- 1Department of Marketing, University of Georgia, Athens, GA, United States
- 2Department of Marketing, George Washington University, Washington, DC, United States
- 3Yahoo, Sunnyvale, CA, United States
The COVID-19 pandemic has led to tremendous disruptions in people’s everyday activities, including the pursuit of education. Internet search data may provide insights into potential audiences’ interest in online education. Using Internet search data, we examined the impact of COVID-19 on people’s interest in supplementary online education in the US over nine months (10/14/2019–07/19/2020). We found there was increased interest in supplementary online education after WHO announced COVID-19 as a pandemic, with a greater increase among females than males. We found that the increased interest in online education persisted after the stay-at-home orders were lifted; in addition, we identified concerns over unemployment as a key variable that significantly explained the variance in the interest in online education, even after controlling for COVID cases and deaths. Policymakers and online education platforms may take advantage of people’s, especially women’s increased interest in online education when designing policies or marketing mix.
1. Introduction
In 2020, the outbreak of the COVID-19 pandemic disrupted social and economic life around the world. The World Health Organization (WHO) declared COVID-19 a global pandemic on March 11, 2020, and the Centers for Disease Control and Prevention (CDC) recommended social distancing in March (Ghebreyesus, 2020). In March and April 2020, most states in the US issued stay-at-home orders in response to the pandemic (Lee, 2020), most of which were enforced till early June (USA Today, 2021). The COVID-19 pandemic, as a highly contagious disease, has significantly changed education. The need for social distancing posed a barrier for education that involved face-to-face instructions and physical contact. Meanwhile, unemployment rates kept rising and reached a record high (Long and Van Dam, 2020), potentially led to more demands for education in order to succeed on the job market. Thus, the pandemic may provide an opportunity for supplementary online education, i.e., online education for professional development that can be pursued voluntarily by individuals of different ages and backgrounds. In addition, women had been hurt disproportionally by the economic situation during the pandemic (Connley, 2020a) and the unemployment rate was higher among women (United Nations, 2020). Since education provides an opportunity to lower the risk of unemployment (Gray et al., 2012), women may seek education for professional development in times when they are disproportionally threatened by unemployment. Thus, it is crucial to investigate the interest in supplementary online education during the pandemic, and the gender heterogeneity.
In this article, we focused on people’s interest in online education for supplementary learning opportunities (i.e., not school classes), as well as the related gender heterogeneity. We used online search volume to track and measure the interest among potential audiences, which complements existing approaches that study online education (see the Literature Review Section).
We obtained weekly search intensity of online-education-related queries between October 14, 2019 and July 19, 2020.1 WHO declared COVID-19 a pandemic on March 11, 2020, and hence our data covered the time periods before and after the outbreak of the pandemic. Further, we obtained search queries related to unemployment concerns, and compared the search intensity of online education with that of unemployment.
With this method, we documented a dramatic increase (52.7%) in searches of online education after the outbreak. Females demonstrated a greater increase in searches of online education compared to males (127.3% more than males). The above effects persisted after the stay-at-home orders were lifted. Combining the search intensity of unemployment concerns and search intensity of online education, and utilizing a difference-in-differences (DID) analysis, we provided suggestive evidence on a mechanism: COVID-19 increased concerns over employment, which may in turn result in greater interest in online education, especially among females.
The findings have multiple implications for industry practitioners. First, we establish online search volume as a metric to measure interest among potential consumers of online education. Compared with previous studies that examined students who had already participated in online education, this metric captures the future potential and serves as a quick capture of population-level interest in online education. The method – using online search to track interest in online education – also complements previous research methods. Compared with case studies, our method provides overall estimations without focusing too much on a specific platform or class category (e.g., STEM). Compared with survey or interview, this method avoids recall bias or social concerns (see the literature review section for details.) Second, the research on gender heterogeneity may assist platforms in assessing the potential growth in the female segment during COVID-19 and constructing courses to accommodate the segment’s varied needs. Third, the relationship between gender and unemployment concerns also provides unique implications on why COVID-19 leads to differential interest in online education and how industry practitioners could leverage the public crisis to grow.2
In the rest of the paper, we provide literature review, introduce our data and analysis, present model results, and discuss the implications, originality, and future research.
2. Literature review
2.1. Growth in online education during the pandemic
The COVID-19 pandemic has created significant challenges for education globally. “94% of learners worldwide were affected by the pandemic” (Ulukol, 2022). At the international level, educational providers moved education online (Crawford et al., 2020), which was further confirmed by studies in various countries such as Romania (Boca, 2021; Butnaru et al., 2021), Turkey (Cinar et al., 2021; Dindar et al., 2022), India (Chakraborty et al., 2021), Bulgaria (Ivanova, 2021), China (Feng et al., 2021; Liang et al., 2022), Saudi Arabia (AlMatham et al., 2022), Korea (Herr et al., 2021), Uganda (Damani et al., 2022) and Russia (Sushko and Pronchev, 2021). In general, online education has gained popularity during COVID-19 (Radha et al., 2020).
Supplementary online education (SOE) experienced great growth right after the breakout of COVID-19 (e.g., Shah, 2020; Impey and Formanek, 2021; Choudhuri and Dilip Kumar, 2022). For example, industry reports showed that Coursera experienced a 640% increase in enrollment from mid-March to mid-April in 2020 compared to the same period in 2019 after it decided to make all courses free through May 2020 (Shah, 2020); Udemy’s enrollment increased by 400% in February and March (Shah, 2020). Using a multiple case study approach, Impey and Formanek, 2021 found enrollment surged in three massive open online astronomy classes within 100 days after the breakout of COVID-19. Many supplementary education platforms responded to the pandemic by reducing or waiving fees on thousands of offerings (McCluskey, 2020), which in part drove the enrollment surges on these platforms.
However, the documented surges of online education were typically based on enrollment or revenue metrics, which were heavily affected by (1) the heavy price promotions run by online education platforms during COVID-19 and (2) the stay-at-home (i.e., lockdown) orders. Thus, the growth metrics in previous studies (e.g., enrollment and revenue) cannot isolate the interest in online education from external factors (e.g., price promotions, lockdown orders). Instead, our study examined consumer interest in online education, measured by online search volume; it offered a metric to evaluate the growth of the potential consumer base, rather than the observed enrollment. Thus, our approach complements existing methods with a focus on the interest among potential (rather than existing) consumers.
2.2. Gender, online education, and employment status
Ng (2019) called for more research to address diverse online learners’ needs. Past research on gender diversity in online education has mainly focused on (1) learning and interaction styles and (2) course completion, with case studies, surveys or interviews. Women employed more self-regulated learning strategies (Li, 2019; Liu et al., 2021), and posted more comments in the discussion forum (Caspi et al., 2008; Shi et al., 2020). Using a case study, Christensen et al. (2013) found that women were less likely to take massive open online courses (MOOCs). Based on the data from over 67,000 learners in 20 MOOCs, Kizilcec and Halawa (2015) concluded that women (vs. men) were less likely to persist in courses. Women were also shown to be more likely to withdraw because of the conflicts between their caregiving responsibilities and student roles (McGivney, 2004; Müller, 2008; Littenberg-Tobias and Reich, 2020). Even for women who completed MOOCs, Veletsianos et al. (2021) reported that they were more inconsistent in their learning time. In contrast, a few studies did not support the association between gender and online course completion (e.g., Morris et al., 2015; Shrader et al., 2016; Jiang et al., 2018). These inconclusive findings typically focused on a specific course or a few courses in one platform, which did not take an overall perspective. Also, they focused on students who had enrolled in the online platform. These studies were not on the interest in online education among potential audiences, we fill this gap using Internet search data.
Past research also showed unemployment was significantly associated with learners’ persistence and content coverage in MOOCs, with unemployed learners completing more content (Cisel, 2014; Morris et al., 2015). Cisel (2014) also found that unemployed learners set a higher learning target and covered more content than students. Since people go back to school during times of high unemployment (Kahn, 2010) and one of the reasons people take SOE is to prepare for their future careers (Liu et al., 2015), unemployment may motivate people to pursue education. During the pandemic, SOE was used extensively to supplement university education (e.g., Jiang et al., 2022), to train postgraduates and teachers (e.g., Bulvinska and Kapralova, 2022; Ponnaiah et al., 2022), to teach various topics such as English (e.g., Wang, 2022) and technology (e.g., Saikat et al., 2022), or even used as an alternative for internship (e.g., Singh and Sharma, 2021), and thus may be an effective tool to prepare people for the future job market.
Despite the insights on the gender differences in course completion and learning styles and the relationship between unemployment and learners’ motivation, little has been done to jointly examine the interplay between COVID-19, gender, and unemployment. However, women had been hurt disproportionally by the economic situation during the pandemic (Connley, 2020a) and the unemployment rate was higher among women (Carli, 2020; United Nations, 2020; Connley, 2020a). This disproportional impact still held when states started to reopen in June 2020, with women’s unemployment rate 1.3 times higher than the unemployment rate women faced during the Great Recession (Connley, 2020b); it was claimed that COVID-19 had created the first American’s female recession (Carrazana, 2020). Education, as a way to empower oneself to be more competitive in future job market, may be in a greater demand during the pandemic, especially among women.
Our study fills multiple gaps and complements existing studies. First, instead of learning styles or completion rates, we investigated gender differences in the interest in online education. Second, we investigated how the gender difference in unemployment rates during COVID-19 may explain females’ greater increase in the interest in online education.
2.3. Research methods for online education
Most previous research on online education relied on surveys, interviews, or case studies. Although subjective measures are better suited to understand the underlying psychology, these measures may also be subject to recall bias or social concerns (Thompson and Phua, 2005, Kim et al., 2018). Survey participants may be unwilling to disclose their real attitude due to legal or social concerns (Thompson and Phua, 2005, Kim et al., 2018); for instance, when asked about interest in getting educated, people may state that they were interested even if they were not. Case studies may involve only a few courses in one platform (e.g., Impey and Formanek, 2021) and may not cover the overall interest in online education across the society.
To complement the above methods, we used search queries from a large search engine3 as a measure of males’ and females’ interest in online education. Online data has been increasingly used as a measure of human behavior in multiple domains. For example, Twitter users’ sentiments have been used to identify the challenges of remote work (Saura et al., 2022); mobile data has been used to track COVID-19 infection (for a review, see Ribeiro-Navarrete et al., 2021). Specific to the use of search data, Bento et al. (2020) used search volume of “coronavirus”-related terms to examine the public’s information-seeking responses to news of local COVID-19 cases. Marketing studies have used the search volume of a product to measure consumers’ purchase interest (Kim and Hanssens, 2017; Wang et al., 2019). Finance studies have used the search volume of a firm’s stock symbol to measure investors’ interest in the stock (Da et al., 2011; deHaan et al., 2015). As far as we know, we are the first to use search volume as a metric to measure people’s interest in online education. The method circumvents the recall or social concern biases of surveys, interviews or case studies. In addition, previous studies on online education focused on students who had enrolled in education platforms, which were only a fraction of all potential students with probable selection bias; whereas our metric can provide insights into the whole potential consumer base of online education.
3. Data and analysis
3.1. Data
We collaborated with a large search engine company headquartered in the US and used searches of online-education-related queries as an approximation of people’s interest in online education. Prior research indicated that web search volume can be a good proxy for the level of interest in a subject (Wang et al., 2019; Bento et al., 2020). We obtained weekly (using Monday as the start of a week) data of online education search intensity between October 14, 2019 and July 19, 2020.
To start, we first obtained a dictionary of keywords related to online education from a variety of resources, including names of top online learning websites/platforms,4 popular online courses,5 and common terms related to online education and learning.6 We excluded terms related to kid education (specifically, “kid,” “kids,” “child,” “children,” “k12,” “k-12,” “pre-k,” and “pre-k”) to avoid the issue that people may search for online education for their kids rather than for themselves. We collected searches of queries that contain keywords in the dictionary, and thus obtained the search volume of the queries related to online education.
To investigate the heterogeneous effect of different demographic groups, we divided the data into two gender groups (males and females) and five age groups (24 yr or below, 25 to 34 yr, 35 to 49 yr, 50 to 64 yr, and 65 yr. or above), and hence demographic groups in total. We obtained the weekly search volume of queries related to online education for each of the 10 demographic groups.7
Finally, for each demographic group defined by gender and age, we calculated search intensity of online education as the share of the search queries of online education out of all searches during the week. The search intensity data was further scaled so that the largest number was 100. The calculation is the industry standard and is similar to Google Trend (Jun et al., 2018). See Web Appendix A, Table A1 for summary statistics.
3.2. Model-free analyses
We present model-free analyses in Figures 1A,B, which depict the overall weekly search intensity of online education in 2019 and 2020, and the metrics for males and females separately.
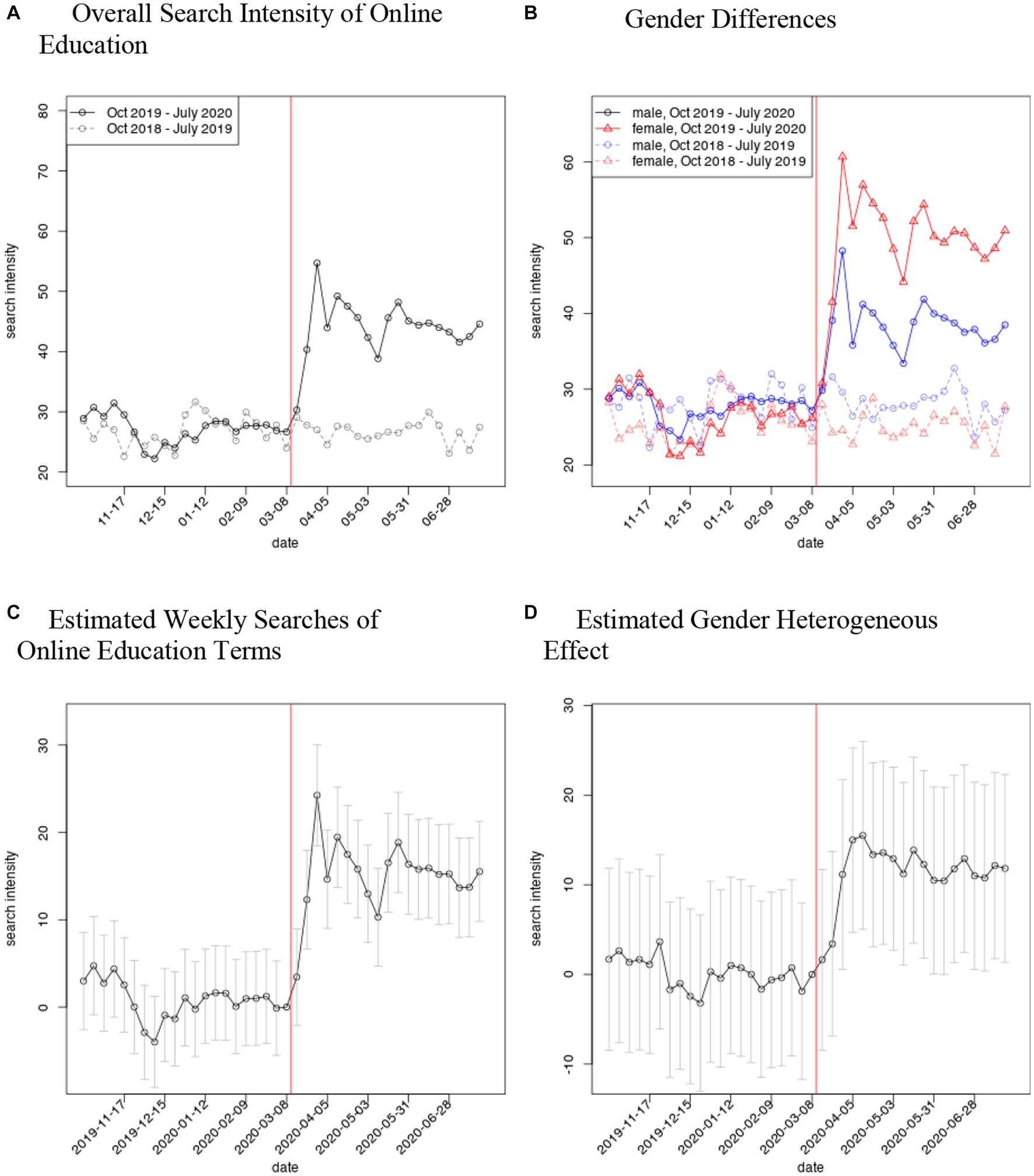
Figure 1. (A) and (B) depict model-free results: weekly search intensity of online education in 2020 (solid line) and 2019 (dashed line). (A) depicts the search intensity of online education in 2020 (solid line) and 2019 (dashed line). (B) depicts the search broken down by gender (with males represented by blue round dots, and females represented by red triangle dots). (C) and (D) depict DID model results. (C) depicts the estimated , the coefficient of each week indicator as in Model (1). It shows that there was a significant increase in searches of online education after the week of March 11, 2020. (D) depicts the estimated , the coefficient of the interaction of week indicator and female indicator as in Model (3). It shows that females demonstrated greater increases in searches of online education than males after the week of March 11, 2020. The vertical red line indicates the date of March 11, 2020. The grey bars represent the 95% confidence intervals. Figures (A) and (B) include data from both Oct 2018 – July 2019 and Oct 2019 – July 2020, and, thus, we do not include the year in the dates under the x-axis.
4. Empirical analyses and results
4.1. Impact of COVID-19 on interest in online education
To investigate how people’s interest in online education changed during the pandemic, we controlled for people’s age and gender and estimated search intensity of online education for each week in Model (1):
is the search intensity in week t among users of age group i and gender j. The dependent variable (DV) is the search intensity (i.e., the proportions of the search volume of online-education-related queries out of all searches).8
The age group index i takes five values (0 to 4) corresponding to the five age groups as described in the Data section, with the age group 24 years old or below as the baseline. The gender index j takes values 0 (males) and 1 (females); we used males as the baseline and define the dummy variable if . Hence, we controlled for gender and age group fixwed effects with the terms and .
is the week indicator, where k indexes each week. The WHO declared COVID-19 as a pandemic on March 11, 2020. Because March 11 was a Wednesday, we used the week of March 2–8, 2020 (the week before WHO declared COVID-19 as a pandemic) as the baseline. Hence, the coefficient captures search intensity of online education relative to the baseline week. We plotted the estimated and the corresponding confidence intervals in Figure 1C, which shows significantly higher search intensity of online education as the pandemic progresses.
To demonstrate the magnitude of the increase in search intensity of online education, we further estimated a model with a binary variable to replace the week indicators, as in Model (2):
is a binary variable which equals 0 for the weeks in the pre-pandemic period (before March 11, 2020) and 1 otherwise (the week of March 11, 2020 or after). Hence its coefficient, , captures the change in search intensity of online education after the outbreak of COVID-19 compared to the pre-pandemic period. Column (1) of Table 1 shows results of Model (2). Comparing the coefficient in Model (2) with the pre-pandemic average search intensity of online education (i.e., on or before March 08, 2020), we found that search intensity of online education increased by 52.7% (95% CI, 47.7 to 57.7%) after the outbreak.9
4.2. Gender heterogeneous effect
We then examined the differential effect among females and males. We incorporated the interaction of week indicators and female indicator in Model (1), again with the week of March 2–8, 2020 (the week before WHO declared COVID-19 as a pandemic) as the baseline, as in Model (3).
The coefficient captures whether there was a greater increase in search intensity of online education among females than males with the progress of the pandemic. We plotted the estimated and the corresponding confidence intervals in Figure 1D, which shows that females had significantly more increased searches of online education than males during the pandemic.
Again, to demonstrate the magnitude of gender heterogeneity, we further estimated a model with a binary variable to indicate the time period after WHO’s announcement of pandemic and used the variable to replace the week indicators, as in Model (4).
The coefficient captures whether there was a greater increase in search intensity of online education among females than males after the outbreak of the pandemic. Column (2) of Table 1 shows the results. The coefficient is positive and significant, which shows that the increased searches of online education among females were significantly higher compared to males during the pandemic. Since we used males as the baseline, the coefficient captures the increased searches among males during the pandemic. Calculating the ratio of and , we found that compared to males, the increased search intensity was 127.3% (95% CI, 85.7 to 187.2%) higher among females during the pandemic.
We conducted additional analyses. We ruled out the seasonality effect by conducting similar analyses using data of the previous year (October 2018–July 2019). See Web Appendix B, Table B1, Columns (1) and (2) respectively. We also used US COVID-19 cases and deaths data from CDC as predictors (details in Web Appendix C) and demonstrated that searches of online education and the related gender heterogeneity were related to the severity of COVID-19, as reported in Columns (3) and (4) of Table 1. We further examined the gender heterogeneity among each age group separately and found statistically significant gender heterogeneity in every age group below 64 years old (details in Web Appendix E). In Web Appendix D, we presented an analysis in which we split the time after WHO declaring pandemic into a lockdown phase (March 9–May 31, 2020) when stay-at-home orders were implemented in most US states, and a “new normal” phase (on or after June 1st, 2020) when most lockdown orders were lifted. The results indicated that the increased interest in online education persisted after the stay-at-home orders were lifted.
5. Potential mechanism: concerns over unemployment
A potential explanation of the increased interest in online education and the higher increase among females may be the increased concerns over unemployment during the pandemic, especially among women. The pandemic led to outrageously high unemployment rate and women had been hurt disproportionally (Carli, 2020; United Nations, 2020; Connley, 2020a). It was claimed that COVID-19 had created the first American’s female recession (Carrazana, 2020). Education, as a way to empower oneself to be more competitive in future job market, may be in a greater demand during the pandemic, especially among women, who had been hurt disproportionally.
To examine concerns over unemployment, we obtained the search intensity of unemployment-related terms. Similar to search intensity of online education, search intensity of unemployment-related terms was calculated as the ratio of the search volume of queries related to unemployment (e.g., “unemployment” and “layoff”) and the whole search volume, and was further scaled so that the largest number is 100. We plotted the model-free trends of search intensity of unemployment-related terms in Figure 2. Figure 2A depicts search intensity of unemployment-related terms in general, and Figure 2B depicts search intensities of unemployment-related terms from males and females. Comparing Figures 1, 2, we found the trends of search intensity of unemployment-related terms coincided with those of search intensity of online education.10
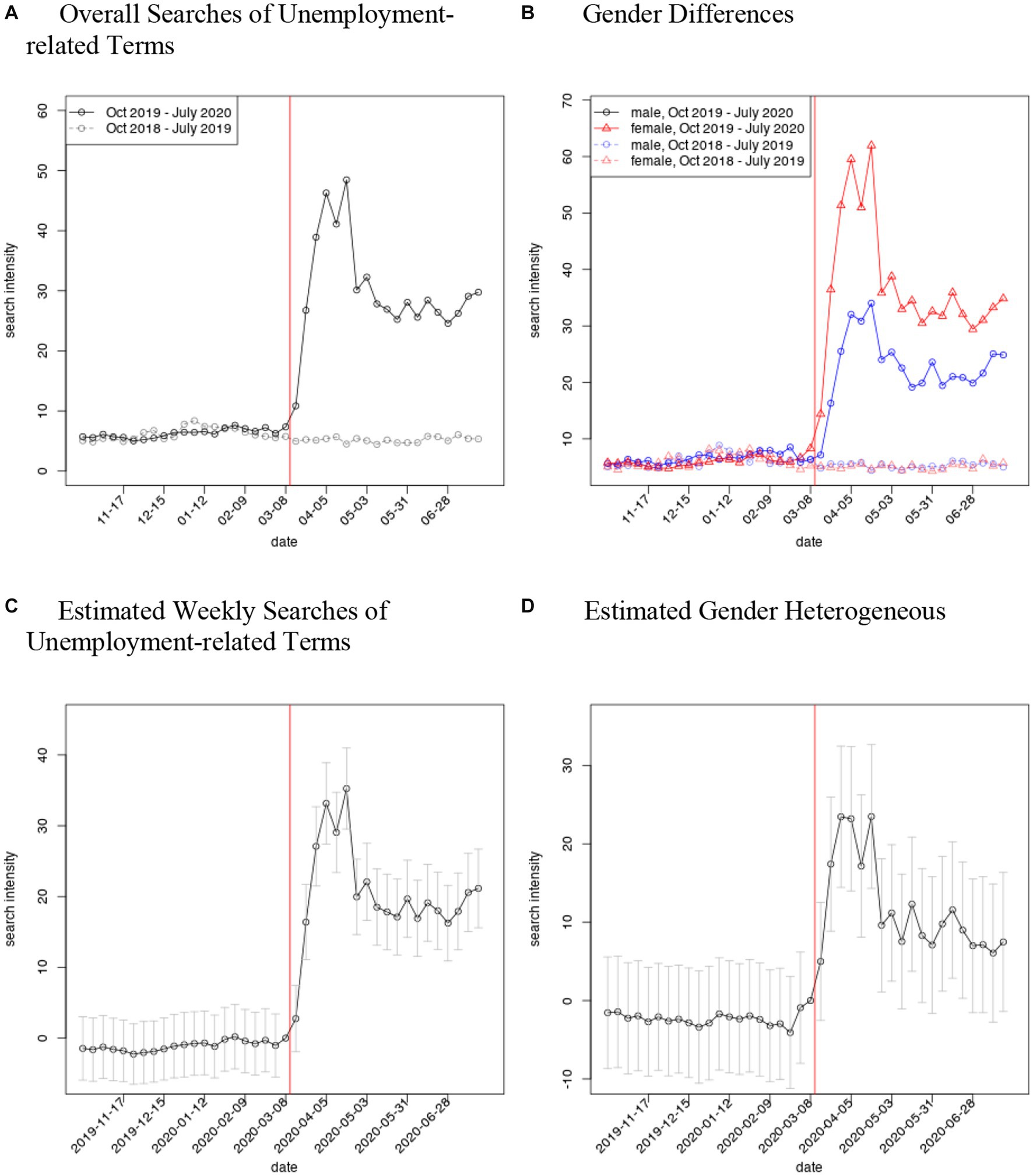
Figure 2. (A) and (B) depict model-free results: weekly search intensity of unemployment-related terms in 2020 (solid line) and 2019 (dashed line). (A) depicts the search intensity of unemployment-related terms in 2020 (solid line) and 2019 (dashed line). (B) further depicts the search broken down by gender (with males represented by blue round dots, and females represented by red triangle dots). (C) and (D) depict DID model results: (C) depicts the estimated , the coefficient of the week indicator in Model (1) with search intensity of unemployment-related terms as DV. It shows that there was a significant increase in searches of unemployment-related terms after the week of March 11, 2020. (D) depicts the estimated , the coefficient of the interaction of the week indicator and female indicator as in Model (3). It shows females demonstrated greater increases in searches of unemployment-related terms than males after the week of March 11, 2020. The vertical red line indicates the date of March 11, 2020. The grey bars represent the 95% confidence intervals. Figures (A) and (B) include data from both Oct 2018 – July 2019 and Oct 2019 – July 2020, and, thus, we do not include the year in the dates under the x-axis.
5.1. Impact of COVID-19 on concerns over unemployment
We then estimated Models (1) and (3) with search intensity of unemployment-related terms as DV. We plotted the fitted of Model (1) (which represent the weekly search intensity of unemployment-related terms after controlling for age and gender) and of Model (3) (which represent the differential effect among females and males) in Figures 2C, D respectively. The results suggest that there were increased searches of unemployment-related terms after the outbreak of pandemic, and there were greater increases among females than males.
To demonstrate the magnitude of the effect, we again used the binary indicator to replace the week indicators, estimated Models (2) and (4) with search intensity of unemployment-related terms as DV, and presented the results in Columns (5) and (6) of Table 1. The pattern for searches of unemployment was similar to what we have observed for searches of online education: search intensity of unemployment-related terms increased significantly during the pandemic, and the increased search intensity was significantly higher among females than males. We also estimated models with search intensity of unemployment-related terms as DV and COVID-19 cases and deaths as predictors (details in Web Appendix C). We reported the results in Columns (7) and (8) of Table 1, which show that as COVID-19 cases and deaths increased, search intensity of unemployment-related terms also increased, and the increased search intensity was greater among females than males. These estimates were similar to the results of searches of online education in Table 1, which suggested unemployment concerns may potentially underlie greater searches of online education.
5.2. Testing the potential mechanism
Next, we employed the same method as in Han et al. (2022) to demonstrate the relationship between concerns over unemployment and interest in online education using a difference-in differences (DID) analysis. We referred to the period October 2019 to July 2020 as the current year and October 2018 to July 2019 the previous year. First, we matched the data from the current year with data from the previous year. We used the current year as the treatment group and the previous year as the control group, and then used a DID model to obtain the causal effect of COVID-19 on concerns over unemployment and interest in online education separately, as in Model (5).
where is a binary indicator that equals 1 for the current year (October 2019 to July 2020) and 0 for the previous year (October 2018 to July 2019), and is again the binary indicator that equals 1 if a week is in the season of pandemic, that is, between the week of March 11 and July 19 in 2019 or 2020. Thus, the coefficient captures the causal effect of COVID-19 on concerns over unemployment (using search intensity of unemployment-related terms as the DV ) and interest in online education (using search intensity of online education as the DV ). Both coefficients are positive and significant (concerns over unemployment: 21.75, p < 0.01; interest in online education: 14.80, p < 0.01).
To further test the potential mechanism of concerns over unemployment, we need to empirically establish a correlation between the concerns over unemployment and the interest in online education. For example, if we observe that for every week that concerns over unemployment increased, the interest in online education also increased, then it would suggest changes in concerns over unemployment may underlie changes in the interest in online education. We explored this potential mechanism by examining the variation of these two variables in our data across time. Specifically, we estimated the impact of COVID-19 on concerns over unemployment and interest in online education in each of the 19 weeks after the outbreak with DID models. Next, we regressed the by-week DID estimates for interest in online education on the by-week DID estimates for concerns over unemployment. We presented the results in Column (1) of Table F1 in Web Appendix F. The coefficient is positive and significant, suggesting that the two variables change in the same direction. The R-square is 0.469, which indicates that a substantial proportion of the variations of interest in online education can be explained by concerns over unemployment.
One alternative explanation for such a correlation is that the severity of the pandemic varies in different weeks. To rule out this explanation, we included the US COVID-19 new cases and deaths (per 100,000 people) in each week in the regression, and reported the results in Column (2) of Table F1 in Web Appendix F. Again, the DID estimates of concerns over unemployment are positive and significant after controlling for COVID cases and deaths. Notably, the coefficients of the COVID-19 new cases and deaths are not significant, suggesting that COVID severity no longer significantly affected the interest in online education after controlling for the DID estimates of concerns over unemployment. The result further supported our conjectured mechanism and suggested that the increase in concerns over unemployment was likely to drive the increased interest in online education.
6. Discussion and conclusion
6.1. General discussion
We show that Internet search data can be a useful tool to track and measure the interest among potential customers of online education. Using Internet search data, we provided empirical evidence that interest in online education increased dramatically after the outbreak of the COVID-19 pandemic and females (vs. males) demonstrated a greater increase in the interest in online education. We also found that the effect may be explained by unemployment concerns. While the study is in the context of COVID-19, the Internet search metric can be readily applied to future studies (see Section 6.4).
6.2. Theoretical implications
Ng (2019) called for more research to address diverse online learners’ needs. While previous research has mainly focused on gender differences in (1) learning and interaction styles and (2) course completion of already-enrolled students, we focused on interest in online education as a growth potential for the supplementary online education, especially how COVID-19 triggered the differential demand between females and males. While much previous research found females (vs. males) were less likely to enroll in (Christensen et al., 2013) or complete online courses (McGivney, 2004; Müller, 2008; Kizilcec and Halawa, 2015; Littenberg-Tobias and Reich, 2020), we found when faced with a greater unemployment during COVID-19, females (vs. males) were more interested in pursuing supplementary online education, and females’ increased interest persisted after lockdown orders were lifted. Our research, together with previous research, suggested that different factors drive females’ completion versus interest in supplementary online education. We also provided insights into the mechanism. We found that females’ increased interest in education can be explained by their greater unemployment concerns. Although past research examined the role of gender and unemployment in online education separately, we are among the first to link the two heterogeneities. Finally, with regard to methodology, past research relied on case studies or surveys to derive insights from only a few courses in one or more platforms (e.g., Impey and Formanek, 2021). This partly explains the inconclusive findings in online education, because the approach is highly sensitive to the specific course disciplines and platforms selected. Our method of using search volume data complements past research using case study and survey approaches, and allows us to take an overall view.
6.3. Managerial and practical implications
Our findings suggested that the pandemic may be a catalyst for online education. As online education becomes an increasingly important supplement to mainstream school education, online education platforms may take advantage of people’s, especially women’s increased interest in online education during the pandemic. For example, online education firms may design marketing mix to target female consumers. We also recommend governments and social workers to investigate the need for online education, especially among females, when there is a public health crisis.
6.4. Limitations and future research
There are several limitations that call for future research. First, like most of the previous literature on gender differences, we only obtained data on two genders, and the genders were inferred by the collaborating company based on the users’ online behaviors, rather than categories that users identify with. To further study the interest in online education from a gender perspective, it is necessary to obtain more detailed gender information, such as non-binary and self-identified genders.
Second, our data was correlational, and thus did not allow us to examine the causal relationship between unemployment concerns and the need for online education. More detailed Internet search data are needed to further pin down the mechanisms. Future research may use individual-level online search data or vary unemployment concerns to examine the causal relationship between the two variables.
Third, online search data can be readily applied from a global point of view, as online search is prevalent in most countries. Our search data came from the US population, and thus provided US residents’ interest in online education and related gender differences. We conjecture the specific factors that drive people, especially females, to online education (i.e., unemployment) may be similar across countries. The proposed metric and method based on Internet search data can be readily applied to other countries, and we encourage future research to compile data from various countries to derive more comprehensive insights globally.
Data availability statement
The original contributions presented in the study are included in the article/Supplementary material, further inquiries can be directed to the corresponding authors.
Author contributions
LJ and PW conceptualized the study, carried out the literature searches, wrote and revised the manuscript. All authors approved the final version of the manuscript.
Funding
The paper received support from Terry-Sanford Research Award at the Terry College of Business, University of Georgia in 2022 and 2023.
Conflict of interest
JY was employed by Yahoo.
The remaining authors declare that the research was conducted in the absence of any commercial or financial relationships that could be construed as a potential conflict of interest.
Publisher’s note
All claims expressed in this article are solely those of the authors and do not necessarily represent those of their affiliated organizations, or those of the publisher, the editors and the reviewers. Any product that may be evaluated in this article, or claim that may be made by its manufacturer, is not guaranteed or endorsed by the publisher.
Supplementary material
The Supplementary material for this article can be found online at: https://www.frontiersin.org/articles/10.3389/feduc.2023.1142689/full#supplementary-material
Footnotes
1. ^In this study, we used weekly search volume of keywords related to online education to represent people’s interest, using Monday as the start of a week. Also, we intended to obtain 4 months’ data after March 11, 2020 (i.e., after WHO declared COVID-19 a global pandemic). Thus, we used July 19, 2020, which was approximately 4 months after March 11, 2020, as the end date of the data. It was also the longest data period we could get from the collaborating company.
2. ^Our gender data are limited to two genders (male and female); also, a user’s gender is inferred from the user’s online behaviors by the collaborating company rather than a category that the user self-identifies with. See details in Section 3 and more discussions on these limitations in Section 6.
3. ^The identity of the search engine is kept anonymous as specified in the pre-agreement with the search engine.
4. ^https://www.bestcollegereviews.org/50-top-online-learning-sites/ and https://www.learnworlds.com/online-learning-platforms/
5. ^https://coursemethod.com/popular-online-courses.html
6. ^https://www.uni-prep.com/online-education/understanding-online-learning-terms/
7. ^The collaborating company collected user behaviors on its webpages and inferred the users’ genders and ages based on users’ behavioral data. It is a common practice in the industry, as described in, for example, Bi et al. (2013) and Hu et al. (2007).
8. ^Hence, the DV is an estimated proportion with sampling variance. Therefore, when estimating the model, we weigh the observations by the inverse of the standard errors associated with each observed DV, as in Saxonhouse (1976) and Hornstein (2013). The weighting applies to Models (1) to (6) in this paper.
9. ^From Table 1 column (1), we found that the increase in search intensity of online education was 14.28. Before the pandemic, the average search intensity of online education was 27.11. Dividing 14.28 by 27.11, we obtained the percentage increase 52.7%. For the confidence interval, using the standard error of the estimated coefficient, which was 0.69, we obtained the 95% CI of the increase in search intensity of online education to be (14.28–1.960.69, 14.28 + 1.960.69), that is, (12.93, 15.63). Dividing the lower and upper bounds by 27.11, we obtained the CI of the percentage change to be (47.7, 57.7%).
10. ^Comparing Figures 1, 2, the COVID-19’s impact on the search intensity of online education looked more persistent compared to its impact on the search intensity of unemployment-related terms. We conjecture that this is because, while employment is to address an immediate need, online education is for a long-term need for the future. Thus, the interest in online education may be more persistent. However, we do not have data on people’s perception of employment or online education, and hence, we leave this to future research.
References
AlMatham, K., AlWadie, A., Kasule, O., AlFadil, S., and Al-Shaya, O. (2022). Assessment of postgraduate online medical education during the COVID-19 pandemic in Saudi Arabia: a cross-sectional study. Adv. Med. Educ. Pract. 13, 1143–1157. doi: 10.2147/AMEP.S370308
Bento, A. I., Nguyen, T., Wing, C., Lozano-Rojas, F., Ahn, Y. Y., and Simon, K. (2020). Evidence from internet search data shows information-seeking responses to news of local COVID-19 cases. Proc. Natl. Acad. Sci. U. S. A. 117, 11220–11222. doi: 10.1073/pnas.2005335117
Bi, B., Shokouhi, M., Kosinski, M., and Graepel, T. (2013). “Inferring the demographics of search users: social data meets search queries.” in Proceedings of The 22nd International Conference on World Wide Web, 131–140.
Boca, G. D. (2021). Factors influencing students’ behavior and attitude towards online education during COVID-19. Sustainability 13:7469. doi: 10.3390/su13137469
Bulvinska, O. I., and Kapralova, I. M. (2022). Use of massive open online courses in the professional development of university teachers. Inform. Technol. Learn. Tools 88, 273–290. doi: 10.33407/itlt.v88i2.4568
Butnaru, G. I., Niță, V., Anichiti, A., and Brînză, G. (2021). The effectiveness of online education during covid 19 pandemic—a comparative analysis between the perceptions of academic students and high school students from Romania. Sustainability 13:5311. doi: 10.3390/su13095311
Carli, L. L. (2020). Women, gender equality and COVID-19. Gender in Management 35, 647–655. doi: 10.1108/GM-07-2020-0236
Carrazana, C. (2020). Coronavirus pandemic creates America’s first female recession amid child care, unemployment woes. USA Today. Available at: doi:https://www.usatoday.com/story/news/politics/elections/2020/08/03/coronavirus-pandemic-sets-women-back-amid-unemployment-child-care-crisis/5573123002/
Caspi, A., Chajut, E., and Saporta, K. (2008). Participation in class and in online discussions: gender differences. Computers Educ. 50, 718–724. doi: 10.1016/j.compedu.2006.08.003
Chakraborty, P., Mittal, P., Gupta, M. S., Yadav, S., and Arora, A. (2021). Opinion of students on online education during the COVID-19 pandemic. Hum. Behav Emerging Technol. 3, 357–365. doi: 10.1002/hbe2.240
Choudhuri, S., and Dilip kumar,. (2022). Online education and its viability during pandemic. J. Pharm. Negat., 13, 1308–1314. doi: 10.47750/pnr.2022.13.S01.157
Christensen, G., Steinmetz, A., Alcorn, B., Bennett, A., Woods, D., and Emanuel, E. (2013). The MOOC phenomenon: who takes massive open online courses and why? Available at SSRN :2350964. doi: 10.2139/ssrn.2350964
Cinar, M., Ekici, M., and Demir, O. (2021). MEDICATION OR BAND-AID? REVISITING UNIVERSITY STUDENTS’READINESS FOR ONLINE EDUCATION. Turk. Online J. Dist. Educ. 22, 176–191. doi: 10.17718/tojde.906848
Cisel, M. (2014). “Analyzing completion rates in the first French xMOOC”. EProceedings of the European MOOC stakeholder summit, 26–32.
Connley, C. (2020a). Coronavirus job losses disproportionately impact women. CNBC. Available at: https://www.cnbc.com/2020/05/14/coronavirus-job-losses-disproportionately-impact-women.html/
Connley, C. (2020b). Despite record job gains in June, new data shows that women are still being left behind. CNBC. Available at: www.cnbc.com/2020/07/08/since-coronavirus-pandemic-started-only-a-third-of-womens-lost-jobs-have-returned.html/
Crawford, J., Butler-Henderson, K., Rudolph, J., Malkawi, B., Glowatz, M., Burton, R., et al. (2020). COVID-19: 20 countries’ higher education intra-period digital pedagogy responses. J. Appl. Learning & Teaching 3, 1–20. doi: 10.37074/jalt.2020.3.1.7
Da, Z., Engelberg, J., and Gao, P. (2011). In search of attention. J. Financ. 66, 1461–1499. doi: 10.1111/j.1540-6261.2011.01679.x
Damani, K., Daltry, R., Jordan, K., Hills, L., and Evans, L. (2022). EdTech for Ugandan girls: affordances of different technologies for girls’ secondary education during the Covid-19 pandemic. Dev. Policy Rev. 40:e12619. doi: 10.1111/dpr.12619
deHaan, E., Shevlin, T., and Thornock, J. (2015). Market (in)attention and the strategic scheduling and timing of earnings announcements. J. Account. Econ. 60, 36–55. doi: 10.1016/j.jacceco.2015.03.003
Dindar, M., Çelik, I., and Muukkonen, H. (2022). WedontWantDistanceEducation: A thematic analysis of higher education students’ social media posts about online education during Covid-19 pandemic Technology. Knowledge Learning 27, 1337–1355. doi: 10.1007/s10758-022-09621-x
Feng, X., Ioan, N., and Li, Y. (2021). Comparison of the effect of online teaching during COVID-19 and pre-pandemic traditional teaching in compulsory education. J. Educ. Res. 114, 307–316. doi: 10.1080/00220671.2021.1930986
Ghebreyesus, T. A. (2020). WHO director-General’s opening remarks at the media briefing on COVID-19. Geneva: World Health Organization.
Gray, J., McPherson, A., and Raffe, D. (2012). Reconstructions of secondary education: Theory, myth and practice since the second world war. London: Routledge.
Han, B. R., Sun, T., Chu, L. Y., and Wu, L. (2022). COVID-19 and E-commerce operations: Evidence from Alibaba. Manuf. Serv. Oper. Manag. 24, 1261–1885. doi: 10.1287/msom.2021.1075
Herr, L., Jih, M. K., Shin, J., Chae, Y. K., Lee, H.-S., Choi, S. C., et al. (2021). The perspective of undergraduate dental students on web-based learning in pediatric dentistry during the COVID-19 pandemic: a Korean multicenter cross-sectional survey. BMC Med. Educ. 21, 1–9. doi: 10.1186/s12909-021-02928-w
Hornstein, A. S. (2013). Corporate capital budgeting and CEO turnover. J. Corp. Finance, 20, 41–58. doi: 10.1016/j.jcorpfin.2012.11.003
Hu, J., Zeng, H.-J., Li, H., Niu, C., and Chen, Z. (2007). “Demographic prediction based on user’s browsing behavior.” in Proceedings of the 16th International Conference on World Wide Web, 151–160.
Impey, C., and Formanek, M. (2021). MOOCS and 100 days of COVID: enrollment surges in massive open online astronomy classes during the coronavirus pandemic. Soc. Sci. Hum. Open 4:100177. doi: 10.1016/j.ssaho.2021.100177
Ivanova, V. (2021). Online training in higher education: an alternative during COVID-19. Strengths and weaknesses of online training. Strategies Policy Sci. Educ. J. 29, 263–275.
Jiang, H., Islam, A. Y. M. A., Gu, X., Spector, J. M., and Chen, S. (2022). Technology-enabled e-learning platforms in Chinese higher education during the pandemic age of COVID-19. SAGE Open 12:215824402210950. doi: 10.1177/21582440221095085
Jiang, S., Schenke, K., Eccles, J. S., Xu, D., and Warschauer, M. (2018). Cross-national comparison of gender differences in the enrollment in and completion of science, technology, engineering, and mathematics massive open online courses. PLoS One 13:e0202463. doi: 10.1371/journal.pone.0202463
Jun, S. P., Yoo, H. S., and Choi, S. (2018). Ten years of research change using Google trends: from the perspective of big data utilizations and applications. Technol. Forecast. Soc. Chang. 130, 69–87. doi: 10.1016/j.techfore.2017.11.009
Kahn, L. B. (2010). The long-term labor market consequences of graduating from college in a bad economy. Labour Econ. 17, 303–316. doi: 10.1016/j.labeco.2009.09.002
Kim, H., and Hanssens, D. M. (2017). Advertising and word-of-mouth effects on pre-launch consumer interest and initial sales of experience products. J. Interact. Mark. 37, 57–74. doi: 10.1016/j.intmar.2016.08.001
Kim, M., Xiong, G., and Kim, K. (2018). Where does pride lead? Corporate managerial hubris and strategic emphasis. J. Acad. Mark. Sci. 46, 537–556. doi: 10.1007/s11747-017-0547-4
Kizilcec, R. F., and Halawa, S. (2015). “Attrition and achievement gaps in online learning”. in L@S ‘15: Proceedings of the Second (2015) ACM Conference on Learning @ Scale, 57–66.
Lee, A. (2020). These states have implemented stay-at-home orders. Here’s what that means for you. CNN. Available at: https://www.cnn.com/2020/03/23/us/coronavirus-which-states-stay-at-home-order-trnd/index.html
Li, K. (2019). MOOC learners’ demographics, self-regulated learning strategy, perceived learning and satisfaction: a structural equation modeling approach. Computers Educ. 132, 16–30. doi: 10.1016/j.compedu.2019.01.003
Liang, Y., Zhang, J., Maxwell, A., Kan, C., Hou, N., Sun, X., et al. (2022). Online education plight and countermeasures for MBBS in Chinese regional medical schools based on the OBE concept during COVID-19 pandemic. Front. Public Health 9:806809. doi: 10.3389/fpubh.2021.806809
Littenberg-Tobias, J., and Reich, J. (2020). Evaluating access, quality, and equity in online learning: a case study of a MOOC-based blended professional degree program. Internet High. Educ. 47:100759. doi: 10.1016/j.iheduc.2020.100759
Liu, H., He, W., Zhao, L., and Hong, J. C. (2021). Gender differences in self-regulated online learning during the COVID-19 lockdown. Front. Psychol. 12:752131. doi: 10.3389/fpsyg.2021.752131
Liu, M., Kang, J., and McKelroy, E. (2015). Examining learners’ perspective of taking a MOOC: reasons, excitement, and perception of usefulness. Educ. Media Int. 52, 129–146. doi: 10.1080/09523987.2015.1053289
Long, H., and Van Dam, A. (2020). U.S. unemployment rate soars to 14.7%, worst since depression era. In The Washington Post.
McGivney, V. (2004). Understanding persistence in adult learning. Int. J. Phytoremediation 19, 33–46. doi: 10.1080/0268051042000177836
Morris, N. P., Morris, N. P., Hotchkiss, S., and Swinnerton, B. (2015). “Can demographic information predict MOOC learner outcomes?”. Proceedings of the European MOOC Stakeholder. eMOOCs Conference 2015.
Müller, T. (2008). Persistence of women in online degree-completion programs. Int. Rev. Res. Open Distance Learn. 9. doi: 10.19173/irrodl.v9i2.455
Ng, C. (2019). Shifting the focus from motivated learners to motivating distributed environments: a review of 40 years of published motivation research in distance education. Distance Educ. 40:1892. doi: 10.1080/01587919.2019.1681892
Ponnaiah, M., Bhatnagar, T., Ganeshkumar, P., Bhar, D., Elumalai, R., Vijayageetha, M., et al. (2022). Design and implementation challenges of massive open online course on research methods for Indian medical postgraduates and teachers–descriptive analysis of inaugural cycle. BMC Med. Educ. 22:369. doi: 10.1186/s12909-022-03423-6
Radha, R., Mahalakshmi, K., Kumar, V. S., and Saravanakumar, A. R. (2020). E-learning during lockdown of Covid-19 pandemic: a global perspective. Int. J. Control Automation 13
Ribeiro-Navarrete, S., Saura, J. R., and Palacios-Marqués, D. (2021). Towards a new era of mass data collection: assessing pandemic surveillance technologies to preserve user privacy. Technol. Forecast. Soc. Chang. 167:120681. doi: 10.1016/j.techfore.2021.120681
Saikat, S., Dhillon, J. S., Alias, R., and Fatima, M. A. (2022). C19MOOC: a remote learning reference framework for science and technology education. Informatics 9:53. doi: 10.3390/informatics9030053
Saura, J. R., Ribeiro-Soriano, D., and Saldaña, P. Z. (2022). Exploring the challenges of remote work on twitter users’ sentiments: from digital technology development to a post-pandemic era. J. Bus. Res. 142, 242–254. doi: 10.1016/j.jbusres.2021.12.052
Shah, D. (2020). By the numbers: MOOCs during the pandemic. Available at: https://www.classcentral.com/report/mooc-stats-pandemic/ (Accessed August 16, 2020).
Shi, L., Cristea, A. I., Toda, A. M., and Oliveira, W.. Revealing the hidden patterns: a comparative study on profiling subpopulations of MOOC students. Proceedings of the 28th international conference on information systems development: Information systems beyond 2020 (2020), ISD.
Shrader, S., Wu, M., Owens, D., and Ana, K. (2016). Massive open online courses (MOOCS): participant activity, demographics, and satisfaction. Online Learn. J. 20, 199–216. doi: 10.24059/olj.v20i2.596
Singh, A., and Sharma, A. (2021). Acceptance of MOOCs as an alternative for internship for management students during COVID-19 pandemic: an Indian perspective. Int. J. Educ. Manag. 35, 1231–1244. doi: 10.1108/IJEM-03-2021-0085
Sushko, V. A., and Pronchev, G. B. (2021). Online learning in the context of pandemic in Russia. Revista Romaneasca Pentru Educatie Multidimensionala 13, 01–17. doi: 10.18662/rrem/13.2/407
Thompson, E. R., and Phua, F. T. T. (2005). Reliability among Senior Managers of the Marlowe-Crowne Short-Form Social Desirability Scale. J. Bus. Psychol. 19, 541–554. doi: 10.1007/s10869-005-4524-4
Ulukol, B. (2022). Online education and effects during Covid-19 pandemic. BRAIN. Broad Res. Artificial Intelligence and Neuroscience 13, 534–545. doi: 10.18662/brain/13.1/298
USA Today. (2021). COVID-19 restrictions. USA Today. Available at: https://www.usatoday.com/storytelling/coronavirus-reopening-america-map/
Veletsianos, G., Kimmons, R., Larsen, R., and Rogers, J. (2021). Temporal flexibility, gender, and online learning completion. Distance Educ. 42, 22–36. doi: 10.1080/01587919.2020.1869523
Wang, K. (2022). Statistics and analysis of effective data on online teaching of college English audiovisual teaching. Math. Probl. Eng. 2022, 1–7. doi: 10.1155/2022/8956891
Keywords: COVID-19, internet search data, education, gender differences, age groups
Citation: Wang P, Jiang L and Yang J (2023) Internet search data showed increased interest in supplementary online education during the COVID-19 pandemic, with females showing a greater increase. Front. Educ. 8:1142689. doi: 10.3389/feduc.2023.1142689
Edited by:
Casper Zhang, The University of Hong Kong, Hong Kong SAR, ChinaReviewed by:
Constantin Aurelian Ionescu, Valahia University of Târgoviște, RomaniaAlexandra Hosszu, University of Bucharest, Romania
Copyright © 2023 Wang, Jiang and Yang. This is an open-access article distributed under the terms of the Creative Commons Attribution License (CC BY). The use, distribution or reproduction in other forums is permitted, provided the original author(s) and the copyright owner(s) are credited and that the original publication in this journal is cited, in accordance with accepted academic practice. No use, distribution or reproduction is permitted which does not comply with these terms.
*Correspondence: Li Jiang, lijiang1@gwu.edu
†These authors have contributed equally to this work