- 1Interdisciplinary Laboratory for Research in Education, EA 7483, INSPE, University of New Caledonia, Noumea, New Caledonia
- 2ICARE, EA 7483, University of Reunion, Saint-Denis, France
- 3LaPsyDé, CNRS, University of Paris, Paris, France
This study aimed to evaluate the impact of an educational cognitive neuroscience intervention (NeuroStratE) focusing on teaching the functioning of the brain and practical tools to address the procrastination behavior of 199 students enrolled at university from 2019 to 2021. The evolution of procrastination behavior is measured by specific scales and the planning ability through the Tower of Hanoi test. We compared the change in procrastination behaviors and planning ability between the pre and post-test to those of a control group. Procrastination behavior reduction and planning ability improved more in the students who followed the intervention (N = 179) than those in the control group (N = 20). Using mixed methods, these results were qualitatively refined with student feedback on the value of the intervention program, along with individual student interviews. This study highlights that it is particularly courses of procrastination and associated practical tools which contributed to reduced procrastination behavior and improved the planning ability.
1. Introduction
Neuroscience has been making significant advances in the field of education for several years. It is increasingly acknowledged as being useful in understanding and facilitating learning (Thomas et al., 2019). Increasingly advanced knowledge of brain activity, particularly during learning processes, appeals to the educational community, which sees in it a possible implementation of interesting tools for improving academic success (Howard-Jones et al., 2016).
In the cognitive neurosciences, it is the executive functions and their study which, because of their function, are of interest to the educational community. Numerous intervention programs are emerging, which demonstrate multiple impacts, particularly on executive functions. Diamond and Ling (2016) studied more than 84 interventions programs aimed at improving executive functions.
Academic success concerns all students: from early education right through to university study. Cognitive neuroscience can shed light on all levels of education; specifically, an improvement in executive functions can contribute to academic success across the board.
In the current study we were interested in university students. Students arriving at university often lack autonomy and the ability to organize themselves (Morlaix and Perret, 2013). It would therefore be important to offer them intervention programs based on cognitive neuroscience, with the aim of improving their executive functions. With this in mind, the current study aimed to explore the impact of an intervention program on procrastination behavior and planning ability. It is based on teaching in neurosciences (NeuroStratE: Neuroscience Strategies and Education; Cherrier et al., 2020), provided for bachelor students.
1.1. Executive functions and planning ability
Executive functions (EFs) are defined as high-level cognitive brain processes (Lezak, 1982) allowing an adapted and targeted response to the environment. The frontal lobes are generally referred to as the brain structures involved in carrying out executive functions. On the one hand, the clinical cases have made it possible to define the cerebral localization of these high-level functions. On the other hand, brain imaging techniques (such as positron emission tomography (PET) and magnetic and functional resonance imaging (fMRI)) make it possible to observe the activity of the frontal lobes during cognitive and executive tasks.
The current study was based on the Miyake model (Miyake et al., 2000), which defines three basic EFs which are classically differentiated: working memory, inhibitory control and cognitive flexibility. These basic Efs, according to Diamond’s model (Diamond, 2013), allow one to implement higher-level executive functions: reasoning, problem-solving (fluid intelligence) and planning. Executive functions and their training would be considered a key factor in academic success. Studies reveal a good predictive power of executive functions at the primary education stage, (Cortés Pascual et al., 2019). Zelazo and Carlson (2012) propose that research in education should focus on executive functions because they are fundamental to the development of learning as well as for the processing and organization of the information received. Gunzenhauser and Nückles (2021) are interested in the transfer of executive function training to academic success, showing that the link is close between these two components. In a review of the literature, Diamond and Ling (2020) show the impact of executive function training on academic success. Our study focused on one of these higher-level EFs, namely planning, which can be defined as the ability to develop a sequence of actions in order to achieve a defined goal. This requires defining a goal and determining the intermediate steps and appropriate associated strategies needed to execute an action plan to achieve that goal (Scholnick and Friedman, 1987; Baker-Sennett et al., 1993). The study of the planning function interest the students because they are common activities in university work.
1.2. Procrastination behavior
Procrastination is classically defined as the tendency to want to delay things for the future. While it is difficult to clearly define the behavior (Ferrari et al., 1995) the definition proposed by Klingsieck (2013), p. 26, was adopted for this study: “Procrastination can […] be defined as the voluntary delay of an intended and necessary and/or [personally] important activity, despite expecting potential negative consequences that outweigh the positive consequences of the delay”. The causes of procrastination are multifactorial. Procrastination has been linked to personality traits such conscientiousness, neuroticism, perfectionism but also to anxiety, depression, or stress. It also involves motivational and volitional factors (intrinsic motivation, goal orientation, self-regulation, time-management) and situational factors (task difficulty, task attractiveness, task specificity; Steel, 2007; Klingsieck, 2013).
From a neurological point of view, procrastination can simply be defined as a conflict between the pre-frontal cortex, which is the analytical seat, and the limbic system, which notably processes emotions. Emotions are central to procrastination behavior. Many studies show the importance of taking emotions into account, particularly in the school environment (Eckert et al., 2016; Pychyl and Sirois, 2016; Bytamar et al., 2020).
Procrastination is very common among students. Estimates indicate that 80–95% of students (O’Brien, 2002), or at least half of all students (Ozer et al., 2009), procrastinate. Procrastination is negatively correlated with academic performance (Kim and Seo, 2015), especially at the university level (Osiurak et al., 2015). Thus, reducing procrastination behaviors would appear critical in supporting students’ academic achievement (Grunschel et al., 2018; Zacks and Hen, 2018). Strategies need to be adapted to the factors at the root of the procrastination behavior and the contexts in which they are observed (Klingsieck, 2013). Whether it is a problem of motivation, management or projection in time, habit, beliefs, perfectionism or self-esteem, each time it will be necessary to implement strategies and tools related to these particular aspects. The procrastinator first needs to be specified and defined via measurement scales (Mann, 1982; Solomon and Rothblum, 1984; Lay, 1986; Steel, 2010). They then need to go through a phase of reflection and introspection to know what kind of procrastinator they are and in which contexts they procrastinate (Schouwenburg, 2004). What would also appear useful is a combination of theory and practical aspects to promote understanding toward eventual self-regulation (setting objectives, planning and time management), which is one of the more important associated aims (Schouwenburg, 2004).
1.3. Present study and proposed hypothesis
We seek to determine the impact of neuroscience training on academic success. Measuring the evolution of a behavior common to students: procrastination and of an executive function: planning can be a means. Why propose a link between procrastination and planning? If, as argued above, one of the more appropriate ways of improving procrastination is self-regulation, which involves setting objectives, planning and time management (Schouwenburg, 2004), an improvement of planning function would be expected. The executive function of planning is precisely this cognitive ability which involves the sequence of steps necessary for the achievement of a specific goal.
Similarly, combining theoretical aspects of procrastination with associated practical tools stands out among those learning strategies which aim to overcome this behavior. Thus, the implementation of a training system for learners, combining knowledge in cognitive neuroscience, with the presentation of appropriate tools, may make it possible on the one hand to modify this attitude of procrastination toward academic work as well as in the organization of daily life, and could on the other hand have an effect on the executive function of planning.
In a previous study, NeuroStratE (Cherrier et al., 2020) was implemented with secondary school students. The results indicated no improvement in school results but better self-knowledge. In the current study we tested the part of the program which related to procrastination in university students.
The present study aims to assess the effect of an intervention which focuses on teaching undergraduate students the functioning of the brain and providing practical tools (NeuroStratE, Cherrier et al., 2020). Participants’ procrastination behaviors and planning ability were measured at the start and the end of a semester. In light of findings of previous studies (Osiurak et al., 2015; Steel and Klingsieck, 2016; Gunzenhauser and Nückles, 2021) we hypothesize that students following the intervention would exhibit fewer procrastination behaviors and show an improvement in their planning ability to a larger extent than students of the control group not exposed to the intervention (active control group).
2. Method
2.1. Participants and context
The final sample consisted of 199 university students aged 17–24 years. Participant details are described in Table 1. The intervention was part of an action to improve student academic success at the University.
In this sense, our study could be described as action research (McNiff, 2013). In terms of action, we needed to adapt to the constraints imposed by the university. In this case, students chose the teaching of neurosciences as an option. If they did not choose it, they followed another course (of their choice) for the same amount of time. Those students constituted the active control group. This was beneficial for our research study as we were provided with a readily available control group. It is also this particular context of research which will explain that we have suffered a loss of students and in particular on the control group between the pre and the post test. Indeed, the latter reorient themselves or leave the university.
The intervention was proposed to students of the Bachelor over 1 semester for a duration of 20 h/semester. The module was offered to 2 departments the Department of Science and Technology and the Department of Literature, Languages and Human Sciences (Table 1). Students of the active control group were enrolled in the Bachelor in 2020. For the remainder of this paper, the active control group will be designated as the control group.
2.2. Intervention program
The NeuroStratE program (Cherrier et al., 2020) is structured around 4 themes: learning to learn, learning to concentrate, learning to memorize, learning to put strategies in place. Each intervention comprises: neurophysiological and neurocognitive content; activities allowing students to experience and test the theme; and finally, the implementation of strategies which were evaluated from 1 week to the next. In the interests of space, we will not detail here the entire program provided during the training (Cherrier et al., 2020). Rather, we will direct our attention to the effect of “the learning to put strategies in place,” which directly concerns procrastination, the focus of our study.
This study aspect allowed students to address two main elements: knowing how to manage time and knowing how to set goals, enabling them to plan their own work. It is through the behavioral theme of procrastination that these elements have been approached. This theme and its tools were treated in 6 h (2 h of neurobiological aspects and 4 h for tools and using) spread over 3 weeks.
In this theme, we began by defining procrastination as behavior, followed by an explanation of the associated neurophysiological processes (conflict between prefrontal cortex and limbic cortex). Next, we sought to define the procrastination profile of each student, asking them to determine in which context and for what task they were procrastinating. We also allowed them to measure their degree of procrastination using a variety of scales. Three scales were used: a general procrastination scale (Lay, 1986); a scale of decision-making procrastination (Mann, 1982); and a scale of procrastination for academic work (Solomon and Rothblum, 1984; Osiurak et al., 2015). These measurements were made at the beginning and end of the module. We then proposed different tools. Among the tools used were the Pomodoro technique (Cirillo, 2006, 2009); the setting of objectives with the SMART method (Drucker, 1954; Doran, 1981; Lawlor and Hornyak, 2012); the organization of tasks with the Eisenhower Matrix (Kirillov et al., 2015); planning tools (agendas, bullet journal, smart phone applications...); and finally, a feedback mechanism to test them and see what works for them. Each student had to define their procrastination profile including the evaluation on the above-mentioned scales and had to test strategies (those proposed in class or elsewhere) and present their assessment to the other students but also in a final report evaluating the training module.
2.3. Measurement tools
In order to evaluate the learning program, we decided to employ a mixed methods approach (Johnson and Onwuegbuzie, 2004), which utilizes both quantitative and qualitative tools, termed explanatory design (Creswell and Plano Clark, 2018). The first phase of the research was characterized by the collection and analysis of quantitative data. Qualitative data analyzed secondarily generally deepen and explain in more detail the first quantitative results (Creswell and Plano Clark, 2018). Qualitative analyzes thus make it possible to give more prominence to quantitative data and to provide avenues for reflection (Figure 1).
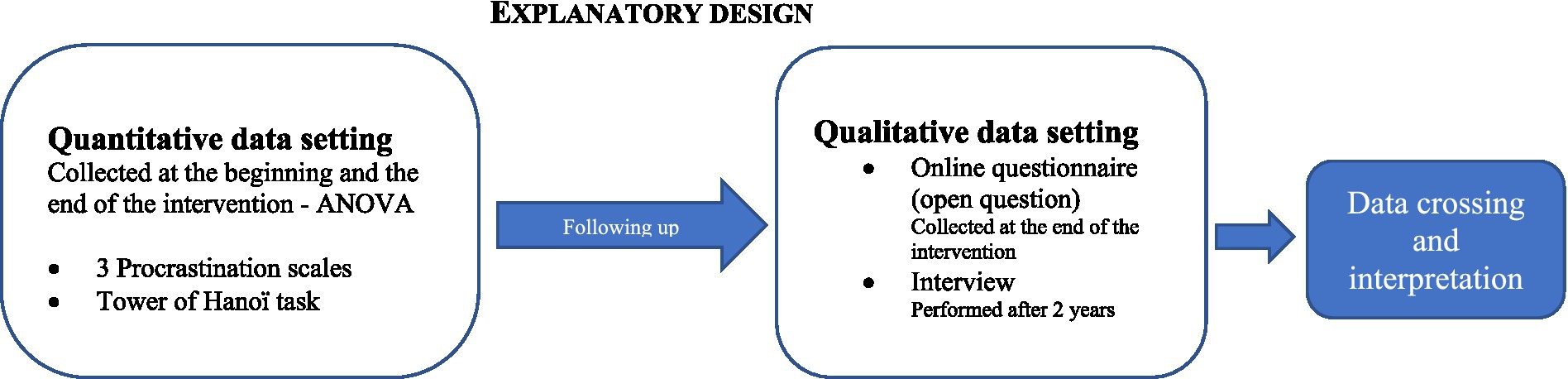
Figure 1. Methodologic design: sequential mixed methods designs: explanatory design (Creswell and Plano Clark, 2018).
2.3.1. The quantitative analysis
For both the test and the control group, procrastination behaviors and planning ability were measured at the beginning (initial time) and the end of the semester (final time).
2.3.1.1. Procrastination behaviors
Procrastination behaviors were assessed with three seminal scales: a general procrastination scale (Lay, 1986); a decision-making procrastination scale (Mann, 1982); and a procrastination scale for academic work (Solomon and Rothblum, 1984; Osiurak et al., 2015). Lay’s procrastination scale, “General Procrastination Scale” (GPS; Lay, 1986) targets the more general aspects of procrastination, i.e., postponing things. Mann’s “Decisional Procrastination Questionnaire” (DPQ; Mann, 1982) focuses on aspects related to decision-making. The academic procrastination scale (Osiurak et al., 2015 inspired by the Procrastination Assessment Scale-Students PASS, Solomon and Rothblum, 1984) focuses on highlighting procrastination in the specific context of academic work. For all these scales participants indicate their degree of agreement on a Likert scale ranging from 1 (it’s not me) to 5 (it’s me). Each scale has several questions and a specific count point depending on the answers to these questions. Thus, the scores vary from −15 to +21 for the Lay (1986) scale from 5 to 25 for the Mann (1982) scale and from 12 to 60 for the academic procrastination scale (Osiurak et al., 2015). Low scores indicate a low tendency to procrastinate while high scores indicate a strong tendency to procrastinate.
2.3.1.2. Planning ability
We asked participants to perform The Tower of Hanoi test to evaluate planning ability (Simon, 1975). This test examines the subject’s ability to solve complex problems, in asking them to formulate a plan to reach the solution. The plan must include an overall objective which is broken down into various sub-objectives which must be carefully ordered. The Tower of Hanoi test includes three vertical rods and a number of graduated-sized donut-shaped disks. Initially all the disks are arranged pyramidally on the first rod, the largest disk being positioned at the base. The task is to move all the disks from rod 1 to rod 3 through rod 2 and keeping the pyramidal stack of the start, with as little movement as possible. There are constraints: only one disk can be moved at a time and one cannot arrange a larger disk on top of a smaller disk. As part of our study, we tested this puzzle with 4 disks and 5 disks on a computerized version. The minimum number of movements for a solution is 2n–1, where n is the number of disks (for 4 disks: 15 movements, for 5 disks: 31 movements). The lower the number of moves to find the solution the better the planning ability. Students having reported less than the minimum number of movements were excluded from the analysis of planning ability.
2.3.1.3. Statistics
We ran separate mixed effect 2 (Group: Test vs. Control) X 2 (Time: T initial vs. T final) analysis of variances (ANOVAs) on each of the procrastination scales and on the scores in the Tower of Hanoi task. The scores in the Tower of Hanoi test (i.e., the number of moves) were first power transformed because of a noncompliance for the ANOVA hypothesis. We performed the lmer and anova functions of the lmerTest R package which is able to compute type III ANOVA tables for fixed-effect terms with Satterthwaite’s method (Giesbrecht and Burns, 1985; Hrong-Tai Fai and Cornelius, 1996; Schaalje et al., 2002; Kuznetsova et al., 2017). Effect sizes are reported in terms of partial eta-squared ( ) computed with the eta_squared function from the effectsize R package (Ben-Shachar et al., 2020). The contrasts and post-hoc tests were computed with the lsmeans function from the emmeans R package, p-values were adjusted with Holm’s correction and Cohen’s d for effect sizes were computed with the eff_size function from the emmeans R package (Holm, 1979; Lenth et al., 2021). These analyzes were conducted using R 4.1.0 (R Core Team, 2021) with an accepted type 1 error probability set at α = 0.05.
2.3.2. The qualitative analysis
The qualitative analysis focused on several measurements. We will present here only those that concern this study. An online questionnaire, offered to all participants, included 2 questions: “Indicate the positive points of this training” and “Indicate the negative points of this training.” A collect interview of 15 min duration was conducted in 2021, where we put questions to students who took the module during the year 2019. The main question concerned what they had retained of the cognitive neuroscience module they followed in 2019. Among the sub-questions we asked them if they thought that knowing theoretical aspects in neuroscience about the functioning of their brain and associated behaviors had been of interest to them or if only the methodological tools proposed were sufficient. Only 20 students who took the module in 2019 could be contacted and agreed to be interviewed, the others are no longer present at the University.
To analyze this qualitative data, we implemented 3 phases: a pre-analysis, which is a rather intuitive step and which involves a general reading to choose the global areas of investigation and lead to a categorization of the data (Mayring, 2000; Robert and Bouillaguet, 2007; Bardin, 2013; Schreier et al., 2019); and a second phase, where themes and sub-themes are defined by identifying keywords. The set is listed on a grid. To ensure internal consistency the analysis followed a correspondence of the items: word by word or sentence by sentence or ideas by ideas, etc.
The last phase concerned the interpretation of the classified data where simple statistical operations (such as percentages) could be performed to provide quantified information.
3. Results
3.1. Procrastination behaviors
The Table 2 presents the results obtained on the different procrastination scales tested with the students who followed the training program (2019 S1, 2020 S1, S2 and 2021 S1) and with the control group (2020 S1), in pre (T initial) and post (T final) training. The Figure 2 offers a more visual presentation of the same results through boxplots.

Table 2. Students’ average and standard deviation for procrastination scores (Lay, Mann and Academic) in the test and control groups on the pre and post training period.
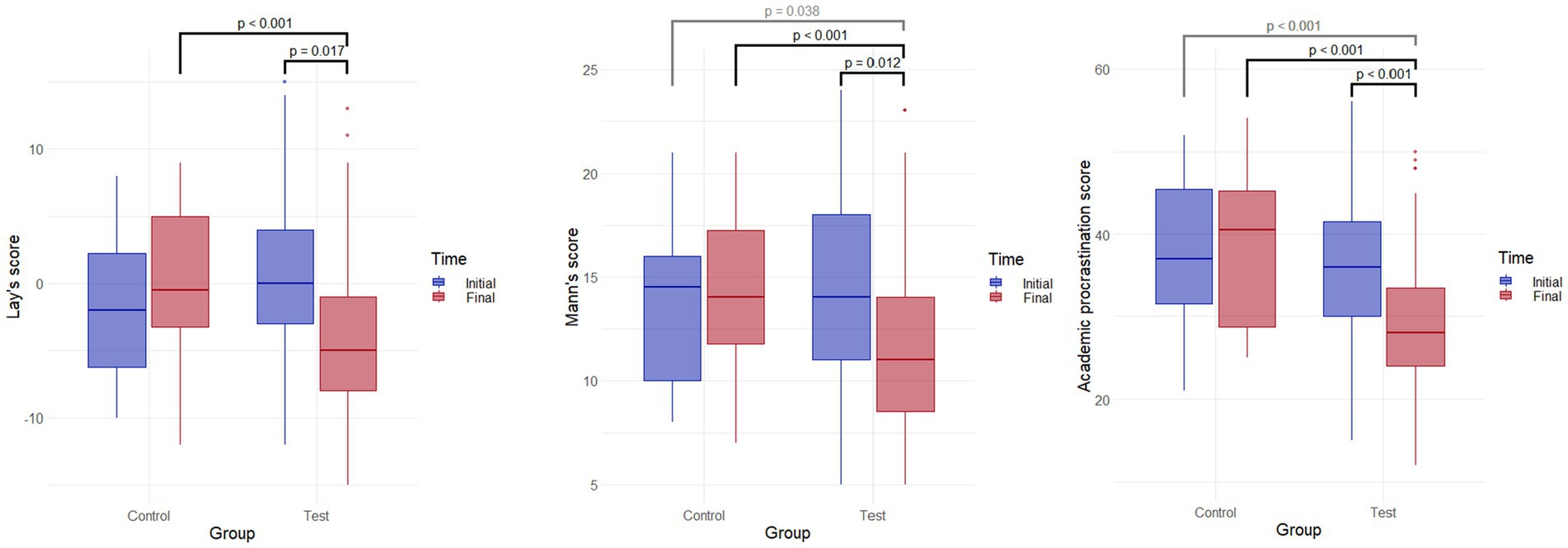
Figure 2. Boxplots of students’ procrastination scores according to studied groups: Lay’s scale, Mann’s scale, Academic scale.
The ANOVA on the scores in the Lay’s scale revealed a significant two-way interaction among time (T initial and T final) and group (Test and Control), Besides, we found a main effect of time, . When considering contrasts and post-hoc tests, significant differences were found between the test group and the control group at T final [ ] and between T initial and T final in the test group ( ) (See Table 2; Figure 2).
The ANOVA on the scores in the Mann’s scale revealed a significant two-way interaction among time (T initial and T final) and group (Test and Control), Moreover, we found a main effect of time, . When considering contrasts and post-hoc tests, significant differences were found between the test group and the control group at T final ( ), between the test group at T final and the control group at T initial ( ) and between T initial and T final in the test group ( ) (See Table 2; Figure 2).
The ANOVA on the academic procrastination scale revealed a significant two-way interaction among time (T initial and T final) and group (Test and Control), . We found a main effect of time, ; as well as a main effect of group, . When considering contrasts and post-hoc tests, significant differences were found between the test group and the control group at T final ( ), between the test group at T final and the control group at T initial ( ) and between T initial and T final in the test group ( ) (See Table 2; Figure 2).
3.2. Planning ability
The Table 3 shows the results obtained in the Tower of Hanoï task, for 4 and 5 disks, with students who followed the training program (2019 S1, 2020 S1, S2, and 2021 S1) and with the control group (2020 S1), in pre (T initial) and post (T final) training. The Figure 3 offers a more visual presentation of the same results through boxplots.

Table 3. Students’ average and standard deviation for planning scores (number of movements in the Tower of Hanoi task with 4 disks and 5 disks) in the test and control groups on the pre an post training period.
The ANOVA for four disks, after a power transformation (−2.31) of the number of movements, revealed a significant two-way interaction among time (T initial and T final) and group (Test and Control), . When considering contrasts and post-hoc tests, a significant difference was found only between T initial and T final in the test group ( ) (See Table 3; Figure 3).
The ANOVA for five disks, after a power transformation (−1.25) of the number of movements, revealed a significant two-way interaction among time (T initial and T final) and group (Test and Control), . When considering contrasts and post-hoc tests, a significant difference was found only between T initial and T final in the test group ( ) (See Table 3; Figure 3).
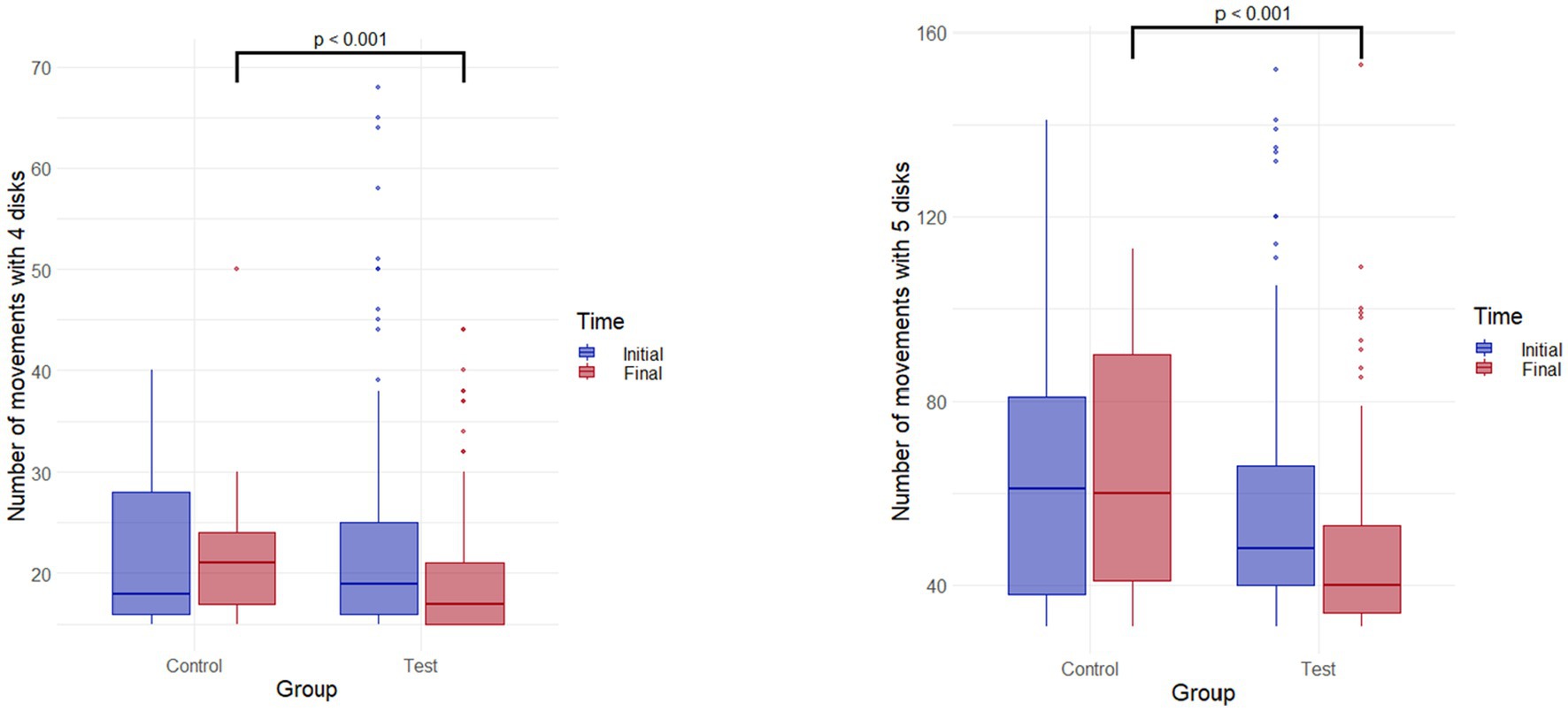
Figure 3. Boxplots of students’ number of movements in the Tower of Hanoi task, during the two periods (initial and final) according to studied groups: 4 disks and 5 disks.
These results show an improvement in declared procrastination between the beginning and the end of the training as well as better scores in the Tower of Hanoi compared to the control group.
3.3. The qualitative analysis
As part of a mixed method analysis (Johnson and Onwuegbuzie, 2004) we used the mixed method of explanatory design (Creswell and Plano Clark, 2018; Creswell and Plano Clark, 2018), which uses qualitative data to give more context and to deepen the analysis of quantitative data. Here, two additional elements of analysis were examined: the answers of the students to a question on the positive and negative aspects of the program posed using an online questionnaire and the elements of response collected during individual interviews with students who took the module during the year 2019.
3.3.1. Analysis of student responses on the intervention program
The self-evaluation by students who followed the program, via the questionnaire, comprised an open-ended question on the positive and negative aspects of the program.
The different responses are categorized and referenced in two Tables showing ideas expressed as percentages. We only show here the items that are of interest to our study.
These results indicate that students who benefited from the program during the year 2019, 2020 and 2021 found that the module had given them a better knowledge of themselves (between 69.04 and 55.73%). They mention in particular having acquired better time management through planning and setting specific objectives (between 31.14 and 46.42%). On the other hand, they found the module much too short and much too condensed (between 69.77 and 8.33%). They indicated that they did not have enough time to appropriate the concepts, use the tools and interact with other students during the sessions. At the same time, they indicated that it led to a lack of time and an overload of personal work (between 9.3 and 30%).
3.3.1.1. Analysis of contents of student interviews
In 2021 we interviewed students who took the module in 2019 during a 15-min interview with a specific question.
Of the 20 students interviewed, 100% found it useful, important, or essential to study neurobiological aspects in combination with the methods proposed for each theme. 100% remembered the methods offered and were able to quote and explain them. 90% still used these methods according to their needs. Finally, even if they no longer really remembered the theoretical neurobiological content, at 85%, and specified that they should be reactivated at some stage, they all found that it lent meaning to the content, scientific credibility and provided a concrete light on their behavior. This provided a complete rationale to the tools used afterwards. They therefore concluded that theoretical neurobiological data cannot and should not be separated from the methods and tools.
4. Discussion
In this study we investigated whether a neuroscience program (NeuroStratE) through metacognition, could have an impact on procrastination and executive planning function in university students.
Impact was measured through changes in behavior, procrastination and the executive function of planning. We hypothesized an improvement of these aspects following an intervention program (NeuroStratE; Cherrier et al., 2020), with a more significant improvement to be seen after a longer duration of the intervention.
Utilizing a methodological approach (Johnson and Onwuegbuzie, 2004), in particular explanatory design (Creswell and Plano Clark, 2018), an examination of results highlighted a number of elements: the program leads to an improvement in procrastination behavior in parallel with planning ability, better time management and planning, and better self-knowledge. The combination of theoretical neurobiological content and practical tools is also an important factor in the observed improvements.
4.1. Reduction of procrastination behavior
If we compare the results in the test groups at the beginning and at the end of the program, we found that procrastination behaviors in general, in the contexts of decision making and academic performance, decreased from the start to the end of the semester in students who followed the intervention in comparison to the ones who did not (Table 2; Figure 2). For each procrastination scale, our results showed significant two-way interaction among time and group. Thus, the post-hoc tests notably revealed significant differences in the test group between T initial and T final as well as significant differences between test group and control group at T final while these differences did not occur at T initial. Although the effect sizes of interactions were small or medium ( from 0.05 to 0.10), these couple of significant differences suggest an effect of the NeuroStratE program on procrastination.
It was clear that setting up a training system in cognitive neuroscience by working on both theoretical and practical aspects led to an improvement in procrastination behavior. Similarly, the training content seems to be a relevant factor, both in understanding the phenomenon in the axes proposed (setting objectives, time planning) (Table 4), but also in the search by each student for the strategies most appropriate to themselves. This confirms what goal-setting theorists argue: that goal setting is a real lever for motivating behavior. Designing specific, motivating and committed goals leads to greater task performance (Bandura, 1997; Bandura and Locke, 2003; Locke and Latham, 2006). In particular, when linked to procrastination behavior, the setting of objectives (Steel, 2007) with other organizational and planning techniques and therefore through self-regulation, shows an improvement in procrastination.
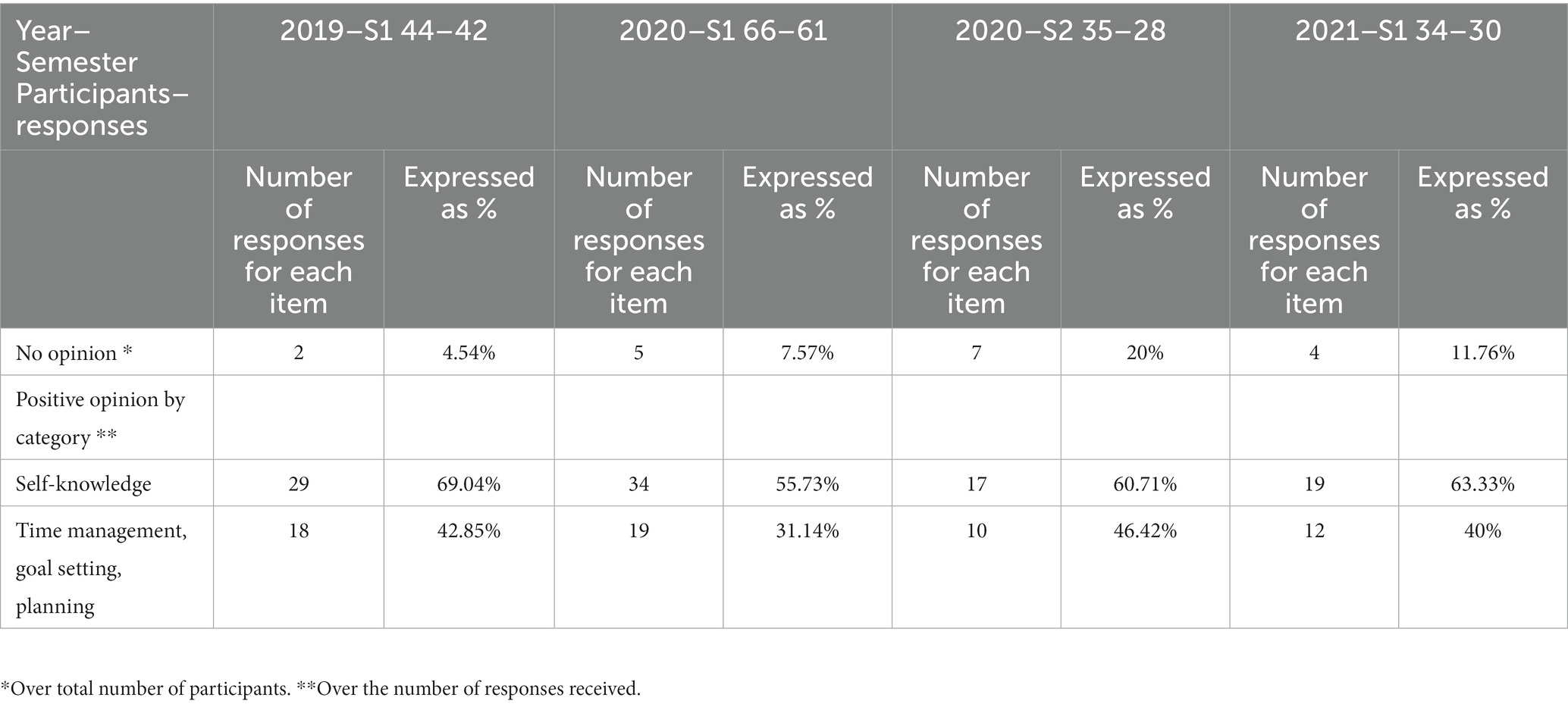
Table 4. Analysis of the contents on the positive aspects of the program, number of responses and percentages for the program delivered (only on items related to our topic).
4.2. Improvement of planning ability
Planning ability assessed in the Tower of Hanoi task improved to a greater extent from the start to the end of each semester in students who followed the program compared to those who did not. For each planning ability test (4 and 5 disks), our results showed significant two-way interaction among time and group. Thus, the post-hoc tests notably revealed significant differences in the test group between T initial and T final. Although the effect sizes of interactions were small ( ), the highlighted significant differences suggest a small effect of the NeuroStratE program on planning ability.
Succeeding on 4 disks is an easier task than on 5 disks because it requires less planning (Welsh, 1991). If we compare the scores obtained in the test groups with 4 disks and 5 disks, we note that the improvement is more significant on the scores of the 5 disks (Table 3). The impact of the program in the direction of improvement is therefore highlighted since the improvement is more significant on 5 disks. This is the case even if the fact of having made the 4 disks previously could serve as training for the 5 disks (Borys et al., 1982). It is known that learning to plan tasks through play or in everyday life improves the planning function (St-Laurent and Moss, 2002). The intervention designed to improve procrastination behavior through time management and goal setting leads to a transfer effect on planning ability.
4.3. Self-knowledge, better time management
Analysis of the contents on the positive aspects of the program (Table 4) shows that the students who benefited from the program found that the module gave them a better knowledge of themselves (between 69.04 and 55.73%) (Table 4). They mention too having acquired better time management through planning and setting specific objectives (between 31.14 and 46.42%). This confirms the results found on the improvement of procrastination.
These aspects are consistent with previous studies (Cherrier et al., 2020), which demonstrate that intervention programs on the knowledge of one’s brain, with the aim of improving student success, lead to better self-knowledge and therefore better self-esteem, which contributes to academic success (Martinot, 2001).
4.4. Importance of learning time and the implementation of training and habits
The feedback from students (Table 5) considerably highlights the lack of time to properly process the themes. Learning time is therefore an important factor. The importance of learning time has already been stressed in a previous study (Cherrier et al., 2020), as well as the importance of establishing new habits and reinforcing them through repetition. Indeed, setting up new habits is very taxing for the brain and requires repetition (Graybiel, 2008; Smith and Graybiel, 2013) and motivation (Kalivas and Volkow, 2005).
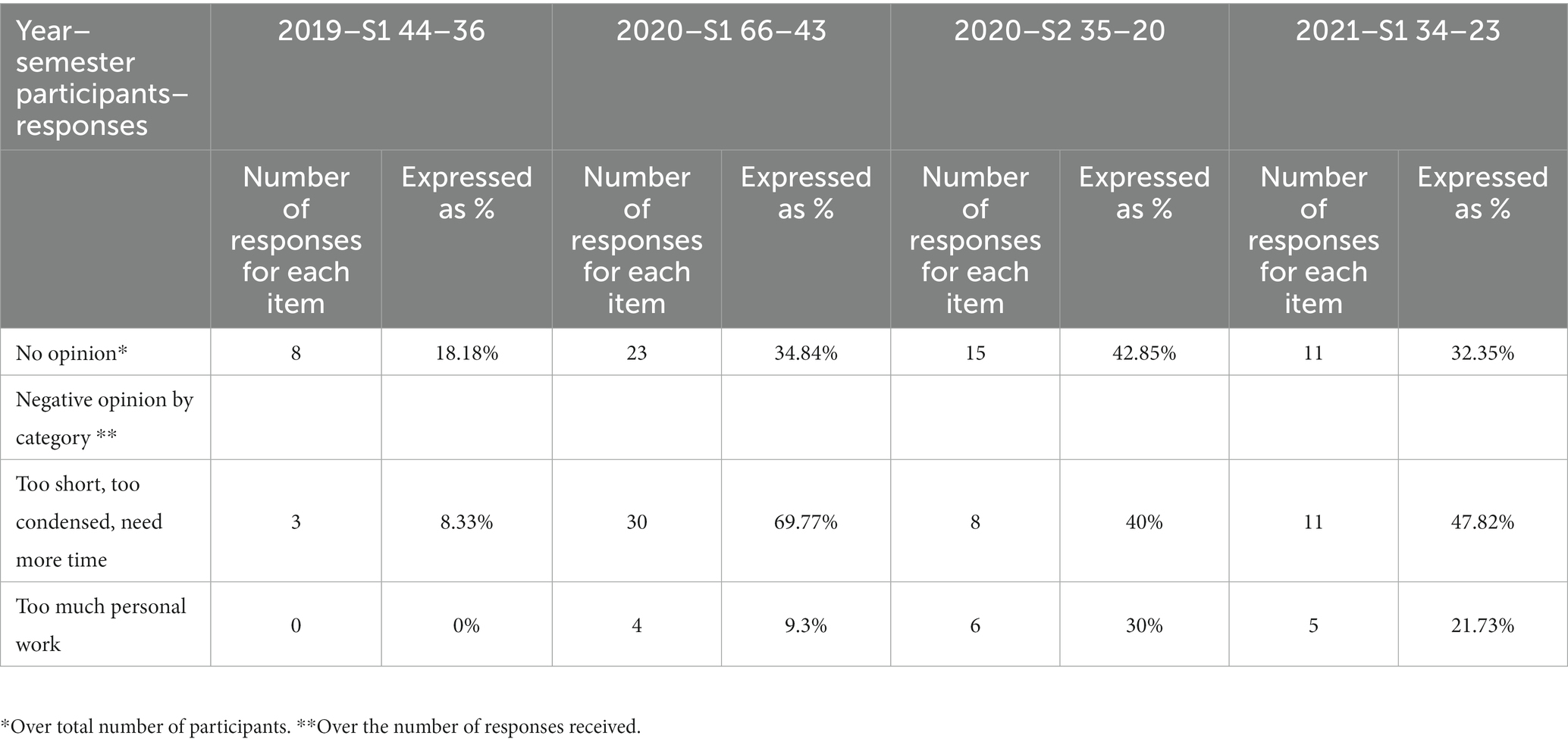
Table 5. Analysis of the contents on the negative aspects of the program, number of responses and percentages for the program delivered (only on items related to our topic).
4.5. Contributions of neuroscience to the intervention program
These results seem to show that the training program improves procrastination and also planning. But we can ask whether it is sufficient to only emphasize tools and methods or if a combination with education about neurobiological aspects does indeed improve the training. There is a relevant element toward the latter effect: it is the testimony of the students (Table 6), which reveals that the theoretical neurobiological data cannot and must not be dissociated from the methods and tools employed because they help to make sense of the methods and tools, bring scientific credibility and assist with an understanding of one’s own behaviors.
4.6. Limitations of the present study and research perspectives
Our study has a number of limitations. First, the study was undertaken in parallel with a university-wide intervention seeking to improve student academic success. Some constraints were imposed by the university, namely the size of groups. Next, the assessment of procrastination behavior is based on self-assessment, having the potential for bias by way of social desirability. In addition, we did not collect data on student grades so could not assess any effect of the program on academic achievement. Finally, it remains unclear whether the improvements observed in procrastination behavior and planning ability are due to the procrastination sequence on its own or to the training program as a whole.
There is also the question of whether the improvement in behavior and executive function remains (here procrastination and planning). The interview of the students who followed the program in 2019 (Table 6) shows that 90% of students continued to use the methods and tools, which is a very positive reflection on the long-term impact of the NeuroStratE program. Nevertheless, students could have been re-tested on the degree of procrastination and the planning function in order to compare their results with the initial ones, taking into account that students will have acquired more maturity after 2 or 3 additional years spent at university.
It is also noted that even if students state that the theoretical aspects on the knowledge of brain function are necessary for a better use of the tools, in order to be sure of our results it would have been necessary to provide the training with and without the neurobiological theoretical content and to carry out the same measurements for confirmation, something which remains difficult to put in parallel with the initial program.
5. Conclusion
Our study showed that an intervention focusing on teaching the functioning of the brain and practical tools (setting objectives and time management) to control procrastination behavior (NeuroStratE; Cherrier et al., 2020) reduces procrastination behavior and improves planning ability in undergraduate students. Thus, providing training in cognitive neuroscience on the functioning of one’s brain, through metacognition and through the use of adapted and targeted tools, has an impact in improving behaviors (here procrastination), brain functions (here the planning function), but also attitude and state of mind (here self-regulation and better self-knowledge), of university students. The use of tools and methods alone seemed insufficient for students and it is the combination of the two (neurobiological theoretical knowledge and practical tools) that will enhance their outcomes.
Data availability statement
The original contributions presented in the study are included in the article/supplementary material, further inquiries can be directed to the corresponding author.
Ethics statement
The studies involving human participants were reviewed and approved by Ethics committee of University of New Caledonia. The patients/participants provided their written informed consent to participate in this study.
Author contributions
SC wrote the article. GW processed the data. SF and GB proofread and corrected. All authors contributed to the article and approved the submitted version.
Acknowledgments
We would like to thank the students from the University of New Caledonia for their willingness to collaborate in this work. We would also like to thank Adjunct Associate Professor Daniel Nicholls (Western Sydney University, Australia) for his help with the English transcript.
Conflict of interest
The authors declare that the research was conducted in the absence of any commercial or financial relationships that could be construed as a potential conflict of interest.
Publisher’s note
All claims expressed in this article are solely those of the authors and do not necessarily represent those of their affiliated organizations, or those of the publisher, the editors and the reviewers. Any product that may be evaluated in this article, or claim that may be made by its manufacturer, is not guaranteed or endorsed by the publisher.
References
Baker-Sennett, J., Matusov, E., and Rogoff, B. (1993). Planning as developmental process. Adv. Child Dev. Behav. 24, 253–281. doi: 10.1016/S0065-2407(08)60305-5
Bandura, A., and Locke, E. A. (2003). Negative self-efficacy and goal effects revisited. J. Appl. Psychol. 88, 87–99. doi: 10.1037/0021-9010.88.1.87
Ben-Shachar, M., Lüdecke, D., and Makowski, D. (2020). Effectsize: estimation of effect size indices and standardized parameters. J. Open Source Softw. 5:2815. doi: 10.21105/joss.02815
Borys, S. V., Spitz, H. H., and Dorans, B. A. (1982). Tower of Hanoi performance of retarded young adults and nonretarded children as a function of solution length and goal state. J. Exp. Child Psychol. 33, 87–110. doi: 10.1016/0022-0965(82)90008-X
Bytamar, J. M., Saed, O., and Khakpoor, S. (2020). Emotion regulation difficulties and academic procrastination. Front. Psychol. 11:524588. doi: 10.3389/fpsyg.2020.524588
Cherrier, S., Le-Roux, P.-Y., Gérard, F.-M., Wattelez, G., and Galy, O. (2020). Impact of a neuroscience intervention (NeuroStratE) on the school performance of high school students: academic achievement, self-knowledge and autonomy through a metacognitive approach. Trends Neurosci. Educ. 18:100125. doi: 10.1016/j.tine.2020.100125
Cortés Pascual, A., Moyano Muñoz, N., and Quílez Robres, A. (2019). The relationship between executive functions and academic performance in primary education: review and meta-analysis. Front. Psychol. 10:1582. doi: 10.3389/fpsyg.2019.01582
Creswell, J. W., and Plano Clark, V. L.. (2018). Designing and Conducting Mixed Methods. 3rd. New York: Sage.
Diamond, A. (2013). Executive functions. Annu. Rev. Psychol. 64, 135–168. doi: 10.1146/annurev-psych-113011-143750
Diamond, A., and Ling, D. S. (2016). Conclusions about interventions, programs, and approaches for improving executive functions that appear justified and those that, despite much hype, do not. Dev. Cogn. Neurosci. 18, 34–48. doi: 10.1016/j.dcn.2015.11.005
Diamond, A., and Ling, D. S. (2020). “Review of the evidence on, and fundamental questions about, efforts to improve executive functions, including working memory” in Cognitive and Working Memory Training: Perspectives from Psychology, Neuroscience, and Human Development. eds. J. M. Novick, M. F. Bunting, M. R. Dougherty, and R. W. Engle (Oxford: Oxford University Press), 143–431.
Doran, G. T. (1981). There’s s.M.a.R.T. way to write managements’s goals and objectifs. Manag. Rev. 70:35.
Eckert, M., Ebert, D. D., Lehr, D., Sieland, B., and Berking, M. (2016). Overcome procrastination: enhancing emotion regulation skills reduce procrastination. Learn. Individ. Differ. 52, 10–18. doi: 10.1016/j.lindif.2016.10.001
Ferrari, J. R., Johnson, J. L., and McCown, W. G.. (1995). Procrastination and Task Avoidance: Theory, Research, and Treatment. Berlin, Germany: Springer Science and Business Media.
Giesbrecht, F. G., and Burns, J. C. (1985). Two-stage analysis based on a mixed model: large-sample asymptotic theory and small-sample simulation results. Biometrics 41:477. doi: 10.2307/2530872
Graybiel, A. M. (2008). Habits, rituals, and the evaluative brain. Annu. Rev. Neurosci. 31, 359–387. doi: 10.1146/annurev.neuro.29.051605.112851
Grunschel, C., Patrzek, J., Klingsieck, K. B., and Fries, S. (2018). “I’ll stop procrastinating now!” fostering specific processes of self-regulated learning to reduce academic procrastination. J. Prev. Interv. Community 46, 143–157. doi: 10.1080/10852352.2016.1198166
Gunzenhauser, C., and Nückles, M. (2021). Training executive functions to improve academic achievement: tackling avenues to far transfer. Front. Psychol. 12:1350. doi: 10.3389/fpsyg.2021.624008
Howard-Jones, P. A., Varma, S., Ansari, D., Butterworth, B., De Smedt, B., Goswami, U., et al. (2016). The principles and practices of educational neuroscience: comment on bowers (2016). Psychol. Rev. 123, 620–627. doi: 10.1037/rev0000036
Hrong-Tai Fai, A., and Cornelius, P. L. (1996). Approximate F-tests of multiple degree of freedom hypotheses in generalized least squares analyses of unbalanced split-plot experiments. J. Stat. Comput. Simul. 54, 363–378. doi: 10.1080/00949659608811740
Johnson, R. B., and Onwuegbuzie, A. J. (2004). Mixed methods research: a research paradigm whose time has come. Educ. Res. 33, 14–26. doi: 10.3102/0013189X033007014
Kalivas, P. W., and Volkow, N. D. (2005). The neural basis of addiction: a pathology of motivation and choice. Am. J. Psychiatr. 162, 1403–1413. doi: 10.1176/appi.ajp.162.8.1403
Kim, K. R., and Seo, E. H. (2015). The relationship between procrastination and academic performance: a meta-analysis. Personal. Individ. Differ. 82, 26–33. doi: 10.1016/j.paid.2015.02.038
Kirillov, A. V., Tanatova, D. K., Vinichenko, M. V., and Makushkin, S. A. (2015). Theory and practice of time-Management in Education. Asian Soc. Sci. 11:p193. doi: 10.5539/ass.v11n19p193
Kuznetsova, A., Brockhoff, P. B., and Christensen, R. H. B. (2017). lmerTest package: tests in linear mixed effects models. J. Stat. Softw. 82, 1–26. doi: 10.18637/jss.v082.i13
Lawlor, K. B., and Hornyak, M. J.. (2012). Smart Goals: How the Application of Smart Goals can Contribute to Achievement of Student Learning Outcomes. Developments in Business Simulation and Experiential Learning: Proceedings of the Annual ABSEL Conference, No. 39. Available at: https://absel-ojs-ttu.tdl.org/absel/index.php/absel/article/view/90
Lay, C. H. (1986). At last, my research article on procrastination. J. Res. Pers. 20, 474–495. doi: 10.1016/0092-6566(86)90127-3
Lenth, R. V., Buerkner, P., Herve, M., Love, J., Riebl, H., and Singmann, H.. (2021). Emmeans: Estimated Marginal Means, Aka Least-Squares Means (1.7.0) [Computer Software]. Available at: https://CRAN.R-project.org/package=emmeans
Lezak, M. D. (1982). The problem of assessing executive functions. Int. J. Psychol. 17, 281–297. doi: 10.1080/00207598208247445
Locke, E. A., and Latham, G. P. (2006). New directions in goal-setting theory. Curr. Dir. Psychol. Sci. 15, 265–268. doi: 10.1111/j.1467-8721.2006.00449.x
Mann, L. (1982). “The decisional procrastination scale” in Procrastination and Task Avoidance: Theory, Research and Treatment. eds. J. R. Ferrari, J. L. Johnson, and W. McCown (New York: Plenum Press), 71–96.
Martinot, D. (2001). Connaissance de soi et estime de soi: Ingrédients pour la réussite scolaire. Rev. Sci. Educ. 27, 483–502. doi: 10.7202/009961ar
Mayring, P. (2000). Qualitative content analysis. Forum Qual. Soc. Res. 1:1089. doi: 10.17169/FQS-1.2.1089
McNiff, J.. (2013). Action Research: Principles and Practice. London: Routledge and CRC Press. Available at: https://www.routledge.com/Action-Research-Principles-and-practice/McNiff/p/book/9780415535267
Miyake, A., Friedman, N. P., Emerson, M. J., Witzki, A. H., Howerter, A., and Wager, T. D. (2000). The Unity and Diversity of executive functions and their contributions to complex “frontal lobe” tasks: a latent variable analysis. Cogn. Psychol. 41, 49–100. doi: 10.1006/cogp.1999.0734
Morlaix, S., and Perret, C. (2013). L’évaluation du Plan Réussite en Licence: Quelles actions pour quels effets? Analyse sur les résultats des étudiants en première année universitaire. Rech. Éduc. 15:15. doi: 10.4000/ree.7390
O’Brien, W. K. (2002). Applying the Transtheoretical Model to Academic Procrastination. 62. Ann Arbor: ProQuest Information and Learning, 5359.
Osiurak, F., Faure, J., Thomas, R., Morange, D., Dumet, N., Tapiero, I., et al. (2015). Predictors of academic procrastination: self-determined motivation, self-esteem and degree of maximization. Prat. Psychol. 21, 19–33. doi: 10.1016/j.prps.2015.01.001
Ozer, B. U., Demir, A., and Ferrari, J. R. (2009). Exploring academic procrastination among Turkish students: possible gender differences in prevalence and reasons. J. Soc. Psychol. 149, 241–257. doi: 10.3200/SOCP.149.2.241-257
Pychyl, T. A., and Sirois, F. M. (2016). “Procrastination, emotion regulation, and well-being” in Procrastination, Health, and Well-being. eds. F. M. Sirois and T. A. Pychyl (Cambridge, Massachusetts: Elsevier Academic Press), 163–188.
R Core Team . (2021). R: The R Project for Statistical Computing. Available at: https://www.r-project.org/ (Accessed October 30, 2021).
Robert, A. D., and Bouillaguet, A. (2007). L’analyse de Contenu [Content Analysis]. Paris: Presses Universitaires de France.
Schaalje, G. B., McBride, J. B., and Fellingham, G. W. (2002). Adequacy of approximations to distributions of test statistics in complex mixed linear models. J. Agric. Biol. Environ. Stat. 7, 512–524. doi: 10.1198/108571102726
Scholnick, E. K., and Friedman, S. L. (1987). “The planning construct in the psychological literature” in Blueprints for Thinking: The Role of Planning in Cognitive Development. eds. S. L. Friedman, E. K. Scholnick, and R. R. Cocking (Cambridge: Cambridge University Press), 3–38.
Schouwenburg, H. C. (2004). Counseling the Procrastinator in Academic Settings. 1st. Washington, DC: American Psychological Association.
Schreier, M., Stamann, C., Janssen, M., Dahl, T., and Whittal, A. (2019). Qualitative content analysis: conceptualizations and challenges in research practice—introduction to the FQS special issue qualitative content analysis I. Forum Qual. Soc. Res. 20:3393. doi: 10.17169/FQS-20.3.3393
Simon, H. A. (1975). The functional equivalence of problem-solving skills. Cogn. Psychol. 7, 268–288. doi: 10.1016/0010-0285(75)90012-2
Smith, K. S., and Graybiel, A. M. (2013). A dual operator view of habitual behavior reflecting cortical and striatal dynamics. Neuron 79, 361–374. doi: 10.1016/j.neuron.2013.05.038
Solomon, L. J., and Rothblum, E. D. (1984). Academic procrastination: frequency and cognitive-behavioral correlates. J. Couns. Psychol. 31, 503–509. doi: 10.1037/0022-0167.31.4.503
Steel, P. (2007). The nature of procrastination: a meta-analytic and theoretical review of quintessential self-regulatory failure. Psychol. Bull. 133, 65–94. doi: 10.1037/0033-2909.133.1.65
Steel, P. (2010). Arousal, avoidant and decisional procrastinators: do they exist? Personal. Individ. Differ. 48, 926–934. doi: 10.1016/j.paid.2010.02.025
Steel, P., and Klingsieck, K. B. (2016). Academic procrastination: psychological antecedents revisited. Aust. Psychol. 51, 36–46. doi: 10.1111/ap.12173
St-Laurent, D., and Moss, E. (2002). Le développement de la planification: influence d’une activité conjointe. [the development of planning: influence of a joint activity]. Enfance 54, 341–361. doi: 10.3917/enf.544.0341
Thomas, M. S. C., Ansari, D., and Knowland, V. C. P. (2019). Annual research review: educational neuroscience: progress and prospects. J. Child Psychol. Psychiatry 60, 477–492. doi: 10.1111/jcpp.12973
Welsh, M. C. (1991). Rule-guided behavior and self-monitoring on the tower of Hanoi disk-transfer task. Cogn. Dev. 6, 59–76. doi: 10.1016/0885-2014(91)90006-Y
Zacks, S., and Hen, M. (2018). Academic interventions for academic procrastination: a review of the literature. J. Prev. Interv. Community 46, 117–130. doi: 10.1080/10852352.2016.1198154
Keywords: procrastination, executive functions, planning, cognitive neuroscience training, innovative learning strategies, brain knowledge
Citation: Cherrier S, Wattelez G, Ferrière S and Borst G (2023) NeuroStratE: An educational neuroscience intervention to reduce procrastination behavior and improve executive planning function in higher students. Front. Educ. 8:1149817. doi: 10.3389/feduc.2023.1149817
Edited by:
Lawrence M. Parsons, The University of Sheffield, United KingdomReviewed by:
Tingyong Feng, Southwest University, ChinaLuis Alberto Furlan, National University of Cordoba, Argentina
Copyright © 2023 Cherrier, Wattelez, Ferrière and Borst. This is an open-access article distributed under the terms of the Creative Commons Attribution License (CC BY). The use, distribution or reproduction in other forums is permitted, provided the original author(s) and the copyright owner(s) are credited and that the original publication in this journal is cited, in accordance with accepted academic practice. No use, distribution or reproduction is permitted which does not comply with these terms.
*Correspondence: Sophie Cherrier, sophie.cherrier@unc.nc