- 1Department of Individual Differences and Psychodiagnostics, Saarland University, Saarbrucken, Germany
- 2ITB Consulting GmbH, Bonn, Germany
- 3Department of Biochemistry and Molecular Cell Biology, University Medical Center Hamburg-Eppendorf (UKE), Hamburg, Germany
- 4Faculty of Medicine, University Heidelberg, Heidelberg, Germany
- 5Department of Individual Differences and Psychodiagnostics, Greifswald University, Greifswald, Germany
Standardized ability tests that are associated with intelligence are often used for student selection. In Germany two different admission procedures to select students for medical studies are used simultaneously; the TMS and the HAM-Nat. Due to this simultaneous use of both a detailed analysis of the construct validity is mandatory. Therefore, the aim of the study is the construct validation of both selection procedures by using data of 4,528 participants (Mage = 20.42, SD = 2.74) who took part in a preparation study under low stakes conditions. This study compares different model specifications within the correlational structure of intelligence factors as well as analysis the g-factor consistency of the admission tests. Results reveal that all subtests are correlated substantially. Furthermore, confirmatory factor analyses demonstrate that both admission tests (and their subtests) are related to g as well as to a further test-specific-factor. Therefore, from a psychometric point of view, the simultaneous use of both student selection procedures appears to be legitimate.
1. Introduction
In general, student selection procedures are usually used when there are more applicants than there are study places. This is especially the case for some study courses, like medicine in Germany.
In this context, specific aptitude tests measuring cognitive abilities and/or specific knowledge are often used as a selection criterion since many years. Numerous studies indicate that cognitive abilities predict school performance (Roth et al., 2015), educational attainment (Deary et al., 2007), training success, job performance (Schmidt and Hunter, 1998; Hülsheger et al., 2007; Kramer, 2009), and success in university studies (Hell et al., 2007; Schult et al., 2019). In general intelligence can be defined as a broad cognitive ability that includes the understanding of complex ideas, adaptability to environmental conditions, learning from experience, and problem solving through analysis (cf. Neisser et al., 1996). Concerning the construct validity of intelligence Spearman (1904) already noted that different indicators of cognitive ability usually show positive intercorrelations (i.e., positive manifold). This led him to the assumption that all intelligence tests are determined by one general factor (g) and that g in turn can be assessed by every intelligence test (i.e., indifference of indicators). In current higher-order factor models g is regarded as a factor standing at the apex of a hierarchy of intercorrelated subordinate group-factors (cf. Jensen, 1998; McGrew, 2009) and there is considerable evidence that different intelligence tests tap the same general latent factor (Johnson et al., 2004, 2008). Going beyond classical higher-order models, recent studies (Gignac, 2006, 2008; Brunner et al., 2012; Valerius and Sparfeldt, 2014) argue that they can be extended by nested-factors that account for systematic residual variance not covered by g. The results of Valerius and Sparfeldt (2014) for example show that the fit of a nested-factor model was relatively better than a higher-order or general-factor model.
Due to the federal structure of the educational system in Germany, universities are sovereign to decide about the selection criteria for their students. The current practice in medical studies is that universities use one of two tests explicitly developed for the selection of medical students (Schwibbe et al., 2018): the Hamburger Naturwissenschaftstest (HAM-Nat; en. Hamburg Natural Science Test; Hissbach et al., 2011) and the Test für medizinische Studiengänge (TMS; en. Test for Medical Studies; Kadmon et al., 2012). Within the scope of a nation-wide research project (“Studierendenauswahlverbund stav”; en. student admission research network), the existing tests as well as three additional reasoning tests, which were developed within the stav, were examined under low- and high stakes conditions. In 2020, the HAM-Nat consisted of four different scales measuring natural science knowledge as well as numerical, verbal, and figural reasoning. Those three reasoning scales, which measure fluid intelligence, were added to the original HAM-Nat in order to enable a broader measurement of cognitive abilities beside the crystallized intelligence. Overall, 2,234 people participated 2020 in the 2:15 h session at three universities. The TMS consists of 8 specific modules measuring different cognitive abilities and has a total working time of 5:07 h. It was used by 37 universities and had 37,092 applications in the year 2022.
Previous studies showed that the test scores from both possess predictive validity and the included items suitable psychometric properties in terms of internal consistency (Hell et al., 2007; Hissbach et al., 2011; Kadmon et al., 2012; Werwick et al., 2015; Schult et al., 2019). As all of these studies exclusively deal with only one of the tests, there is currently no evidence concerning the construct validity between their test scores. This can be regarded as a research gap for three reasons: (1) With respect to the comparability of the selection procedures it would generally be important to know if different universities apply different standards. (2) If both tests assess the exact same construct, it would be more economical to only use one test. (3) Nested factors that are specific for each of the two tests could explain variance of study aptitude that is not covered by the other one. A combined test could therefore allow a better prediction of study success than both tests alone.
This study is a first step to close this research gap. We are able to provide first evidence concerning the construct validity between the scores of two tests by using a large sample of applicants that completed a short version of the Ham-Nat science test plus the three reasoning tests (numerical, verbal, and figural) from the stav-project,1 as well as four of eight subtests from the TMS. In the following, we refer to the four TMS-modules as “TMS” and to the combination of HAM-Nat and the three reasoning subtests as “HAM-Nat.” In doing so, we compared the following models also presented in Figure 1:
• g-model: In a first step we analysed the classical g-factor model in the sense of Spearman (1904). Here, all subtests load on a single general factor and all other variance is regarded as measurement error.
• HO-model: Taking higher-order factor models into account (Jensen, 1998; McGrew, 2009) we inspected a model with separate group factors which represent the shared variance of the subtests within the HAM-Nat or the TMS and give rise to a superordinate g-factor.
• NF-model: The idea of a nested-factor structure (Gignac, 2006, 2008; Brunner et al., 2012; Valerius and Sparfeldt, 2014) was evaluated by a model in which variance not bound by g is explained by specific factors within the HAM-Nat or the TMS.
• TS-model: Following Johnson et al. (2004, 2008) we analysed a test-specific model with separate g-factors for the subtests of the HAM-Nat and the TMS. Shared variance between the tests is represented by correlation between the test-specific factors.
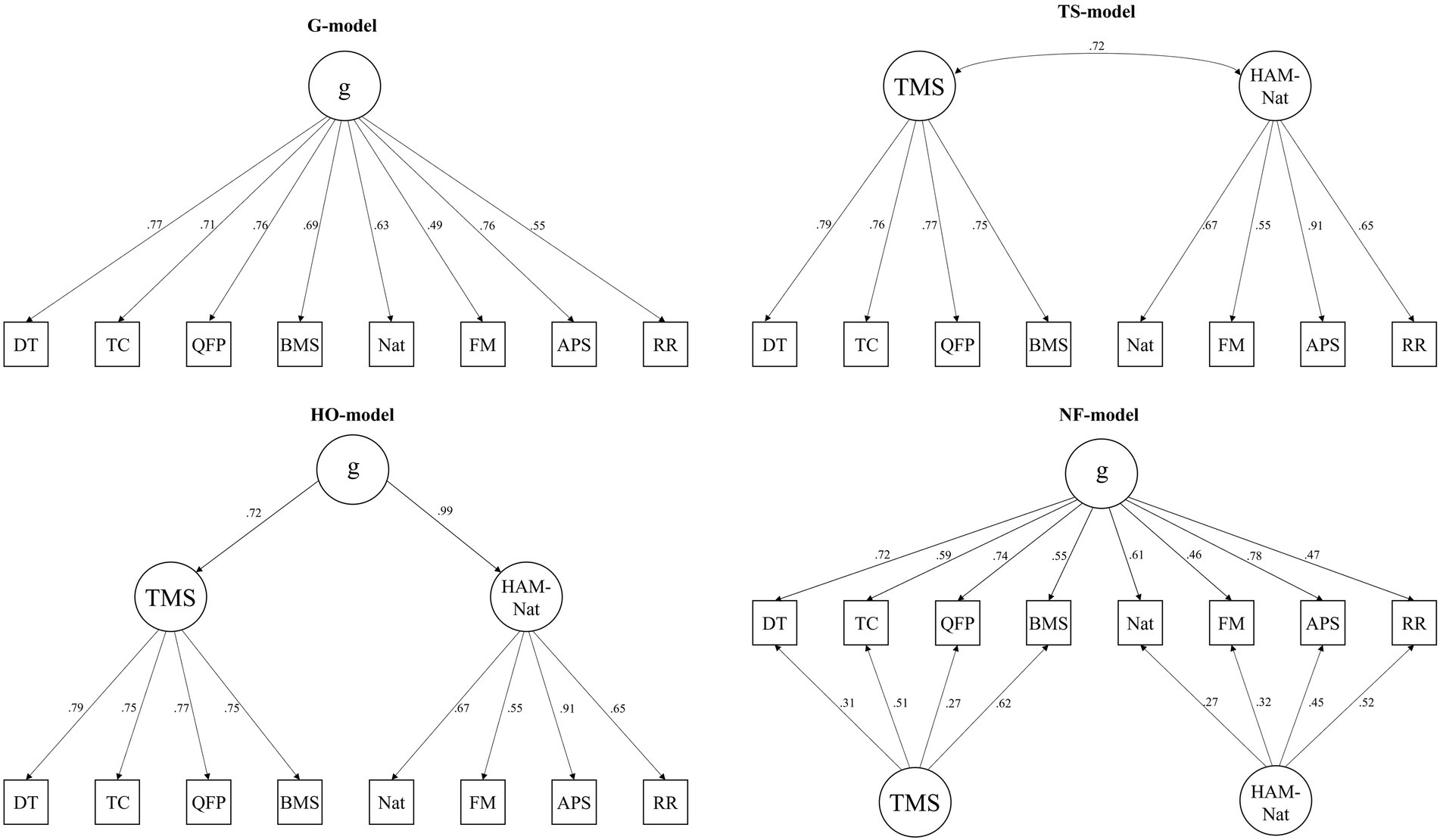
Figure 1. Confirmatory factor analyses for alternative models estimating general and specific factors of both admission tests.
2. Methods
2.1. Sample and procedure
Table 1 shows the demographic details for the total sample as well as for the samples in the subtests. The total sample consisted of 4,537 participants with a mean age of 20.42 years (SD = 2.74, 16 ≤ age ≤ 56). All participants were registered for the TMS high stakes test carried out in 2021. Respondents received a link and completed a practice online test at home in an unsupervised setting.
The test preparation study consisted of eight different subtests, all of which are used for admission tests in medicine (four subtests of the eight TMS-scales, all four subtests of the HAM-Nat). While the HAM-Nat subtests were presented in random order, the TMS subtests were presented en-bloc in the same order as under high stakes conditions. The reason for this inconsistent approach is that we offered a cost-free preparation study to all participants registered to the TMS in 2021. The incentive to participate in our study was a practice condition as close as possible to the original test format. Therefore, the order of the individual subtests of this selection test was standardized identically to that of the real student selection test. However, in order to meet our research requirements and the state-of-the-art of randomisation, we decided to present the single HAM-Nat scales in random order. In contrast to the high stakes conditions, all subtests were presented in an online version here. Respondents received detailed feedback of their results as a further incentive.
2.2. Materials
Respondents completed eight different subtests. Thereof, four tests (DT, TC, QFP, BMS) are subtests of the TMS, and the remaining four (HST, FM, APS, RR) of the HAM-Nat. All tests have in common that they are presented as multiple-choice questions.
Diagrams and tables (DT): Respondents are provided with data presented in tables or diagrams (e.g., a figure showing the relationship between blood clotting time and the number of platelets in patients with different diseases and therapies) and have to analyse them to infer specific information not directly presented in the material (e.g., find out whether the blood clotting time can be normal even if the number of platelets is severely reduced).
Text comprehension (TC): This subtest contains four longer scientific texts (e.g., about growth hormones, related control loops and feedback mechanisms) and six questions for each text concerning specific information that can be derived (e.g., An adult patient has an increased concentration of GH. According to the text, what factors can be the cause of it?). All questions can be answered without any prior knowledge.
Quantitative and formal problems (QFP): In this subtest respondents receive descriptions of complex arithmetic relations in a biomedical context and have to understand them in order to answer related questions (e.g., the formula of the energy charge E describing the energetic situation of a cell is explained. Then it must be calculated how the energy charge of a cell with certain proportions of ATP, ADP and AMP changes when the available ADP is converted into AMP).
Basic medical and scientific understanding (BMS): The aim of this subtest is to assess the ability to extract complex and demanding information from a text. Respondents receive texts dealing with medical and scientific topics (e.g., transport mechanisms for small ions) and have to decide which of several statements can be derived from the text (e.g., if a certain pharmaceutical agent inhibits transport of potassium ions into the extracellular space). In contrast to TC-tasks, the presented texts are shorter, and only one question per text has to be answered. Again, no prior knowledge is necessary to answer the questions.
HAM-Nat science test (Nat): The questions of this subtest deal with school knowledge in biology, chemistry, physics, and mathematics at the upper secondary school level relevant to the medical field. The questions can only be solved by using prior knowledge not included in the question (e.g., calculating the molar mass of acetonic acid when only the molecular formula and the molar masses are given).
Figural matrices (FM): The items of this subtest are 3 × 3 matrices filled with geometric symbols that follow certain design rules (e.g., symbols in the first and second cell of a row add up in the third cell). The last cell of the matrix is left empty, and respondents have to select the symbols which logically complete it.
Arithmetic problem solving (APS): In this subtest respondents receive short descriptions of arithmetic relations (e.g., After a price reduction of 20 percent, product A costs four times as much as product B, which costs 20 euros. How much did product A cost before the price reduction?).
Relational reasoning (RR): Respondents receive a set of premises (e.g., City A is larger than city C; City B is smallest; City D is smaller than city A) have to integrate them logically to find answers to corresponding questions (e.g., Which is the biggest city?).
2.3. Statistical analysis
All statistical analyses were carried out using R version 3.5.1. We computed Cronbach’s alpha (α), item difficulties (p) as well as the part-whole corrected item-total correlations (rit) for each of the eight subtests. Furthermore, all intercorrelations between the mean scores in the subtests were calculated. The construct validity models presented in the introduction were tested by conducting confirmatory factor analyses in the R package lavaan (Rosseel, 2012) using the maximum likelihood estimator. We calculated the χ2 goodness of fit statistic, the root mean square error of approximation (RMSEA), the standardized root mean square residual (SRMR), the comparative fit index (CFI), and the Tucker-Lewis Index (TLI). Following (Hu and Bentler, 1999) CFI values greater than 0.95, TLI values greater than 0.95, RMSEA values close to 0.06 and SRMR values smaller than 0.08 were regarded as indicators of good model fits. Akaike’s Information Criterion (AIC) and the Bayesian Information Criterion (BIC) were used to compare the different construct validity models, with lower values indicating a better fit (Schwarz, 1978). A difference of the RMSEAs between two models (ΔRMSEA) greater than 0.015 was regarded as an additional indicator of the difference of model fits (Chen, 2007).
3. Results
3.1. Item statistics, internal consistency, correlations
The descriptive statistics for the item difficulties and item-total correlations of the subtests as well as the internal consistencies can be found in Table 2. It can be seen that items of all subtests cover a considerably wide range of difficulties and that the item-total correlations as well as the internal consistencies can be described as acceptable.
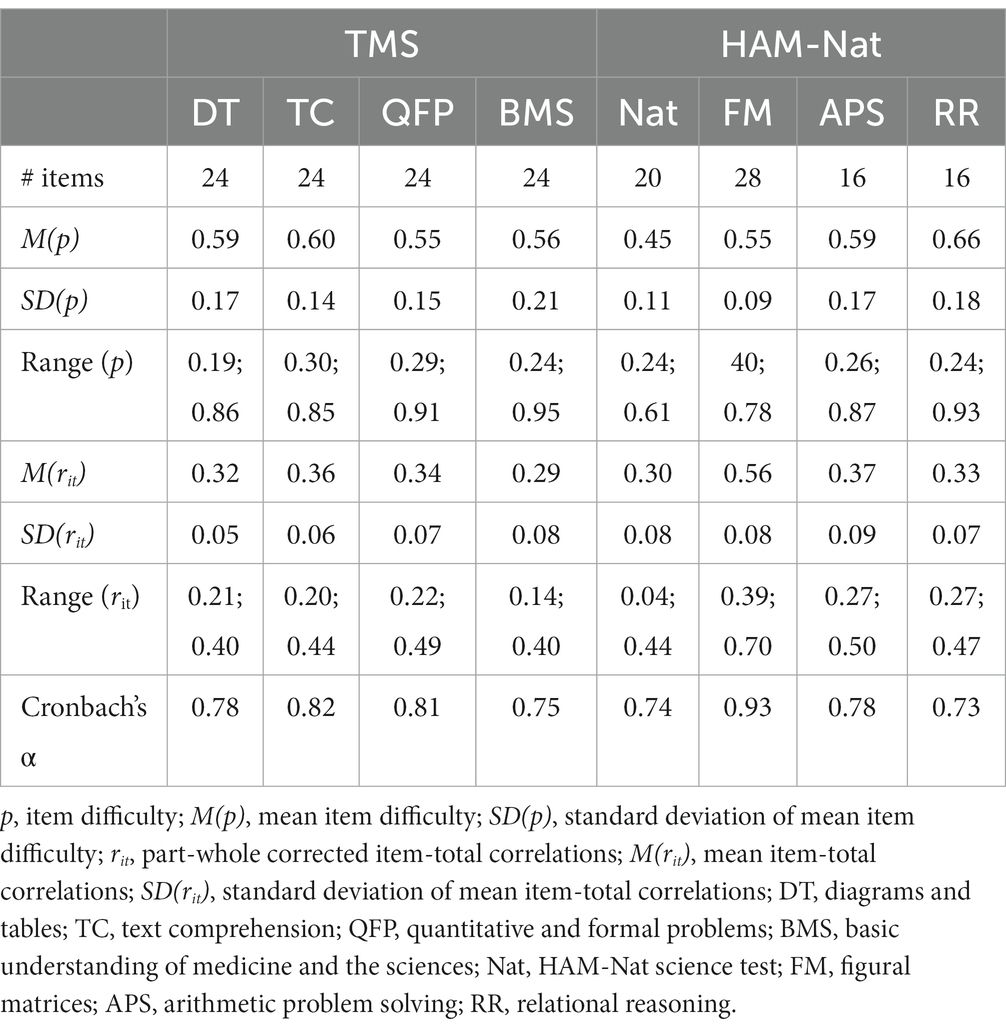
Table 2. Number of items of all test parts as well as Cronbach’s alpha, item difficulties and item-total correlations.
The correlations between the sum scores of the subtests are presented in Table 3. All subtests show substantial and significant correlations with the other ones (0.25 ≤ r ≤ 0.67). The highest mean correlation can be found among the subtests of the TMS (M(r) = 0.53) while lower mean correlations can be found among the HAM-Nat subtests (M(r) = 0.46) and between the HAM-Nat and the TMS subtests (M(r) = 0.38).
3.2. Results of the confirmatory factor analyses
The results of the confirmatory factor analyses are presented in Figure 1. All factor loadings and (if applicable) latent correlations were significant and substantial. The fit indices of the four tested models as well as the McDonald’s Omega (ω) of their latent variables are shown in Table 4. The χ2 goodness of fit statistic was significant for all of the models. The fit indices (CFI, TLI, RMSEA, SRMR) for the g-model and HO-model indicate model misfit while they predominantly did not exceed the cut-offs for the other two models. The NF-model, on the other hand, had an excellent fit. A comparison of the information criteria (AIC, BIC) reveals that they were lowest in the NF-model, followed by the TS-, the HO and the G-model. The same pattern is revealed by inspecting the ΔRMSEA (see Table 4). Thus, it seems that both tests are indicators for a general intelligence factor. Intelligence can be inferred with the help of both tests and construct validity therefore exists.
4. Discussion
The goal of this study was to provide first insights concerning the construct validity of the scores from the existing admission tests developed for the selection of medical students in Germany.
Our findings are based on a large sample of respondents that completed a broad and representative set of subtests included in the two tests. The basic psychometric properties of the subtests (difficulty, item total correlation, internal consistency) demonstrate the suitability of the database for further analyses. The inspection of the intercorrelations between the sum scores of the subtests clearly shows a positive manifold. Besides this, the correlations of the subtests within the HAM-Nat and the TMS were higher than the correlations between them. The confirmatory factor analyses reveal a more differentiated picture. For the models comprising only a single general factor (g-model) or a higher-order structure in which test-specific group-factors give rise to a general factor (HO-model) we found fit indices that were considerably below the respective cut-offs. The models containing test-specific factors that are independent from a general factor (TS-model, NF-model) showed better fit indices. This is in line with our results concerning the information criteria which would also favour the models with independent test-specific factors.
The results of our study are in line with the previous literature dealing with the construct validity of intelligence. The positive manifold of the subtests of the HAM-Nat and the TMS show that they share a substantial amount of variance. This corresponds with Spearman (1904) idea of g and higher-order factor models (e.g., Jensen, 1998; McGrew, 2009) that conceptualize a general intellectual ability that is independent from the test used to assess it. This also shows that a considerable amount of systematic variance exists that is not shared by the two tests. Therefore, our results support the recent literature that found evidence for test-specific ability factors beyond g (e.g., Brunner et al., 2012; Valerius and Sparfeldt, 2014).
It should be noted that this study was conducted under low stakes conditions in an unsupervised setting. It is therefore possible that participants spent more time on each subtest, used prohibited tools (e.g., calculators, taking notes) or had a lower overall motivation than under a high stakes condition. It is therefore possible that both tests represent the construct even better under high-stakes conditions, since the participants work in a more focused manner, which might result in a higher validity of the test score due to reduced error variance. On the other hand, people are better prepared under high-stakes conditions. It is precisely this preparation that could have an influence on the test result and lead to an increased error variance under high-stakes conditions. The higher motivation among participants can also influence the result (Levacher et al., 2021). To account for this possibility, an attempt was made to increase the motivation of the participants, as the study provided an opportunity to prepare for the high-stakes test and the results were re-ported back as an incentive. Additionally, it is worth noting, that all participants for this study were chosen from the database of people who were registered for the TMS. Therefore, they may not have been prepared for the HAM-Nat, potentially affecting their motivation to complete this part of the assessment. For this reason, it was decided in advance to present only very easy items of the Nat, which may also have had an influence on the results. With regard to the TMS, comparability to the full TMS may be impaired, as only four of the eight subscales were administered and the items used had been published before in preparation books, so that some participants may have known them already. With respect to the current selection practice for medical students in Germany it is noteworthy that the large amount of shared variance between the two tests shows that universities using either the HAM-Nat or the TMS do not apply entirely different standards. Nevertheless, neglecting the specific ability aspects not shared by the two tests could result in a loss of valuable information. Given this fact it could be reasonable to combine both tests or at least parts of them.
To achieve this, in a further study the specific variances should be examined in more detail to consider predictive validity. For this purpose, a regression with the study success as criterion and the variance of the g-factor as well as the two specific variances (HAM-Nat and TMS) as predictors should be estimated. In this way, it can be analysed whether, in addition to g, test-specific variance predicts study success, or whether the test-specific variance merely reflects methodological variance.
5. Conclusion
Taken together, both subtest groups under study (TMS and HAM-Nat) seem to measure a very similar cognitive ability, despite different theoretical concepts. It can be assumed, that both subtest groups are related to g as well as to a further test-specific-factor. Even if both specific-factors are correlated, specific-non-shared parts remain. Therefore, the non-shared variance of each test should be further analysed by including university grades in our models to investigate their incremental validity. Referring to our findings, the parallel use of both procedures for the selection of students seems to be legitimate from a test-theoretical point of view.
Data availability statement
The data analyzed in this study is subject to the following licenses/restrictions: Due to data privacy restrictions of the stav, data cannot be shared with external researchers. Requests to access these datasets should be directed to a29udGFrdEBwcm9qZWt0LXN0YXYuZGU=.
Ethics statement
Ethical review and approval was not required for the study on human participants in accordance with the local legislation and institutional requirements. Written informed consent from the participants' legal guardian/next of kin was not required to participate in this study in accordance with the national legislation and the institutional requirements.
Author contributions
JL: conceptualization, data curation, formal analysis, methodology, validation, visualization, and writing – original draft preparation. MK and FS: writing – review and editing. SS and ME: conceptualization (online preparation study) and writing – review and editing. JH: conceptualization, project administration, and writing – review and editing. NB: conceptualization, funding acquisition, project administration, supervision, and writing – review and editing. All authors contributed to the article and approved the submitted version.
Funding
This work was partly funded by the Federal Republic of Germany, Federal Ministry of Education and Research (funding code: 01GK1801A).
Acknowledgments
We would like to thank all project partners of the stav who were involved in the test administration. We acknowledge support by the Deutsche Forschungsgemeinschaft (DFG, German Research Foundation) and Saarland University within the “Open Access Publication Funding” program.
Conflict of interest
SS is partner of the ITB Consulting GmbH, the organization developing the TMS.
The remaining authors declare that the research was conducted in the absence of any commercial or financial relationships that could be construed as a potential conflict of interest.
Publisher’s note
All claims expressed in this article are solely those of the authors and do not necessarily represent those of their affiliated organizations, or those of the publisher, the editors and the reviewers. Any product that may be evaluated in this article, or claim that may be made by its manufacturer, is not guaranteed or endorsed by the publisher.
Footnotes
^The stav-project investigates different subtests within the framework of the Studierendenauswahl-Verbund (stav; en. Student Selection Network). It investigates the HAM-Nat, to which three subtests were added, and the TMS. One aim of stav is to evaluate the different subtests in order to scientifically find out how the current student admission procedures in medicine could be improved.
References
Brunner, M., Nagy, G., and Wilhelm, O. (2012). A tutorial on hierarchically structured constructs. J. Pers. 80, 796–846. doi: 10.1111/j.1467-6494.2011.00749.x
Chen, F. F. (2007). Sensitivity of goodness of fit indexes to lack of measurement invariance. Struct. Equ. Model. Multidiscip. J. 14, 464–504. doi: 10.1080/10705510701301834
Deary, I. J., Strand, S., Smith, P., and Fernandes, C. (2007). Intelligence and educational achievement. Intelligence 35, 13–21. doi: 10.1016/j.intell.2006.02.001
Gignac, G. E. (2006). A confirmatory examination of the factor structure of the multidimensional aptitude battery: contrasting oblique, higher order, and nested factor models. Educ. Psychol. Meas. 66, 136–145. doi: 10.1177/0013164405278568
Gignac, G. E. (2008). Higher-order models versus direct hierarchical models: g as superordinate or breadth factor? Psychol. Sci. Q. 50, 21–43.
Hell, B., Trapmann, S., and Schuler, H. (2007). Eine metaanalyse der validität von fachspezifischen studierfähigkeitstests im deutschsprachigen raum. Empirische Pädagogik 21, 251–270.
Hissbach, J. C., Klusmann, D., and Hampe, W. (2011). Dimensionality and predictive validity of the HAM-Nat, a test of natural sciences for medical school admission. BMC Med. Educ. 11:83. doi: 10.1186/1472-6920-11-83
Hu, L., and Bentler, P. M. (1999). Cutoff criteria for fit indexes in covariance structure analysis: conventional criteria versus new alternatives. Struct. Equ. Model. Multidiscip. J. 6, 1–55. doi: 10.1080/10705519909540118
Hülsheger, U. R., Maier, G. W., and Stumpp, T. (2007). Validity of general mental ability for the prediction of job performance and training success in Germany: a meta-analysis1. Int. J. Sel. Assess. 15, 3–18. doi: 10.1111/j.1468-2389.2007.00363.x
Jensen, A. R. (1998). The g Factor: The Science of Mental Ability. Praeger Publishers/Greenwood Publishing Group Westport.
Johnson, W., Bouchard, T. J., Krueger, R. F., McGue, M., and Gottesman, I. I. (2004). Just one g: consistent results from three test batteries. Intelligence 32, 95–107. doi: 10.1016/S0160-2896(03)00062-X
Johnson, W., Te Nijenhuis, J., and Bouchard, T. J. (2008). Still just 1 g: consistent results from five test batteries. Intelligence 36, 81–95. doi: 10.1016/j.intell.2007.06.001
Kadmon, G., Kirchner, A., Duelli, R., Resch, F., and Kadmon, M. (2012). Warum der test für medizinische studiengänge (TMS)? Z. Evid. Fortbild. Qual. Gesundhwes. 106, 125–130. doi: 10.1016/j.zefq.2011.07.022
Kramer, J. (2009). Allgemeine intelligenz und beruflicher erfolg in deutschland. Psychol. Rundsch. 60, 82–98. doi: 10.1026/0033-3042.60.2.82
Levacher, J., Koch, M., Hissbach, J., Spinath, F. M., and Becker, N. (2021). You can play the game without knowing the rules—but you’re better off knowing them: the influence of rule knowledge on figural matrices tests. Eur. J. Psychol. Assess. 38, 15–23. doi: 10.1027/1015-5759/a000637
McGrew, K. S. (2009). CHC theory and the human cognitive abilities project: standing on the shoulders of the giants of psychometric intelligence research. Intelligence 37, 1–10. doi: 10.1016/j.intell.2008.08.004
Neisser, U., Boodoo, G., Bouchard, T. J. Jr., Boykin, A. W., Brody, N., Ceci, S. J., et al. (1996). Intelligence: knowns and unknowns. Am. Psychol. 51, 77–101. doi: 10.1037/0003-066X.51.2.77
Rosseel, Y. (2012). Lavaan: an R package for structural equation modeling and more version 0.5-12 (BETA). J. Stat. Softw. 48, 1–36. doi: 10.18637/jss.v048.i02
Roth, B., Becker, N., Romeyke, S., Schäfer, S., Domnick, F., and Spinath, F. M. (2015). Intelligence and school grades: a meta-analysis. Intelligence 53, 118–137. doi: 10.1016/j.intell.2015.09.002
Schmidt, F. L., and Hunter, J. E. (1998). The validity and utility of selection methods in personnel psychology: practical and theoretical implications of 85 years of research findings. Psychol. Bull. 124, 262–274. doi: 10.1037/0033-2909.124.2.262
Schult, J., Hofmann, A., and Stegt, S. J. (2019). Leisten fachspezifische Studierfähigkeitstests im deutschsprachigen Raum eine valide Studienerfolgsprognose? Z. Entwicklungspsychol. Padagog. Psychol. 51, 16–30. doi: 10.1026/0049-8637/a000204
Schwarz, G. (1978). Estimating the dimension of a model. Ann. Stat. 6, 461–464. doi: 10.1214/aos/1176344136
Schwibbe, A., Lackamp, J., Knorr, M., Hissbach, J., Kadmon, M., and Hampe, W. (2018). Medizinstudierendenauswahl in deutschland. Bundesgesundheitsbl. Gesundheitsforsch. Gesundheitsschutz 61, 178–186. doi: 10.1007/s00103-017-2670-2
Spearman, C. (1904). “General intelligence,” objectively determined and measured. Am. J. Psychol. 15, 201–293. doi: 10.2307/1412107
Valerius, S., and Sparfeldt, J. R. (2014). Consistent g- as well as consistent verbal-, numerical- and figural-factors in nested factor models? Confirmatory factor analyses using three test batteries. Intelligence 44, 120–133. doi: 10.1016/j.intell.2014.04.003
Keywords: student selection, cognitive ability, construct validity, psychometrics, g-factor
Citation: Levacher J, Koch M, Stegt SJ, Hissbach J, Spinath FM, Escher M and Becker N (2023) The construct validity of the main student selection tests for medical studies in Germany. Front. Educ. 8:1120129. doi: 10.3389/feduc.2023.1120129
Edited by:
Michael Sailer, Ludwig Maximilian University of Munich, GermanyReviewed by:
Matthias Ziegler, Humboldt University of Berlin, GermanyFlorian G. Hartmann, Paris Lodron University Salzburg, Austria
Copyright © 2023 Levacher, Koch, Stegt, Hissbach, Spinath, Escher and Becker. This is an open-access article distributed under the terms of the Creative Commons Attribution License (CC BY). The use, distribution or reproduction in other forums is permitted, provided the original author(s) and the copyright owner(s) are credited and that the original publication in this journal is cited, in accordance with accepted academic practice. No use, distribution or reproduction is permitted which does not comply with these terms.
*Correspondence: Julie Levacher, ✉ anVsaWUubGV2YWNoZXJAdW5pLXNhYXJsYW5kLmRl