to show a relatively larger gender gap (a 15 percentage point male-favorable gap for the first, lowest decile vs. a 10 percentage point gap for other deciles). This larger gap is due to weaker math intentions among girls and stronger math intentions among boys from the poorest wealth decile. The pattern in less affluent countries is more in line with our hypotheses (Figures 2C, D). We expected that only children from the richest households would differ from others by displaying a greater gender gap in math intentions (H2b). We tend to find this. In less affluent countries, girls from the richest wealth deciles (9 and 10) prefer math less often than girls from other deciles, whereas boys from the richest deciles do not differ from boys from other deciles. This makes for a larger gender gap in the wealthiest households. Yet, the difference in the gap is not large, a 6 percentage point gap in the richest deciles and a 4 to 5 percentage point gap in other deciles.
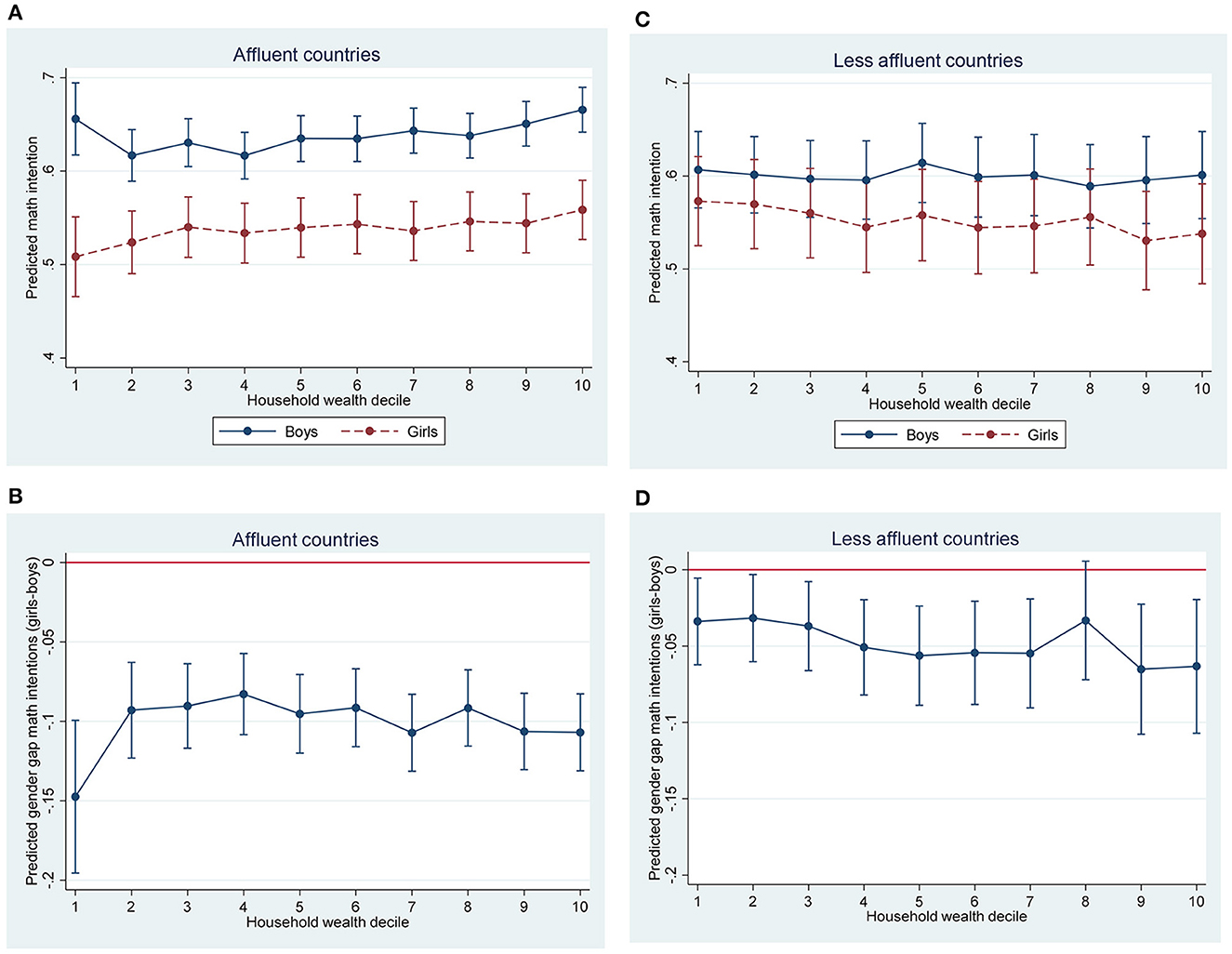
Figure 2. (A) Predictive margins of students' math intentions by household wealth decile and gender, for affluent countries (with 95% confidence intervals). (B) Predicted gender gap (girls minus boys) in students' math intentions by household wealth decile for affluent countries (with 95% confidence intervals). (C) Predictive margins of students' math intentions by household wealth decile and gender, for less affluent countries (with 95% confidence intervals). (D) Predicted gender gap (girls minus boys) in students' math intentions by household wealth decile for less affluent countries (with 95% confidence intervals).
Note that for less affluent countries, the wealth effects for girls and boys separately also seem more in line with our predictions than for affluent countries. There appears to be a negative wealth effect for girls (in line with H1a) and no effect for boys (in line with H1b) in less affluent countries (Figure 2C). Still, the wealth effect for girls from non-affluent countries is small and does not differ significantly from that of boys (cf. Supplementary Table A7).
Table 3, finally, displays to what extent household wealth can account for GEP regarding math intentions. We, therefore, estimated regressions pooled for girls and boys and interacted with all variables, individual-level and macro-level (GDP), by gender. We do this one time without household wealth and its interaction with gender (Model 1) and one time with these covariates (Model 2). Model 1 displays the Gender-Equality Paradox regarding math intentions: the higher (logged) the GDP, the greater the gender gap in math intentions at the disadvantage of girls. One standard deviation in logged GDP increases the gender gap, which is eight percentage points in general, by two percentage points (b = −0.020). GEP regarding math intentions was also shown by Breda et al. (2020) with the PISA 2012 data with a different outcome measure and method. Model 2 displays that accounting for household wealth and the interaction of wealth by gender hardly explains GEP, as the interactive effect of being female by logged GDP only slightly decreases (from b = −0.020 in M1 to b = −0.019 in M2). This finding rejects our hypothesis (H3). The finding is due to the insignificant interaction effect of household wealth with the gender gap in math intentions (i.e., the “female x household wealth” parameter). Although household wealth is higher in more affluent countries, if household wealth is not associated with a greater gender gap in math intentions, it cannot explain why in more affluent countries, the gender gap in these math intentions is generally higher. Additional analyses reveal that parental education and household cultural possessions neither attribute for GEP: although these parental characteristics are associated with a greater gender gap in math intentions (cf. Table 3), they do not associate strongly with societal affluence (GDP), so they cannot account for GEP (cf. Supplementary Table A8).
6. Conclusion and discussion
The Gender-Equality-Paradox (GEP) is the surprising macro-level empirical regularity of a larger male-favorable gender gap in math and STEM preferences and choices in more affluent and gender-equal countries. GEP has theoretically been explained by greater economic opportunities for gender-essentialist self-expression in more advanced societies, yet this explanation lacks a proper test on the individual level. This study fills this gap by testing whether household-level wealth is associated with greater gender differences in math preferences and whether household wealth can account for GEP. Multilevel regressions of 15-year-old students' intentions to study math rather than language in 60 countries from PISA 2012 display that the economic opportunity/need mechanism does not hold at the micro level. Household wealth is not associated with a greater gender gap in math intentions: it increases girls' and boys' math intentions slightly and does so to the same extent. Household wealth can, therefore, not account for the macro-level regularity of GEP regarding math intentions, although household wealth is clearly higher in affluent than non-affluent countries.
These findings largely align with Wright et al.'s (2021) finding of a non-significant family income effect on the choice of female-dominated majors and go against the economic opportunity/need for an explanation of GEP (Charles and Bradley, 2009; Charles, 2011, 2017; Charles et al., 2014). According to this explanation, more economic resources provide opportunities for gendered self-realization along gender-stereotypical lines, and weaker economic resources create a need to opt for and prefer more lucrative STEM and math pathways. However, we do not find such gendered patterns at the individual level. Only in less affluent countries do women seem to decreasingly aspire for math with increasing household wealth, while men in these countries are unaffected. Yet, even there, household wealth is not significantly associated with the gender gap in math intentions.
These findings raise two important questions and suggest several lines of future research. The first question is why household wealth does not have its predicted effects on math intentions, in particular, why it does not lower girls' math intentions but slightly raises them. The first answer may lie in the investigated outcome measure. Focusing on students' preferences to study math or language, analogous to the divide between STEM and non-STEM, may hide interesting economic resource/need effects. Some non-STEM studies, such as business studies and economics, do not require strong math abilities but are still attractive in terms of wage and career prospects. Children from poorer households may disproportionally aspire to such studies, a pattern we cannot study with our data. This pleas to study household economic resource effects in future analyses in greater detail by looking at specific major choices, as Codiroli McMaster (2017) did for the U.K. using an indirect SES measure. An additional reason to focus on study choices is that these choices, other than math preferences, involve costs, possibly altering economic resource effects. The second answer to why household wealth may not have its predicted effects is that parental economic resources may have other than its assumed functions. Parental economic resources may not only affect students' incentives to prefer and choose certain studies but also may serve to support children. High-income parents, for example, send their children more often to schools with stronger support for math and science (Xie et al., 2015). This may raise students' math and STEM interest. Future studies, therefore, may investigate the distinct pathways by which parents of higher social origin influence offspring's math and STEM preferences.
The second question regarding this study's outcomes is how to explain GEP when economic opportunity at the individual level is not the responsible mechanism. As stated before, alternative explanations of GEP exist, such as the educational system and labor market features of affluent and non-affluent societies and gendered math and STEM stereotypes. Yet, these alternative explanations can neither account for GEP (cf. Charles, 2017). A potential other explanation may be the size of the welfare state (Mandel and Semyonov, 2006). A large welfare state may have pushed women more into non-STEM and men into STEM pathways through its combination of extensive educational choice opportunities, a large public sector allowing to combine work and care more easily, and high welfare support. This could be investigated in future research. Another explanation sees GEP more critically. A recent study by Fors Connolly et al. (2020) showed that GEP regarding human values exists cross-sectionally, yet not if modeled longitudinally applying country-fixed effects. This finding casts doubts on causal interpretations of GEP, including GEP regarding math and STEM study preferences and choices (cf. Blasko et al., 2018; but see Charles, 2017). GEP may be an artifact of a factor both influencing societal advancement and gender differences in study preferences. A candidate factor may be a country's STEM sector size, as it is both associated with a larger gender gap in STEM preferences (cf. Blasko et al., 2018) and economic growth. Here too, there is an urge for further research.
Data availability statement
Publicly available datasets were analyzed in this study. This data can be found here: https://www.oecd.org/pisa/pisaproducts/pisa2012database-downloadabledata.htm.
Author contributions
The author confirms being the sole contributor of this work and has approved it for publication.
Funding
This publication was funded by the publishing fund of the University of Innsbruck.
Conflict of interest
The author declares that the research was conducted in the absence of any commercial or financial relationships that could be construed as a potential conflict of interest.
Publisher's note
All claims expressed in this article are solely those of the authors and do not necessarily represent those of their affiliated organizations, or those of the publisher, the editors and the reviewers. Any product that may be evaluated in this article, or claim that may be made by its manufacturer, is not guaranteed or endorsed by the publisher.
Supplementary material
The Supplementary Material for this article can be found online at: https://www.frontiersin.org/articles/10.3389/feduc.2023.1155492/full#supplementary-material
Footnotes
1. ^In additional analyses, Breda et al. (2020) ran student-level regressions controlling for observable student characteristics and noted the same findings as aggregate-level analyses. However, these student-level analyses did not account for the country variation in the gender effect (“random slope”) so that the conclusion on GEP—which involves the interactive effect of gender by economic development—and its explanation is potentially biased (cf. Heisig and Schaeffer, 2019).
2. ^That family wealth possessions are an appropriate measure of household wealth is also evidenced by the high correlation at the country level between average household wealth and logged GDP (r =0.85; N = 60; p < 0.01).
3. ^These are the alpha values excluding the item DVD player and the three country-specific items because these items had different meaning for different countries.
4. ^When parental education, household cultural possessions, and household size were controlled for, household wealth effects on math intentions were larger. Cultural possessions was the responsible variable for this change. Including cultural possessions increased the household wealth effect from an insignificant to a positive effect for girls, with the effect of cultural possessions being negative in itself. Yet, the change in the coefficient is small and for boys even smaller (cf. Supplementary Tables A2, A3).
5. ^Four of the 24 less affluent (Q1-2) countries are OECD countries (Hungary, Mexico, Poland, and Turkey). Six of 36 affluent countries (Q3-5) countries are non-OECD countries (Croatia, Hongkong, Lithuania, Singapore, Qatar, and United Arab Emirates).
6. ^Robustness analyses of three-level models (students nested within schools and schools within countries) displayed the same study outcomes (cf. Supplementary Table A5). We checked this to account of the PISA sampling procedure, where schools where sampled within countries and students within schools (OECD, 2014a). Given this outcome and the fact that we do not have school-level hypotheses, we proceeded with the more parsimonious two-level models (students nested within countries).
7. ^Additional, unreported country-specific regressions revealed in 4 of 60 countries a negative household wealth effect on girls' math intentions. In eight countries, the effect is positive and in 48 countries, non-significant. For boys, we found in three countries a negative household wealth effect, in 13 countries a positive effect, and in 44 countries a non-significant effect. The household wealth effects for girls and boys vary significantly across countries, as assessed with a multilevel model including a random slope for household wealth (findings not reported).
References
Barinaga, M. (1994). Surprises across the cultural divide. Science. 263:1468–1472. doi: 10.1126/science.263.5152.1468
Black, D. A., Haviland, A. M., Sanders, S. G., and Taylor, L. J. (2008). Gender wage disparities among the highly educated. J. Human Res. 43, 630–659. doi: 10.1353/jhr.2008.0030
Blasko, Z., Pokropek, A., and Sikora, J. (2018). Science Career Plans of Adolecents: Patterns, Trends and Gender Divides (JRC Science for Policy Report EUR 28878 EN). Luxembourg: Publications Office of the European Union. doi: 10.2760/251627
Boehnke, M. (2011). Gender role attitudes around the globe: egalitarian vs. traditional views. Asian J. Social Sci. 39, 57–74. doi: 10.1163/156853111X554438
Breda, T., Jouini, E., Napp, C., and Thebault, G. (2020). Gender stereotypes can explain the gender-equality paradox. Proc. Nat. Acad. Sci. 117, 31063–31069. doi: 10.1073/pnas.2008704117
Breda, T., and Napp, C. (2019). Girls' comparative advantage in reading can largely explain the gender gap in math-related fields. Proc. Nat. Acad. Sci. 116, 15435–15440. doi: 10.1073/pnas.1905779116
Charles, M. (2017). Venus, mars, and math: gender, societal affluence, and eighth graders' aspirations for STEM. Socius. 3, 1–16. doi: 10.1177/2378023117697179
Charles, M., and Bradley, K. (2002). Equal but separate? A cross-national study of sex segregation in higher education. Am. Sociol. Rev. 67, 573–599. doi: 10.2307/3088946
Charles, M., and Bradley, K. (2009). Indulging our gendered selves? Sex segregation by field of study in 44 countries. Am. J. Sociol. 114:924–976. doi: 10.1086/595942
Charles, M., Harr, B., Cech, E., and Hendley, A. (2014). Who likes math where? Gender differences in eighthgraders' attitudes around the world. Int. Stud. Sociol. 24, 85–112. doi: 10.1080/09620214.2014.895140
Chen, X., and Soldner, M. (2014). STEM Attrition: College Students' Paths Into and Out of STEM Fields. Washington, DC: Natl. Cent. Educ. Stat. Available online at: https://nces.ed.gov/pubs2014/2014001rev.pdf
Christie, P., and Shannon, M. (2001). Educational attainment and the gender wage gap: evidence from the 1986 and 1991 Canadian Censuses. Econ. Educ. Rev. 20, 165–180. doi: 10.1016/S0272-7757(99)00058-8
Codiroli McMaster, N. (2017). Who studies STEM subjects at A level and degree in England? An investigation into the intersections between students' family background, gender and ethnicity in determining choice. Br. Educ. Res. J. 43, 528–553. doi: 10.1002/berj.3270
Dorius, S., and Firebaugh, G. (2010). Trends in global gender inequality. Social Forces 88, 1941–1968. doi: 10.1353/sof.2010.0040
Falk, A., and Hermle, J. (2018). Relationship of gender differences in preferences to economic development and gender equality. Science. 362, eaas9899. doi: 10.1126/science.aas9899
Fors Connolly, F., Goossen, M., and Hjerm, M. (2020). Does gender equality cause gender differences in values? Reassessing the gender-equality-personality paradox. Sex Roles. 83, 101–113. doi: 10.1007/s11199-019-01097-x
Heisig, J. P., and Schaeffer, M. (2019). Why you should always include a random slope for the lower-level variable involved in a cross-level interaction. Eur. Sociol. Rev. 35, 258–279. doi: 10.1093/esr/jcy053
Inglehart, R. F., and Welzel, C. (2005). Modernization, Cultural Change, and Democracy: The Human Development Sequence. New York: Cambridge University Press.
Legewie, J., and DiPrete, T. A. (2014). Pathways to science and engineering bachelor's degrees for men and women. Sociological Science 1:41–48. doi: 10.15195/v1.a4
Leppel, K., Williams, M. L., and Waldauer, C. (2001). The impact of parental occupation and socio-economic status on choice of college major. J. Fam. Econ. Issues 22 (4):373–394. doi: 10.1023/A:1012716828901
Ma, Y. (2009). Family socio-economic status, parental involvement and college major choices—gender, race/ethnicity, and nativity patterns. Sociol. Persp. 52, 211–234. doi: 10.1525/sop.2009.52.2.211
Maltese, A. V., and Tai, R. H. (2011). Pipeline persistence: examining the association of educational experiences with earned degrees in STEM among U.S. students. Sci. Educ. 95, 877–907. doi: 10.1002/sce.20441
Mandel, H., and Semyonov, M. (2006). A welfare state paradox: state interventions and women's employment opportunities in 22 countries. Am. J. Sociol. 111, 1910–1949. doi: 10.1086/499912
Marsh, H. W., Parker, P. D., Guo, J., Basarkod, G., Niepel, C., and Van Zanden, B. (2021). Illusory gender-equality paradox, math self-concept, and frame-of-reference effects: new integrative explanations for multiple paradoxes. J. Pers. Soc. Psychol. 121, 168–183. doi: 10.1037/pspp0000306
Mau, W. C. (2003). Factors that influence persistence in science and engineering career aspirations. Career Dev. Q. 51, 234–243. doi: 10.1002/j.2161-0045.2003.tb00604.x
McDaniel, A. E. (2016). The role of cultural contexts in explaining cross-national gender gaps in STEM expectations. Eur. Sociol. Rev. 32, 122–133. doi: 10.1093/esr/jcv078
Mood, C. (2010). Logistic regression: why we cannot do what we think we can do, and what we can do about it. Eur. Sociol. Rev. 26, 67–82. doi: 10.1093/esr/jcp006
OECD (2014b). PISA 2012 Technical Report: Scaling Procedures and Construct Validation of Context Questionnaire Data. Paris: PISA, OECD Publishing
OECD (2017). Education at a Glance 2017: OECD Indicators. Paris: PISA, OECD Publishing. Available online at: https://www.oecd-ilibrary.org/education/education-at-a-glance-2017_eag-2017-en
Richardson, S. S., Reiches, M. W., Bruch, J., Boulicault, M., Noll, N. E., and Shattuck-Heidorn, H. (2020). is there a gender-equality paradox in science, technology, engineering, and math (STEM)? commentary on the study by stoet and geary (2018). Psychol. Sci. 31, 338–341. doi: 10.1177/0956797619872762
Sikora, J., and Pokropek, A. (2012). Gender segregation of adolescent science career plans in 50 countries. Sci. Educ. 96, 234–264. doi: 10.1002/sce.20479
Snijders, T. A. B., and Bosker, R. J. (1999). Multilevel Analysis: An Introduction to Basic and Advanced Multilevel Modeling. Thousand Oaks: Sage Publications.
Stoet, G., and Geary, D. C. (2018). The gender-equality paradox in science, technology, engineering, and mathematics education. Psychol. Sci. 29, 581–593. doi: 10.1177/0956797617741719
Traynor, A., and Raykov, T. (2013). Household possessions indices as wealth measures: a validity evaluation. Comp. Educ. Rev. 57, 662–688. doi: 10.1086/671423
Van de Werfhorst, H. G. (2017). Gender segregation across fields of study in post-secondary education: trends and social differentials. Eur. Sociol. Rev. 33, 449–464. doi: 10.1093/esr/jcx040
World Bank (2020). Available online at: https://data.worldbank.org/indicator/NY.GDP.PCAP.KD
Wright, A. L., Roscigno, V. J., and Quadlin, N. (2021). First-generation students, college majors and gendered pathways. Sociol. Q. 64, 67–90. doi: 10.1080/00380253.2021.1989991
Xie, Y., Fang, M., and Shauman, K. STEM education. (2015) Annu. Rev. Sociol. 41, 331–57. doi: 10.1146/annurev-soc-071312-145659
Keywords: STEM, math, gender, household wealth, economic development
Citation: Uunk W (2023) Does the gender-equality paradox hold on the micro level? An assessment of the effect of household wealth on gendered math intentions for 60 countries. Front. Educ. 8:1155492. doi: 10.3389/feduc.2023.1155492
Received: 31 January 2023; Accepted: 30 May 2023;
Published: 22 June 2023.
Edited by:
Nora Müller, GESIS Leibniz Institute for the Social Sciences, GermanyReviewed by:
Davide Gritti, University of Trento, ItalyUlrike Schwabe, German Centre for Higher Education Research and Science Studies (DZHW), Germany
Copyright © 2023 Uunk. This is an open-access article distributed under the terms of the Creative Commons Attribution License (CC BY). The use, distribution or reproduction in other forums is permitted, provided the original author(s) and the copyright owner(s) are credited and that the original publication in this journal is cited, in accordance with accepted academic practice. No use, distribution or reproduction is permitted which does not comply with these terms.
*Correspondence: Wilfred Uunk, V2lsZnJlZC5VdW5rJiN4MDAwNDA7dWliay5hYy5hdA==