- 1Center for Innovative Pharmacy Education and Research, UNC Eshelman School of Pharmacy, University of North Carolina, Chapel Hill, NC, United States
- 2Faculty of Pharmacy and Pharmaceutical Sciences, Monash University, Melbourne, VIC, Australia
Introduction: Core concepts are foundational, discipline-based ideas considered necessary for students to learn, remember, understand, and apply. To evaluate the extent to which a concept is “core,” experts often rate concepts using various criteria, such as importance, complexity, and timeliness. However, there is a lack of validity evidence for core concepts criteria.
Methods: Using a three-facet Many-Facet Rasch Measurement (MFRM) model, we analyzed 7,558 ratings provided by 21 experts/raters for 74 pharmacology core concepts using five criteria measured on a five-point scale.
Results: All of the criteria had Infit or Outfit MnSq values within the expected range (0.5 < MnSq < 1.7), suggesting the criteria contained an acceptable amount of variability; a reliability index of approximately 1.00 suggested that the criteria were reliably separated with a high degree of confidence. The rating scale Outfit MnSq statistics also fell within the 0.5–1.7 model fit limits; the “average measure” and “Rasch-Andrich thresholds” increased in magnitude as the rating scale categories increased, suggesting that core concepts with higher ratings were in fact meeting the criteria more convincingly than those with lower ratings. Adjusting expert ratings using the MFRM facets (e.g., rater severity) resulted in reorganization of core concepts rankings.
Conclusion: This paper is a novel contribution to core concepts research and is intended to inform other disciplines seeking to develop, implement, and refine core concepts within the biomedical sciences and beyond.
1. Introduction
Core concepts are foundational ideas that experts agree are critical for all students in their discipline to learn, remember, understand, and apply (Libarkin, 2008). Over the past three decades, many disciplines have demonstrated the utility of reaching consensus on the core concepts of their discipline, and identifying ways to assess student attainment of those concepts. Research on core concepts has led to the adoption of new evidence-based approaches to undergraduate teaching and assessment in numerous disciplines, including biology, chemistry, genetics, physics, and physiology (e.g., Hestenes et al., 1992; Libarkin, 2008; McFarland et al., 2017). Examples of research-based core concepts include gravity in physics (Hestenes et al., 1992), homeostasis in physiology (Michael et al., 2017), and drug absorption in pharmacology (White et al., 2021). In all these disciplines, core concept research and development began with the identification of a consensus list of core concepts. To this point, however, there has been no validity evidence published for the criteria used to identify and evaluate the suitability of potential core concepts.
Over the past 30 years, various methodologies have been employed to identify core concepts in higher education. Most researchers have elicited opinions of individual disciplinary experts through interviews, surveys, or Delphi methods (e.g., Boneau, 1990; Landrum, 1993; Brewer and Smith, 2011; Parekh et al., 2018). Other researchers have extracted core concepts from textbooks through page-by-page expert analysis (Zechmeister and Zechmeister, 2000) or data-mining techniques (Foster et al., 2012). Some have used multiple methods, such as combining expert input and data-mining techniques (Landrum, 1993; White et al., 2022).
Whatever methods are used, identifying a long list of potential/candidate/provisional core concepts is only a first step in the process. Next, disciplinary experts need to apply criteria to determine which of the concepts are sufficiently “core” to make the final short list. Researchers have used varying criteria and rating scales to evaluate the extent to which a concept is core. Boneau (1990), for example, asked experts to rate psychology terms on a five-point scale, with the highest rating reserved for those terms that “every psychology baccalaureate should be able to discuss and relate to other terms.” Three years later, Landrum (1993) refined this criterion with a four-point scale focused on importance, ranging from 1—unimportant to 4—very important. Parekh et al. (2018) used three criteria—importance, difficulty, and timelessness—each rated on a 10-point scale. For example, a 10 for importance was described as “Absolutely essential; leaving this topic out would be egregious, and topic is appropriate for the target,” a 10 for difficulty was described as “Few, if any, students will have mastered this topic after the target course or curriculum” and a 10 for timelessness was described as “Foundational and highly relevant across essentially all technologies throughout the foreseeable future.”
The judgment of disciplinary experts is critical in the process of core concept identification. However, input from experts can be subject to rater bias, defined as the conscious or unconscious tendencies that influence the rating process. Conscious or unconscious tendencies that contribute to rater bias are construct-irrelevant; however conscious tendencies could also contribute to accurate ratings. There are more than 40 known types of rater errors that result in bias, including assimilation effect (i.e., intentionally providing ratings that likely will be similar to other raters to avoid appearing extreme), fatigue (i.e., providing a questionable rating as a result of feeling tired), hurriedness (i.e., providing ratings that are influenced by one’s desire to quickly complete the task), and severity (i.e., providing ratings that are unduly harsh or critical; Royal, 2018). Using clearly defined criteria and scale-point anchors in the rating process can mitigate this bias, decreasing the likelihood of construct-irrelevant variance (i.e., measurement inflation or deflation due to uncontrolled or systematic measurement error, or “noise”).
To date, research explicating construct-irrelevant variance in core concept ratings—as well as potentially adjusting for its effects—remains unexplored. Validating the criteria that characterize core concepts in this way is critical for advancing the methodology of core concepts research for higher education. As such, the purpose of this study was to explore our core concepts rating criteria, specifically examining how raters and criteria influenced the ratings of core concepts, and how the rating scale performed. While data used in this study are drawn from core concept research in pharmacology—defined as the science of drugs or medicines and their interactions with biological systems—the analyses employed could be applicable to any discipline researching core concepts. This paper is the first of its kind in core concepts research and is intended to inform other disciplines seeking to develop, implement, and refine core concepts within the biomedical sciences and beyond.
2. Methods
2.1. Development of criteria
In an earlier study involving pharmacology educators, a literature review was used to identify criteria that could be used to distinguish core concepts from other concepts or terms (White et al., 2021). Five criteria were drawn from multiple disciplines and further refined as follows: Fundamental—foundational, essential to learn and understand the discipline and representing the notion that all students who have taken a course in the discipline should understand the concept (Boneau, 1990); Useful—can be employed to solve problems and interpret new scenarios in the discipline (Harlen, 2010; Michael et al., 2017); Enduring—likely to remain unchanged over generations; (Parekh et al., 2018; Tweedie et al., 2020); Challenging—difficult for students to learn (Parekh et al., 2018); and, Complex—made up of many underlying facts and sub-concepts (Michael et al., 2017).
2.2. Data collection
A starting list of 74 pharmacology concepts were identified using a combination of text mining and expert survey (White et al., 2022). Initially, 590 terms were produced by survey of 201 international pharmacology experts, and a further 100 terms were produced via text mining. These 690 terms were consolidated to a list of 74 candidate core concepts after removal of duplicates and lemmatization (i.e., aggregating close synonyms) by the researchers. Participants were identified and invited via email by the research team to participate based on their expertise in pharmacology. Participants consisted of 12 women and nine men from 15 countries across six continents: Australia, Brazil, Canada, China, Colombia, India, Ireland, Japan, Lebanon, Malta, Nigeria, Qatar, Sweden, the United States and the United Kingdom. Ten participants reported a teaching qualification at Graduate Certificate or higher level with pharmacology teaching experience ranging from 2 to 41 years (median 15 years).
Participants attended an online session in which they received information about the study, including a workshop training session on core concepts, including practice applying the five criteria that would be used to evaluate them: challenging, complex, enduring, fundamental, and useful. The participants then worked individually to rate each concept using the five criteria. Each criterion was measured on a scale from 1—not at all to 5—extremely. For example, participants were asked to rate the extent to which the core concept “drug absorption” was challenging, the extent to which it was complex, the extent to which it was enduring, and so on. Twenty-one participants rated the 74 concepts using the five criteria, for a total of 7,770 possible ratings. Data were collected via submission of individual files to the research team. Approximately 3% of the ratings were missing, for a total of 7,558 ratings in the dataset. The research was conducted under the approved protocol #31379 of the Monash University Ethics in Human Research Committee.
2.3. Data analysis
Variance in core concept ratings represents the dispersion or spread of the ratings. Understanding sources of variance in core concept ratings, such as rater severity and concept difficulty, can be handled by a number of statistical approaches. Many-Facet Rasch Measurement (MFRM) models can identify and adjust ratings based on the influence of various facets, such as rater bias (i.e., rater severity or leniency). MFRM calculates location estimates that are adjusted for variations in the location of other facets and provides a “fair average” score that adjusts for the facets in the model (Linacre, 1989). This statistical approach can be used to examine the reliability of rated assessments and quantify the amount of error caused by sources of variation, such as raters, criteria, and concepts. In health professions education, for example, researchers have used the MFRM to estimate rater severity in admissions interviews and standardized assessments (e.g., Iramaneerat et al., 2008; Roberts et al., 2010; Zeeman et al., 2017; Malau-Aduli et al., 2019).
In this study, a three-facet MFRM analysis was conducted to determine rater severity, criterion difficulty, and core concept suitability. Facets Version 3.71.4 (Beaverton, Oregon) was used to analyze the three facets simultaneously and independently so that they could be calibrated onto a single logit scale. MFRM was used to describe the severity of each rater, difficulty of each criterion, and suitability of each concept; it also adjusted ratings based on these facets to provide a more accurate reflection of core concept suitability.
Facets software provides mean-square (MnSq) error statistics to describe the degree to which each rater, concept, and criterion fit within the MFRM (i.e., whether the ratings have been confounded by construct-irrelevant factors; Eckes, 2011). These fit statistics are either unweighted Outfit MnSq scores (i.e., a measure sensitive to outliers) or weighted Infit MnSq scores (i.e., less sensitive to outliers). MnSq values greater than 1 indicate an unexpected level of variability; MnSq equal to 1 indicates the facet fit exactly as expected in the MFRM; MnSq less than 1 represents less variability than expected (Linacre, 1995). When MnSq values are greater than 2.0 they can disrupt the MFRM, introducing excessive variability, while MnSq values less than 0.5 are often considered to represent too little variability but do not destabilize the model. MnSq values within 1.7 and 0.5 are considered acceptable, as recommended by Bond and Fox (2013) for clinical observation. It may be worth noting that recommendations for critical MnSq values have been developed and widely adopted from practical experience by many researchers, and may be limited in their discussion of the Rasch fit statistics (e.g., Wolfe, 2013; Seol, 2016).
After the initial MFRM model was run, MnSq values were examined visually by the researcher for each concept. Due to the potential for values greater than 2.0 to destabilize the model, concepts with Infit or Outfit MnSq values of this magnitude were explored for removal from the analysis. In this analysis, the MnSq values fell below 2.0; as such, no ratings were removed, leaving a total of 7,558 data points in the final analysis. In addition, separation and reliability statistics were examined. Low item separation, for example, (<3, item reliability <0.9) may indicate that the sample is not large enough to confirm the construct validity of the instrument while low person separation (<2, person reliability <0.8) may imply that the instrument was not sensitive enough to distinguish between high and low performing concepts (Linacre, 2023).
3. Results
In the three-facet MFRM of all 7,558 ratings, the mean and sample standard deviation of the standardized residuals of all observations were 0.01 and 1.00, respectively. Table 1 summarizes MFRM statistics for core concepts, raters, and criteria in terms of mean, standard error, infit, outfit, chi-square value, and separation statistics. Rasch measures from the MFRM accounted for 35% of total variance in the core concept ratings, leaving 65% of variance unaccounted for by the model.
While rating scales have certain psychometric and practical advantages, they can also be subject to certain errors (e.g., rater bias, misuse, and misinterpretation). Several essential guidelines should be met for a well-functioning rating scale (Linacre, 2003). First, there should be a minimum of 10 observations for each scale category. In this study, observations ranged from 387 to 2,346; however it should be noted that these observations were not distributed regularly across categories (Table 2). Second, average measures should advance monotonically with category as average measures that are disordered or very close together suggest that those points on the rating scale should be collapsed. In this study, the “average measure” and “Rasch-Andrich thresholds” (also called step calibrations) increased in magnitude as the rating scale categories increased (e.g., average measure −0.25 for 1, to 1.34 for 5), suggesting that core concepts with higher ratings were in fact meeting the criteria more convincingly than those with lower ratings. Fourth, the Outfit MnSq statistics should not exceed 2.0. In this study, the MnSq statistics ranged from 0.9 to 1.2, suggesting that each of the scale categories functioned as intended.
Criterion difficulty accounted for 15% of the variance. Challenging and Complex were identified as the most difficult criteria (i.e., the least likely criteria to receive a rating of 5) while Useful, Fundamental, and Enduring were identified as the least difficult (i.e., the most likely criteria to receive a rating of 5). None of the five core concept criteria had Infit or Outfit MnSq values greater than 1.7 or less than 0.5 (Table 3), which suggested that the criteria contained an acceptable amount of variability. The overall Infit mean for the criteria was 1.05 (range 0.85–1.24) and the overall Outfit mean was 1.00 (range 0.86–1.18). High separation and a reliability index of approximately 1.00 suggested that the criteria were reliably separated with a high degree of confidence (p < 0.001). In other words, there is a high probability that criteria estimated with high ratings actually do have higher ratings than criteria estimated with low ratings.
Concept suitability accounted for 8% of the variance. None of the 74 core concepts had Infit or Outfit MnSq values greater than 1.7 or less than 0.5, which suggested that the core concepts contained an acceptable amount of variability. High separation and a reliability index of 0.92 suggested the concepts were reliably separated with a high degree of confidence (p < 0.001). In other words, there is a high probability that core concepts estimated with high ratings actually do rank higher than concepts estimated with low ratings. MFRM fair averages, which are adjusted for the facets entered into the model (e.g., rater bias), resulted in reorganization of core concepts rankings (Table 4). Pharmacodynamics, for example, was the highest rated core concept by experts and ranked 11th by the MFRM. In most cases, the expert ratings were adjusted to lower MFRM fair averages.
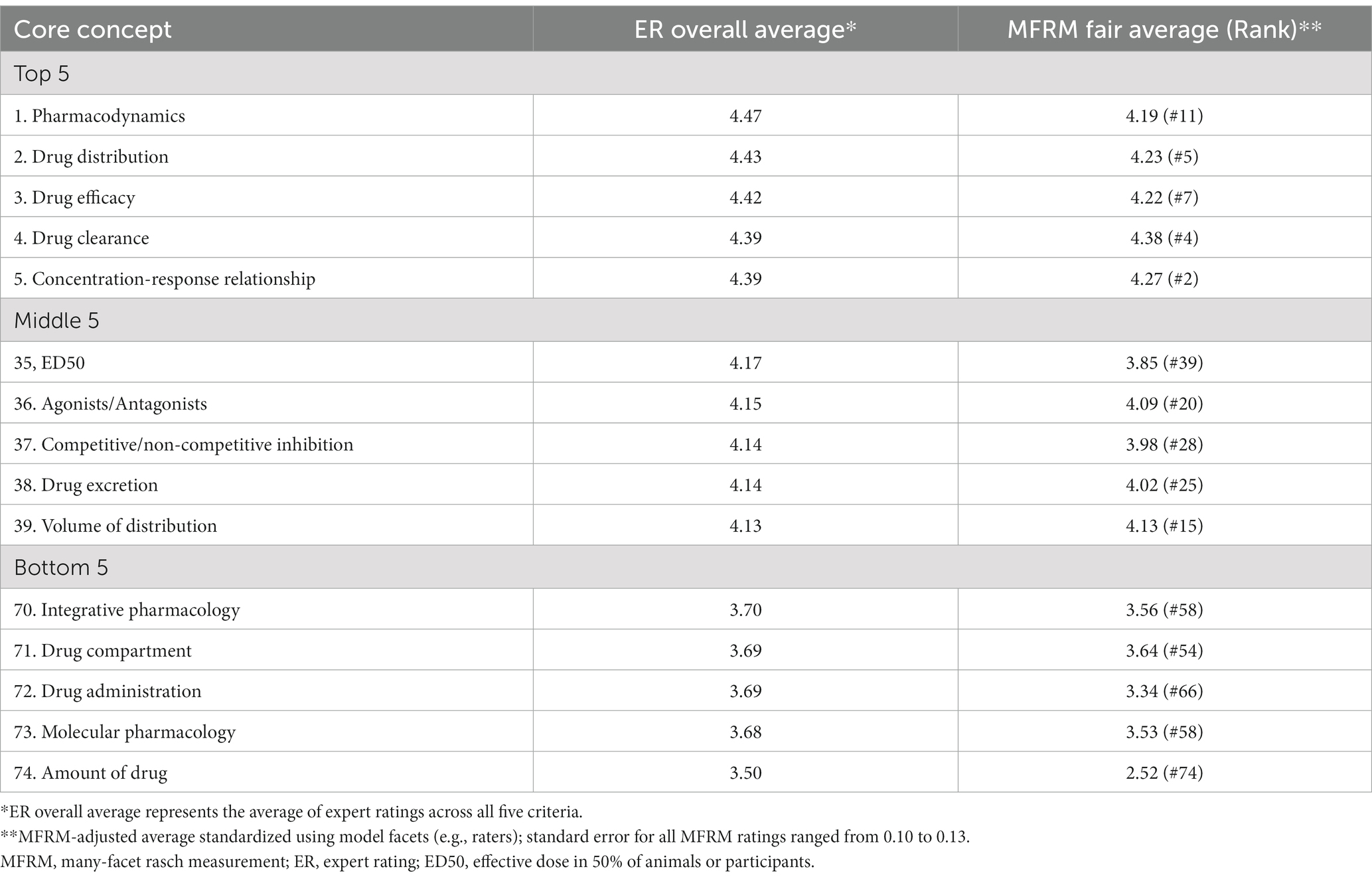
Table 4. Comparison of core concepts rankings from delphi expert ratings and MFRM with rater adjustments.
Rater severity accounted for 13% of the variance in the ratings. Of the 21 raters, one (5%) had Infit and Outfit MnSqs greater than 1.7, meaning that the rater displayed a significantly unexpected degree of variability in their ratings of the core concepts. None of raters (0%) had an Infit MnSq and Outfit MnSq of less than 0.5, suggesting that their ratings discriminated between concepts to the expected degree. High separation and a reliability index of approximately 1.00 suggested that the criteria were reliably separated with a high degree of confidence (p < 0.001).
The Wright Map, illustrating the MFRM results on a common equal-interval logit scale, is shown in Figure 1. All data points are plotted on a common equal-interval logit scale from −1 to 2. The second column positions concepts according to their suitability, with the less suitable concepts on top. The third column positions raters according to their severity, starting from the most sever rater on top to the most lenient at the bottom. The fourth column illustrates the difficulty of the criteria, with the most difficult criteria at the top. The horizontal dotted lines in the last column—“Scale”—indicate the rating scale category thresholds, which illustrate the point at which the likelihood of receiving the next higher rating is equivalent to the likelihood of receiving the next lower rating. At a glance, it appears that the raters and criteria tend to group toward the bottom of the scale while concepts tend to group toward the top (i.e., raters/criteria and concepts are skewed in opposite directions), suggesting that more research may be needed to explore how well raters and criteria are targeted to the concepts.
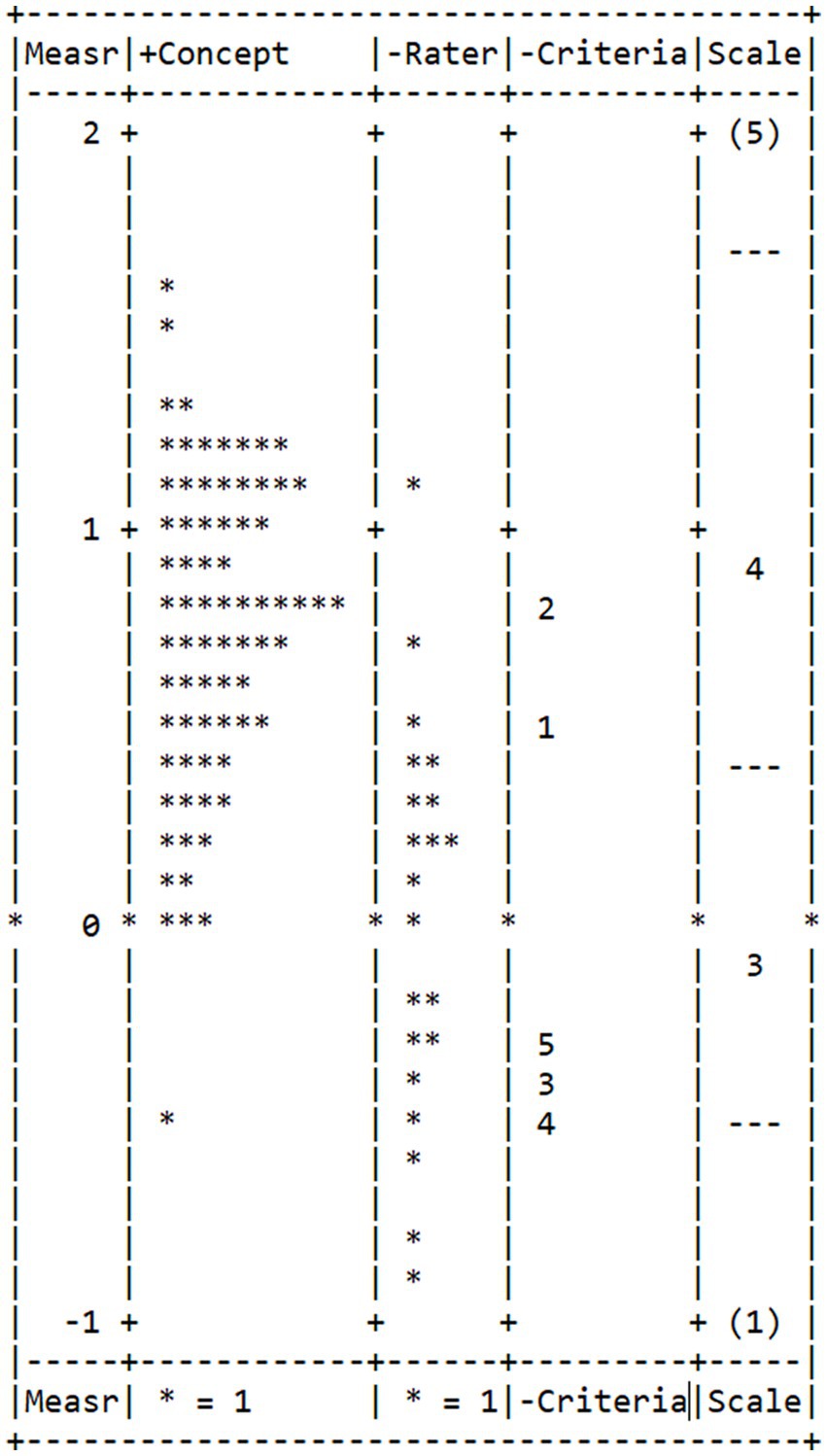
Figure 1. Variable map (also known as a Wright Map) showing ratings for the 74 core concepts estimated by the MFRM using core concept suitability, rater severity, and criterion difficulty. Criterion 1 = Complex; Criterion 2 = Challenging; Criterion 3 = Enduring; Criterion 4 = Fundamental; and Criterion 5 = Useful.
4. Discussion
Core concepts offer a promising approach to education, with additional disciplines beginning—and advocating for—the identification of core concepts for their training programs (e.g., Angelo et al., 2022). Identifying and refining the criteria that characterize core concepts is critical for advancing core concepts research. Ultimately, the aim is to ensure that the core concepts being taught and assessed are the most critical for student learning and success in a given discipline. This study has used rigorous measurement to better elucidate core concepts research, providing evidence regarding the use of specific criteria and rating scales for expert evaluation of concept suitability. Using a three-facet MFRM, we have provided support for specific core concepts criteria (i.e., challenging, complex, enduring, fundamental, and useful), a rating scale (i.e., 1—not at all to 5—extremely), and the core concepts themselves. High separation and reliabilities indicated a sufficient sample and low measurement error, and suggested that the criteria and rating scale were sensitive enough to distinguish between suitable and (potentially) unsuitable core concepts (Linacre, 2023).
As they relate specifically to the criteria used to evaluate core concept suitability, a number of findings are worth noting. First, our analysis indicated that the five criteria were in fact measuring five distinct aspects of core concepts, supporting the use of each criterion. However, there also appears to be a pattern of grouping in the criteria, with challenging and complex relatively similar to one another and fundamental, useful, and enduring relatively similar to one another. This suggests that the five criteria could be collapsed into no fewer than two criteria, which may help reduce the amount of time needed to identify core concepts. The Rasch-Andrich Thresholds in Table 2 also suggest that the rating scale may also be collapsible into fewer categories, which could further improve efficiency in the rating process. Second, the criteria varied in terms of their difficulty—for example, concepts were less likely to get a high rating for challenging than for fundamental. As such, researchers should give some thought to what this type of variation means for core concepts research (e.g., Should certain criteria be weighted more or less in the core concept identification process?).
The proportion of variance reflecting rater severity aligned with other MFRM studies in biomedical sciences. For example, McLaughlin et al. (2017) identified 16% rater severity and Singer et al. (2016) identified 9% rater severity in MFRM models of admissions interview ratings. Similarly, research on standardized assessments in medical education indicated that raters accounted for approximately 15–17% of score variance (Floreck and De Champlain, 2001; Sebok et al., 2015). A benefit of MFRM is the ability to adjust estimates of core concept suitability for differences in rater severity even when it is not possible for all raters to rate all concepts. In theory, this adjustment should provide more accurate ratings for core concepts and improve comparability of concepts. However, conscious tendencies to be severe or lenient in the rating process can also be accurate, complicating the need for adjustment. In addition, MFRM assumes that rater severity does not change during the rating process and therefore does not account for rater severity drift. As such, consideration should be given to strategies for identifying sources of rater severity and reducing the influence of rater bias in core concept identification, such as additional rater training, more stringent expert selection criteria, or adjusting for potentially confounding rater characteristics in the MFRM. For example, raters in this study were not trained to meet a certain criterion (e.g., interrater agreement) which could be an opportunity for improvement.
The core concepts used in this study were sourced from data mining textbooks and surveying experts (White et al., 2022). Nine of the top 10 concepts from the MFRM analysis were also identified as core concepts by experts in the three-round Delphi study by White et al. (2022): drug clearance, drug mechanism of action, concentration-response relationship, drug distribution, drug half-life, drug efficacy, dose–response curve, mechanism of drug action, and drug metabolism (White et al., 2022); during the Delphi process, experts reframed pharmacokinetics as a category of core concepts instead of its own concept and combined several concepts, such as concentration-response relationship and dose–response curve. None of the bottom five concepts from the MFRM analysis were identified as core concepts in the Delphi process. The MFRM provided adjusted ratings that may further indicate the extent to which each concept was “core.” Although the fair averages shifted the rankings of the concepts, the MFRM generally confirmed the expert opinions in the Delphi study. Drug administration, for example, was ranked #72 by the Delphi participants and #66 by the MFRM. However, in a few cases, the rankings were more disparate—for example, Volume of Distribution was ranked #39 by Delphi participants and #15 by the MFRM. Researchers should consider the implications of these types of adjustments for core concept research and the potential use of thresholds or cut scores that separate concepts that are core from those that are not.
An added benefit of this research is access to the Delphi results of White et al. (2022), which represent a negotiated list of the same pharmacology core concepts based on iterative expert input. Researchers should consider whether MFRM could be used in conjunction with, or even instead of Delphi, to identify core concepts. Delphi exercises require significant time and resources (Olsen et al., 2021), and the majority of core concepts that reached the threshold of the Delphi in this case were highly ranked in the MFRM. Therefore, MFRM could potentially replace one or more rounds of the Delphi exercise or could be used to create cut scores. Alternatively, the MFRM data could be used to distinguish between basic concepts, which might be predicted to be rated as fundamental, useful and enduring, but not challenging or complex, and more advanced concepts, which might be predicted to be rated highly on all criteria.
Overall, the model reported in this paper accounts for a suitable proportion of variance compared to other published MFRM models (e.g., Roberts et al., 2010). However, the model does leave just over 60% of ratings variability unaccounted for, suggesting that there is room for improvement. Further core concepts research and techniques for decreasing variability in ratings may prove useful for improving identification process. Refining the criteria to better target the intended constructs, employing additional rater training, rethinking the qualifications for experts, and including additional facets in the MFRM may account for additional variability.
This study is the first of its kind in core concepts research and, as such, has several limitations. First, the data were generated from a relatively small sample. Second, the model was limited to three facets, leaving some question as to how other, unavailable facets might have influenced the results (e.g., educational background). Third, this study focused on MFRM, leaving some question as to how this methodology might be integrated with other commonly used methods for core concept identification. For example, the criteria used in this study could be further refined by extrapolation from concept inventory data, in that the ratings on the five criteria could be compared to the success of students on items that test attainment of those particular concepts. Future research should focus on improving the facets described in this model, identifying additional facets that might influence core concept ratings, and exploring the integration of MFRM into the core concept identification process.
5. Conclusion
This study demonstrates the feasibility of using MFRM for evaluating and validating data generated for the purpose of identifying core concepts in STEM disciplines similar to Pharmacology. The data generated in this study support the use of five different criteria for identifying core concepts. This study raises several interesting questions and opportunities related to how the MFRM might be used within core concept research.
Data availability statement
The datasets presented in this study can be found in online repositories. The names of the repository/repositories and accession number(s) can be found at: openICPSR at https://www.openicpsr.org/openicpsr/project/184242/version/V3/view.
Ethics statement
The studies involving human participants were reviewed and approved by Monash University Ethics in Human Research Committee. The patients/participants provided their written informed consent to participate in this study.
Author contributions
JM, TA, and PW contributed to conception and design of the study. JM organized the database, performed the statistical analysis, and wrote the first draft of the manuscript. PW provided the data and wrote sections of the manuscript. All authors contributed to the article and approved the submitted version.
Acknowledgments
The authors would like to acknowledge Kyle Fassett for his support.
Conflict of interest
The authors declare that the research was conducted in the absence of any commercial or financial relationships that could be construed as a potential conflict of interest.
Publisher’s note
All claims expressed in this article are solely those of the authors and do not necessarily represent those of their affiliated organizations, or those of the publisher, the editors and the reviewers. Any product that may be evaluated in this article, or claim that may be made by its manufacturer, is not guaranteed or endorsed by the publisher.
References
Angelo, T. A., McLaughlin, J. E., Munday, M. R., and White, P. J. (2022). Defining core conceptual knowledge: why pharmacy education needs a new, evidence-based approach. Curr. Pharm. Teach. Learn. 14, 929–932. doi: 10.1016/j.cptl.2022.07.014
Bond, T. G., and Fox, C. M. (2013). Applying the Rasch Model: Fundamental Measurement in the Human Sciences New York: Psychology Press.
Boneau, C. A. (1990). Psychological literacy: a first approximation. Am. Psychol. 45, 891–900. doi: 10.1037/0003-066X.45.7.891
Brewer, C. A., and Smith, D. (2011). Vision and Change in Undergraduate Biology Education: A Call to Action. Washington, DC: American Association for the Advancement of Science.
Floreck, L. M., and De Champlain, A. F. (2001). Assessing sources of score variability in a multisite medical performance assessment: an application of hierarchical line modeling. Acad. Med. 76, S93–S95. doi: 10.1097/00001888-200110001-00031
Foster, J.M., Sultan, M.A., Devaul, H., Okoye, I., and Sumner, T. (2012). “Identifying core concepts in educational resources” in Proceedings of the 12th ACM/IEEE-CS Joint Conference on Digital Libraries, 35–42.
Harlen, W. (Ed.) (2010). Principles and Big Ideas of Science Education. Hatfield, Herts: Association for Science Education.
Hestenes, D., Wells, M., and Swackhamer, G. (1992). Force concept inventory. Phys. Teach. 30, 141–158. doi: 10.1119/1.2343497
Iramaneerat, C., Yudkowsky, R., Myford, C. M., and Downing, S. M. (2008). Quality control of an OSCE using generalizability theory and many-faceted Rasch measurement. Adv. Health Sci. Educ. 13, 479–493. doi: 10.1007/s10459-007-9060-8
Landrum, R. E. (1993). Identifying core concepts in introductory psychology. Psychol. Rep. 72, 659–666. doi: 10.2466/pr0.1993.72.2.659
Libarkin, J. (2008). “Concept inventories in higher education science.” in Workshop 2 in Promising Practices in Undergraduate STEM Education Conference. October 13–14, 2008. National Research Council. Available at: https://sites.nationalacademies.org/cs/groups/dbassesite/documents/webpage/dbasse_072624.pdf (Accessed January 17, 2023).
Linacre, J. M. (1989). Many-faceted Rasch measurement. Doctoral dissertation. The University of Chicago.
Linacre, J. M. (2023). Reliability and separation of measures. Available at: https://www.winsteps.com/winman/reliability.htm (Accessed January 17, 2023).
Malau-Aduli, B. S., Alele, F., Collares, C. F., Reeve, C., Van der Vleuten, C., Holdsworth, M., et al. (2019). Validity of the scan of postgraduate educational environment domains (SPEED) questionnaire in a rural general practice training setting. BMC Med. Educ. 19:25. doi: 10.1186/s12909-019-1455-8
McFarland, J. L., Price, R. M., Wenderoth, M. P., Martinková, P., Cliff, W., Michael, J., et al. (2017). Development and validation of the homeostasis concept inventory. CBE life. Sci. Educ. 16:ar35. doi: 10.1187/cbe.16-10-0305
McLaughlin, J. E., Singer, D., and Cox, W. C. (2017). Candidate evaluation using targeted construct assessment in the multiple mini-interview: a multifaceted Rasch model analysis. Teach. Learn. Med. 29, 68–74. doi: 10.1080/10401334.2016.1205997
Michael, J., Cliff, W., McFarland, J., Modell, H., and Wright, A. (2017). “The 'unpacked' core concept of homeostasis” in The Core Concepts of Physiology: A New Paradigm for Teaching Physiology (New York: Springer), 45–54.
Olsen, A. A., Wolcott, M. D., Haines, S. T., Janke, K. K., and McLaughlin, J. E. (2021). How to use the Delphi method to aid in decision making and build consensus in pharmacy education. Curr. Pharm. Teach. Learn. 13, 1376–1385. doi: 10.1016/j.cptl.2021.07.018
Parekh, G., DeLatte, D., Herman, G. L., Oliva, L., Phatak, D., Scheponik, T., et al. (2018). Identifying core concepts of cybersecurity: results of two Delphi processes. IEEE Trans. Educ. 61, 11–20. doi: 10.1109/TE.2017.2715174
Roberts, C., Rothnie, I., Zoanetti, N., and Crossley, J. (2010). Should candidate scores be adjusted for interviewer stringency or leniency in the multiple mini-interview? Med. Educ. 44, 690–698. doi: 10.1111/j.1365-2923.2010.03689.x
Royal, K. D. (2018). Forty-five common rater errors in medical and health professions education. Educ. Health Prof. 1, 33–35. doi: 10.4103/EHP.EHP_27_18
Sebok, S. S., Roy, M., Klinger, D. A., and De Champlain, A. F. (2015). Examiners and content and site: oh my! A national organization’s investigation of score variation in large-scale performance assessments. Adv. Health Sci. Educ. Theory Pract. 20, 581–594. doi: 10.1007/s10459-014-9547-z
Seol, H. (2016). Using the bootstrap method to evaluate the critical range of misfit for polytomous Rasch fit statistics. Psychol. Rep. 118, 937–956. doi: 10.1177/0033294116649434
Singer, D., McLaughlin, J. E., and Cox, W. C. (2016). The multiple mini-interview as an admission tool for a PharmD program satellite campus. Am. J. Pharm. Educ. 80:121. doi: 10.5688/ajpe807121
Tweedie, J., Palermo, C., Wright, H. H., and Pelly, F. E. (2020). Using document analysis to identify core concepts for dietetics: the first step in promoting conceptual learning. Nurs. Health Sci. 22, 675–684. doi: 10.1111/nhs.12712
White, P. J., Davis, E. A., Santiago, M., Angelo, T., Shield, A., Babey, A. M., et al. (2021). Identifying the core concepts of pharmacology education. Pharmacol. Res. Perspect. 9:e00836. doi: 10.1002/prp2.836
White, P. J., Guilding, C., Angelo, T., Kelly, J., Gorman, L., Tucker, S. J., et al. (2022). Identifying the core concepts of pharmacology education: a global initiative. Br. J. Pharmacol. 1–13.doi: 10.1111/bph.16000
Wolfe, E. W. (2013). A bootstrap approach to evaluating person and item fit to the Rasch model. J. Appl. Meas. 14, 1–9.
Zechmeister, J. S., and Zechmeister, E. B. (2000). Introductory textbooks and psychology's core concepts. Teach. Psychol. 27, 6–11. doi: 10.1207/S15328023TOP27
Keywords: core concepts, concept inventories, criteria, pharmacology, many-facet rasch measurement
Citation: McLaughlin JE, Angelo TA and White PJ (2023) Validating criteria for identifying core concepts using many-facet rasch measurement. Front. Educ. 8:1150781. doi: 10.3389/feduc.2023.1150781
Edited by:
Robbert Smit, St. Gallen University of Teacher Education, SwitzerlandReviewed by:
Michael Peeters, University of Toledo, United StatesKathy Ellen Green, University of Denver, United States
Stefanie A. Wind, University of Alabama, United States
Copyright © 2023 McLaughlin, Angelo and White. This is an open-access article distributed under the terms of the Creative Commons Attribution License (CC BY). The use, distribution or reproduction in other forums is permitted, provided the original author(s) and the copyright owner(s) are credited and that the original publication in this journal is cited, in accordance with accepted academic practice. No use, distribution or reproduction is permitted which does not comply with these terms.
*Correspondence: Jacqueline E. McLaughlin, SmFjcXVpX21jbGF1Z2hsaW5AdW5jLmVkdQ==